DECO: Pure convolutional Query-Based detector surpasses DETR!
Title: DECO: Query-Based End-to-End Object Detection with ConvNets
Paper: https://arxiv.org/pdf/2312.13735 .pdf
Source code: https://github.com/xinghaochen/DECO
Original text: https://zhuanlan.zhihu.com/p/686011746@王云河
Introduction
After the introduction of Detection Transformer (DETR), there was a craze in the field of object detection, and many subsequent studies improved the original DETR in terms of accuracy and speed. However, the discussion continues as to whether Transformers can completely dominate the visual field. Some studies such as ConvNeXt and RepLKNet show that CNN structures still have great potential in the field of vision.
Our work explores how to use the pure convolution architecture to obtain a DETR-like framework detector with high performance. In tribute to DETR, we call our approach DECO
(Detection ConvNets). Using a similar structural setting to DETR and using different Backbones, DECO achieved 38.6% and 40.8% AP on COCO and 35 FPS and 28 FPS on V100, achieving better performance than DETR. Paired with modules such as multi-scale features similar to RT-DETR, DECO achieved a speed of 47.8% AP and 34 FPS. The overall performance has good advantages compared with many DETR improvement methods.
Method
Network architecture
The main feature of DETR is to use the structure of Transformer Encoder-Decoder to process an input image using A set of Query interacts with image features and can directly output a specified number of detection frames, thus eliminating dependence on post-processing operations such as NMS. The overall architecture of DECO we proposed is similar to DETR. It also includes Backbone for image feature extraction, an Encoder-Decoder structure to interact with Query, and finally outputs a specific number of detection results. The only difference is that DECO's Encoder and Decoder are purely convolutional structures, so DECO is a Query-Based end-to-end detector composed of pure convolution.
Encoder
DETR's Encoder structure replacement is relatively straightforward. We choose to use 4 ConvNeXt Blocks to form the Encoder structure. Specifically, each layer of the Encoder is implemented by stacking a 7x7 depth convolution, a LayerNorm layer, a 1x1 convolution, a GELU activation function and another 1x1 convolution. In addition, in DETR, because the Transformer architecture has permutation invariance to the input, positional encoding needs to be added to the input of each layer of encoder, but for the Encoder composed of convolutions, there is no need to add any positional encoding
Decoder
In comparison, the replacement of Decoder is much more complicated. The main function of the Decoder is to fully interact with image features and Query, so that Query can fully perceive the image feature information and thereby predict the coordinates and categories of targets in the image. The Decoder mainly includes two inputs: the feature output of the Encoder and a set of learnable query vectors (Query). We divide the main structure of Decoder into two modules: Self-Interaction Module (SIM) and Cross-Interaction Module (CIM).
Here, the SIM module mainly integrates the output of the Query and the upper Decoder layer. This part of the structure can be composed of several convolutional layers, using 9x9 depthwise convolution and 1x1 convolution performs information interaction in the spatial dimension and channel dimension respectively, fully obtaining the required target information and sending it to the subsequent CIM module for further target detection feature extraction. Query is a set of randomly initialized vectors. This number determines the number of detection frames finally output by the detector. Its specific value can be adjusted according to actual needs. For DECO, because all structures are composed of convolutions, we turn Query into two dimensions. For example, 100 Queries can become 10x10 dimensions.
The main function of the CIM module is to allow image features and Query to fully interact, so that Query can fully perceive the image feature information, thereby predicting the coordinates and categories of targets in the image. For the Transformer structure, it is easy to achieve this goal by using the cross attention mechanism, but for the convolution structure, how to fully interact with the two features is the biggest difficulty.
To fuse the global features of the SIM output and encoder output of different sizes, we must first spatially align the two and then fuse them. First, we perform nearest neighbor upsampling on the SIM output:
Make the upsampled features have the same size as the global features output by the Encoder, then fuse the upsampled features with the global features output by the encoder, and then enter depth convolution for feature After the interaction, add the residual input:
Finally, the interacted features are used for channel information interaction through FNN, and then pooled to the target number to get the output embedding of the decoder:
Finally we send the output embedding to the detection head for subsequent classification and regression.
Multi-scale features
Like the original DETR, the DECO obtained by the above framework has a common shortcoming, that is, the lack of multi-scale features, which has a great impact on high-precision target detection. . Deformable DETR integrates features of different scales by using a multi-scale deformable attention module, but this method is strongly coupled with the Attention operator, so it cannot be used directly on our DECO. In order to allow DECO to handle multi-scale features, we use a cross-scale feature fusion module proposed by RT-DETR after the features output by the Decoder. In fact, a series of improvement methods have been derived after the birth of DETR. We believe that many strategies are also applicable to DECO, and we hope that interested people can discuss it together.
Experiment
We conducted experiments on COCO and compared DECO and DETR while keeping the main architecture unchanged, such as keeping the number of Query consistent and the number of Decoder layers unchanged. Wait, just replace the Transformer structure in DETR with our convolution structure as described above. It can be seen that DECO achieves better accuracy and faster tradeoff than DETR.
We also compared DECO with multi-scale features and more target detection methods, including many DETR variants, as shown in the figure below It can be seen that DECO has achieved very good results and achieved better performance than many previous detectors.
The structure of DECO in the article has undergone many ablation experiments and visualizations, including the specific fusion strategy (addition, dot multiplication, Concat) selected in Decoder, and Query There are also some interesting findings on how to set the dimensions to achieve optimal results. For more detailed results and discussion, please refer to the original article.
Summary
This article aims to study whether it is possible to build a query-based end-to-end target detection framework without using a complex Transformer architecture. A new detection framework called Detection ConvNet (DECO) is proposed, including a backbone network and a convolutional encoder-decoder structure. By carefully designing the DECO encoder and introducing a novel mechanism, the DECO decoder is able to achieve the interaction between the target query and image features through convolutional layers. Comparisons were made with previous detectors on the COCO benchmark, and despite simplicity, DECO achieved competitive performance in terms of detection accuracy and running speed. Specifically, using ResNet-50 and ConvNeXt-Tiny backbones, DECO achieved 38.6% and 40.8% AP on the COCO validation set at 35 and 28 FPS respectively, outperforming the DET model. It is hoped that DECO provides a new perspective on designing object detection frameworks.
The above is the detailed content of DECO: Pure convolutional Query-Based detector surpasses DETR!. For more information, please follow other related articles on the PHP Chinese website!

Hot AI Tools

Undresser.AI Undress
AI-powered app for creating realistic nude photos

AI Clothes Remover
Online AI tool for removing clothes from photos.

Undress AI Tool
Undress images for free

Clothoff.io
AI clothes remover

AI Hentai Generator
Generate AI Hentai for free.

Hot Article

Hot Tools

Notepad++7.3.1
Easy-to-use and free code editor

SublimeText3 Chinese version
Chinese version, very easy to use

Zend Studio 13.0.1
Powerful PHP integrated development environment

Dreamweaver CS6
Visual web development tools

SublimeText3 Mac version
God-level code editing software (SublimeText3)

Hot Topics
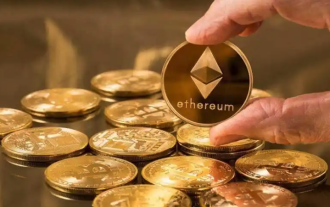
Since its inception in 2009, Bitcoin has become a leader in the cryptocurrency world and its price has experienced huge fluctuations. To provide a comprehensive historical overview, this article compiles Bitcoin price data from 2009 to 2025, covering major market events, changes in market sentiment, and important factors influencing price movements.
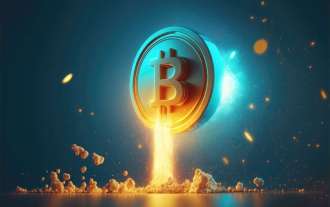
With the popularity of cryptocurrencies, virtual currency trading platforms have emerged. The top ten virtual currency trading platforms in the world are ranked as follows according to transaction volume and market share: Binance, Coinbase, FTX, KuCoin, Crypto.com, Kraken, Huobi, Gate.io, Bitfinex, Gemini. These platforms offer a wide range of services, ranging from a wide range of cryptocurrency choices to derivatives trading, suitable for traders of varying levels.
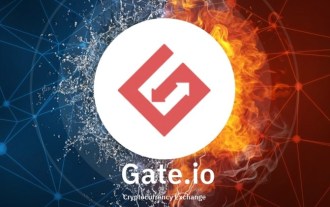
How to adjust Sesame Open Exchange to Chinese? This tutorial covers detailed steps on computers and Android mobile phones, from preliminary preparation to operational processes, and then to solving common problems, helping you easily switch the Sesame Open Exchange interface to Chinese and quickly get started with the trading platform.
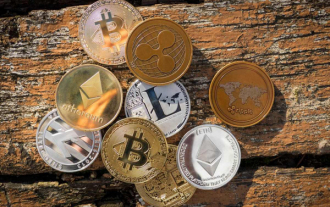
Since its creation in 2009, Bitcoin’s price has experienced several major fluctuations, rising to $69,044.77 in November 2021 and falling to $3,191.22 in December 2018. As of December 2024, the latest price has exceeded $100,204.
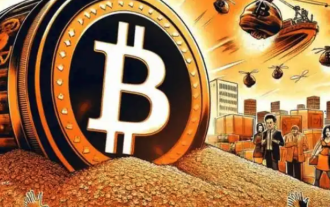
Bitcoin, as a cryptocurrency, has experienced significant market volatility since its inception. This article will provide an overview of the historical price of Bitcoin since its birth to help readers understand its price trends and key moments. By analyzing Bitcoin's historical price data, we can understand the market's assessment of its value, factors affecting its fluctuations, and provide a basis for future investment decisions.
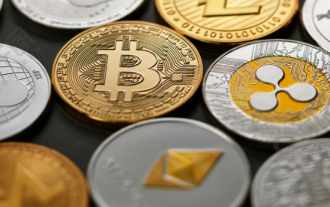
The top ten cryptocurrency trading platforms include: 1. OKX, 2. Binance, 3. Gate.io, 4. Kraken, 5. Huobi, 6. Coinbase, 7. KuCoin, 8. Crypto.com, 9. Bitfinex, 10. Gemini. Security, liquidity, handling fees, currency selection, user interface and customer support should be considered when choosing a platform.
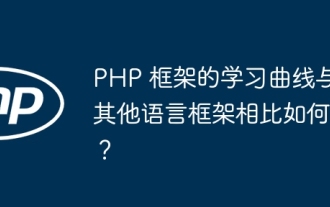
The learning curve of a PHP framework depends on language proficiency, framework complexity, documentation quality, and community support. The learning curve of PHP frameworks is higher when compared to Python frameworks and lower when compared to Ruby frameworks. Compared to Java frameworks, PHP frameworks have a moderate learning curve but a shorter time to get started.
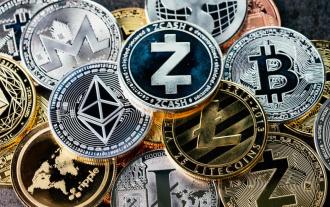
The top ten cryptocurrency exchanges in China are arranged by trading volume as follows: 1. Binance; 2. Huobi; 3. OKEx; 4. Anyin; 5. Matcha; 6. Gate.io; 7. KuCoin; 8. BitMart; 9.JEX; 10.LBank. These exchanges offer a wide range of trading pairs, low trading fees, and professional services tailored to specific user needs.
