


Stability AI open source new release: 3D generation introduces video diffusion model, quality consistency up, 4090 playable
Stability AI, the company behind Stable Diffusion, has launched something new.
What this time brings is new progress in Tusheng 3D:
Stable Video 3D (SV3D) based on Stable Video Diffusion can generate high-quality 3D networks with only one picture grid.
Stable Video Diffusion (SVD) is a model previously released by Stability AI for generating high-resolution videos. The advent of SV3D marks the first time that the video diffusion model has been successfully applied to the field of 3D generation.
Officially stated that based on this, SV3D has greatly improved the quality and view consistency of 3D generation.
The model weights are still open source, but they can only be used for non-commercial purposes. If you want to use them commercially, you have to buy a Stability AI membership~
Not much to say , let’s take a look at the details of the paper.
Using the video diffusion model for 3D generation
Introducing the latent video diffusion model, the core purpose of SV3D is to use the temporal consistency of the video model to improve the consistency of 3D generation.
And the video data itself is easier to obtain than 3D data.
Stability AI provides two versions of SV3D this time:
- SV3D_u: Generate orbit video based on a single image.
- SV3D_p: Extends the functionality of SV3D_u to create 3D model videos based on specified camera paths.
The researchers also improved the 3D optimization technology: using a coarse-to-fine training strategy to optimize NeRF and DMTet meshes to generate 3D objects.
They also designed a special loss function called Masked Score Distillation Sampling (SDS) to improve performance by optimizing areas that are not directly visible in the training data. Quality and consistency of generated 3D models.
At the same time, SV3D introduces a lighting model based on spherical Gaussian to separate lighting effects and textures, effectively reducing built-in lighting problems while maintaining texture clarity.
Specifically in terms of architecture, SV3D contains the following key components:
- UNet: SV3D is built on the basis of SVD and contains a multi-layer UNet, where each layer has a series of residual blocks (including 3D convolutional layers) and two to process spatial and temporal information respectively. Transformer module.
- Conditional input: The input image is embedded into the latent space through the VAE encoder, and will be merged with the noise potential state and input into UNet together; the CLIP embedding matrix of the input image is used as the cross attention of each Transformer module Key-value pairs for the force layer.
- Camera trajectory encoding: SV3D designs two types of trajectories, static and dynamic, to study the impact of camera attitude conditions. In a static orbit, the camera surrounds the object at regularly spaced azimuth angles; a dynamic orbit allows for irregularly spaced azimuth angles and different elevation angles.
The camera's motion trajectory information and the time information of the diffusion noise will be input into the residual module together and converted into sinusoidal position embeddings. Then these embedded information will be integrated and linearly transformed, and added to the noise The time step is embedded in.
This design aims to improve the model's ability to process images by finely controlling camera trajectories and noise input.
In addition, SV3D uses CFG (classifier-free guidance) during the generation process to control the sharpness of the generation, especially when generating the last few frames of the track, using triangles CFG scaling to avoid over-sharpening.
The researchers trained SV3D on the Objaverse dataset, with an image resolution of 575×576 and a field of view of 33.8 degrees. The paper reveals that all three models (SV3D_u, SV3D_c, SV3D_p) were trained on 4 nodes for about 6 days, each node equipped with 8 80GB A100 GPUs.
Experimental results
In terms of new perspective synthesis (NVS) and 3D reconstruction, SV3D surpasses other existing methods and reaches SOTA.
#From the results of qualitative comparison, the multi-view view generated by SV3D has richer details and is closer to the original input image. In other words, SV3D can more accurately capture details and maintain consistency when viewing angle changes in understanding and reconstructing the 3D structure of objects.
Such results have aroused the emotion of many netizens:
It is conceivable that in the next 6-12 months, 3D generation Technology will be used in gaming and video projects.
There are always some bold ideas in the comment area...
And the project is open source, the first wave of friends have already started playing it, and they can run it on 4090.
Reference link:
[1]https://twitter.com/StabilityAI/status/1769817136799855098.
[2]https://stability.ai/news/introducing-stable-video-3d.
[3]https://sv3d.github.io/index.html.
The above is the detailed content of Stability AI open source new release: 3D generation introduces video diffusion model, quality consistency up, 4090 playable. For more information, please follow other related articles on the PHP Chinese website!

Hot AI Tools

Undresser.AI Undress
AI-powered app for creating realistic nude photos

AI Clothes Remover
Online AI tool for removing clothes from photos.

Undress AI Tool
Undress images for free

Clothoff.io
AI clothes remover

AI Hentai Generator
Generate AI Hentai for free.

Hot Article

Hot Tools

Notepad++7.3.1
Easy-to-use and free code editor

SublimeText3 Chinese version
Chinese version, very easy to use

Zend Studio 13.0.1
Powerful PHP integrated development environment

Dreamweaver CS6
Visual web development tools

SublimeText3 Mac version
God-level code editing software (SublimeText3)

Hot Topics
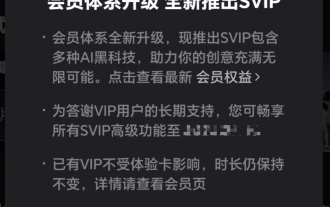
This site reported on June 27 that Jianying is a video editing software developed by FaceMeng Technology, a subsidiary of ByteDance. It relies on the Douyin platform and basically produces short video content for users of the platform. It is compatible with iOS, Android, and Windows. , MacOS and other operating systems. Jianying officially announced the upgrade of its membership system and launched a new SVIP, which includes a variety of AI black technologies, such as intelligent translation, intelligent highlighting, intelligent packaging, digital human synthesis, etc. In terms of price, the monthly fee for clipping SVIP is 79 yuan, the annual fee is 599 yuan (note on this site: equivalent to 49.9 yuan per month), the continuous monthly subscription is 59 yuan per month, and the continuous annual subscription is 499 yuan per year (equivalent to 41.6 yuan per month) . In addition, the cut official also stated that in order to improve the user experience, those who have subscribed to the original VIP
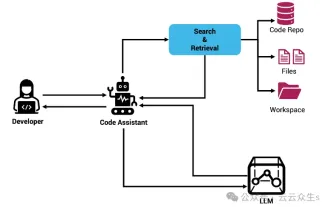
Improve developer productivity, efficiency, and accuracy by incorporating retrieval-enhanced generation and semantic memory into AI coding assistants. Translated from EnhancingAICodingAssistantswithContextUsingRAGandSEM-RAG, author JanakiramMSV. While basic AI programming assistants are naturally helpful, they often fail to provide the most relevant and correct code suggestions because they rely on a general understanding of the software language and the most common patterns of writing software. The code generated by these coding assistants is suitable for solving the problems they are responsible for solving, but often does not conform to the coding standards, conventions and styles of the individual teams. This often results in suggestions that need to be modified or refined in order for the code to be accepted into the application

To learn more about AIGC, please visit: 51CTOAI.x Community https://www.51cto.com/aigc/Translator|Jingyan Reviewer|Chonglou is different from the traditional question bank that can be seen everywhere on the Internet. These questions It requires thinking outside the box. Large Language Models (LLMs) are increasingly important in the fields of data science, generative artificial intelligence (GenAI), and artificial intelligence. These complex algorithms enhance human skills and drive efficiency and innovation in many industries, becoming the key for companies to remain competitive. LLM has a wide range of applications. It can be used in fields such as natural language processing, text generation, speech recognition and recommendation systems. By learning from large amounts of data, LLM is able to generate text
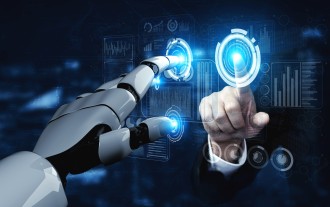
Large Language Models (LLMs) are trained on huge text databases, where they acquire large amounts of real-world knowledge. This knowledge is embedded into their parameters and can then be used when needed. The knowledge of these models is "reified" at the end of training. At the end of pre-training, the model actually stops learning. Align or fine-tune the model to learn how to leverage this knowledge and respond more naturally to user questions. But sometimes model knowledge is not enough, and although the model can access external content through RAG, it is considered beneficial to adapt the model to new domains through fine-tuning. This fine-tuning is performed using input from human annotators or other LLM creations, where the model encounters additional real-world knowledge and integrates it
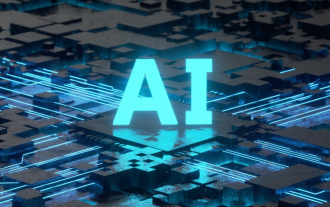
Editor |ScienceAI Question Answering (QA) data set plays a vital role in promoting natural language processing (NLP) research. High-quality QA data sets can not only be used to fine-tune models, but also effectively evaluate the capabilities of large language models (LLM), especially the ability to understand and reason about scientific knowledge. Although there are currently many scientific QA data sets covering medicine, chemistry, biology and other fields, these data sets still have some shortcomings. First, the data form is relatively simple, most of which are multiple-choice questions. They are easy to evaluate, but limit the model's answer selection range and cannot fully test the model's ability to answer scientific questions. In contrast, open-ended Q&A
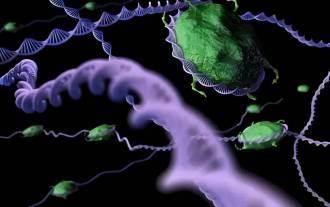
Editor | KX In the field of drug research and development, accurately and effectively predicting the binding affinity of proteins and ligands is crucial for drug screening and optimization. However, current studies do not take into account the important role of molecular surface information in protein-ligand interactions. Based on this, researchers from Xiamen University proposed a novel multi-modal feature extraction (MFE) framework, which for the first time combines information on protein surface, 3D structure and sequence, and uses a cross-attention mechanism to compare different modalities. feature alignment. Experimental results demonstrate that this method achieves state-of-the-art performance in predicting protein-ligand binding affinities. Furthermore, ablation studies demonstrate the effectiveness and necessity of protein surface information and multimodal feature alignment within this framework. Related research begins with "S
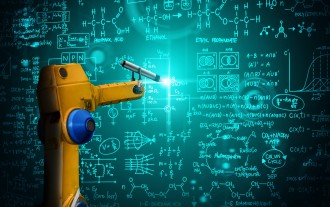
Machine learning is an important branch of artificial intelligence that gives computers the ability to learn from data and improve their capabilities without being explicitly programmed. Machine learning has a wide range of applications in various fields, from image recognition and natural language processing to recommendation systems and fraud detection, and it is changing the way we live. There are many different methods and theories in the field of machine learning, among which the five most influential methods are called the "Five Schools of Machine Learning". The five major schools are the symbolic school, the connectionist school, the evolutionary school, the Bayesian school and the analogy school. 1. Symbolism, also known as symbolism, emphasizes the use of symbols for logical reasoning and expression of knowledge. This school of thought believes that learning is a process of reverse deduction, through existing
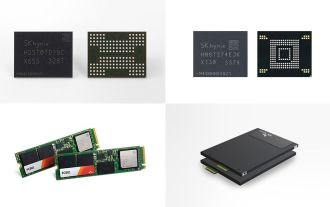
According to news from this site on August 1, SK Hynix released a blog post today (August 1), announcing that it will attend the Global Semiconductor Memory Summit FMS2024 to be held in Santa Clara, California, USA from August 6 to 8, showcasing many new technologies. generation product. Introduction to the Future Memory and Storage Summit (FutureMemoryandStorage), formerly the Flash Memory Summit (FlashMemorySummit) mainly for NAND suppliers, in the context of increasing attention to artificial intelligence technology, this year was renamed the Future Memory and Storage Summit (FutureMemoryandStorage) to invite DRAM and storage vendors and many more players. New product SK hynix launched last year
