


CVPR 2024 | Zero-sample 6D object pose estimation framework SAM-6D, one step closer to embodied intelligence
In many practical applications, object pose estimation plays a key role, such as in fields such as embodied intelligence, robot operation, and augmented reality.
In this field, the first task to receive attention is Instance-level 6D pose estimation, which requires annotated data about the target object for model training to make the depth model object-specific properties and cannot be transferred to new objects. Later, the research focus gradually turned to category-level 6D pose estimation, which is used to process unseen objects, but requires that the object belongs to a known category of interest.
And Zero-sample 6D pose estimation is a more generalized task setting. Given a CAD model of any object, it aims to detect the target object in the scene and estimate Its 6D pose. Despite its significance, this zero-shot task setting faces significant challenges in both object detection and pose estimation.
## Figure 1. Zero-sample 6D object pose estimation task
Recently, segment all models SAM [1] has attracted much attention, and its excellent zero-sample segmentation ability is eye-catching. SAM achieves high-precision segmentation through various cues, such as pixels, bounding boxes, text and masks, etc., which also provides reliable support for the zero-sample 6D object pose estimation task, demonstrating its promising potential. Therefore, a new zero-sample 6D object pose estimation framework SAM-6D was proposed by researchers from Cross-Dimensional Intelligence, the Chinese University of Hong Kong (Shenzhen), and South China University of Technology. This research has been recognized by CVPR 2024.- Paper link: https://arxiv.org/pdf/2311.15707.pdf
- Code Link: https://github.com/JiehongLin/SAM-6D
Instance Segmentation Model (ISM) and Pose Estimation Model (PEM), to achieve the target from RGB-D scene images ; Among them, ISM uses SAM as an excellent starting point, combined with carefully designed object matching scores to achieve instance segmentation of arbitrary objects, and PEM solves the object pose problem through a local-to-local two-stage point set matching process. An overview of the SAM-6D is shown in Figure 2.
##
Overall, the technical contributions of SAM-6D can be summarized as follows:
SAM-6D is an innovative zero-sample 6D pose estimation framework that gives the CAD of any object The model implements instance segmentation and pose estimation of target objects from RGB-D images, and performs excellently on the seven core data sets of BOP [2].
SAM-6D leverages the zero-shot segmentation capability of the segmentation-everything model to generate all possible candidate objects and designs a novel object matching score to identify objects corresponding to the target object. candidate.
SAM-6D regards pose estimation as a local-to-local point set matching problem, adopts a simple but effective Background Token design, and proposes a two-dimensional algorithm for arbitrary objects. Stage point set matching model; the first stage implements coarse point set matching to obtain the initial object pose, and the second stage uses a novel sparse to dense point set transformer to perform fine point set matching to further optimize the pose.
Instance Segmentation Model (ISM)
SAM-6D uses the Instance Segmentation Model (ISM) to detect and segment masks of arbitrary objects .
Given a cluttered scene represented by RGB images, ISM utilizes the zero-shot transfer capability of the Segmentation Everything Model (SAM) to generate all possible candidates. For each candidate object, ISM calculates an object match score to estimate how well it matches the target object in terms of semantics, appearance, and geometry. Finally, by simply setting a matching threshold, instances matching the target object can be identified.
The calculation of the object matching score is obtained by the weighted sum of the three matching items:
Semantic matching items - For the target object, ISM renders multiple views Object template, and use the DINOv2 [3] pre-trained ViT model to extract the semantic features of the candidate object and object template, and calculate the correlation score between them. The semantic matching score is obtained by averaging the top K highest scores, and the object template corresponding to the highest correlation score is regarded as the best matching template.
Appearance matching item - For the best matching template, use the ViT model to extract image block features and calculate the correlation between it and the block features of the candidate object to obtain the appearance matching item Score, used to distinguish semantically similar but visually different objects.
Geometric Match - ISM also designed a geometric match score to account for factors such as differences in shape and size of different objects. The average of the rotation corresponding to the best matching template and the point cloud of the candidate object can give a rough object pose, and the bounding box can be obtained by rigidly transforming and projecting the object CAD model using this pose. Calculating the intersection-over-union (IoU) ratio between the bounding box and the candidate bounding box can obtain the geometric matching score.
Pose Estimation Model (PEM)
For each candidate object that matches the target object, SAM-6D utilizes a Pose Estimation Model (PEM) to predict its relative 6D pose of the object CAD model.
The sampling point sets of segmented candidate objects and object CAD models are represented as and
respectively, where N_m and N_o represent the number of their points; at the same time, the characteristics of these two point sets are represented as
and
, C represents the number of channels of the feature. The goal of PEM is to obtain an assignment matrix that represents the local-to-local correspondence from P_m to P_o; due to occlusion, P_o only partially matches P_m, and due to segmentation inaccuracy and sensor noise, P_m only partially matches Partial AND matches P_o.
In order to solve the problem of assigning non-overlapping points between two point sets, ISM equips them with Background Tokens, marked and
, which can effectively establish local-to-local relationships based on feature similarity. Correspondence. Specifically, the attention matrix can first be calculated as follows:
Then the distribution matrix can be obtained
and
represent softmax operations along rows and columns respectively, and
represents a constant. The value of each row in
(except the first row) represents the matching probability of each point P_m in the point set P_m with the background and the midpoint of P_o. By locating the index of the maximum score, the point matching P_m can be found ( including background).
Once the calculation is , all matching point pairs {(P_m,P_o)} and their matching scores can be gathered, and finally weighted SVD is used to calculate the object pose.
## Based on the strategy of Background Token, two point set matching stages are designed in PEM. The model structure is shown in Figure 3, which includes three modules: feature extraction, rough point set matching and fine point set matching.
The rough point set matching module implements sparse correspondence to calculate the initial object pose, and then uses the pose to transform the point set of the candidate object to achieve position coding learning.The fine point set matching module combines the position encoding of the sample point sets of the candidate object and the target object, thereby injecting the rough correspondence in the first stage, and further establishing dense correspondence to obtain a more precise object pose. In order to effectively learn dense interactions at this stage, PEM introduces a novel sparse to dense point set transformer, which implements interactions on sparse versions of dense features, and utilizes Linear Transformer [5] to transform the enhanced sparse features into Diffusion back into dense features.
Experimental results
For the two sub-models of SAM-6D, the instance segmentation model (ISM) is built based on SAM without the need for network retraining and finetune, while the pose estimation model (PEM) is provided by MegaPose [4] Large-scale ShapeNet-Objects and Google-Scanned-Objects synthetic datasets for training.
To verify its zero-sample capability, SAM-6D was tested on seven core data sets of BOP [2], including LM-O, T-LESS, TUD-L, IC-BIN, ITODD, HB and YCB-V. Tables 1 and 2 show the comparison of instance segmentation and pose estimation results of different methods on these seven datasets, respectively. Compared with other methods, SAM-6D performs very well on both methods, fully demonstrating its strong generalization ability.
## to be compared.
Table 2. Comparison of attitude estimation results of different methods on the seven core data sets of BOP
Figure 4 shows Visualization results of detection segmentation and 6D pose estimation of SAM-6D on seven BOP data sets, where (a) and (b) are the test RGB images and depth maps respectively, (c) is the given target object, and (d) and (e) are the visualization results of detection segmentation and 6D pose respectively.
## Figure 4. Visualization results of SAM-6D on the seven core data sets of BOP.For more implementation details of SAM-6D, welcome to read the original paper.References:
[1] Alexander Kirillov et. al., "Segment anything."
[2] Martin Sundermeyer et. al., "Bop challenge 2022 on detection, segmentation and pose estimation of specific rigid objects."
[3] Maxime Oquab et. al., "Dinov2 : Learning robust visual features without supervision."
[4] Yann Labbe et. al., "Megapose: 6d pose estimation of novel objects via render & compare .”
[5] Angelos Katharopoulos et. al., “Transformers are rnns: Fast autoregressive
transformers with linear attention.”
The above is the detailed content of CVPR 2024 | Zero-sample 6D object pose estimation framework SAM-6D, one step closer to embodied intelligence. For more information, please follow other related articles on the PHP Chinese website!

Hot AI Tools

Undresser.AI Undress
AI-powered app for creating realistic nude photos

AI Clothes Remover
Online AI tool for removing clothes from photos.

Undress AI Tool
Undress images for free

Clothoff.io
AI clothes remover

AI Hentai Generator
Generate AI Hentai for free.

Hot Article

Hot Tools

Notepad++7.3.1
Easy-to-use and free code editor

SublimeText3 Chinese version
Chinese version, very easy to use

Zend Studio 13.0.1
Powerful PHP integrated development environment

Dreamweaver CS6
Visual web development tools

SublimeText3 Mac version
God-level code editing software (SublimeText3)

Hot Topics

The AIxiv column is a column where this site publishes academic and technical content. In the past few years, the AIxiv column of this site has received more than 2,000 reports, covering top laboratories from major universities and companies around the world, effectively promoting academic exchanges and dissemination. If you have excellent work that you want to share, please feel free to contribute or contact us for reporting. Submission email: liyazhou@jiqizhixin.com; zhaoyunfeng@jiqizhixin.com In the development process of artificial intelligence, the control and guidance of large language models (LLM) has always been one of the core challenges, aiming to ensure that these models are both powerful and safe serve human society. Early efforts focused on reinforcement learning methods through human feedback (RL
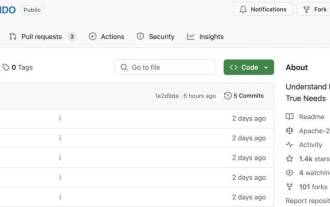
It is also a Tusheng video, but PaintsUndo has taken a different route. ControlNet author LvminZhang started to live again! This time I aim at the field of painting. The new project PaintsUndo has received 1.4kstar (still rising crazily) not long after it was launched. Project address: https://github.com/lllyasviel/Paints-UNDO Through this project, the user inputs a static image, and PaintsUndo can automatically help you generate a video of the entire painting process, from line draft to finished product. follow. During the drawing process, the line changes are amazing. The final video result is very similar to the original image: Let’s take a look at a complete drawing.
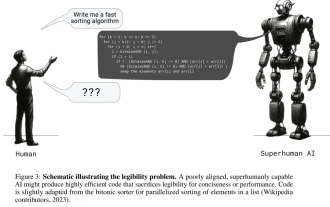
If the answer given by the AI model is incomprehensible at all, would you dare to use it? As machine learning systems are used in more important areas, it becomes increasingly important to demonstrate why we can trust their output, and when not to trust them. One possible way to gain trust in the output of a complex system is to require the system to produce an interpretation of its output that is readable to a human or another trusted system, that is, fully understandable to the point that any possible errors can be found. For example, to build trust in the judicial system, we require courts to provide clear and readable written opinions that explain and support their decisions. For large language models, we can also adopt a similar approach. However, when taking this approach, ensure that the language model generates

The AIxiv column is a column where this site publishes academic and technical content. In the past few years, the AIxiv column of this site has received more than 2,000 reports, covering top laboratories from major universities and companies around the world, effectively promoting academic exchanges and dissemination. If you have excellent work that you want to share, please feel free to contribute or contact us for reporting. Submission email: liyazhou@jiqizhixin.com; zhaoyunfeng@jiqizhixin.com The authors of this paper are all from the team of teacher Zhang Lingming at the University of Illinois at Urbana-Champaign (UIUC), including: Steven Code repair; Deng Yinlin, fourth-year doctoral student, researcher

Recently, the Riemann Hypothesis, known as one of the seven major problems of the millennium, has achieved a new breakthrough. The Riemann Hypothesis is a very important unsolved problem in mathematics, related to the precise properties of the distribution of prime numbers (primes are those numbers that are only divisible by 1 and themselves, and they play a fundamental role in number theory). In today's mathematical literature, there are more than a thousand mathematical propositions based on the establishment of the Riemann Hypothesis (or its generalized form). In other words, once the Riemann Hypothesis and its generalized form are proven, these more than a thousand propositions will be established as theorems, which will have a profound impact on the field of mathematics; and if the Riemann Hypothesis is proven wrong, then among these propositions part of it will also lose its effectiveness. New breakthrough comes from MIT mathematics professor Larry Guth and Oxford University
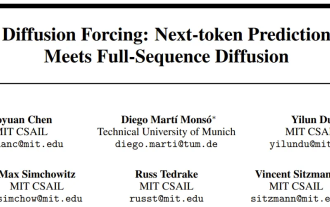
Currently, autoregressive large-scale language models using the next token prediction paradigm have become popular all over the world. At the same time, a large number of synthetic images and videos on the Internet have already shown us the power of diffusion models. Recently, a research team at MITCSAIL (one of whom is Chen Boyuan, a PhD student at MIT) successfully integrated the powerful capabilities of the full sequence diffusion model and the next token model, and proposed a training and sampling paradigm: Diffusion Forcing (DF). Paper title: DiffusionForcing:Next-tokenPredictionMeetsFull-SequenceDiffusion Paper address: https:/
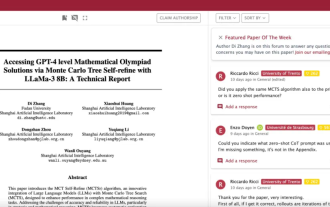
cheers! What is it like when a paper discussion is down to words? Recently, students at Stanford University created alphaXiv, an open discussion forum for arXiv papers that allows questions and comments to be posted directly on any arXiv paper. Website link: https://alphaxiv.org/ In fact, there is no need to visit this website specifically. Just change arXiv in any URL to alphaXiv to directly open the corresponding paper on the alphaXiv forum: you can accurately locate the paragraphs in the paper, Sentence: In the discussion area on the right, users can post questions to ask the author about the ideas and details of the paper. For example, they can also comment on the content of the paper, such as: "Given to
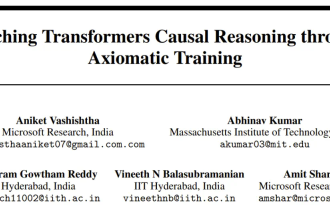
Show the causal chain to LLM and it learns the axioms. AI is already helping mathematicians and scientists conduct research. For example, the famous mathematician Terence Tao has repeatedly shared his research and exploration experience with the help of AI tools such as GPT. For AI to compete in these fields, strong and reliable causal reasoning capabilities are essential. The research to be introduced in this article found that a Transformer model trained on the demonstration of the causal transitivity axiom on small graphs can generalize to the transitive axiom on large graphs. In other words, if the Transformer learns to perform simple causal reasoning, it may be used for more complex causal reasoning. The axiomatic training framework proposed by the team is a new paradigm for learning causal reasoning based on passive data, with only demonstrations
