


What is the difference between AI inference and training? do you know?
If I want to sum up the difference between AI training and reasoning in one sentence, I think it is most appropriate to use "one minute on stage, ten years of hard work off stage".
Xiao Ming has been dating the goddess he has long admired for many years, and he has quite a lot of experience in asking her out, but he is still confused about the mystery.
With the help of AI technology, can accurate predictions be achieved?
Xiao Ming thought over and over again and summarized the variables that may affect whether the goddess accepts the invitation: whether it is a holiday, the weather is bad, too hot/too cold, in a bad mood, sick, etc. He has an appointment, relatives come to the house...etc.
Picture
We weight and sum these variables. If it is greater than a certain threshold, the goddess will definitely accept the invitation. So, how much weight do these variables have, and what are the thresholds?
This is a very complex problem that is difficult to solve accurately through simple methods. Therefore, Xiao Ming plans to conduct research using deep neural networks and apply them to large amounts of accumulated data for training, so that the artificial intelligence model can learn the patterns on its own.
Picture
Xiao Ming’s biggest advantage is that he has rich data accumulation. So he organized and accurately listed all the variables and mapped them exactly to whether the offer was successful or not. This practice is called "data annotation".
Picture
Once you have the data, feed it to the AI. AI reads each set of data, evaluates it using the initial default weights, and then obtains the results of its own analysis. This process is called "forward propagation".
Then, check whether the AI results are correct.
Here you need to introduce a "loss function" to calculate the difference between the result and the correct answer. If the result is not ideal, it will go back to optimize and adjust the weights, and obtain the results again for evaluation. This process is called "back propagation".
After inspection, it was found that the evaluation results and the correct answers are one step closer. After many rounds of iterations, the correct answer is gradually approached by adjusting parameter weights. This process is called "gradient descent".
Picture
After many rounds of in-depth baptism of known data, the accuracy of AI evaluation is already quite high. So Xiao Ming ended the training, fixed the parameter weights, trimmed off the redundant parameters whose weights were not activated, and declared to enter the next stage.
It’s time to test the results of the hard work done some time ago!
Picture
So, Xiao Ming chose a good and auspicious day to prepare all the new parameters and input them into the AI. The AI quickly gave its own assessment conclusion: the goddess will accept the invitation!
The above process is called "reasoning".
Xiao Ming took a shower and changed clothes, tidied up carefully, booked movie tickets, and carefully asked the goddess for her opinion. Sure enough, the goddess agreed!
From then on, before each invitation, Xiao Ming would devoutly ask the AI to predict whether it would be successful. It turns out that AI can get it right most of the time. We can say that the "generalization" effect of AI is very good.
Picture
#To sum up, the so-called AI training is the process of letting the neural network learn new capabilities from existing data. .
This process is very complicated, just like receiving nine years of compulsory education since childhood. It involves the close cooperation of schools, books, teachers and other factors. The data throughput is large, it is intensive calculation, and it costs a lot of money. Time training is very necessary.
The so-called AI reasoning is to input new data to the trained AI and let it solve new problems of the same type.
This is like a student graduating from college, leaving school, books, and teachers, and using the knowledge learned to independently deal with new problems. The data throughput is relatively small, but he needs to be on call at any time. Give answers quickly and well.
The AI applications we generally come into contact with are APPs trained by service providers. We propose various tasks above, and the background responds quickly and gives answers in seconds. These all belong to AI reasoning.
Mastering AI well will allow us to work with ease and get twice the result with half the effort.
The above is the detailed content of What is the difference between AI inference and training? do you know?. For more information, please follow other related articles on the PHP Chinese website!

Hot AI Tools

Undresser.AI Undress
AI-powered app for creating realistic nude photos

AI Clothes Remover
Online AI tool for removing clothes from photos.

Undress AI Tool
Undress images for free

Clothoff.io
AI clothes remover

Video Face Swap
Swap faces in any video effortlessly with our completely free AI face swap tool!

Hot Article

Hot Tools

Notepad++7.3.1
Easy-to-use and free code editor

SublimeText3 Chinese version
Chinese version, very easy to use

Zend Studio 13.0.1
Powerful PHP integrated development environment

Dreamweaver CS6
Visual web development tools

SublimeText3 Mac version
God-level code editing software (SublimeText3)

Hot Topics


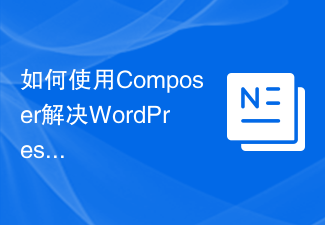
When managing WordPress websites, you often encounter complex operations such as installation, update, and multi-site conversion. These operations are not only time-consuming, but also prone to errors, causing the website to be paralyzed. Combining the WP-CLI core command with Composer can greatly simplify these tasks, improve efficiency and reliability. This article will introduce how to use Composer to solve these problems and improve the convenience of WordPress management.
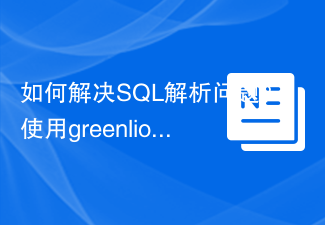
When developing a project that requires parsing SQL statements, I encountered a tricky problem: how to efficiently parse MySQL's SQL statements and extract the key information. After trying many methods, I found that the greenlion/php-sql-parser library can perfectly solve my needs.
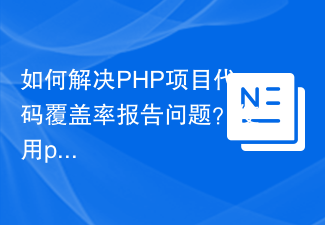
When developing PHP projects, ensuring code coverage is an important part of ensuring code quality. However, when I was using TravisCI for continuous integration, I encountered a problem: the test coverage report was not uploaded to the Coveralls platform, resulting in the inability to monitor and improve code coverage. After some exploration, I found the tool php-coveralls, which not only solved my problem, but also greatly simplified the configuration process.
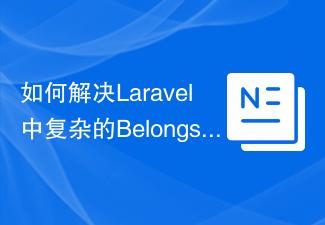
In Laravel development, dealing with complex model relationships has always been a challenge, especially when it comes to multi-level BelongsToThrough relationships. Recently, I encountered this problem in a project dealing with a multi-level model relationship, where traditional HasManyThrough relationships fail to meet the needs, resulting in data queries becoming complex and inefficient. After some exploration, I found the library staudenmeir/belongs-to-through, which easily installed and solved my troubles through Composer.
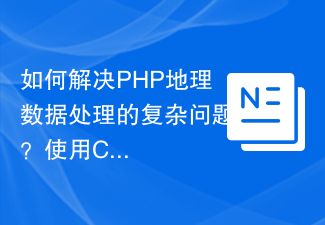
When developing a Geographic Information System (GIS), I encountered a difficult problem: how to efficiently handle various geographic data formats such as WKT, WKB, GeoJSON, etc. in PHP. I've tried multiple methods, but none of them can effectively solve the conversion and operational issues between these formats. Finally, I found the GeoPHP library, which easily integrates through Composer, and it completely solved my troubles.
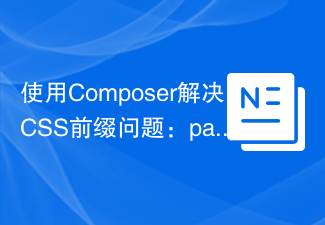
I'm having a tricky problem when developing a front-end project: I need to manually add a browser prefix to the CSS properties to ensure compatibility. This is not only time consuming, but also error-prone. After some exploration, I discovered the padaliyajay/php-autoprefixer library, which easily solved my troubles with Composer.
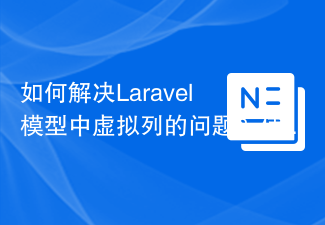
During Laravel development, it is often necessary to add virtual columns to the model to handle complex data logic. However, adding virtual columns directly into the model can lead to complexity of database migration and maintenance. After I encountered this problem in my project, I successfully solved this problem by using the stancl/virtualcolumn library. This library not only simplifies the management of virtual columns, but also improves the maintainability and efficiency of the code.
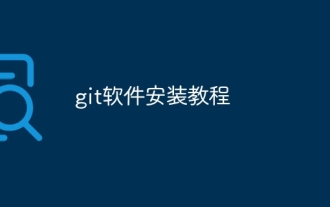
Git Software Installation Guide: Visit the official Git website to download the installer for Windows, MacOS, or Linux. Run the installer and follow the prompts. Configure Git: Set username, email, and select a text editor. For Windows users, configure the Git Bash environment.
