


Mamba's super evolved form subverts Transformer in one fell swoop! Single A100 running 140K context
The Mamba architecture, which previously detonated the AI circle, has launched a super variant today!
Artificial intelligence unicorn AI21 Labs has just open sourced Jamba, the world’s first production-level Mamba large model!
Jamba has performed well in multiple benchmark tests and is on par with some of the strongest open source Transformers currently.
Especially when comparing Mixtral 8x7B, which has the best performance and is also a MoE architecture, there are also winners and losers.
Specifically it——
- is the first production-grade Mamba model based on the new SSM-Transformer hybrid architecture
- Long text processing throughput increased by 3 times compared to Mixtral 8x7B
- Achieved 256K ultra-long context window
- It is the only model of the same scale that can process 140K contexts on a single GPU
- Released under the Apache 2.0 open source license, with heavy open rights
The previous Mamba could only do 3B due to various restrictions, and was also questioned whether it could take over the Transformer The banner, while RWKV, Griffin, etc., which are also linear RNN families, have only expanded to 14B.
——Jamba directly went to 52B this time, allowing the Mamba architecture to compete head-on with the production-level Transformer for the first time.
Based on the original Mamba architecture, Jamba incorporates the advantages of Transformer to make up for the shortcomings of the state space model (SSM). inherent limitations.
It can be considered that this is actually a new architecture - a mixture of Transformer and Mamba, the most important thing The nice thing is, it can run on a single A100.
It provides an ultra-long context window of up to 256K, a single GPU can run 140K context, and the throughput is 3 times that of Transformer!
Compared with Transformer, it is very shocking to see how Jamba scales to huge context lengths
Jamba adopts the MoE solution. 12B of the 52B are active parameters. The current model has open weights under Apache 2.0 and can be downloaded on huggingface.
Model download: https://huggingface.co/ai21labs/Jamba-v0.1
New milestone for LLM
The release of Jamba marks two important milestones for LLM:
First, the successful integration of Mamba with the Transformer architecture , and the second is to successfully upgrade the new form of model (SSM-Transformer) to production-level scale and quality.
The current large models with the strongest performance are all based on Transformer, although everyone has also realized the two main shortcomings of the Transformer architecture:
Large memory footprint: Transformer's memory footprint expands with the context length. Running long context windows or massively parallel batch processing requires a lot of hardware resources, which limits large-scale experimentation and deployment.
As the context grows, the inference speed will slow down: Transformer’s attention mechanism causes the inference time to grow squarely relative to the sequence length, and the throughput will become slower and slower. Because each token depends on the entire sequence before it, it becomes quite difficult to achieve very long contexts.
Years ago, two big guys from Carnegie Mellon and Princeton proposed Mamba, which instantly ignited people’s hopes.
Mamba以SSM為基礎,增加了選擇性提取資訊的能力、以及硬體上高效的演算法,一舉解決了Transformer存在的問題。
這個新領域馬上就吸引了大量的研究者,arXiv上一時湧現了大量關於Mamba的應用和改進,例如將Mamba用於視覺的Vision Mamba。
不得不說,現在的科學研究領域實在是太捲了,把Transformer引入視覺(ViT)用了三年,但Mamba到Vision Mamba只花了一個月。
不過原始Mamba的上下文長度較短,加上模型本身也沒有做大,所以很難打過SOTA的Transformer模型,尤其是在與召回相關的任務上。
Jamba於是更進一步,透過Joint Attention and Mamba架構,整合了Transformer、Mamba、以及專家混合(MoE)的優勢,同時優化了記憶體、吞吐量和效能。
Jamba是第一個達到生產級規模(52B參數)的混合架構。
如下圖所示,AI21的Jamba架構採用blocks-and-layers的方法,使Jamba能夠成功整合這兩種架構。
每個Jamba區塊都包含一個注意力層或一個Mamba層,然後是一個多層感知器(MLP)。
Jamba的第二個特點,是利用MoE來增加模型參數的總數,同時簡化推理中所使用的活動參數的數量,從而在不增加計算要求的情況下提高模型容量。
為了在單一80GB GPU上最大限度地提高模型的品質和吞吐量,研究人員優化了使用的MoE層和專家的數量,為常見的推理工作負載留出足夠的記憶體。
對比Mixtral 8x7B等類似大小的基於Transformer的模型,Jamba在長上下文上做到了3倍的加速。
Jamba將在不久之後加入NVIDIA API目錄。
長上下文又出新選手
最近,各大公司都在卷長上下文。
具有較小上下文視窗的模型,往往會忘記最近對話的內容,而具有較大上下文的模型則避免了這種陷阱,可以更好地掌握所接收的數據流。
不過,具有長上下文視窗的模型,往往是計算密集的。
新創公司AI21 Labs的生成式模型就證明,事實並非如此。
Jamba在具有至少80GB顯存的單一GPU(如A100)上運行時,可以處理多達140,000個token。
這相當於大約105,000字,或210頁,是一本長度適中的長篇小說的篇幅。
相較之下,Meta Llama 2的上下文窗口,只有32,000個token,需要12GB的GPU顯存。
以今天的標準來看,這種上下文視窗顯然是偏小的。
對此,有網友也第一時間表示,性能什麼的都不重要,關鍵的是Jamba有256K的上下文,除了Gemini,其他人都沒有這麼長,— —而Jamba可是開源的。
Jamba真正的獨特之處
從表面上看,Jamba似乎並不起眼。
無論是昨天風頭正盛的DBRX,還是Llama 2,現在都已經有大量免費提供、可下載的生成式AI模型。
而Jamba的獨特之處,是藏在模型底下的:它同時結合了兩種模型架構-Transformer和狀態空間模型SSM。
一方面,Transformer是複雜推理任務的首選架構。它最核心的定義特徵,就是「注意力機制」。對於每個輸入數據,Transformer會權衡所有其他輸入的相關性,並從中提取以產生輸出。
另一方面,SSM結合了早期AI模型的多個優點,例如遞歸神經網路和卷積神經網絡,因此能夠實現長序列資料的處理,且運算效率更高。
雖然SSM有自己的限制。但一些早期的代表,例如由普林斯頓和CMU提出的Mamba,就可以處理比Transformer模型更大的輸出,在語言生成任務上也更優。
對此,AI21 Labs產品負責人Dagan表示-
#雖然也有一些SSM模型的初步範例,但Jamba是第一個生產規模的商業級模型。
在他看來,Jamba除了創新和趣味性可供社群進一步研究,還提供了巨大的效率,和吞吐量的可能性。
目前,Jamba是基於Apache 2.0許可發布的,使用限制較少但不能商用。後續的微調版本,預計將在幾週內推出。
即便還處在研究的早期階段,但Dagan斷言,Jamba無疑展示了SSM架構的巨大前景。
「這個模型的附加價值-無論是因為尺寸或架構的創新-都可以很容易地安裝到單一GPU上。」
The above is the detailed content of Mamba's super evolved form subverts Transformer in one fell swoop! Single A100 running 140K context. For more information, please follow other related articles on the PHP Chinese website!

Hot AI Tools

Undresser.AI Undress
AI-powered app for creating realistic nude photos

AI Clothes Remover
Online AI tool for removing clothes from photos.

Undress AI Tool
Undress images for free

Clothoff.io
AI clothes remover

AI Hentai Generator
Generate AI Hentai for free.

Hot Article

Hot Tools

Notepad++7.3.1
Easy-to-use and free code editor

SublimeText3 Chinese version
Chinese version, very easy to use

Zend Studio 13.0.1
Powerful PHP integrated development environment

Dreamweaver CS6
Visual web development tools

SublimeText3 Mac version
God-level code editing software (SublimeText3)

Hot Topics
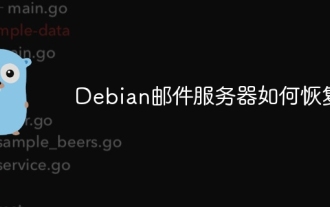
Detailed Steps for Restoring Debian Mail Server This article will guide you on how to restore Debian Mail Server. Before you begin, it is important to remember the importance of data backup. Recovery Steps: Backup Data: Be sure to back up all important email data and configuration files before performing any recovery operations. This will ensure that you have a fallback version when problems occur during the recovery process. Check log files: Check mail server log files (such as /var/log/mail.log) for errors or exceptions. Log files often provide valuable clues about the cause of the problem. Stop service: Stop the mail service to prevent further data corruption. Use the following command: su
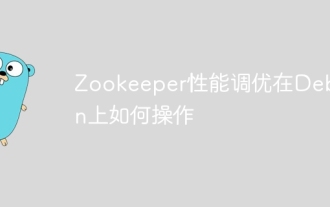
This article describes how to optimize ZooKeeper performance on Debian systems. We will provide advice on hardware, operating system, ZooKeeper configuration and monitoring. 1. Optimize storage media upgrade at the system level: Replacing traditional mechanical hard drives with SSD solid-state drives will significantly improve I/O performance and reduce access latency. Disable swap partitioning: By adjusting kernel parameters, reduce dependence on swap partitions and avoid performance losses caused by frequent memory and disk swaps. Improve file descriptor upper limit: Increase the number of file descriptors allowed to be opened at the same time by the system to avoid resource limitations affecting the processing efficiency of ZooKeeper. 2. ZooKeeper configuration optimization zoo.cfg file configuration
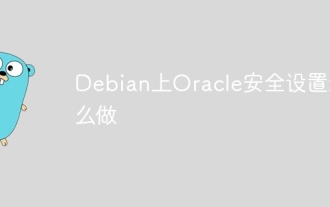
To strengthen the security of Oracle database on the Debian system, it requires many aspects to start. The following steps provide a framework for secure configuration: 1. Oracle database installation and initial configuration system preparation: Ensure that the Debian system has been updated to the latest version, the network configuration is correct, and all required software packages are installed. It is recommended to refer to official documents or reliable third-party resources for installation. Users and Groups: Create a dedicated Oracle user group (such as oinstall, dba, backupdba) and set appropriate permissions for it. 2. Security restrictions set resource restrictions: Edit /etc/security/limits.d/30-oracle.conf
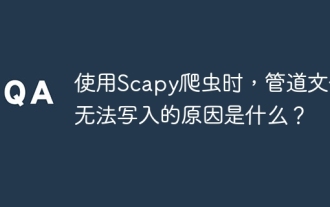
Discussion on the reasons why pipeline files cannot be written when using Scapy crawlers When learning and using Scapy crawlers for persistent data storage, you may encounter pipeline files...
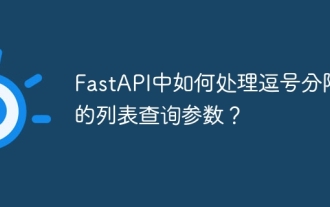
Fastapi ...
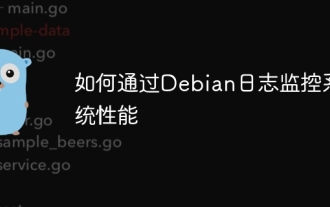
Mastering Debian system log monitoring is the key to efficient operation and maintenance. It can help you understand the system's operating conditions in a timely manner, quickly locate faults, and optimize system performance. This article will introduce several commonly used monitoring methods and tools. Monitoring system resources with the sysstat toolkit The sysstat toolkit provides a series of powerful command line tools for collecting, analyzing and reporting various system resource metrics, including CPU load, memory usage, disk I/O, network throughput, etc. The main tools include: sar: a comprehensive system resource statistics tool, covering CPU, memory, disk, network, etc. iostat: disk and CPU statistics. mpstat: Statistics of multi-core CPUs. pidsta
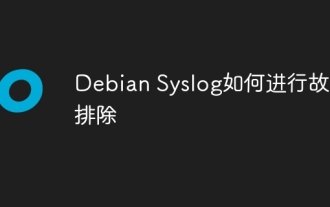
Syslog for Debian systems is a key tool for system administrators to diagnose problems. This article provides some steps and commands to troubleshoot common Syslog problems: 1. Log viewing real-time viewing of the latest log: tail-f/var/log/syslog viewing kernel logs (start errors and driver problems): dmesg uses journalctl (Debian8 and above, systemd system): journalctl-b (viewing after startup logs), journalctl-f (viewing new logs in real-time). 2. System resource monitoring and viewing process and resource usage: psaux (find high resource occupancy process) real-time monitoring
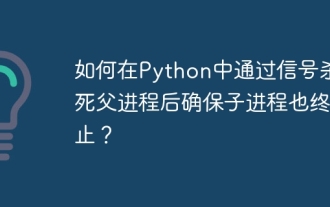
The problem and solution of the child process continuing to run when using signals to kill the parent process. In Python programming, after killing the parent process through signals, the child process still...
