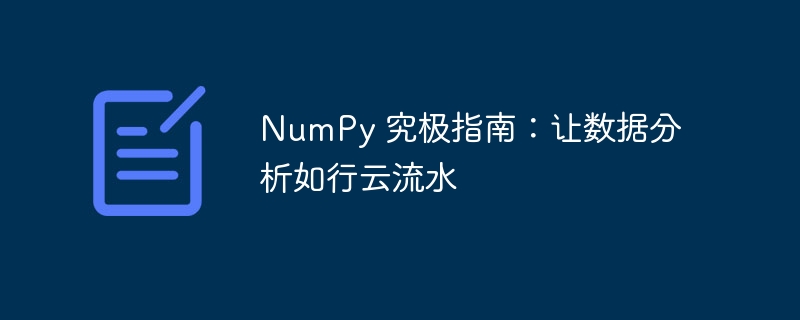
NumPy (Numerical python) is a powerful Python library dedicated to scientific computing and data analysis. It provides a wide range of features to help you efficiently handle multi-dimensional arrays, perform complex mathematical operations, and parse complex data sets.
Core concepts of NumPy
NumPy is built around the following core concepts:
-
ndarray: Multidimensional array is the main data structure for data storage in NumPy.
-
Axis: Dimensions of the array. For example, a 2D array has row and column axes.
-
Data types: NumPy supports a variety of data types, including integers, floating point numbers, strings, and Boolean values.
-
Indexing and slicing: ndarrays can be accessed using indexing and slicing, which provides flexible data access and modification.
Basic operations of NumPy
NumPy provides a series of basic operations, including:
-
Create and initialize arrays:
np.array()
, np.zeros()
, np.ones()
-
Mathematical operations:
np.add()
, np.subtract()
, np.multiply()
, np.divide()
-
Logical operations:
np.greater()
, np.less()
, np.equal()
-
Aggregation functions:
np.sum()
, np.mean()
, np.std()
-
Broadcast: The ability to perform operations on arrays with different shapes.
Advanced features for data science
NumPy also provides advanced features for data science, including:
-
Linear algebra operations: Matrix multiplication, inversion, eigenvalue decomposition.
-
Statistical functions: Histogram, quantile, correlation coefficient.
-
Fourier Transform: Used for frequency analysis and signal processing.
-
Random number generation: Used for simulation and sampling.
-
File I/O: Load and save arrays from a file or database .
Integration of NumPy with other libraries
NumPy is compatible with other popular data science libraries, including:
-
Pandas: Data frame and time series processing.
-
Matplotlib and Seaborn: Data Visualization.
-
Scikit-learn: Machine Learning.
Advantages of NumPy
Using NumPy has the following advantages:
-
Efficient data processing: NumPy is implemented in C language and provides excellent performance.
-
Easy to use: Provides an intuitive and flexible api that is easy to use even for beginners.
-
Extensive ecosystem: Compatible with other data science libraries, extending the functionality of NumPy.
Use cases for NumPy
NumPy is widely used in various data analysis fields, including:
-
Image processing: Image segmentation, feature extraction.
-
Signal processing: Filtering, noise elimination.
-
Machine learning: Feature engineering, model training.
-
Scientific calculation: Numerical integration and differential equation solving.
-
Financial analysis: Stock price modeling, risk management.
in conclusion
NumPy is an essential tool for data analysis and scientific computing. Its power, ease of use, and flexible ecosystem make it ideal for efficiently processing complex data sets and gaining deep understanding of your data. By mastering NumPy, you can take your data analysis to the next level and unleash the power of data to gain valuable insights and drive decisions.
The above is the detailed content of The Ultimate Guide to NumPy: Making Data Analysis Smooth. For more information, please follow other related articles on the PHP Chinese website!