


RV fusion performance is amazing! RCBEVDet: Radar also has spring, the latest SOTA!
Written before&The author’s personal understanding
The main issue this discussion paper focuses on is the application of 3D target detection technology in the process of autonomous driving. Although the development of environmental vision camera technology provides high-resolution semantic information for 3D object detection, this method is limited by issues such as the inability to accurately capture depth information and poor performance in bad weather or low-light conditions. In response to this problem, the discussion proposed a new multi-mode 3D target detection method-RCBEVDet that combines surround-view cameras and economical millimeter-wave radar sensors. This method provides richer semantic information and a solution to problems such as poor performance in bad weather or low-light conditions by comprehensively using information from multiple sensors. In response to this problem, the discussion proposed a new multi-mode 3D target detection method-RCBEVDet that combines surround-view cameras and economical millimeter-wave radar sensors. By comprehensively using information from multi-mode sensors, RCBEVDet is able to provide high-resolution semantic information and exhibit good performance in severe weather or low-light conditions. The core of this method to improve automatic
RCBEVDet lies in two key designs: RadarBEVNet and Cross-Attention Multi-layer Fusion Module (CAMF). RadarBEVNet is designed to efficiently extract radar features and it includes a dual-stream radar backbone network RCS (Radar Cross Section) aware BEV (Bird's Eye View) encoder. Such a design uses point cloud-based and transformer-based encoders to process radar points, update radar point features interactively, and use radar-specific RCS characteristics as prior information of target size to optimize the point feature distribution in BEV space. The CAMF module solves the azimuth error problem of radar points through a multi-modal cross-attention mechanism, achieving dynamic alignment of BEV feature maps of radar and cameras and adaptive fusion of multi-modal features through channel and spatial fusion. In the implementation, the point feature distribution in the BEV space is optimized by interactively updating the radar point features and using the radar-specific RCS characteristics as prior information of the target size. The CAMF module solves the azimuth error problem of radar points through a multi-modal cross-attention mechanism, achieving dynamic alignment of BEV feature maps of radar and cameras and adaptive fusion of multi-modal features through channel and spatial fusion.
The new method proposed in the paper solves the existing problems through the following points:
- Efficient radar feature extractor : Through the dual-stream radar backbone and RCS-aware BEV encoder design, it is specifically optimized for the characteristics of radar data and solves the shortcomings of using an encoder designed for lidar to process radar data.
- Powerful radar-camera feature fusion module: Using a deformed cross-attention mechanism, it effectively handles the spatial misalignment problem between the surround image and the radar input, and improves the fusion effect.
The main contributions of the paper are as follows:
- Proposed a novel radar-camera multi-modal 3D target detector RCBEVDet, which achieves high accuracy, high efficiency and Strongly robust 3D object detection.
- An efficient feature extractor for radar data, RadarBEVNet, is designed, which improves the efficiency and accuracy of feature extraction through dual-stream radar backbone and RCS-aware BEV encoder.
- The Cross-Attention Multi-layer Fusion module is introduced to achieve precise alignment and efficient fusion of radar and camera features through the deformation cross-attention mechanism.
- Achieved new state-of-the-art performance in radar-camera multi-modal 3D object detection on nuScenes and VoD datasets, while achieving an optimal balance between accuracy and speed and demonstrating robustness under sensor failure Good robustness under circumstances.
Detailed explanation of RCBEVDet
##RadarBEVNet
RadarBEVNet is proposed in this paper The network architecture used for effective mine vehicle BEV (bird view) feature extraction mainly includes two core components: a dual-stream radar backbone network and an RCS (radar cross-sectional area) sensing BEV encoder. A dual-stream radar backbone network is used to extract rich feature representations from multi-channel radar data. It is built on a deep convolutional neural network (CNN), alternating between nested convolution and pooling layers for feature extraction and dimensionality reduction operations to gradually obtain extractionDual-stream radar backbone
The dual-stream radar backbone network consists of a point-based backbone and a converter-based backbone. The point-based backbone network learns radar features through multi-layer perceptron (MLP) and maximum pooling operations. The process can be simplified to the following formula: here represents radar point features. After adding feature dimensions through MLP , and then extract global information through the maximum pooling operation and connect it with high-dimensional features. The converter is based on the interference block and introduces the distance modulated attention mechanism (DMSA). By considering the distance information between radar points, it optimizes the model's ability to gather adjacent information and promotes the convergence of the model. The self-attention of the DMSA mechanism can be expressed as:RCS-aware BEV encoder
In order to solve the sparsity problem of BEV features produced by traditional radar BEV encoders, an RCS-aware BEV encoder is proposed. It uses RCS as prior information of target size and spreads radar point features to multiple pixels in the BEV space instead of a single pixel to increase the density of BEV features. This process is implemented through the following formula:
where, is the Gaussian BEV weight map based on RCS, which is optimized by maximizing the weight map of all radar points. Finally, the features obtained by RCS spreading are connected and processed by MLP to obtain the final RCS-aware BEV features.
Overall, RadarBEVNet efficiently extracts the features of radar data by combining a dual-stream radar backbone network and an RCS-aware BEV encoder, and optimizes the feature distribution of the BEV space by using RCS as a priori for target size. , which provides a strong foundation for subsequent multi-modal fusion.
Cross-Attention Multi-layer Fusion Module
Cross-Attention Multi-layer Fusion Module (CAMF) is a An advanced network structure for dynamic alignment and fusion of multi-modal features, especially designed for dynamic alignment and fusion of bird's-eye view (BEV) features generated by radar and cameras. This module mainly solves the problem of feature misalignment caused by the azimuth error of radar point clouds. Through the deformable cross-attention mechanism (Deformable Cross-Attention), it effectively captures the small deviations of radar points and reduces the standard cross-attention. computational complexity.
CAMF utilizes a deformed cross-attention mechanism to align the BEV features of cameras and radars. Given a sum of BEV features for a camera and a radar, learnable position embeddings are first added to sum and then converted to query and reference points as keys and values. The calculation of multi-head deformation cross attention can be expressed as:
where represents the index of the attention head, represents the index of the sampling key, and is the total number of sampling keys. Represents the sampling offset and is the attention weight calculated by and .
After aligning the BEV features of camera and radar through cross-attention, CAMF uses channel and spatial fusion layers to aggregate multi-modal BEV features. Specifically, two BEV features are first concatenated, and then fed into the CBR (convolution-batch normalization-activation function) block and the fused features are obtained through residual connection. The CBR block sequentially consists of a convolutional layer, a batch normalization layer and a ReLU activation function. After that, three CBR blocks are applied consecutively to further fuse multi-modal features.
Through the above process, CAMF effectively achieves precise alignment and efficient fusion of radar and camera BEV features, providing rich and accurate feature information for 3D target detection, thus improving detection performance.
Related experiments
In the comparison of 3D target detection results on the VoD validation set, RadarBEVNet fuses camera and radar data, It shows excellent performance in terms of average precision (mAP) performance within the entire annotation area and within the area of interest. Specifically, for the entire annotated area, RadarBEVNet achieved AP values of 40.63%, 38.86%, and 70.48% respectively in the detection of cars, pedestrians, and cyclists, increasing the comprehensive mAP to 49.99%. In the area of interest, that is, in the driving channel close to the vehicle, RadarBEVNet's performance is even more outstanding, reaching 72.48%, 49.89% and 87.01% AP values in the detection of cars, pedestrians and cyclists respectively, and the comprehensive mAP reached 69.80%.
These results reveal several key points. First, RadarBEVNet can fully utilize the complementary advantages of the two sensors by effectively fusing camera and radar inputs, improving the overall detection performance. Compared with methods that only use radar, such as PointPillar and RadarPillarNet, RadarBEVNet has a significant improvement in comprehensive mAP, which shows that multi-modal fusion is particularly important to improve detection accuracy. Secondly, RadarBEVNet performs particularly well in areas of interest, which is particularly critical for autonomous driving applications because targets in areas of interest usually have the greatest impact on real-time driving decisions. Finally, although the AP value of RadarBEVNet is slightly lower than some single-modal or other multi-modal methods in the detection of cars and pedestrians, RadarBEVNet shows its overall performance advantages in cyclist detection and comprehensive mAP performance. RadarBEVNet achieves excellent performance on the VoD verification set by fusing multi-modal data from cameras and radars, especially demonstrating strong detection capabilities in areas of interest that are critical to autonomous driving, proving its effectiveness as a The potential of 3D object detection methods.
This ablation experiment demonstrates RadarBEVNet’s continued improvement in 3D object detection performance as it gradually adds major components. Starting from the baseline model BEVDepth, the added components at each step significantly improve NDS (core metric, reflecting detection accuracy and completeness) and mAP (average precision, reflecting the model's ability to detect objects).
- Add time information: By introducing time information, NDS and mAP are improved by 4.4 and 5.4 percentage points respectively. This shows that temporal information is very effective in improving the accuracy and robustness of 3D object detection, probably because the temporal dimension provides additional dynamic information that helps the model better understand the dynamic characteristics of the scene and objects.
- Join PointPillar BEVFusion (based on the fusion of radar and camera): This step further improves NDS and mAP, increasing by 1.7 and 1.8 percentage points respectively. This shows that by fusing radar and camera data, the model can obtain a more comprehensive scene understanding, making up for the limitations of single modal data.
- Introducing RadarBEVNet: NDS and mAP increased again by 2.1 and 3.0 percentage points respectively. As an efficient radar feature extractor, RadarBEVNet optimizes the processing of radar data and improves the quality and effectiveness of features, which is crucial to improving the overall detection performance.
- Add CAMF (Cross Attention Multi-layer Fusion Module): Through fine feature alignment and fusion, NDS is increased by 0.7 percentage points, and mAP is slightly improved to 45.6, showing the improvement in feature fusion aspects of effectiveness. Although the improvement in this step is not as significant as the previous steps, it still proves the importance of accurate feature alignment in improving detection performance during the multi-modal fusion process.
- Add time supervision: Finally, after the introduction of time supervision, NDS increased slightly by 0.4 percentage points to 56.8, while mAP decreased slightly by 0.3 percentage points to 45.3. This shows that temporal supervision can further improve the performance of the model in the temporal dimension, although the contribution to mAP may be slightly limited by the impact of specific experimental settings or data distribution.
Overall, this series of ablation experiments clearly demonstrates the contribution of each major component in RadarBEVNet to improving 3D object detection performance, from the introduction of temporal information to complex multi-modality Fusion strategy, each step brings performance improvement to the model. In particular, the sophisticated processing and fusion strategies for radar and camera data prove the importance of multi-modal data processing in complex autonomous driving environments.
Discussion
The RadarBEVNet method proposed in the paper effectively improves the accuracy and robustness of 3D target detection by fusing multi-modal data from cameras and radars. It performs particularly well in complex autonomous driving scenarios. By introducing RadarBEVNet and Cross-Attention Multi-layer Fusion Module (CAMF), RadarBEVNet not only optimizes the feature extraction process of radar data, but also achieves precise feature alignment and fusion between radar and camera data, thereby overcoming the problem of using a single sensor data limitations, such as radar bearing errors and camera performance degradation in low light or adverse weather conditions.
In terms of advantages, the main contribution of RadarBEVNet is its ability to effectively process and utilize complementary information between multi-modal data, improving detection accuracy and system robustness. The introduction of RadarBEVNet makes the processing of radar data more efficient, and the CAMF module ensures the effective fusion of different sensor data, making up for their respective shortcomings. In addition, RadarBEVNet demonstrated excellent performance on multiple data sets in experiments, especially in areas of interest that are crucial in autonomous driving, showing its potential in practical application scenarios.
In terms of shortcomings, although RadarBEVNet has achieved remarkable results in the field of multi-modal 3D target detection, the complexity of its implementation has also increased accordingly, and may require more computing resources and processing time, which to a certain extent Limits its deployment in real-time application scenarios. In addition, although RadarBEVNet performs well in cyclist detection and overall performance, there is still room for improvement in performance on specific categories (such as cars and pedestrians), which may require further algorithm optimization or more efficient feature fusion strategies to solve.
In short, RadarBEVNet has demonstrated significant performance advantages in the field of 3D target detection through its innovative multi-modal fusion strategy. Although there are some limitations, such as higher computational complexity and room for performance improvement on specific detection categories, its potential in improving the accuracy and robustness of autonomous driving systems cannot be ignored. Future work can focus on optimizing the computational efficiency of the algorithm and further improving its performance on various target detections to promote the widespread deployment of RadarBEVNet in actual autonomous driving applications.
Conclusion
The paper introduces RadarBEVNet and Cross-Attention Multi-layer Fusion Module (CAMF) by fusing camera and radar data, showing significant results in the field of 3D target detection. Improved performance, especially in key scenarios of autonomous driving. It effectively utilizes the complementary information between multi-modal data to improve detection accuracy and system robustness. Despite the challenges of high computational complexity and room for performance improvement in some categories, \ours has shown great potential and value in promoting the development of autonomous driving technology, especially in improving the perception capabilities of autonomous driving systems. Future work can focus on optimizing algorithm efficiency and further improving detection performance to better adapt to the needs of real-time autonomous driving applications.
The above is the detailed content of RV fusion performance is amazing! RCBEVDet: Radar also has spring, the latest SOTA!. For more information, please follow other related articles on the PHP Chinese website!

Hot AI Tools

Undresser.AI Undress
AI-powered app for creating realistic nude photos

AI Clothes Remover
Online AI tool for removing clothes from photos.

Undress AI Tool
Undress images for free

Clothoff.io
AI clothes remover

Video Face Swap
Swap faces in any video effortlessly with our completely free AI face swap tool!

Hot Article

Hot Tools

Notepad++7.3.1
Easy-to-use and free code editor

SublimeText3 Chinese version
Chinese version, very easy to use

Zend Studio 13.0.1
Powerful PHP integrated development environment

Dreamweaver CS6
Visual web development tools

SublimeText3 Mac version
God-level code editing software (SublimeText3)

Hot Topics


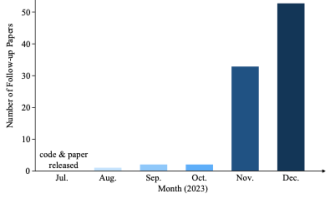
Written above & the author’s personal understanding Three-dimensional Gaussiansplatting (3DGS) is a transformative technology that has emerged in the fields of explicit radiation fields and computer graphics in recent years. This innovative method is characterized by the use of millions of 3D Gaussians, which is very different from the neural radiation field (NeRF) method, which mainly uses an implicit coordinate-based model to map spatial coordinates to pixel values. With its explicit scene representation and differentiable rendering algorithms, 3DGS not only guarantees real-time rendering capabilities, but also introduces an unprecedented level of control and scene editing. This positions 3DGS as a potential game-changer for next-generation 3D reconstruction and representation. To this end, we provide a systematic overview of the latest developments and concerns in the field of 3DGS for the first time.
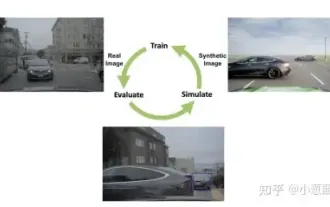
Yesterday during the interview, I was asked whether I had done any long-tail related questions, so I thought I would give a brief summary. The long-tail problem of autonomous driving refers to edge cases in autonomous vehicles, that is, possible scenarios with a low probability of occurrence. The perceived long-tail problem is one of the main reasons currently limiting the operational design domain of single-vehicle intelligent autonomous vehicles. The underlying architecture and most technical issues of autonomous driving have been solved, and the remaining 5% of long-tail problems have gradually become the key to restricting the development of autonomous driving. These problems include a variety of fragmented scenarios, extreme situations, and unpredictable human behavior. The "long tail" of edge scenarios in autonomous driving refers to edge cases in autonomous vehicles (AVs). Edge cases are possible scenarios with a low probability of occurrence. these rare events
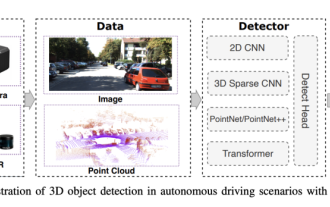
0.Written in front&& Personal understanding that autonomous driving systems rely on advanced perception, decision-making and control technologies, by using various sensors (such as cameras, lidar, radar, etc.) to perceive the surrounding environment, and using algorithms and models for real-time analysis and decision-making. This enables vehicles to recognize road signs, detect and track other vehicles, predict pedestrian behavior, etc., thereby safely operating and adapting to complex traffic environments. This technology is currently attracting widespread attention and is considered an important development area in the future of transportation. one. But what makes autonomous driving difficult is figuring out how to make the car understand what's going on around it. This requires that the three-dimensional object detection algorithm in the autonomous driving system can accurately perceive and describe objects in the surrounding environment, including their locations,
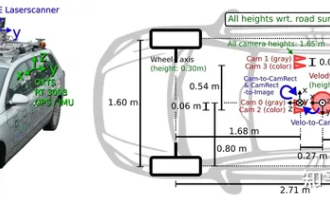
The first pilot and key article mainly introduces several commonly used coordinate systems in autonomous driving technology, and how to complete the correlation and conversion between them, and finally build a unified environment model. The focus here is to understand the conversion from vehicle to camera rigid body (external parameters), camera to image conversion (internal parameters), and image to pixel unit conversion. The conversion from 3D to 2D will have corresponding distortion, translation, etc. Key points: The vehicle coordinate system and the camera body coordinate system need to be rewritten: the plane coordinate system and the pixel coordinate system. Difficulty: image distortion must be considered. Both de-distortion and distortion addition are compensated on the image plane. 2. Introduction There are four vision systems in total. Coordinate system: pixel plane coordinate system (u, v), image coordinate system (x, y), camera coordinate system () and world coordinate system (). There is a relationship between each coordinate system,
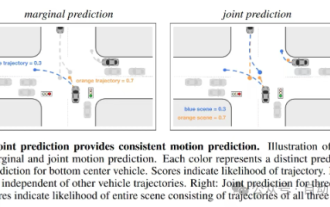
Trajectory prediction plays an important role in autonomous driving. Autonomous driving trajectory prediction refers to predicting the future driving trajectory of the vehicle by analyzing various data during the vehicle's driving process. As the core module of autonomous driving, the quality of trajectory prediction is crucial to downstream planning control. The trajectory prediction task has a rich technology stack and requires familiarity with autonomous driving dynamic/static perception, high-precision maps, lane lines, neural network architecture (CNN&GNN&Transformer) skills, etc. It is very difficult to get started! Many fans hope to get started with trajectory prediction as soon as possible and avoid pitfalls. Today I will take stock of some common problems and introductory learning methods for trajectory prediction! Introductory related knowledge 1. Are the preview papers in order? A: Look at the survey first, p
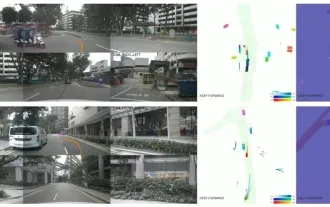
In the past month, due to some well-known reasons, I have had very intensive exchanges with various teachers and classmates in the industry. An inevitable topic in the exchange is naturally end-to-end and the popular Tesla FSDV12. I would like to take this opportunity to sort out some of my thoughts and opinions at this moment for your reference and discussion. How to define an end-to-end autonomous driving system, and what problems should be expected to be solved end-to-end? According to the most traditional definition, an end-to-end system refers to a system that inputs raw information from sensors and directly outputs variables of concern to the task. For example, in image recognition, CNN can be called end-to-end compared to the traditional feature extractor + classifier method. In autonomous driving tasks, input data from various sensors (camera/LiDAR

Original title: SIMPL: ASimpleandEfficientMulti-agentMotionPredictionBaselineforAutonomousDriving Paper link: https://arxiv.org/pdf/2402.02519.pdf Code link: https://github.com/HKUST-Aerial-Robotics/SIMPL Author unit: Hong Kong University of Science and Technology DJI Paper idea: This paper proposes a simple and efficient motion prediction baseline (SIMPL) for autonomous vehicles. Compared with traditional agent-cent
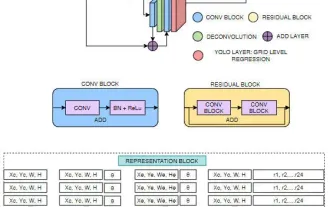
Target detection is a relatively mature problem in autonomous driving systems, among which pedestrian detection is one of the earliest algorithms to be deployed. Very comprehensive research has been carried out in most papers. However, distance perception using fisheye cameras for surround view is relatively less studied. Due to large radial distortion, standard bounding box representation is difficult to implement in fisheye cameras. To alleviate the above description, we explore extended bounding box, ellipse, and general polygon designs into polar/angular representations and define an instance segmentation mIOU metric to analyze these representations. The proposed model fisheyeDetNet with polygonal shape outperforms other models and simultaneously achieves 49.5% mAP on the Valeo fisheye camera dataset for autonomous driving
