


More than 13 times faster than manual work, 'robot + AI' discovers the best electrolyte for batteries and accelerates materials research
Editor | Ziluo
The traditional material research and development model mainly relies on "trial and error" experimental methods or accidental discoveries, and its research and development process generally lasts 10-20 years.
Data-driven methods based on machine learning (ML) can accelerate the design of new materials for clean energy technologies. However, its practical application in materials research is still limited due to the lack of large-scale high-fidelity experimental databases.
Recently, research teams from the Pacific Northwest National Laboratory and Argonne National Laboratory in the United States designed a highly automated workflow that combines a high-throughput experimental platform with the most advanced active learning algorithms to Efficient screening of binary organic solvents with optimal solubility for the anolyte. The goal of this research is to improve the performance and stability of energy storage systems to promote the widespread application of renewable energy. Traditionally, research involving anolytes usually requires a lot of trial and error experiments, which is time-consuming and labor-intensive. Using this automated workflow, researchers can more quickly screen out suitable binary compounds. In addition to an efficient workflow designed to develop high-performance redox flow batteries, this machine learning-guided High-throughput robotic platforms provide a powerful and versatile approach to accelerate the discovery of functional materials.
The reviewer commented: "This study shows that an AI-guided robotic platform can effectively find non-intuitive combinations of solvents and electrolytes in energy applications. This work has important implications for the battery community."
The research is titled "
An integrated high-throughput robotic platform and active learning approach for accelerated discovery of optimal electrolyte formulations" and was published in "Nature Communications" on March 29, 2024 "superior.
Paper link:
for It is crucial to ensure the development of clean energy technology applications and achieve deep decarbonization of electricity, so designing materials with targeted functional properties of tools is critical to developing clean energy technology applications and achieving deep decarbonization of electricity. Traditional trial-and-error methods are costly and time-consuming, so design tools are inherently expensive and time-saving.
The solubility of redox active molecules is an important factor in determining the energy density of redox flow batteries (RFB). However, electrolyte materials discovery is limited by the lack of experimental solubility data sets that are critical to exploit data-driven approaches.
Nonetheless, the development of highly soluble redox-active organic molecules (ROMs) for non-aqueous RFBs (NRFBs) remains a daunting task due to the lack of standardization of organic solvent systems and application-relevant experimental solubility data. task.
By utilizing the automated high-throughput experiment (HTE) platform, the reliability and efficiency of the "excess solute" solubility measurement method can be improved and the NRFB's solubility database can be constructed. However, even with HTE systems, the diversity of potential solvent mixtures makes the screening process more time-consuming and expensive.
Active learning (AL), and specifically Bayesian optimization (BO), has proven to be a reliable method to accelerate the search for electrolytes needed for energy storage applications. Therefore, a closed-loop experimental workflow guided by BO can be used to minimize HTE execution.
ML-guided high-throughput experimental robotic platformHere, researchers used 2,1,3-benzothiadiazole (2,1,3 -benzothiadiazole (BTZ), a high-performance anolyte with a high degree of delocalized charge density and good chemical stability, serves as a model ROM. The focus is on studying its solubility in various organic solvents, demonstrating the potential of a machine learning-guided high-throughput experiment (HTE) robotic platform to accelerate NRFB electrolyte discovery.
Illustration: Schematic diagram of the closed-loop electrolyte screening process based on a high-throughput experimental platform guided by machine learning (ML). (Source: paper)
Specifically, the researchers designed a closed-loop solvent screening workflow consisting of two connected modules, HTE and BO. The HTE module performs sample preparation and solubility measurements via a high-throughput robotic platform. The BO component consists of a surrogate model and an acquisition function, which together act as an oracle, making solubility predictions and suggesting new solvents for evaluation.
The workflow is shown in the figure below, the specific steps are:More than 13 times faster than manual sample processing The automated platform can prepare saturated solutions with solute excess and quantitative nuclear magnetic resonance (qNMR) with minimal manual intervention ) sample. With the automated HTE workflow, the total experimental time to complete the solubility measurements of 42 samples was approximately 27 hours (~39 minutes/sample, less time per sample when running more samples). This is more than 13 times faster than manually processing samples using the "excess solute" method (approximately 525 minutes per sample). In addition to the speed increase provided by the HTE system, research also placed great emphasis on controlling experimental conditions, such as temperature (20°C) and stabilization time (8 hours), to ensure accurate measurement of BTZ solubility in various organic solvents . Illustration: Overview of the automated high-throughput experiment (HTE) platform. (Source: Paper) Based on a literature review and consideration of solvent properties, the researchers listed 22 potential candidate solvents for BTZ. Then, an additional 2079 binary solvents were further enumerated by combining these 22 single solvents in pairs, each with 9 different volume fractions. Table: List of 22 candidate organic solvents and their physical and chemical properties. (Source: paper) #The platform identifies multiple solvents from a comprehensive library of more than 2000 potential solvents, with prototype redox active molecules2,1,3 -The solubility threshold of benzothiadiazole exceeds 6.20 M. Notably, the comprehensive strategy required solubility assessment for less than 10% of drug candidates, highlighting the efficiency of the new approach. Illustration: Identification of required electrolytes via Bayesian Optimization (BO). (Source: paper) The research results also show that binary solvent mixtures, especially those incorporating 1,4-dioxane (1,4-dioxane), help improve the BTZ solubility. In conclusion, the study demonstrates an ML-guided HTE platform for electrolyte screening, where ML predictions and automated experiments work together to effectively screen binary organic solvents with optimal solubility for BTZ. This research not only helps connect the fields of data science and traditional experimental science, but also lays the foundation for the future development of an autonomous platform dedicated to battery electrolyte screening.
The above is the detailed content of More than 13 times faster than manual work, 'robot + AI' discovers the best electrolyte for batteries and accelerates materials research. For more information, please follow other related articles on the PHP Chinese website!

Hot AI Tools

Undresser.AI Undress
AI-powered app for creating realistic nude photos

AI Clothes Remover
Online AI tool for removing clothes from photos.

Undress AI Tool
Undress images for free

Clothoff.io
AI clothes remover

AI Hentai Generator
Generate AI Hentai for free.

Hot Article

Hot Tools

Notepad++7.3.1
Easy-to-use and free code editor

SublimeText3 Chinese version
Chinese version, very easy to use

Zend Studio 13.0.1
Powerful PHP integrated development environment

Dreamweaver CS6
Visual web development tools

SublimeText3 Mac version
God-level code editing software (SublimeText3)

Hot Topics


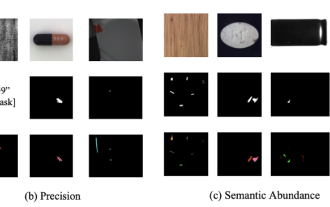
In modern manufacturing, accurate defect detection is not only the key to ensuring product quality, but also the core of improving production efficiency. However, existing defect detection datasets often lack the accuracy and semantic richness required for practical applications, resulting in models unable to identify specific defect categories or locations. In order to solve this problem, a top research team composed of Hong Kong University of Science and Technology Guangzhou and Simou Technology innovatively developed the "DefectSpectrum" data set, which provides detailed and semantically rich large-scale annotation of industrial defects. As shown in Table 1, compared with other industrial data sets, the "DefectSpectrum" data set provides the most defect annotations (5438 defect samples) and the most detailed defect classification (125 defect categories
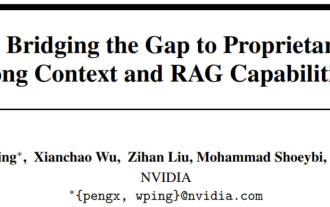
The open LLM community is an era when a hundred flowers bloom and compete. You can see Llama-3-70B-Instruct, QWen2-72B-Instruct, Nemotron-4-340B-Instruct, Mixtral-8x22BInstruct-v0.1 and many other excellent performers. Model. However, compared with proprietary large models represented by GPT-4-Turbo, open models still have significant gaps in many fields. In addition to general models, some open models that specialize in key areas have been developed, such as DeepSeek-Coder-V2 for programming and mathematics, and InternVL for visual-language tasks.
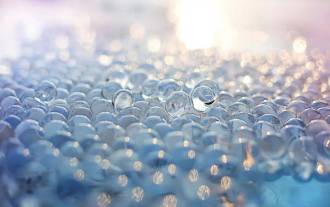
Editor |KX To this day, the structural detail and precision determined by crystallography, from simple metals to large membrane proteins, are unmatched by any other method. However, the biggest challenge, the so-called phase problem, remains retrieving phase information from experimentally determined amplitudes. Researchers at the University of Copenhagen in Denmark have developed a deep learning method called PhAI to solve crystal phase problems. A deep learning neural network trained using millions of artificial crystal structures and their corresponding synthetic diffraction data can generate accurate electron density maps. The study shows that this deep learning-based ab initio structural solution method can solve the phase problem at a resolution of only 2 Angstroms, which is equivalent to only 10% to 20% of the data available at atomic resolution, while traditional ab initio Calculation
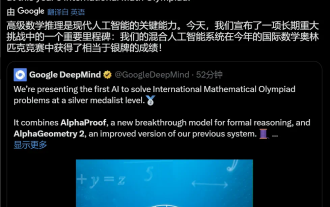
For AI, Mathematical Olympiad is no longer a problem. On Thursday, Google DeepMind's artificial intelligence completed a feat: using AI to solve the real question of this year's International Mathematical Olympiad IMO, and it was just one step away from winning the gold medal. The IMO competition that just ended last week had six questions involving algebra, combinatorics, geometry and number theory. The hybrid AI system proposed by Google got four questions right and scored 28 points, reaching the silver medal level. Earlier this month, UCLA tenured professor Terence Tao had just promoted the AI Mathematical Olympiad (AIMO Progress Award) with a million-dollar prize. Unexpectedly, the level of AI problem solving had improved to this level before July. Do the questions simultaneously on IMO. The most difficult thing to do correctly is IMO, which has the longest history, the largest scale, and the most negative
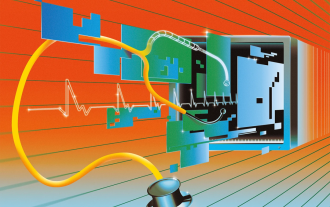
Editor | ScienceAI Based on limited clinical data, hundreds of medical algorithms have been approved. Scientists are debating who should test the tools and how best to do so. Devin Singh witnessed a pediatric patient in the emergency room suffer cardiac arrest while waiting for treatment for a long time, which prompted him to explore the application of AI to shorten wait times. Using triage data from SickKids emergency rooms, Singh and colleagues built a series of AI models that provide potential diagnoses and recommend tests. One study showed that these models can speed up doctor visits by 22.3%, speeding up the processing of results by nearly 3 hours per patient requiring a medical test. However, the success of artificial intelligence algorithms in research only verifies this
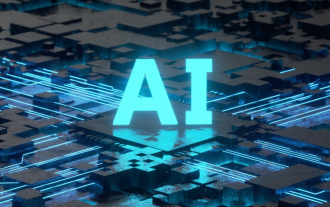
Editor |ScienceAI Question Answering (QA) data set plays a vital role in promoting natural language processing (NLP) research. High-quality QA data sets can not only be used to fine-tune models, but also effectively evaluate the capabilities of large language models (LLM), especially the ability to understand and reason about scientific knowledge. Although there are currently many scientific QA data sets covering medicine, chemistry, biology and other fields, these data sets still have some shortcomings. First, the data form is relatively simple, most of which are multiple-choice questions. They are easy to evaluate, but limit the model's answer selection range and cannot fully test the model's ability to answer scientific questions. In contrast, open-ended Q&A
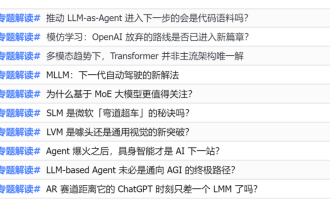
In 2023, almost every field of AI is evolving at an unprecedented speed. At the same time, AI is constantly pushing the technological boundaries of key tracks such as embodied intelligence and autonomous driving. Under the multi-modal trend, will the situation of Transformer as the mainstream architecture of AI large models be shaken? Why has exploring large models based on MoE (Mixed of Experts) architecture become a new trend in the industry? Can Large Vision Models (LVM) become a new breakthrough in general vision? ...From the 2023 PRO member newsletter of this site released in the past six months, we have selected 10 special interpretations that provide in-depth analysis of technological trends and industrial changes in the above fields to help you achieve your goals in the new year. be prepared. This interpretation comes from Week50 2023
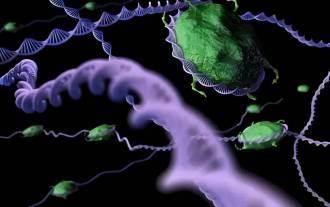
Editor | KX In the field of drug research and development, accurately and effectively predicting the binding affinity of proteins and ligands is crucial for drug screening and optimization. However, current studies do not take into account the important role of molecular surface information in protein-ligand interactions. Based on this, researchers from Xiamen University proposed a novel multi-modal feature extraction (MFE) framework, which for the first time combines information on protein surface, 3D structure and sequence, and uses a cross-attention mechanism to compare different modalities. feature alignment. Experimental results demonstrate that this method achieves state-of-the-art performance in predicting protein-ligand binding affinities. Furthermore, ablation studies demonstrate the effectiveness and necessity of protein surface information and multimodal feature alignment within this framework. Related research begins with "S
