1. Overall architecture of LLM-based Agent
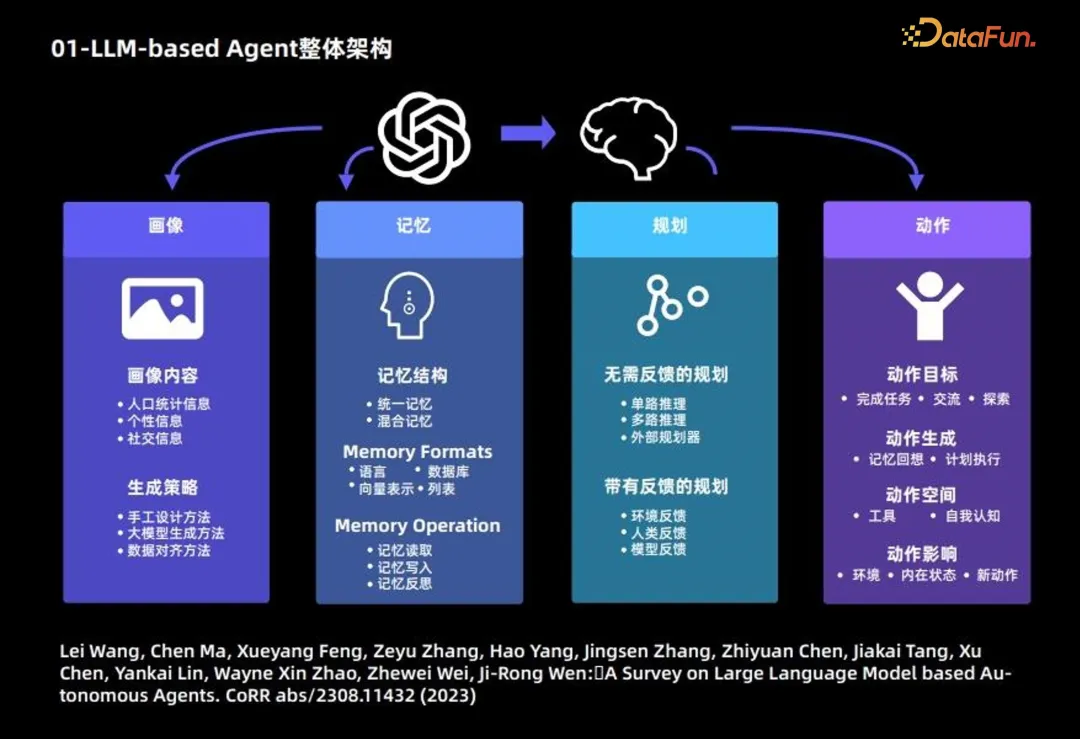
The composition of the large language model Agent is mainly divided into the following 4 Modules:
1. Portrait module: mainly describes the background information of the Agent
The following introduces the main content and generation strategy of the portrait module.
Portrait content is mainly based on 3 types of information: demographic information, personality information and social information.
Generation strategy: 3 strategies are mainly used to generate portrait content:
- Manual design method: Specify by yourself method to write the content of the user portrait into the prompt of the large model; suitable for situations where the number of Agents is relatively small;
- Large model generation method: first specify Use a small number of portraits as examples, and then use a large language model to generate more portraits; suitable for situations with a large number of Agents;
- Data alignment method: required Based on the background information of the characters in the pre-specified data set as the prompt of the large language model, corresponding predictions are made.
2. Memory module: The main purpose is to record Agent behavior and provide support for future Agent decisions
Memory Structure:
- Unified memory: only short-term memory is considered, long-term memory is not considered.
- Hybrid Memory: A combination of long-term and short-term memory.
#Memory form: Mainly based on the following 4 forms.
- Language
- ##Database
- Vector representation
- List
Memory content: Common following 3 operations:
- Memory reading
- Memory writing
- Memory reflection
3. Planning module
- Planning without feedback: The large language model does not require feedback from the external environment during the reasoning process. This type of planning is further subdivided into three types: single-channel based reasoning, which uses a large language model only once to completely output the steps of reasoning; multi-channel based reasoning, drawing on the idea of crowdsourcing, allowing the large language model to generate multiple Reason the path and determine the best path; borrow an external planner.
- Planning with feedback: This planning method requires feedback from the external environment, while the large language model requires feedback from the environment for the next step and subsequent planning. . Providers of this type of planning feedback come from three sources: environmental feedback, human feedback, and model feedback.
4. Action module
- Action goal: The goal of some Agents is to complete a certain There are several tasks, some are communication and some are exploration.
- Action generation: Some agents rely on memory recall to generate actions, and some perform specific actions according to the original plan.
- Action space: Some action spaces are a collection of tools, and some are based on the large language model's own knowledge, considering the entire action space from the perspective of self-awareness.
- Action impact: including the impact on the environment, the impact on the internal state, and the impact on new actions in the future.
The above is the overall framework of Agent. For more information, please refer to the following papers:
Lei Wang, Chen Ma, Xueyang Feng, Zeyu Zhang, Hao Yang, Jingsen Zhang, Zhiyuan Chen, Jiakai Tang, Xu Chen, Yankai Lin, Wayne Xin Zhao, Zhewei Wei, Ji-Rong Wen: A Survey on Large Language Model based Autonomous Agents. CoRR abs /2308.11432 (2023)
2. Key & Difficult Issues of LLM-based Agent
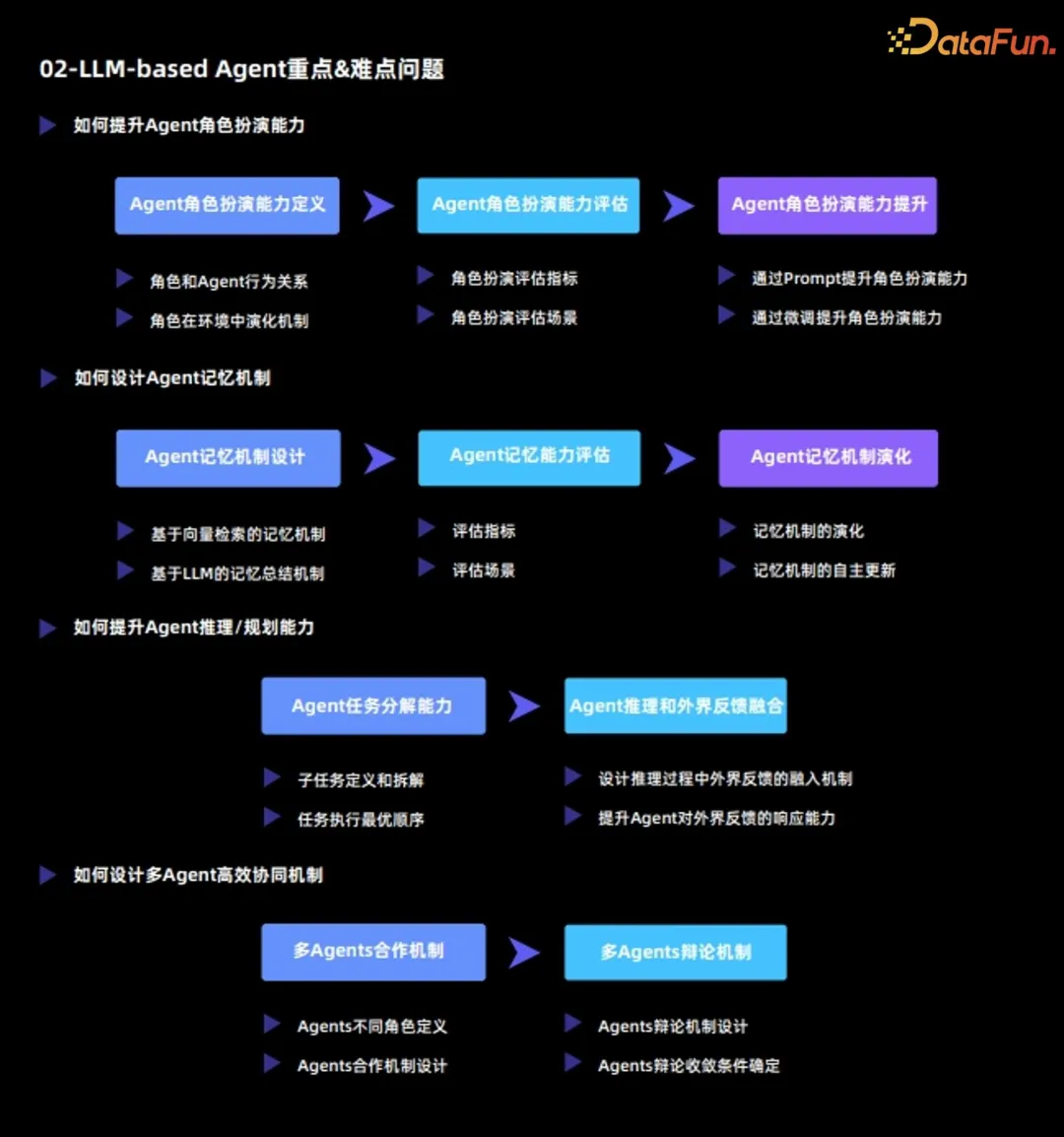
##The key and difficult issues of current large language model Agent mainly include:
1. How to improve Agent’s role-playing ability
The most important function of Agent is to complete specific tasks by playing a certain role , or complete various simulations, so the Agent's role-playing ability is crucial.
(1) Agent role-playing ability definition
Agent role-playing ability is divided into two dimensions:
- The behavioral relationship between the role and the Agent
- The evolution mechanism of the role in the environment
(2)Agent role-playing ability evaluation
After defining the role-playing ability, the next step is to evaluate the Agent role-playing ability from the following two aspects :
- Role-playing evaluation index
- Role-playing evaluation scenario
(3) Improvement of Agent’s role-playing ability
On the basis of the evaluation, the Agent’s role-playing ability needs to be further improved. There are the following two methods:
- Improve role-playing capabilities through prompts: The essence of this method is to stimulate the ability of the original large language model by designing prompts;
- Improve role-playing capabilities through fine-tuning: This method is usually based on external data and re-finetune the large language model to improve role-playing capabilities.
2. How to design the Agent memory mechanism
The biggest difference between Agent and large language model is that Agent can The environment is constantly undergoing self-evolution and self-learning; in this, the memory mechanism plays a very important role. Analyze the Agent's memory mechanism from three dimensions:
(1) Agent memory mechanism design
The following two common memory mechanisms are common:
- Memory mechanism based on vector retrieval
- Memory mechanism based on LLM summary
(2) Agent memory ability evaluation
To evaluate the Agent’s memory ability, it is mainly necessary to determine the following two points:
- Evaluation indicators
- Evaluation scenarios
(3) Agent memory mechanism evolution
Finally, the evolution of Agent memory mechanism needs to be analyzed, including:
- The evolution of memory mechanism
- Autonomous update of memory mechanism
3. How to improve Agent’s reasoning/planning ability
(1) Agent’s task decomposition ability
- Sub-task definition and disassembly
- Optimal order of task execution
(2) Integration of Agent reasoning and external feedback
- Design the integration mechanism of external feedback during the reasoning process: let the Agent and the environment form an interactive whole;
- Improve the Agent's ability to respond to external feedback: On the one hand, the Agent needs to truly respond to the external environment, and on the other hand, the Agent needs to be able to ask questions and seek solutions to the external environment.
4. How to design an efficient multi-Agent collaboration mechanism
(1) Multi-Agents collaboration mechanism
- Agents different role definition
- Agents cooperation mechanism design
(2) Multi-Agents Debate Mechanism
- Agents Debate Mechanism Design
- Agents Debate Convergence Condition Determination
##3. Based on the big User behavior simulation agent of language model
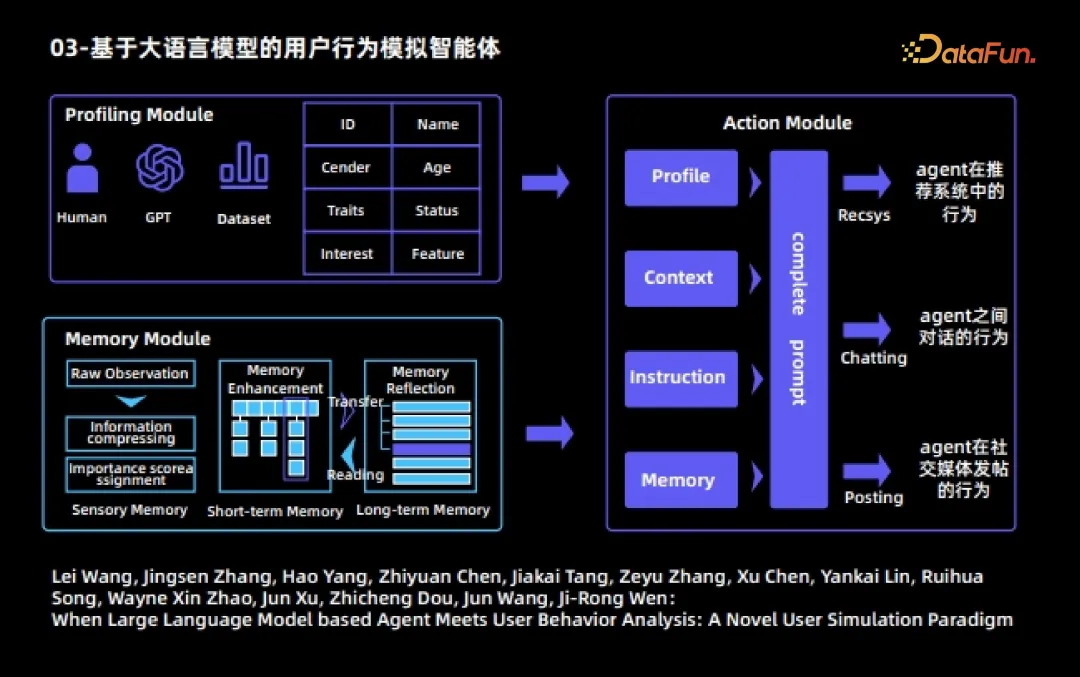
The following will give some actual cases of Agent. The first is a user behavior simulation agent based on a large language model. This agent is also an early work in combining large language model agents with user behavior analysis. In this work, each Agent is divided into three modules:
1. The portrait module
specifies different attributes for different Agents. Such as ID, name, occupation, age, interests and characteristics, etc.
2. Memory module
The memory module includes three sub-modules
(1) Feeling Memory
(2) Short-term memory
- After processing the objectively observed raw observation, the amount of information is generated Higher observations are stored in short-term memory;
- #The storage time of short-term memory contents is relatively short
(3) Long-term memory
- #The content of short-term memory will be automatically transferred to long-term memory after repeated triggering and activation.
- The storage time of long-term memory contents is relatively long
- The contents of long-term memory will be stored according to the Existing memories are subject to independent reflection, sublimation and refinement.
3. Action module
Each Agent can perform three actions:
- Agent’s behavior in the recommendation system, including watching movies, finding the next page, and leaving the recommendation system;
- ## The behavior of conversations between #Agents;
- #Agent’s behavior of posting on social media.
During the entire simulation process, an Agent can freely choose three actions in each round of actions without external interference; we can see Different Agents will talk to each other and autonomously produce various behaviors in social media or recommendation systems. After multiple rounds of simulations, we can observe some interesting social phenomena and the behavior of users on the Internet. law.
For more information, please refer to the following papers:
Lei Wang, Jingsen Zhang, Hao Yang, Zhiyuan Chen, Jiakai Tang, Zeyu Zhang, Xu Chen, Yankai Lin, Ruihua Song, Wayne Xin Zhao, Jun Xu, Zhicheng Dou, Jun Wang, Ji-Rong Wen :When Large Language Model based Agent Meets User Behavior Analysis: A Novel User Simulation Paradigm
4. Based on Multi-agent software development for large language models
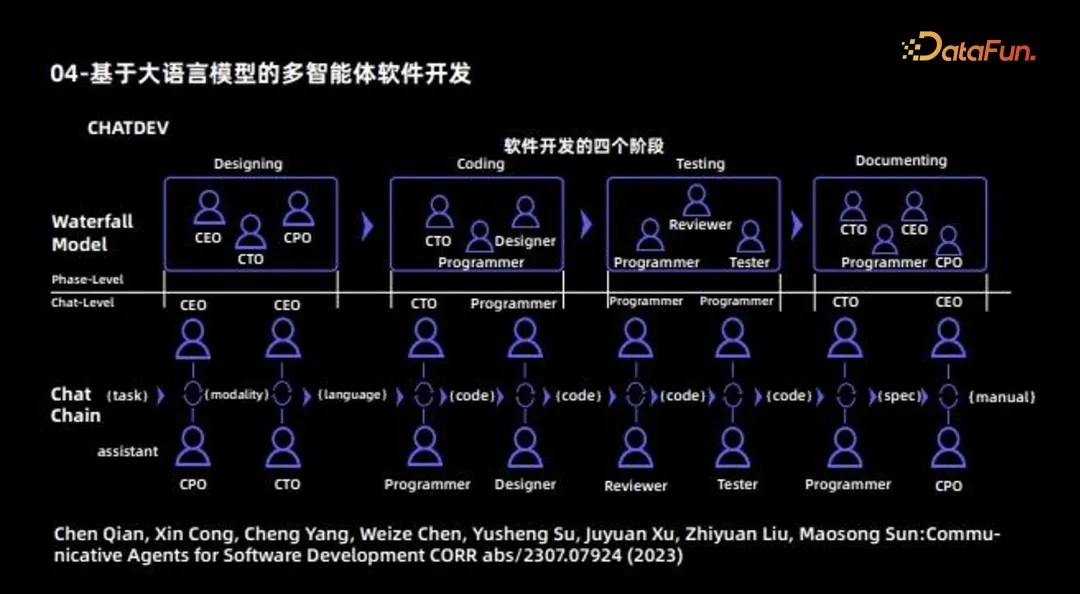
The next Agent example is software development using multi-Agent. This work is also an early work of multi-Agent cooperation, and its main purpose is to use different Agents to develop a complete software. Therefore, it can be regarded as a software company, and different Agents will play different roles: some Agents are responsible for design, including roles such as CEO, CTO, CPO, etc.; some Agents are responsible for coding, and some Agents are mainly responsible for testing; in addition, there are Some agents are responsible for writing documents. In this way, different Agents are responsible for different tasks; finally, the cooperation mechanism between Agents is coordinated and updated through communication, and finally a complete software development process is completed.
#5. Future direction of LLM-based Agent
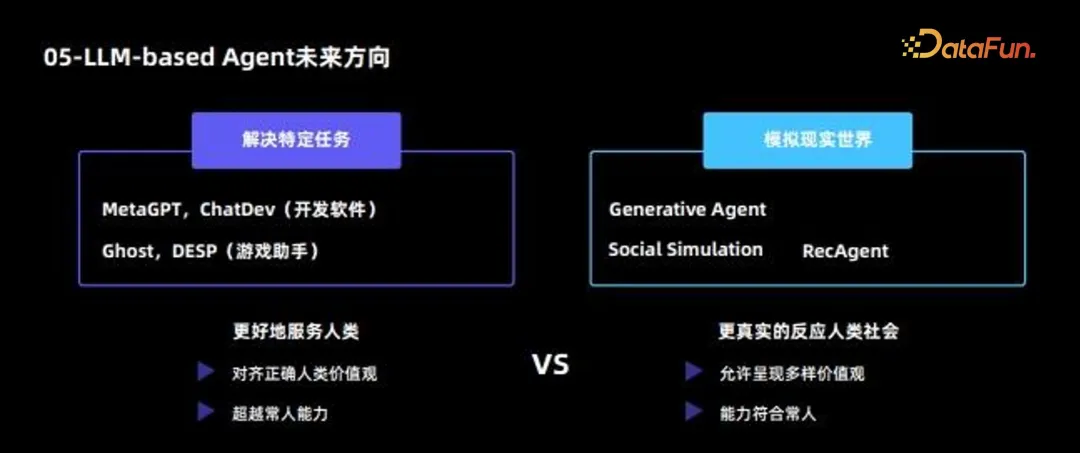
Agents of large language models can currently be divided into two major directions:
Solve specific tasks, such as MetaGPT, ChatDev, Ghost, DESP, etc.-
This type of Agent should ultimately be a "superman" aligned with the correct values of mankind, which has two "qualifiers":
Aligned correctly Human values;
#beyond the capabilities of ordinary people.
Simulate the real world, such as Generative Agent, Social Simulation, RecAgent, etc.-
The abilities required by this type of Agent are completely opposite to the first type. .
Allow Agent to present a variety of values;
We hope that Agent will try to conform to ordinary people instead of going beyond ordinary people.
In addition, the current large language model Agent has the following two pain points:
Illusion problem-
Since the Agent needs to continuously interact with the environment, the hallucinations of each step will be accumulated, which will produce a cumulative effect and make the problem more serious; therefore, the hallucination problem of large models needs further attention here. The solutions include:
Design an efficient human-machine collaboration framework;
Design Plan an efficient human intervention mechanism.
Efficiency issues-
In the simulation process, efficiency is a very important issue; the following table summarizes the time consumption of different Agents under different API numbers.
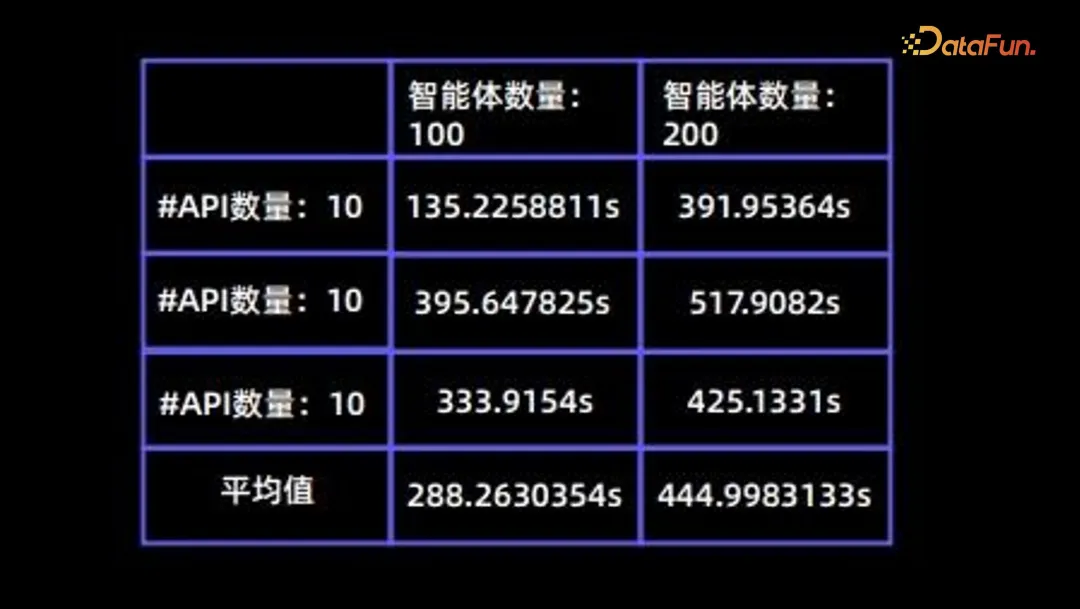
The above is the content shared this time, thank you all.
The above is the detailed content of Al Agent--An important implementation direction in the era of large models. For more information, please follow other related articles on the PHP Chinese website!