


CVPR 2024 high-scoring paper: New generative editing framework GenN2N, unifying NeRF conversion tasks
#The AIxiv column of our website is a column about academic and technical content. In the past few years, the AIxiv column on our website has received more than 2,000 pieces of content, covering top laboratories from major universities and companies around the world, helping to promote academic exchanges and dissemination. If you have excellent work that you want to share, please feel free to contribute or contact us for reporting. The submission email address is liyazhou@jiqizhixin.com; zhaoyunfeng@jiqizhixin.com.
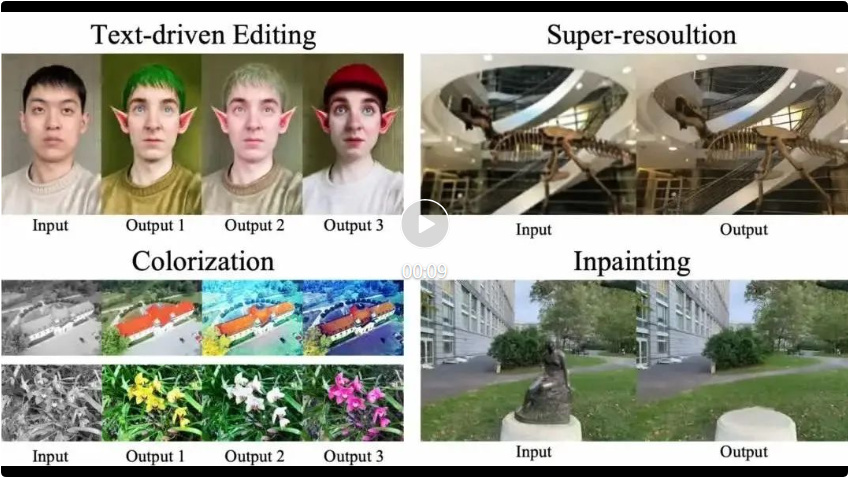
Paper address: https://arxiv.org/abs/2404.02788 Paper homepage: https://xiangyueliu.github.io/GenN2N/ -
Github address: https://github.com/Lxiangyue/GenN2N Paper title: GenN2N: Generative NeRF2NeRF Translation
(positive sample) in the training data, we select an edited picture of the same perspective from the training data Picture
is used as a condition, which prevents the discriminator from being interfered by perspective factors when distinguishing positive and negative samples.
B.NeRF coloring
C.NeRF Super Resolution
D.NeRF Repair
The above is the detailed content of CVPR 2024 high-scoring paper: New generative editing framework GenN2N, unifying NeRF conversion tasks. For more information, please follow other related articles on the PHP Chinese website!

Hot AI Tools

Undresser.AI Undress
AI-powered app for creating realistic nude photos

AI Clothes Remover
Online AI tool for removing clothes from photos.

Undress AI Tool
Undress images for free

Clothoff.io
AI clothes remover

AI Hentai Generator
Generate AI Hentai for free.

Hot Article

Hot Tools

Notepad++7.3.1
Easy-to-use and free code editor

SublimeText3 Chinese version
Chinese version, very easy to use

Zend Studio 13.0.1
Powerful PHP integrated development environment

Dreamweaver CS6
Visual web development tools

SublimeText3 Mac version
God-level code editing software (SublimeText3)

Hot Topics
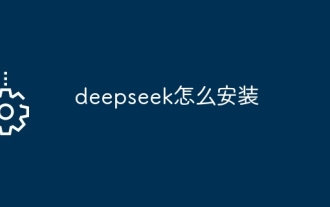
There are many ways to install DeepSeek, including: compile from source (for experienced developers) using precompiled packages (for Windows users) using Docker containers (for most convenient, no need to worry about compatibility) No matter which method you choose, Please read the official documents carefully and prepare them fully to avoid unnecessary trouble.
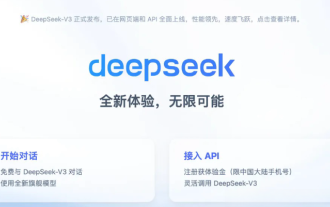
DeepSeekAI Tool User Guide and FAQ DeepSeek is a powerful AI intelligent tool. This article will answer some common usage questions to help you get started quickly. FAQ: The difference between different access methods: There is no difference in function between web version, App version and API calls, and App is just a wrapper for web version. The local deployment uses a distillation model, which is slightly inferior to the full version of DeepSeek-R1, but the 32-bit model theoretically has 90% full version capability. What is a tavern? SillyTavern is a front-end interface that requires calling the AI model through API or Ollama. What is breaking limit
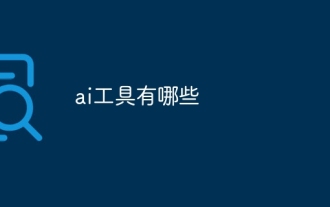
AI tools include: Doubao, ChatGPT, Gemini, BlenderBot, etc.
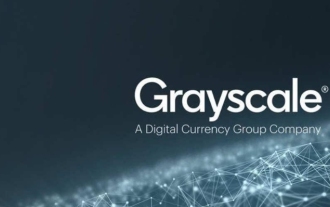
Grayscale Investment: The channel for institutional investors to enter the cryptocurrency market. Grayscale Investment Company provides digital currency investment services to institutions and investors. It allows investors to indirectly participate in cryptocurrency investment through the form of trust funds. The company has launched several crypto trusts, which has attracted widespread market attention, but the impact of these funds on token prices varies significantly. This article will introduce in detail some of Grayscale's major crypto trust funds. Grayscale Major Crypto Trust Funds Available at a glance Grayscale Investment (founded by DigitalCurrencyGroup in 2013) manages a variety of crypto asset trust funds, providing institutional investors and high-net-worth individuals with compliant investment channels. Its main funds include: Zcash (ZEC), SOL,
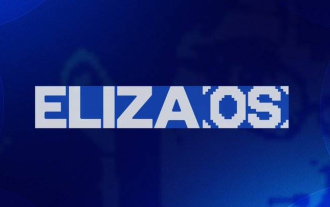
ElizaOSv2: Empowering AI and leading the new economy of Web3. AI is evolving from auxiliary tools to independent entities. ElizaOSv2 plays a key role in it, which gives AI the ability to manage funds and operate Web3 businesses. This article will dive into the key innovations of ElizaOSv2 and how it shapes an AI-driven future economy. AI Automation: Going to independently operate ElizaOS was originally an AI framework focusing on Web3 automation. v1 version allows AI to interact with smart contracts and blockchain data, while v2 version achieves significant performance improvements. Instead of just executing simple instructions, AI can independently manage workflows, operate business and develop financial strategies. Architecture upgrade: Enhanced A
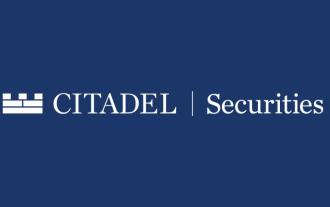
The entry of top market maker Castle Securities into Bitcoin market maker is a symbol of the maturity of the Bitcoin market and a key step for traditional financial forces to compete for future asset pricing power. At the same time, for retail investors, it may mean the gradual weakening of their voice. On February 25, according to Bloomberg, Citadel Securities is seeking to become a liquidity provider for cryptocurrencies. The company aims to join the list of market makers on various exchanges, including exchanges operated by CoinbaseGlobal, BinanceHoldings and Crypto.com, people familiar with the matter said. Once approved by the exchange, the company initially planned to set up a market maker team outside the United States. This move is not only a sign
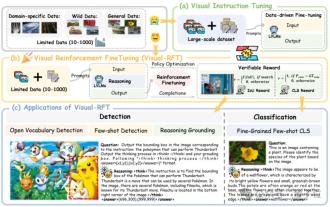
Researchers from Shanghai Jiaotong University, Shanghai AILab and the Chinese University of Hong Kong have launched the Visual-RFT (Visual Enhancement Fine Tuning) open source project, which requires only a small amount of data to significantly improve the performance of visual language big model (LVLM). Visual-RFT cleverly combines DeepSeek-R1's rule-based reinforcement learning approach with OpenAI's reinforcement fine-tuning (RFT) paradigm, successfully extending this approach from the text field to the visual field. By designing corresponding rule rewards for tasks such as visual subcategorization and object detection, Visual-RFT overcomes the limitations of the DeepSeek-R1 method being limited to text, mathematical reasoning and other fields, providing a new way for LVLM training. Vis
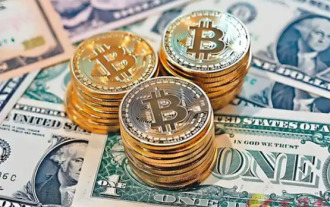
Weekly Observation: Businesses Hoarding Bitcoin – A Brewing Change I often point out some overlooked market trends in weekly memos. MicroStrategy's move is a stark example. Many people may say, "MicroStrategy and MichaelSaylor are already well-known, what are you going to pay attention to?" This is true, but many investors regard it as a special case and ignore the deeper market forces behind it. This view is one-sided. In-depth research on the adoption of Bitcoin as a reserve asset in recent months shows that this is not an isolated case, but a major trend that is emerging. I predict that in the next 12-18 months, hundreds of companies will follow suit and buy large quantities of Bitcoin
