


Artificial Intelligence in Medical Diagnostics Market to Reach $4 Billion by 2028
In healthcare, where accuracy and speed are critical, the integration of artificial intelligence (AI) has become a transformative force. The market for artificial intelligence in medical diagnostics was once an emerging niche, but it has quickly grown into a powerful market with forecasts reaching billions of dollars. The artificial intelligence market size in medical diagnosis will be worth US$1.25 billion in revenue in 2023 and is expected to reach US$4.48 billion by 2028, growing at a CAGR of 29.04% during the forecast period.
The growth of artificial intelligence in medical diagnostics market is driven by several key factors:
Growing demand for artificial intelligence-based solutions:
As the field of modern healthcare continues to evolve and new diseases and conditions are discovered, the demand for artificial intelligence-based medical diagnostic solutions is increasing. Coupled with an aging population and the growing prevalence of chronic diseases, most health systems around the world are struggling to provide high-quality, timely, and accurate diagnosis and treatment with limited resources. Artificial intelligence and the ability to process large amounts of data in real time, including medical images and patient histories, could revolutionize this approach. Using complex algorithms and machine learning, artificial intelligence essentially teaches itself to recognize patterns, providing doctors with valuable information and greatly improving the accuracy and efficiency of diagnosis. This in turn ensures that patients are detected early and treated thoroughly, while enabling health staff to handle the most critical aspects of patient care.
Government Initiatives:
Governments are working towards leveraging the potential of AI to transform the healthcare sector and ensuring an enabling environment is created to promote the integration of AI in medical diagnostics. The government is investing heavily in various initiatives, including R&D funding, enacting favorable regulations, investing in key projects, etc. All these initiatives not only drive innovation but also support public and private sector collaboration, ensuring the rapid growth of the industry. The Government is ensuring that the potential and utility of AI in medical diagnosis is fully exploited as it will lead to better treatment outcomes and more effective healthcare, which will ultimately lead to a healthier society.
Funding for AI-based startups:
Funding has been a key factor in the development of the AI-based medical diagnostics market. Due to the potential benefits of AI technology to the healthcare industry, venture capital firms and individual investors have provided significant financial support to consumer-focused startups. This allows companies to channel funds into research, technology development and commercialization, stimulating intense growth in the industry. Additionally, access to funding enables startups to hire highly qualified professionals and partner with large healthcare institutions to scale their operations, thus driving the adoption of AI solutions across the board.
Cross-Industry Partnerships:
Cross-industry collaboration is a powerful driver of innovation when it comes to AI-powered diagnostic systems. Technology businesses bring advanced algorithms and data analysis capabilities, while healthcare provides domain knowledge and clinical expertise. Collaboration can pool resources to create the most effective AI-driven diagnostic systems that continuously improve patients’ quality of life and work support, thereby saving time and resources.
Emerging Markets:
Emerging markets have good growth prospects for the adoption of AI-based medical diagnostic tools as the healthcare infrastructure in these countries is in the stage of modernizing and awareness is growing to the potential of artificial intelligence in enhancing medical services. Population growth trends in emerging markets indicate high acceptance of emerging health technologies and the need for sustainable solutions to enhance the delivery of health care to their populations. Additionally, its growing resource base, such as capital and expertise, can leverage these markets to enable new applications that leverage artificial intelligence. AI-based medical diagnostics offer promising business growth opportunities in emerging markets to democratize healthcare.
Market constraints for artificial intelligence in the medical diagnostics market include:
Medical personnel are reluctant:
Despite the development of artificial intelligence-based technology tools, many medical practitioners Still showing reluctance to adopt. In many cases, this reluctance is based on unfamiliarity with AI’s capabilities and concerns that machines might replace human labor. To break down this barrier and eliminate the importance of education, efforts such as education and training should be focused on practitioners. These projects should not only highlight the potential of AI to improve diagnostic accuracy and efficiency, but also expose practitioners to AI-based tools to reduce redundancy and increase confidence. By eliminating knowledge gaps and redundancy, practitioners may use AI technology as an asset in diagnostics to benefit patients.
Ambiguous regulatory guidelines:
The existence of unclear or vague regulatory guidelines poses a major challenge to the widespread application of artificial intelligence in medical diagnosis. Healthcare providers and patients may be reluctant to accept new innovations in medicine without clear guidelines regarding the development and implementation of this technology. The safe and ethical application of AI in healthcare can only be achieved through comprehensive legislation that regulates the practice of AI and gives all stakeholders confidence in operational processes. Therefore, regulators, health practitioners and other industry experts must work together to ensure laws and guidance are implemented to address these issues while encouraging innovation. Only through such guidance can technological innovation be effectively applied to medical diagnosis while realizing its full potential.
Limited Interoperability of Artificial Intelligence Solutions:
Interoperability is a critical challenge that needs to be addressed in order to facilitate the smooth incorporation of AI technologies into current healthcare systems. The lack of standard protocols and interoperable options to exchange and share data across platforms limits the full potential of AI in medical diagnostics. To remove this barrier, industry players must collaborate to design standard protocols that can be used for integration and interoperability.
Procurement Costs and Maintenance:
The high acquisition costs and ongoing maintenance requirements associated with AI-based diagnostic systems pose financial challenges to healthcare providers, especially those with limited budgets Healthcare Provider. These costs may include not only the initial investment in AI technology, but also expenses related to training, upgrades, and ongoing support. To overcome this obstacle, efforts must be made to reduce procurement costs and streamline implementation procedures. This may involve exploring cost-effective solutions such as cloud-based platforms or subscription models, as well as leveraging economies of scale through bulk purchasing or collaborative purchasing programs. Additionally, providers can benefit from partnering with an AI vendor that offers comprehensive support services and flexible pricing options, which can help reduce financial barriers and facilitate the adoption of AI in medical diagnostics.
Unstructured Healthcare Data:
The unstructured nature of healthcare data poses a significant obstacle to artificial intelligence systems, which are often optimized for processing structured data formats. Medical records, imaging studies, and other healthcare data often contain free-text annotations, images, and other unstructured information, making it difficult for AI algorithms to extract meaningful insights. Addressing this challenge requires the development of innovative solutions, such as natural language processing (NLP) algorithms and advanced data analytics techniques, to unlock the full potential of unstructured healthcare data for diagnostic purposes. By leveraging these tools, healthcare providers can extract valuable insights from unstructured data sources to increase diagnostic accuracy and improve patient care.
Data Privacy Concerns:
Data privacy concerns are a significant barrier to the adoption of artificial intelligence in medical diagnostics, especially given the sensitivity of healthcare data. Both patients and healthcare providers are concerned about the security and privacy of personal health information when using AI-based technologies. Addressing these issues requires strong data security measures and transparent data governance policies to protect patient privacy while harnessing the power of AI to drive healthcare innovation. Healthcare organizations must prioritize data security and compliance requirements, implementing encryption, access controls and auditing mechanisms to protect sensitive information. Additionally, transparent communication with patients about how data will be used and shared will be critical to building trust and confidence in AI-driven diagnostic systems, ensuring privacy concerns do not hinder the adoption of these transformative technologies.
Summary
As artificial intelligence continues to rapidly rise in the future of the medical diagnostics market, stakeholders must navigate a landscape filled with challenges and opportunities. By addressing key market constraints, fostering collaboration, and embracing emerging trends, the healthcare industry can unleash the full potential of AI, revolutionize medical diagnostics, and usher in a new era of precision medicine.
Through concerted efforts and strategic investment, the vision of AI-powered healthcare can become a reality, transforming the way we diagnose, treat and manage disease. As we stand on the cusp of this technological revolution, the future of medical diagnostics has never been more promising.
The above is the detailed content of Artificial Intelligence in Medical Diagnostics Market to Reach $4 Billion by 2028. For more information, please follow other related articles on the PHP Chinese website!

Hot AI Tools

Undresser.AI Undress
AI-powered app for creating realistic nude photos

AI Clothes Remover
Online AI tool for removing clothes from photos.

Undress AI Tool
Undress images for free

Clothoff.io
AI clothes remover

AI Hentai Generator
Generate AI Hentai for free.

Hot Article

Hot Tools

Notepad++7.3.1
Easy-to-use and free code editor

SublimeText3 Chinese version
Chinese version, very easy to use

Zend Studio 13.0.1
Powerful PHP integrated development environment

Dreamweaver CS6
Visual web development tools

SublimeText3 Mac version
God-level code editing software (SublimeText3)

Hot Topics
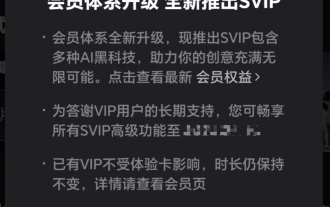
This site reported on June 27 that Jianying is a video editing software developed by FaceMeng Technology, a subsidiary of ByteDance. It relies on the Douyin platform and basically produces short video content for users of the platform. It is compatible with iOS, Android, and Windows. , MacOS and other operating systems. Jianying officially announced the upgrade of its membership system and launched a new SVIP, which includes a variety of AI black technologies, such as intelligent translation, intelligent highlighting, intelligent packaging, digital human synthesis, etc. In terms of price, the monthly fee for clipping SVIP is 79 yuan, the annual fee is 599 yuan (note on this site: equivalent to 49.9 yuan per month), the continuous monthly subscription is 59 yuan per month, and the continuous annual subscription is 499 yuan per year (equivalent to 41.6 yuan per month) . In addition, the cut official also stated that in order to improve the user experience, those who have subscribed to the original VIP
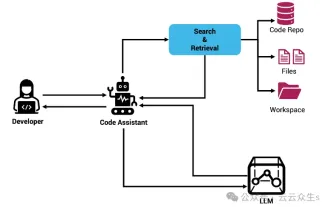
Improve developer productivity, efficiency, and accuracy by incorporating retrieval-enhanced generation and semantic memory into AI coding assistants. Translated from EnhancingAICodingAssistantswithContextUsingRAGandSEM-RAG, author JanakiramMSV. While basic AI programming assistants are naturally helpful, they often fail to provide the most relevant and correct code suggestions because they rely on a general understanding of the software language and the most common patterns of writing software. The code generated by these coding assistants is suitable for solving the problems they are responsible for solving, but often does not conform to the coding standards, conventions and styles of the individual teams. This often results in suggestions that need to be modified or refined in order for the code to be accepted into the application

To learn more about AIGC, please visit: 51CTOAI.x Community https://www.51cto.com/aigc/Translator|Jingyan Reviewer|Chonglou is different from the traditional question bank that can be seen everywhere on the Internet. These questions It requires thinking outside the box. Large Language Models (LLMs) are increasingly important in the fields of data science, generative artificial intelligence (GenAI), and artificial intelligence. These complex algorithms enhance human skills and drive efficiency and innovation in many industries, becoming the key for companies to remain competitive. LLM has a wide range of applications. It can be used in fields such as natural language processing, text generation, speech recognition and recommendation systems. By learning from large amounts of data, LLM is able to generate text
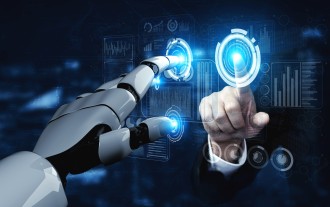
Large Language Models (LLMs) are trained on huge text databases, where they acquire large amounts of real-world knowledge. This knowledge is embedded into their parameters and can then be used when needed. The knowledge of these models is "reified" at the end of training. At the end of pre-training, the model actually stops learning. Align or fine-tune the model to learn how to leverage this knowledge and respond more naturally to user questions. But sometimes model knowledge is not enough, and although the model can access external content through RAG, it is considered beneficial to adapt the model to new domains through fine-tuning. This fine-tuning is performed using input from human annotators or other LLM creations, where the model encounters additional real-world knowledge and integrates it
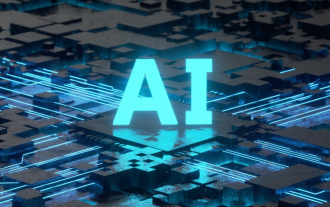
Editor |ScienceAI Question Answering (QA) data set plays a vital role in promoting natural language processing (NLP) research. High-quality QA data sets can not only be used to fine-tune models, but also effectively evaluate the capabilities of large language models (LLM), especially the ability to understand and reason about scientific knowledge. Although there are currently many scientific QA data sets covering medicine, chemistry, biology and other fields, these data sets still have some shortcomings. First, the data form is relatively simple, most of which are multiple-choice questions. They are easy to evaluate, but limit the model's answer selection range and cannot fully test the model's ability to answer scientific questions. In contrast, open-ended Q&A
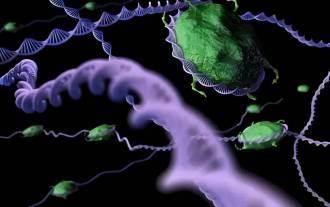
Editor | KX In the field of drug research and development, accurately and effectively predicting the binding affinity of proteins and ligands is crucial for drug screening and optimization. However, current studies do not take into account the important role of molecular surface information in protein-ligand interactions. Based on this, researchers from Xiamen University proposed a novel multi-modal feature extraction (MFE) framework, which for the first time combines information on protein surface, 3D structure and sequence, and uses a cross-attention mechanism to compare different modalities. feature alignment. Experimental results demonstrate that this method achieves state-of-the-art performance in predicting protein-ligand binding affinities. Furthermore, ablation studies demonstrate the effectiveness and necessity of protein surface information and multimodal feature alignment within this framework. Related research begins with "S
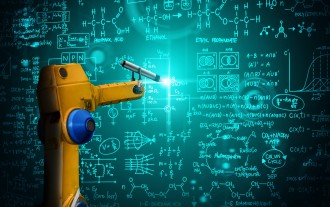
Machine learning is an important branch of artificial intelligence that gives computers the ability to learn from data and improve their capabilities without being explicitly programmed. Machine learning has a wide range of applications in various fields, from image recognition and natural language processing to recommendation systems and fraud detection, and it is changing the way we live. There are many different methods and theories in the field of machine learning, among which the five most influential methods are called the "Five Schools of Machine Learning". The five major schools are the symbolic school, the connectionist school, the evolutionary school, the Bayesian school and the analogy school. 1. Symbolism, also known as symbolism, emphasizes the use of symbols for logical reasoning and expression of knowledge. This school of thought believes that learning is a process of reverse deduction, through existing
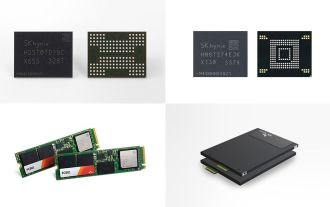
According to news from this site on August 1, SK Hynix released a blog post today (August 1), announcing that it will attend the Global Semiconductor Memory Summit FMS2024 to be held in Santa Clara, California, USA from August 6 to 8, showcasing many new technologies. generation product. Introduction to the Future Memory and Storage Summit (FutureMemoryandStorage), formerly the Flash Memory Summit (FlashMemorySummit) mainly for NAND suppliers, in the context of increasing attention to artificial intelligence technology, this year was renamed the Future Memory and Storage Summit (FutureMemoryandStorage) to invite DRAM and storage vendors and many more players. New product SK hynix launched last year
