


See through 3D representation and generative models of objects: NUS team proposes X-Ray
- Project homepage: https://tau-yihouxiang.github.io/projects/X-Ray/X-Ray.html
- Paper address: https://arxiv.org/abs/2404.14329
- Code address: https://github.com/tau-yihouxiang/ X-Ray
- Dataset: https://huggingface.co/datasets/yihouxiang/X-Ray
Currently, artificial intelligence is developing rapidly in the field of human intelligence. In computer vision, image and video generation technology has become increasingly mature, and models such as Midjourney and Stable Video Diffusion are widely used. However, generative models in the field of 3D vision still face challenges.
Current 3D model generation technology is usually based on multi-angle video generation and reconstruction, such as the SV3D model, by generating multi-angle videos and combining neural radiation fields (NeRF) or 3D Gaussian smooth models ( 3D Gaussian Splatting technology) to build 3D objects step by step. This method is mainly limited to the generation of simple, non-self-occlusion three-dimensional objects, and cannot present the internal structure of the object, making the entire generation process complex and imperfect, showing the complexity and limitations of this technology.
The reason is that there is currently a lack of flexible, efficient and easy to generalize 3D Representation (3D representation).
Figure 1. X-Ray serialized 3D representation
National University of Singapore (NUS) Hu Dr. Run led the research team to release a new 3D representation - X-ray, which can sequentially represent the surface shape and texture of objects seen from the perspective of the camera, and can make full use of the video generation function to generate Model advantages are used to generate 3D objects, and the internal and external 3D structures of the objects can be generated at the same time.
This article will demonstrate in detail the principles, advantages and broad application prospects of X-Ray technology.
Figure 2. Comparison with rendering-based 3D model generation methods.
Technological innovation: 3D representation method of the inner and outer surfaces of the object
X-Ray representation: H×W starting from the center of the camera toward the direction of the object A matrix point emits a ray. In each ray direction, L three-dimensional attribute data including depth, normal vector, color, etc. are recorded one by one at the intersection point with the object's surface, and then these data are organized into the form of L×H×W to realize the creation of any 3D model. Tensor representation, this is the X-Ray representation method proposed by the team.
It is worth noting that the representation is the same as the video format, so the video generation model can be used to make 3D generative models. The specific process is as follows.
Figure 3. X-Ray sample with different number of layers.
1. Encoding process: 3D model to X-Ray
Given a 3D model, usually three-dimensional Grid, first set up a camera to observe the model, and then use the Ray Casting Algorithm to record the properties of all surfaces where each camera ray intersects with the object , including the depth of the surface
, normal vector
, color
, etc., for convenience of instruction, use
to represent the Whether a surface exists at the location.
Then, by obtaining all intersecting surface points such as camera rays, a complete X-Ray 3D expression can be obtained, as shown in the following expression and Figure 3.
Through the encoding process, an arbitrary 3D model is converted into X-Ray. It is the same as the video format and has a different number of frames. Normally, the number of frames L=8 is enough to represent a 3D object.
2. Decoding process: X-Ray to 3D model
Given an X-Ray, you can also It is converted back to a 3D model through the decoding process, so that the 3D model can be generated only by generating X-Ray. The specific process includes two processes: point cloud generation process and point cloud surface reconstruction process.
- X-Ray to point cloud: In addition to the position coordinates of the 3D point, each point also has color and normal vector information.
where r_0 and r_d are the starting point and normalized direction of the camera ray respectively. By processing each camera ray, we get A complete point cloud can be obtained.
- Point cloud to three-dimensional mesh: The next step is to convert the point cloud into a three-dimensional mesh. This is A technology that has been studied for many years, because these point clouds have normal vectors, the Screened Poisson algorithm is used to directly convert the point cloud into a three-dimensional mesh model, which is the final 3D model.
3D model generation based on X-Ray representation
In order to generate high-resolution and diverse 3D X-Ray models, the The team used a video diffusion model architecture similar to the video format. This architecture can process continuous 3D information and improve the quality of X-Ray through upsampling modules to generate high-precision 3D output. The diffusion model is responsible for gradually generating detailed 3D images from noisy data, while the upsampling module enhances image resolution and detail to meet high quality standards. The specific structure is shown in Figure 4.
X-Ray Diffusion Generation Model
Diffusion model uses latent space in X-Ray generation and usually requires custom development of vector quantization - Variational autoencoder (VQ-VAE) [3] performs data compression, a process that lacks ready-made models and increases the training burden.
In order to effectively train high-resolution generators, the team adopted a cascade synthesis strategy to gradually train from low to high resolution through technologies such as Imagen and Stable Cascaded to adapt to the limited computing resources and improve X-Ray image quality.
Specifically, the 3D U-Net architecture in Stable Video Diffusion is used as the diffusion model to generate low-resolution Extract features from sequences to enhance processing and interpretation of X-Ray, which is critical for high-quality results.
X-Ray upsampling model
The diffusion model of the previous stage can only generate low-resolution Ray image. In subsequent stages, the focus is on upgrading these low-resolution X-Rays to higher resolutions.
The team explored two main approaches: point cloud upsampling and video upsampling.
Since a rough representation of shape and appearance has been obtained, encoding this data into a point cloud with color and normals is a straightforward process.
However, the point cloud representation structure is too loose and unsuitable for dense prediction. Traditional point cloud upsampling techniques usually simply increase the number of points, which is useful for improving things such as texture and color. The attribute may not be valid enough. To simplify the process and ensure consistency throughout the pipeline, we chose to use a video upsampling model.
This model is adapted from the spatiotemporal VAE decoder of Stable Video Diffusion (SVD), specially trained from scratch to upsample synthesized X-Ray frames by a factor of 4x while maintaining the original of layers. The decoder is able to perform attention operations independently at the frame level and hierarchical levels. This dual-layer attention mechanism not only improves the resolution, but also significantly improves the overall quality of the image. These features make the video upsampling model a more coordinated and efficient solution in high-resolution X-Ray generation.
Figure 4: 3D model generation framework based on X-Ray representation, including X-Ray diffusion model and X-Ray upsampling model.
Experiment
1. Data set:
Experimental use A filtered subset of the Objaverse dataset was created, from which entries with missing textures and insufficient hints were removed.
This subset contains over 60,000 3D objects. For each object, 4 camera views are randomly selected, covering azimuth angles from -180 to 180 degrees and elevation angles from -45 to 45 degrees, and the distance from the camera to the center of the object is fixed to 1.5.
Then use Blender software for rendering, and generate the corresponding X-Ray through the ray casting algorithm provided by the trimesh library. Through these processes, over 240,000 pairs of images and X-Ray datasets can be created to train generative models.
2. Implementation details:
The X-Ray diffusion model is based on the spatiotemporal UNet architecture used in Stable Video Diffusion (SVD), with slight adjustments: the model is configured to synthesize 8 channels: 1 hit channel, 1 depth channel and 6 Normal channel, compared to the 4 channels of the original network.
Given the significant differences between X-Ray imaging and traditional video, the model was trained from scratch to bridge the large gap between the X-Ray and video fields. Training took place over a week on 8 NVIDIA A100 GPU servers. During this period, the learning rate was kept at 0.0001, using the AdamW optimizer.
Since different X-Ray has different number of layers, pad or crop them to the same 8 layers for better batch processing and training, the frame size of each layer is 64×64. For the upsampling model, the output of the L layer is still 8, but the resolution of each frame is increased to 256×256, which enhances the detail and clarity of the enlarged X-Ray. The results are shown in Figures 5 and 6.
Figure 5: Image to X-Ray and to 3D model generation
Figure 6: Text to X-Ray and to 3D model generation
Future Outlook: New representation brings endless possibilities
With the continuous advancement of machine learning and image processing technology, the application prospects of X-Ray are infinitely broad.
In the future, this technology may be combined with augmented reality (AR) and virtual reality (VR) technology to create a fully immersive 3D experience for users. Education and training fields can also benefit from this, such as providing more intuitive learning materials and simulation experiments through 3D reconstruction.
In addition, the application of X-Ray technology in the fields of medical imaging and biotechnology may change people's understanding and research methods of complex biological structures. Look forward to how it changes the way you interact with the three-dimensional world.
The above is the detailed content of See through 3D representation and generative models of objects: NUS team proposes X-Ray. For more information, please follow other related articles on the PHP Chinese website!

Hot AI Tools

Undresser.AI Undress
AI-powered app for creating realistic nude photos

AI Clothes Remover
Online AI tool for removing clothes from photos.

Undress AI Tool
Undress images for free

Clothoff.io
AI clothes remover

AI Hentai Generator
Generate AI Hentai for free.

Hot Article

Hot Tools

Notepad++7.3.1
Easy-to-use and free code editor

SublimeText3 Chinese version
Chinese version, very easy to use

Zend Studio 13.0.1
Powerful PHP integrated development environment

Dreamweaver CS6
Visual web development tools

SublimeText3 Mac version
God-level code editing software (SublimeText3)

Hot Topics
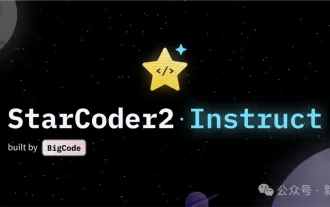
At the forefront of software technology, UIUC Zhang Lingming's group, together with researchers from the BigCode organization, recently announced the StarCoder2-15B-Instruct large code model. This innovative achievement achieved a significant breakthrough in code generation tasks, successfully surpassing CodeLlama-70B-Instruct and reaching the top of the code generation performance list. The unique feature of StarCoder2-15B-Instruct is its pure self-alignment strategy. The entire training process is open, transparent, and completely autonomous and controllable. The model generates thousands of instructions via StarCoder2-15B in response to fine-tuning the StarCoder-15B base model without relying on expensive manual annotation.
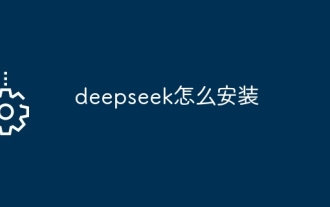
There are many ways to install DeepSeek, including: compile from source (for experienced developers) using precompiled packages (for Windows users) using Docker containers (for most convenient, no need to worry about compatibility) No matter which method you choose, Please read the official documents carefully and prepare them fully to avoid unnecessary trouble.
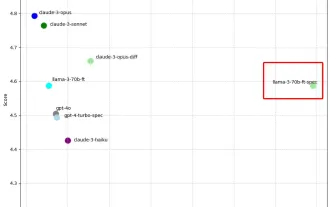
70B model, 1000 tokens can be generated in seconds, which translates into nearly 4000 characters! The researchers fine-tuned Llama3 and introduced an acceleration algorithm. Compared with the native version, the speed is 13 times faster! Not only is it fast, its performance on code rewriting tasks even surpasses GPT-4o. This achievement comes from anysphere, the team behind the popular AI programming artifact Cursor, and OpenAI also participated in the investment. You must know that on Groq, a well-known fast inference acceleration framework, the inference speed of 70BLlama3 is only more than 300 tokens per second. With the speed of Cursor, it can be said that it achieves near-instant complete code file editing. Some people call it a good guy, if you put Curs
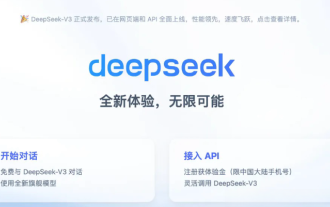
DeepSeekAI Tool User Guide and FAQ DeepSeek is a powerful AI intelligent tool. This article will answer some common usage questions to help you get started quickly. FAQ: The difference between different access methods: There is no difference in function between web version, App version and API calls, and App is just a wrapper for web version. The local deployment uses a distillation model, which is slightly inferior to the full version of DeepSeek-R1, but the 32-bit model theoretically has 90% full version capability. What is a tavern? SillyTavern is a front-end interface that requires calling the AI model through API or Ollama. What is breaking limit
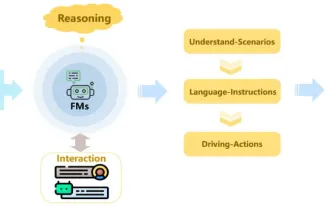
Written above & the author’s personal understanding: Recently, with the development and breakthroughs of deep learning technology, large-scale foundation models (Foundation Models) have achieved significant results in the fields of natural language processing and computer vision. The application of basic models in autonomous driving also has great development prospects, which can improve the understanding and reasoning of scenarios. Through pre-training on rich language and visual data, the basic model can understand and interpret various elements in autonomous driving scenarios and perform reasoning, providing language and action commands for driving decision-making and planning. The base model can be data augmented with an understanding of the driving scenario to provide those rare feasible features in long-tail distributions that are unlikely to be encountered during routine driving and data collection.
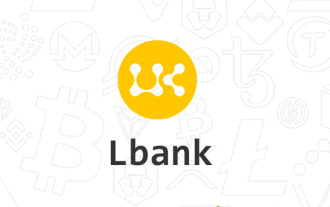
To register for LBank visit the official website and click "Register". Enter your email and password and verify your email. Download the LBank app iOS: Search "LBank" in the AppStore. Download and install the "LBank-DigitalAssetExchange" application. Android: Search for "LBank" in the Google Play Store. Download and install the "LBank-DigitalAssetExchange" application.
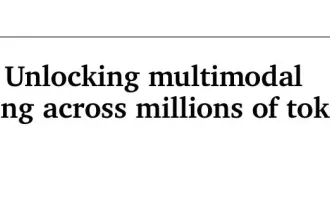
In February this year, Google launched the multi-modal large model Gemini 1.5, which greatly improved performance and speed through engineering and infrastructure optimization, MoE architecture and other strategies. With longer context, stronger reasoning capabilities, and better handling of cross-modal content. This Friday, Google DeepMind officially released the technical report of Gemini 1.5, which covers the Flash version and other recent upgrades. The document is 153 pages long. Technical report link: https://storage.googleapis.com/deepmind-media/gemini/gemini_v1_5_report.pdf In this report, Google introduces Gemini1
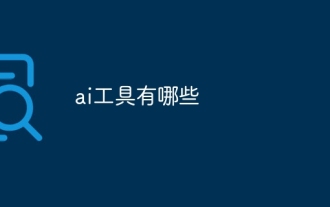
AI tools include: Doubao, ChatGPT, Gemini, BlenderBot, etc.
