


7262 papers were submitted, ICLR 2024 became a hit, and two domestic papers were nominated for outstanding papers.
A total of 5 outstanding paper awards and 11 honorable mentions were selected this year.
ICLR stands for International Conference on Learning Representations. This year is the 12th conference, held in Vienna, Austria, from May 7th to 11th.
In the machine learning community, ICLR is a relatively "young" top academic conference. It is hosted by deep learning giants and Turing Award winners Yoshua Bengio and Yann LeCun. It just held its first session in 2013. However, ICLR quickly gained wide recognition from academic researchers and is considered the top academic conference on deep learning.
This conference received a total of 7262 submitted papers and accepted 2260 papers. The overall acceptance rate was about 31%, which was the same as last year (31.8%). In addition, the proportion of Spotlights papers is 5% and the proportion of Oral papers is 1.2%.
Compared with previous years, whether it is the number of participants or the number of paper submissions, the popularity of ICLR can be said to have greatly improved. .
## Previous ICLR paper data chart
Among the award-winning papers announced recently, the conference selected 5 Outstanding Paper Awards and 11 Honorable Mention Awards.5 Outstanding Paper Awards
Outstanding Paper winners
Paper: Generalization in diffusion models arises from geometry -adaptive harmonic representations
- Paper address: https://openreview.net/pdf?id=ANvmVS2Yr0
- Institution: New York University, Collège de France
- Author: Zahra Kadkhodaie, Florentin Guth, Eero P. Simoncelli, Stéphane Mallat
Thesis: Learning Interactive Real-World Simulators
- Thesis address: https://openreview. net/forum?id=sFyTZEqmUY
- Institutions: UC Berkeley, Google DeepMind, MIT, University of Alberta
- Author: Sherry Yang, Yilun Du, Kamyar Ghasemipour, Jonathan Tompson, Leslie Kaelbling, Dale Schuurmans, Pieter Abbeel
As shown in Figure 3 below, UniSim can simulate a series of rich actions, such as washing hands, taking bowls, cutting carrots, and drying hands in a kitchen scene; the upper right of Figure 3 shows pressing different switches; the bottom of Figure 3 are two navigation scenarios.
## to correspond to the navigation scene in the lower right corner of Figure 3
## The navigation scenario below 3 right
Thesis: Never Train from Scratch: Fair Comparison of Long-sequence Models Requires Data-Driven Priors
- Paper address: https://openreview.net/forum?id=PdaPky8MUn
- Institution: Tel Aviv University, IBM
- Authors: Ido Amos, Jonathan Berant, Ankit Gupta
Paper: Protein Discovery with Discrete Walk-Jump Sampling
- Paper address: https:// openreview.net/forum?id=zMPHKOmQNb
- Institution: Genentech, New York University
- Author: Nathan C. Frey, Dan Berenberg, Karina Zadorozhny, Joseph Kleinhenz, Julien Lafrance-Vanasse, Isidro Hotzel, Yan Wu, Stephen Ra, Richard Bonneau, Kyunghyun Cho, Andreas Loukas, Vladimir Gligorijevic, Saeed Saremi
Paper: Vision Transformers Need Registers
- ##Paper address: https://openreview.net/ forum?id=2dnO3LLiJ1
- Institution: Meta et al
- Author: Timothée Darcet, Maxime Oquab, Julien Mairal, Piotr Bojanowski
- This paper identifies artifacts in the feature map of a vision transformer network, which are characterized by high-norm tokens in low-information background regions.
The authors propose key assumptions for why this phenomenon occurs and provide a simple yet elegant solution using additional register tokens to account for these traces, thereby enhancing the model's performance on a variety of tasks. Insights gained from this work could also impact other application areas.
This paper is well written and provides a good example of conducting research: "Identify the problem, understand why it occurs, and then propose a solution."
11 Honorable MentionsIn addition to 5 outstanding papers, ICLR 2024 also selected 11 honorable mentions.
Paper: Amortizing intractable inference in large language models
- Institution: University of Montreal, University of Oxford
- Authors: Edward J Hu, Moksh Jain, Eric Elmoznino, Younesse Kaddar, Guillaume Lajoie, Yoshua Bengio, Nikolay Malkin
- Paper address: https://openreview.net/forum? id=Ouj6p4ca60
- This paper proposes a promising alternative to autoregressive decoding in large language models from a Bayesian inference perspective, which may inspire subsequent research .
##Institution: DeepMind
- Authors: Ian Gemp, Luke Marris, Georgios Piliouras
- Paper address: https://openreview.net/forum?id=cc8h3I3V4E
- This is a very clearly written paper that contributes significantly to solving the important problem of developing efficient and scalable Nash solvers.
- ##Institution: Peking University, Beijing Zhiyuan Artificial Intelligence Research Institute
- Author: Zhang Bohang Gai Jingchu Du Yiheng Ye Qiwei Hedi Wang Liwei ## Paper address: https://openreview.net/forum?id=HSKaGOi7Ar
- The expressiveness of GNNs is an important topic, and current solutions still have significant limitations. The author proposes a new expressivity theory based on homomorphic counting.
- Author: Ricky T. Q. Chen, Yaron Lipman
- Paper address: https://openreview.net/forum?id=g7ohDlTITL
- This paper discusses the application of general geometry This is a challenging but important problem of generative modeling on manifolds, and a practical and efficient algorithm is proposed. The paper is excellently presented and fully experimentally validated on a wide range of tasks.
- Authors: Shashanka Venkataramanan, Mamshad Nayeem Rizve, Joao Carreira, Yuki M Asano, Yannis Avrithis
- Paper address: https: //openreview.net/forum?id=Yen1lGns2o
- This paper proposes a novel self-supervised image pre-training method by learning from continuous videos. This paper contributes both a new type of data and a method for learning from new data.
- Authors: Yichen Wu, Long-Kai Huang, Renzhen Wang, Deyu Meng, Wei Ying (Ying Wei)
- Paper address: https://openreview.net/forum?id=TpD2aG1h0D
- The author proposes a new meta-continuous learning variance reduction method. The method performs well and not only has practical impact but is also supported by regret analysis.
- Authors: Suyu Ge, Yunan Zhang, Liyuan Liu, Minjia Zhang, Jiawei Han, Jianfeng Gao
- Paper address: https:/ /openreview.net/forum?id=uNrFpDPMyo
- This article aims at the KV cache compression problem (this problem has a great impact on Transformer-based LLM) and uses a simple idea to reduce memory. And it can be deployed without extensive resource-intensive fine-tuning or retraining. This method is very simple and has proven to be very effective.
Authors: Yonatan Oren, Nicole Meister, Niladri S. Chatterji, Faisal Ladhak, Tatsunori Hashimoto
Paper address: https://openreview.net/forum?id= KS8mIvetg2
This paper uses a simple and elegant method for testing whether a supervised learning dataset has been included in the training of a large language model.
Author: Jonathan Richens, Tom Everitt
Paper address: https://openreview.net/forum?id=pOoKI3ouv1
This paper was laid down Considerable progress has been made in the theoretical foundations for understanding the role of causal reasoning in an agent's ability to generalize to new domains, with implications for a range of related fields.
Author: Gautam Reddy
Paper address: https://openreview.net/forum?id=aN4Jf6Cx69
This is a timely and extremely systematic study that explores the mechanisms between in-context learning and in-weight learning as we begin to understand these phenomena.
Authors: Germain Kolossov, Andrea Montanari, Pulkit Tandon
Paper address: https://openreview.net/forum?id=HhfcNgQn6p
This paper establishes a statistical foundation for data subset selection and identifies the shortcomings of popular data selection methods.
Paper: Beyond Weisfeiler-Lehman: A Quantitative Framework for GNN Expressiveness
- Institution: Meta
- Institution: University of Central Florida, Google DeepMind, University of Amsterdam, etc.
- Institutions: City University of Hong Kong, Tencent AI Laboratory, Xi'an Jiaotong University, etc.
##Institution: University of Illinois at Urbana-Champaign, Microsoft
Paper: Proving Test Set Contamination in Black-Box Language Models
##Institution: Stanford University, Columbia University
Institution: Google DeepMind
Institution: Princeton University, Harvard University, etc.
Institution: Granica Computing
Reference link: https://blog.iclr.cc/2024/05/06/iclr-2024-outstanding-paper-awards/
The above is the detailed content of 7262 papers were submitted, ICLR 2024 became a hit, and two domestic papers were nominated for outstanding papers.. For more information, please follow other related articles on the PHP Chinese website!

Hot AI Tools

Undresser.AI Undress
AI-powered app for creating realistic nude photos

AI Clothes Remover
Online AI tool for removing clothes from photos.

Undress AI Tool
Undress images for free

Clothoff.io
AI clothes remover

AI Hentai Generator
Generate AI Hentai for free.

Hot Article

Hot Tools

Notepad++7.3.1
Easy-to-use and free code editor

SublimeText3 Chinese version
Chinese version, very easy to use

Zend Studio 13.0.1
Powerful PHP integrated development environment

Dreamweaver CS6
Visual web development tools

SublimeText3 Mac version
God-level code editing software (SublimeText3)

Hot Topics
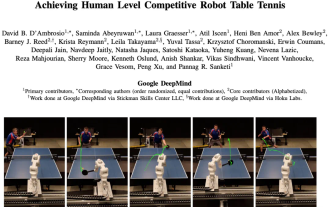
But maybe he can’t defeat the old man in the park? The Paris Olympic Games are in full swing, and table tennis has attracted much attention. At the same time, robots have also made new breakthroughs in playing table tennis. Just now, DeepMind proposed the first learning robot agent that can reach the level of human amateur players in competitive table tennis. Paper address: https://arxiv.org/pdf/2408.03906 How good is the DeepMind robot at playing table tennis? Probably on par with human amateur players: both forehand and backhand: the opponent uses a variety of playing styles, and the robot can also withstand: receiving serves with different spins: However, the intensity of the game does not seem to be as intense as the old man in the park. For robots, table tennis
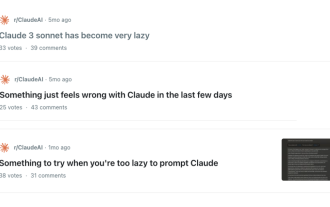
The start of school is about to begin, and it’s not just the students who are about to start the new semester who should take care of themselves, but also the large AI models. Some time ago, Reddit was filled with netizens complaining that Claude was getting lazy. "Its level has dropped a lot, it often pauses, and even the output becomes very short. In the first week of release, it could translate a full 4-page document at once, but now it can't even output half a page!" https:// www.reddit.com/r/ClaudeAI/comments/1by8rw8/something_just_feels_wrong_with_claude_in_the/ in a post titled "Totally disappointed with Claude", full of
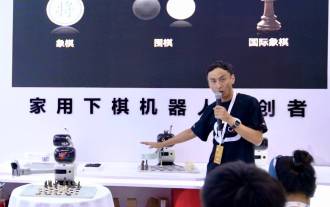
On August 21, the 2024 World Robot Conference was grandly held in Beijing. SenseTime's home robot brand "Yuanluobot SenseRobot" has unveiled its entire family of products, and recently released the Yuanluobot AI chess-playing robot - Chess Professional Edition (hereinafter referred to as "Yuanluobot SenseRobot"), becoming the world's first A chess robot for the home. As the third chess-playing robot product of Yuanluobo, the new Guoxiang robot has undergone a large number of special technical upgrades and innovations in AI and engineering machinery. For the first time, it has realized the ability to pick up three-dimensional chess pieces through mechanical claws on a home robot, and perform human-machine Functions such as chess playing, everyone playing chess, notation review, etc.
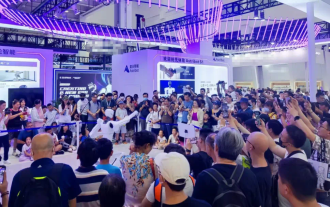
At the World Robot Conference being held in Beijing, the display of humanoid robots has become the absolute focus of the scene. At the Stardust Intelligent booth, the AI robot assistant S1 performed three major performances of dulcimer, martial arts, and calligraphy in one exhibition area, capable of both literary and martial arts. , attracted a large number of professional audiences and media. The elegant playing on the elastic strings allows the S1 to demonstrate fine operation and absolute control with speed, strength and precision. CCTV News conducted a special report on the imitation learning and intelligent control behind "Calligraphy". Company founder Lai Jie explained that behind the silky movements, the hardware side pursues the best force control and the most human-like body indicators (speed, load) etc.), but on the AI side, the real movement data of people is collected, allowing the robot to become stronger when it encounters a strong situation and learn to evolve quickly. And agile

Deep integration of vision and robot learning. When two robot hands work together smoothly to fold clothes, pour tea, and pack shoes, coupled with the 1X humanoid robot NEO that has been making headlines recently, you may have a feeling: we seem to be entering the age of robots. In fact, these silky movements are the product of advanced robotic technology + exquisite frame design + multi-modal large models. We know that useful robots often require complex and exquisite interactions with the environment, and the environment can be represented as constraints in the spatial and temporal domains. For example, if you want a robot to pour tea, the robot first needs to grasp the handle of the teapot and keep it upright without spilling the tea, then move it smoothly until the mouth of the pot is aligned with the mouth of the cup, and then tilt the teapot at a certain angle. . this
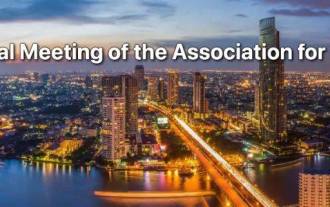
At this ACL conference, contributors have gained a lot. The six-day ACL2024 is being held in Bangkok, Thailand. ACL is the top international conference in the field of computational linguistics and natural language processing. It is organized by the International Association for Computational Linguistics and is held annually. ACL has always ranked first in academic influence in the field of NLP, and it is also a CCF-A recommended conference. This year's ACL conference is the 62nd and has received more than 400 cutting-edge works in the field of NLP. Yesterday afternoon, the conference announced the best paper and other awards. This time, there are 7 Best Paper Awards (two unpublished), 1 Best Theme Paper Award, and 35 Outstanding Paper Awards. The conference also awarded 3 Resource Paper Awards (ResourceAward) and Social Impact Award (
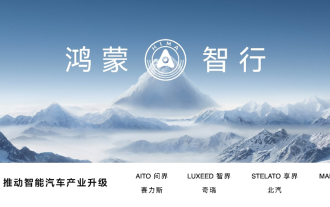
This afternoon, Hongmeng Zhixing officially welcomed new brands and new cars. On August 6, Huawei held the Hongmeng Smart Xingxing S9 and Huawei full-scenario new product launch conference, bringing the panoramic smart flagship sedan Xiangjie S9, the new M7Pro and Huawei novaFlip, MatePad Pro 12.2 inches, the new MatePad Air, Huawei Bisheng With many new all-scenario smart products including the laser printer X1 series, FreeBuds6i, WATCHFIT3 and smart screen S5Pro, from smart travel, smart office to smart wear, Huawei continues to build a full-scenario smart ecosystem to bring consumers a smart experience of the Internet of Everything. Hongmeng Zhixing: In-depth empowerment to promote the upgrading of the smart car industry Huawei joins hands with Chinese automotive industry partners to provide
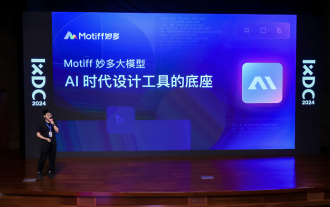
Artificial intelligence is developing faster than you might imagine. Since GPT-4 introduced multimodal technology into the public eye, multimodal large models have entered a stage of rapid development, gradually shifting from pure model research and development to exploration and application in vertical fields, and are deeply integrated with all walks of life. In the field of interface interaction, international technology giants such as Google and Apple have invested in the research and development of large multi-modal UI models, which is regarded as the only way forward for the mobile phone AI revolution. In this context, the first large-scale UI model in China was born. On August 17, at the IXDC2024 International Experience Design Conference, Motiff, a design tool in the AI era, launched its independently developed UI multi-modal model - Motiff Model. This is the world's first UI design tool
