État du graphique Lang auto-écrit
Première réponse de l'IA :
Résultat : Merci de nous avoir contactés, . J'aimerais vous aider, mais je veux m'assurer de bien comprendre vos besoins. Pourriez-vous s'il vous plaît fournir un peu plus de détails sur ce que vous recherchez ? Plus vous partagez d’informations, mieux je peux vous aider ! Je suis impatient de vous aider de toutes les manières possibles. Y a-t-il un domaine particulier que vous aimeriez approfondir ? Votre contribution m’aidera à adapter mon assistance à vos besoins précis. Je m'engage pleinement à rendre cette expérience aussi fluide et agréable que possible pour vous.
Réponse de l'IA, après avoir écrit son propre graphique :
Sortie : Bonjour ! Quoi de neuf? Comment puis-je vous aider aujourd'hui ? Plus vous m’en direz, mieux je pourrai vous aider. Qu'est-ce qui préoccupe votre esprit? Je suis là pour vous aider à trouver une solution qui vous convient. Juste un avertissement, je suis un assistant IA qui apprend encore les ficelles du métier.
Assez incroyable, non ?
Cela semble presque humain. Au cours du week-end, j'ai regardé le film Free Guy avec le gars de Van Wilder, et j'ai réalisé que je pourrais probablement utiliser The GraphState dans @langchain/langgraph pour créer une IA capable d'effectuer des itérations sur elle-même et d'écrire son propre code.
Si vous ne l'avez pas encore réalisé, Claude Sonnet est très bon en codage à 0 plan, et encore meilleur en codage à plans multiples.
Utiliser une bibliothèque npm:sentiment :
Depuis le fichier README.md
Sentiment est un module Node.js qui utilise la liste de mots AFINN-165 et le classement des sentiments Emoji pour effectuer une analyse des sentiments sur des blocs arbitraires de texte d'entrée.
J'ai ajouté une commande simple à l'état de mon graphique qui exécute une analyse des sentiments sur la sortie et fait évoluer le code avec une nouvelle version pour essayer d'obtenir un score plus élevé :
// update state and continue evolution return new Command({ update: { ...state, code: newCode, version: state.version + 1, analysis, previousSentimentDelta: currentSentimentDelta, type: "continue", output }, goto: "evolve" // Loop back to evolve });
Nous ensemençons le langgraph avec un état de graphe initial avec lequel il peut fonctionner (code fondamental si vous voulez) :
const initialWorkerCode = ` import { StateGraph, END } from "npm:@langchain/langgraph"; const workflow = new StateGraph({ channels: { input: "string", output: "string?" } }); // Initial basic response node workflow.addNode("respond", (state) => ({ ...state, output: "I understand your request and will try to help. Let me know if you need any clarification." })); workflow.setEntryPoint("respond"); workflow.addEdge("respond", END); const graph = workflow.compile(); export { graph }; `;
Vous pouvez voir qu'il s'agit d'un nœud de réponse vraiment basique avec un bord attaché.
J'ai le code actuel configuré pour passer par 10 itérations, en essayant d'obtenir un sentiment de 10 ou plus :
if (import.meta.main) { runEvolvingSystem(10, 10); }
A chaque fois, il effectue une analyse :
Analysis: { metrics: { emotionalRange: 0.16483516483516483, vocabularyVariety: 0.7142857142857143, emotionalBalance: 15, sentimentScore: 28, comparative: 0.3076923076923077, wordCount: 91 }, analysis: "The output, while polite and helpful, lacks several key qualities that would make it sound more human-like. Let's analyze the metrics and then suggest improvements:\n" + "\n" + "**Analysis of Metrics and Output:**\n" + "\n" + "* **High Sentiment Score (28):** This is significantly higher than the target of 10, indicating excessive positivity. Humans rarely maintain such a relentlessly upbeat tone, especially when asking clarifying questions. It feels forced and insincere.\n" + "\n" + "* **Emotional Range (0.16):** This low score suggests a lack of emotional variation. The response is consistently positive, lacking nuances of expression. Real human interactions involve a wider range of emotions, even within a single conversation.\n" + "\n" + "* **Emotional Balance (15.00):** This metric is unclear without knowing its scale and interpretation. However, given the other metrics, it likely reflects the overwhelmingly positive sentiment.\n" + "\n" + "* **Vocabulary Variety (0.71):** This is relatively good, indicating a decent range of words. However, the phrasing is still somewhat formulaic.\n" + "\n" + "* **Comparative Score (0.3077):** This metric is also unclear without context.\n" + "\n" + "* **Word Count (91):** A bit lengthy for a simple clarifying request. Brevity is often more human-like in casual conversation.\n" + "\n" + "\n" + "**Ways to Make the Response More Human-like:**\n" + "\n" + `1. **Reduce the Overwhelming Positivity:** The response is excessively enthusiastic. A more natural approach would be to tone down the positive language. Instead of "I'd love to assist you," try something like "I'd be happy to help," or even a simple "I can help with that." Remove phrases like "I'm eager to help you in any way I can" and "I'm fully committed to making this experience as smooth and pleasant as possible for you." These are overly formal and lack genuine warmth.\n` + "\n" + '2. **Introduce Subtlety and Nuance:** Add a touch of informality and personality. For example, instead of "Could you please provide a bit more detail," try "Could you tell me a little more about what you need?" or "Can you give me some more information on that?"\n' + "\n" + "3. **Shorten the Response:** The length makes it feel robotic. Conciseness is key to human-like communication. Combine sentences, remove redundant phrases, and get straight to the point.\n" + "\n" + '4. **Add a touch of self-deprecation or humility:** A slightly self-deprecating remark can make the response feel more relatable. For example, "I want to make sure I understand your needs perfectly – I sometimes miss things, so the more detail the better!"\n' + "\n" + "5. **Vary Sentence Structure:** The response uses mostly long, similar sentence structures. Varying sentence length and structure will make it sound more natural.\n" + "\n" + "**Example of a More Human-like Response:**\n" + "\n" + `"Thanks for reaching out! To help me understand what you need, could you tell me a little more about it? The more detail you can give me, the better I can assist you. Let me know what you're looking for."\n` + "\n" + "\n" + "By implementing these changes, the output will sound more natural, less robotic, and more genuinely helpful, achieving a more human-like interaction. The key is to strike a balance between helpfulness and genuine, relatable communication.\n", rawSentiment: { score: 28, comparative: 0.3076923076923077, calculation: [ { pleasant: 3 }, { committed: 1 }, { help: 2 }, { like: 2 }, { help: 2 }, { eager: 2 }, { help: 2 }, { better: 2 }, { share: 1 }, { please: 1 }, { perfectly: 3 }, { want: 1 }, { love: 3 }, { reaching: 1 }, { thank: 2 } ], tokens: [ "thank", "you", "for", "reaching", "out", "i'd", "love", "to", "assist", "you", "but", "i", "want", "to", "make", "sure", "i", "understand", "your", "needs", "perfectly", "could", "you", "please", "provide", "a", "bit", "more", "detail", "about", "what", "you're", "looking", "for", "the", "more", "information", "you", "share", "the", "better", "i", "can", "help", "i'm", "eager", "to", "help", "you", "in", "any", "way", "i", "can", "is", "there", "a", "particular", "area", "you'd", "like", "to", "explore", "further", "your", "input", "will", "help", "me", "tailor", "my", "assistance", "to", "your", "exact", "needs", "i'm", "fully", "committed", "to", "making", "this", "experience", "as", "smooth", "and", "pleasant", "as", "possible", "for", "you" ], words: [ "pleasant", "committed", "help", "like", "help", "eager", "help", "better", "share", "please", "perfectly", "want", "love", "reaching", "thank" ], positive: [ "pleasant", "committed", "help", "like", "help", "eager", "help", "better", "share", "please", "perfectly", "want", "love", "reaching", "thank" ], negative: [] } } Code evolved, testing new version...
Il utilise cette classe d'analyse pour obtenir un score plus élevé sur le code.
Après 10 itérations, le score est assez élevé :
Final Results: Latest version: 10 Final sentiment score: 9 Evolution patterns used: ["basic","responsive","interactive"]
Ce qui est le plus intéressant, c'est le graphique qu'il crée :
import { StateGraph, END } from "npm:@langchain/langgraph"; const workflow = new StateGraph({ channels: { input: "string", output: "string?", sentiment: "number", context: "object" } }); const positiveWords = ["good", "nice", "helpful", "appreciate", "thanks", "pleased", "glad", "great", "happy", "excellent", "wonderful", "amazing", "fantastic"]; const negativeWords = ["issue", "problem", "difficult", "confused", "frustrated", "unhappy"]; workflow.addNode("analyzeInput", (state) => { const input = state.input.toLowerCase(); let sentiment = input.split(" ").reduce((score, word) => { if (positiveWords.includes(word)) score += 1; if (negativeWords.includes(word)) score -= 1; return score; }, 0); sentiment = Math.min(Math.max(sentiment, -5), 5); return { ...state, sentiment, context: { needsClarification: sentiment === 0, isPositive: sentiment > 0, isNegative: sentiment < 0, topic: detectTopic(input), userName: extractUserName(input) } }; }); function detectTopic(input) { if (input.includes("technical") || input.includes("error")) return "technical"; if (input.includes("product") || input.includes("service")) return "product"; if (input.includes("billing") || input.includes("payment")) return "billing"; return "general"; } function extractUserName(input) { const nameMatch = input.match(/(?:my name is|i'm|i am) (\w+)/i); return nameMatch ? nameMatch[1] : ""; } workflow.addNode("generateResponse", (state) => { let response = ""; const userName = state.context.userName ? `${state.context.userName}` : "there"; if (state.context.isPositive) { response = `Hey ${userName}! Glad to hear things are going well. What can I do to make your day even better?`; } else if (state.context.isNegative) { response = `Hi ${userName}. I hear you're facing some challenges. Let's see if we can turn things around. What's on your mind?`; } else { response = `Hi ${userName}! What's up? How can I help you today?`; } return { ...state, output: response }; }); workflow.addNode("interactiveFollowUp", (state) => { let followUp = ""; switch (state.context.topic) { case "technical": followUp = `If you're having a technical hiccup, could you tell me what's happening? Any error messages or weird behavior?`; break; case "product": followUp = `Curious about our products? What features are you most interested in?`; break; case "billing": followUp = `For billing stuff, it helps if you can give me some details about your account or the charge you're asking about. Don't worry, I'll keep it confidential.`; break; default: followUp = `The more you can tell me, the better I can help. What's on your mind?`; } return { ...state, output: state.output + " " + followUp }; }); workflow.addNode("adjustSentiment", (state) => { const sentimentAdjusters = [ "I'm here to help find a solution that works for you.", "Thanks for your patience as we figure this out.", "Your input really helps me understand the situation better.", "Let's work together to find a great outcome for you." ]; const adjuster = sentimentAdjusters[Math.floor(Math.random() * sentimentAdjusters.length)]; return { ...state, output: state.output + " " + adjuster }; }); workflow.addNode("addHumanTouch", (state) => { const humanTouches = [ "By the way, hope your day's going well so far!", "Just a heads up, I'm an AI assistant still learning the ropes.", "Feel free to ask me to clarify if I say anything confusing.", "I appreciate your understanding as we work through this." ]; const touch = humanTouches[Math.floor(Math.random() * humanTouches.length)]; return { ...state, output: state.output + " " + touch }; }); workflow.setEntryPoint("analyzeInput"); workflow.addEdge("analyzeInput", "generateResponse"); workflow.addEdge("generateResponse", "interactiveFollowUp"); workflow.addEdge("interactiveFollowUp", "adjustSentiment"); workflow.addEdge("adjustSentiment", "addHumanTouch"); workflow.addEdge("addHumanTouch", END); const graph = workflow.compile(); export { graph };
J'ai vu ce code qu'il a écrit et j'ai immédiatement pensé aux pièges de :
Complexité émergente :
Cela fait référence à la complexité qui résulte de l'interaction de composants simples, qui sont dans ce cas les algorithmes du LLM et le vaste ensemble de données sur lequel il a été formé. Le LLM peut générer du code qui, bien que fonctionnel, présente des modèles et des dépendances complexes difficiles à comprendre pleinement pour les humains.
Donc, si nous pouvons revenir un peu en arrière et lui faire écrire un code plus propre, plus simple, nous pourrions être sur la bonne voie.
Quoi qu'il en soit, ce n'était qu'une expérience, car je voulais utiliser la nouvelle fonctionnalité de commande de Langgraphs.
S'il vous plaît, dites-moi ce que vous pensez dans les commentaires.
Ce qui précède est le contenu détaillé de. pour plus d'informations, suivez d'autres articles connexes sur le site Web de PHP en chinois!

Outils d'IA chauds

Undresser.AI Undress
Application basée sur l'IA pour créer des photos de nu réalistes

AI Clothes Remover
Outil d'IA en ligne pour supprimer les vêtements des photos.

Undress AI Tool
Images de déshabillage gratuites

Clothoff.io
Dissolvant de vêtements AI

Video Face Swap
Échangez les visages dans n'importe quelle vidéo sans effort grâce à notre outil d'échange de visage AI entièrement gratuit !

Article chaud

Outils chauds

Bloc-notes++7.3.1
Éditeur de code facile à utiliser et gratuit

SublimeText3 version chinoise
Version chinoise, très simple à utiliser

Envoyer Studio 13.0.1
Puissant environnement de développement intégré PHP

Dreamweaver CS6
Outils de développement Web visuel

SublimeText3 version Mac
Logiciel d'édition de code au niveau de Dieu (SublimeText3)

Sujets chauds










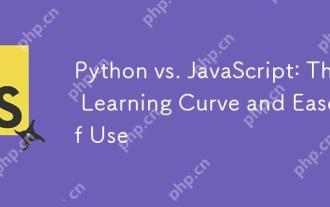
Python convient plus aux débutants, avec une courbe d'apprentissage en douceur et une syntaxe concise; JavaScript convient au développement frontal, avec une courbe d'apprentissage abrupte et une syntaxe flexible. 1. La syntaxe Python est intuitive et adaptée à la science des données et au développement back-end. 2. JavaScript est flexible et largement utilisé dans la programmation frontale et côté serveur.
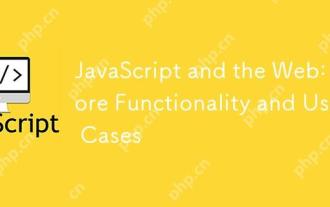
Les principales utilisations de JavaScript dans le développement Web incluent l'interaction client, la vérification du formulaire et la communication asynchrone. 1) Mise à jour du contenu dynamique et interaction utilisateur via les opérations DOM; 2) La vérification du client est effectuée avant que l'utilisateur ne soumette les données pour améliorer l'expérience utilisateur; 3) La communication de rafraîchissement avec le serveur est réalisée via la technologie AJAX.
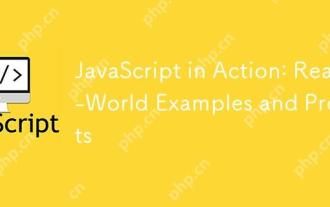
L'application de JavaScript dans le monde réel comprend un développement frontal et back-end. 1) Afficher les applications frontales en créant une application de liste TODO, impliquant les opérations DOM et le traitement des événements. 2) Construisez RestulAPI via Node.js et Express pour démontrer les applications back-end.
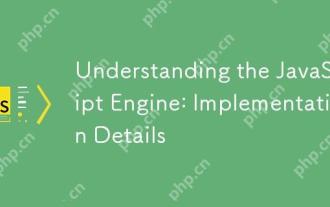
Comprendre le fonctionnement du moteur JavaScript en interne est important pour les développeurs car il aide à écrire du code plus efficace et à comprendre les goulots d'étranglement des performances et les stratégies d'optimisation. 1) Le flux de travail du moteur comprend trois étapes: analyse, compilation et exécution; 2) Pendant le processus d'exécution, le moteur effectuera une optimisation dynamique, comme le cache en ligne et les classes cachées; 3) Les meilleures pratiques comprennent l'évitement des variables globales, l'optimisation des boucles, l'utilisation de const et de locations et d'éviter une utilisation excessive des fermetures.
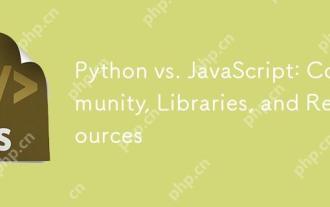
Python et JavaScript ont leurs propres avantages et inconvénients en termes de communauté, de bibliothèques et de ressources. 1) La communauté Python est amicale et adaptée aux débutants, mais les ressources de développement frontal ne sont pas aussi riches que JavaScript. 2) Python est puissant dans les bibliothèques de science des données et d'apprentissage automatique, tandis que JavaScript est meilleur dans les bibliothèques et les cadres de développement frontaux. 3) Les deux ont des ressources d'apprentissage riches, mais Python convient pour commencer par des documents officiels, tandis que JavaScript est meilleur avec MDNWEBDOCS. Le choix doit être basé sur les besoins du projet et les intérêts personnels.
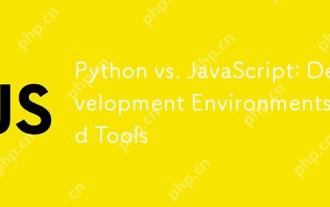
Les choix de Python et JavaScript dans les environnements de développement sont importants. 1) L'environnement de développement de Python comprend Pycharm, Jupyternotebook et Anaconda, qui conviennent à la science des données et au prototypage rapide. 2) L'environnement de développement de JavaScript comprend Node.js, VScode et WebPack, qui conviennent au développement frontal et back-end. Le choix des bons outils en fonction des besoins du projet peut améliorer l'efficacité du développement et le taux de réussite du projet.
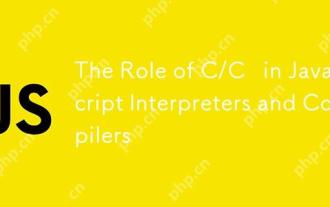
C et C jouent un rôle essentiel dans le moteur JavaScript, principalement utilisé pour implémenter des interprètes et des compilateurs JIT. 1) C est utilisé pour analyser le code source JavaScript et générer une arborescence de syntaxe abstraite. 2) C est responsable de la génération et de l'exécution de bytecode. 3) C met en œuvre le compilateur JIT, optimise et compile le code de point chaud à l'exécution et améliore considérablement l'efficacité d'exécution de JavaScript.
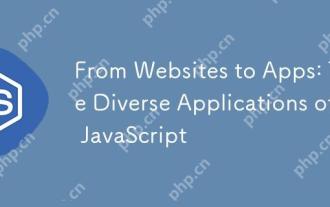
JavaScript est largement utilisé dans les sites Web, les applications mobiles, les applications de bureau et la programmation côté serveur. 1) Dans le développement de sites Web, JavaScript exploite DOM avec HTML et CSS pour réaliser des effets dynamiques et prend en charge des cadres tels que JQuery et React. 2) Grâce à la réactnative et ionique, JavaScript est utilisé pour développer des applications mobiles multiplateformes. 3) Le cadre électronique permet à JavaScript de créer des applications de bureau. 4) Node.js permet à JavaScript d'exécuter le côté du serveur et prend en charge les demandes simultanées élevées.
