


Créer un compagnon d'étude personnalisé à l'aide d'Amazon Bedrock
Je suis actuellement dans mon programme de maîtrise et j'ai toujours voulu trouver des moyens de réduire mes heures d'apprentissage chaque jour. Voilà ! Voici ma solution : créer un compagnon d'étude à l'aide d'Amazon Bedrock.
Nous exploiterons Amazon Bedrock pour exploiter la puissance des modèles de base (FM) tels que GPT-4 ou T5.
Ces modèles nous aideront à créer une IA générative capable de répondre aux requêtes des utilisateurs sur divers sujets de mon programme de maîtrise tels que la physique quantique, l'apprentissage automatique et plus encore. Nous explorerons comment affiner le modèle, mettre en œuvre une ingénierie avancée des invites et tirer parti de la génération augmentée par récupération (RAG) pour fournir des réponses précises aux étudiants.
Allons-y !
Étape 1 : Configuration de votre environnement sur AWS
Pour commencer, assurez-vous que votre compte AWS est configuré avec les autorisations nécessaires pour accéder à Amazon Bedrock, S3 et Lambda (j'ai appris cela à mes dépens après avoir découvert que je devais insérer ma carte de débit :( ) . Vous travaillerez avec des services AWS comme Amazon S3, Lambda et Bedrock.
.- Créez un compartiment S3 pour stocker votre matériel d'étude
- Cela permettra au modèle d'accéder aux matériaux pour le réglage et la récupération.
- Accédez à la console Amazon S3 et créez un nouveau compartiment, par exemple « study-materials ».
Télécharger du contenu éducatif sur S3. Dans mon cas, j'ai créé des données synthétiques à ajouter qui sont pertinentes pour mon programme de maîtrise. Vous pouvez créer le vôtre en fonction de vos besoins ou ajouter d'autres ensembles de données de Kaggle.
[ { "topic": "Advanced Economics", "question": "How does the Lucas Critique challenge traditional macroeconomic policy analysis?", "answer": "The Lucas Critique argues that traditional macroeconomic models' parameters are not policy-invariant because economic agents adjust their behavior based on expected policy changes, making historical relationships unreliable for policy evaluation." }, { "topic": "Quantum Physics", "question": "Explain quantum entanglement and its implications for quantum computing.", "answer": "Quantum entanglement is a physical phenomenon where pairs of particles remain fundamentally connected regardless of distance. This property enables quantum computers to perform certain calculations exponentially faster than classical computers through quantum parallelism and superdense coding." }, { "topic": "Advanced Statistics", "question": "What is the difference between frequentist and Bayesian approaches to statistical inference?", "answer": "Frequentist inference treats parameters as fixed and data as random, using probability to describe long-run frequency of events. Bayesian inference treats parameters as random variables with prior distributions, updated through data to form posterior distributions, allowing direct probability statements about parameters." }, { "topic": "Machine Learning", "question": "How do transformers solve the long-range dependency problem in sequence modeling?", "answer": "Transformers use self-attention mechanisms to directly model relationships between all positions in a sequence, eliminating the need for recurrent connections. This allows parallel processing and better capture of long-range dependencies through multi-head attention and positional encodings." }, { "topic": "Molecular Biology", "question": "What are the implications of epigenetic inheritance for evolutionary theory?", "answer": "Epigenetic inheritance challenges the traditional neo-Darwinian model by demonstrating that heritable changes in gene expression can occur without DNA sequence alterations, suggesting a Lamarckian component to evolution through environmentally-induced modifications." }, { "topic": "Advanced Computer Architecture", "question": "How do non-volatile memory architectures impact traditional memory hierarchy design?", "answer": "Non-volatile memory architectures blur the traditional distinction between storage and memory, enabling persistent memory systems that combine storage durability with memory-like performance, requiring fundamental redesign of memory hierarchies and system software." } ]
Étape 2 : exploiter Amazon Bedrock pour les modèles de base
Lancez Amazon Bedrock puis :
- Accédez à la console Amazon Bedrock.
- Créez un nouveau projet et sélectionnez le modèle de fondation souhaité (par exemple, GPT-3, T5).
- Choisissez votre cas d'utilisation, dans ce cas, un compagnon d'étude.
- Sélectionnez l'option de réglage fin (si nécessaire) et téléchargez l'ensemble de données (votre contenu éducatif depuis S3) pour un réglage fin.
- Affiner le modèle de fondation :
Bedrock affinera automatiquement le modèle de fondation sur votre ensemble de données. Par exemple, si vous utilisez GPT-3, Amazon Bedrock l'adaptera pour mieux comprendre le contenu éducatif et générer des réponses précises sur des sujets spécifiques.
Voici un extrait de code Python rapide utilisant le SDK Amazon Bedrock pour affiner le modèle :
import boto3 # Initialize Bedrock client client = boto3.client("bedrock-runtime") # Define S3 path for your dataset dataset_path = 's3://study-materials/my-educational-dataset.json' # Fine-tune the model response = client.start_training( modelName="GPT-3", datasetLocation=dataset_path, trainingParameters={"batch_size": 16, "epochs": 5} ) print(response)
Enregistrer le modèle affiné : Après le réglage fin, le modèle est enregistré et prêt à être déployé. Vous pouvez le trouver dans votre compartiment Amazon S3 sous un nouveau dossier appelé fine-tuned-model.
Étape 3 : implémenter la génération augmentée par récupération (RAG)
1. Configurer une fonction Amazon Lambda :
- Lambda traitera la demande et interagira avec le modèle affiné pour générer des réponses.
- La fonction Lambda récupérera les documents d'étude pertinents de S3 en fonction de la requête de l'utilisateur et utilisera RAG pour générer une réponse précise.
Code Lambda pour la génération de réponses : Voici un exemple de la façon dont vous pouvez configurer une fonction Lambda pour utiliser le modèle affiné pour générer des réponses :
[ { "topic": "Advanced Economics", "question": "How does the Lucas Critique challenge traditional macroeconomic policy analysis?", "answer": "The Lucas Critique argues that traditional macroeconomic models' parameters are not policy-invariant because economic agents adjust their behavior based on expected policy changes, making historical relationships unreliable for policy evaluation." }, { "topic": "Quantum Physics", "question": "Explain quantum entanglement and its implications for quantum computing.", "answer": "Quantum entanglement is a physical phenomenon where pairs of particles remain fundamentally connected regardless of distance. This property enables quantum computers to perform certain calculations exponentially faster than classical computers through quantum parallelism and superdense coding." }, { "topic": "Advanced Statistics", "question": "What is the difference between frequentist and Bayesian approaches to statistical inference?", "answer": "Frequentist inference treats parameters as fixed and data as random, using probability to describe long-run frequency of events. Bayesian inference treats parameters as random variables with prior distributions, updated through data to form posterior distributions, allowing direct probability statements about parameters." }, { "topic": "Machine Learning", "question": "How do transformers solve the long-range dependency problem in sequence modeling?", "answer": "Transformers use self-attention mechanisms to directly model relationships between all positions in a sequence, eliminating the need for recurrent connections. This allows parallel processing and better capture of long-range dependencies through multi-head attention and positional encodings." }, { "topic": "Molecular Biology", "question": "What are the implications of epigenetic inheritance for evolutionary theory?", "answer": "Epigenetic inheritance challenges the traditional neo-Darwinian model by demonstrating that heritable changes in gene expression can occur without DNA sequence alterations, suggesting a Lamarckian component to evolution through environmentally-induced modifications." }, { "topic": "Advanced Computer Architecture", "question": "How do non-volatile memory architectures impact traditional memory hierarchy design?", "answer": "Non-volatile memory architectures blur the traditional distinction between storage and memory, enabling persistent memory systems that combine storage durability with memory-like performance, requiring fundamental redesign of memory hierarchies and system software." } ]
3. Déployez la fonction Lambda : Déployez cette fonction Lambda sur AWS. Il sera invoqué via API Gateway pour gérer les requêtes des utilisateurs en temps réel.
Étape 4 : exposer le modèle via API Gateway
Créer une passerelle API :
Accédez à la console API Gateway et créez une nouvelle API REST.
Configurez un point de terminaison POST pour appeler votre fonction Lambda qui gère la génération de réponses.
Déployer l'API :
Déployez l'API et rendez-la accessible au public en utilisant un domaine personnalisé ou une URL par défaut d'AWS.
Étape 5 : Créer une interface simplifiée
Enfin, créez une application Streamlit simple pour permettre aux utilisateurs d'interagir avec votre compagnon d'étude.
import boto3 # Initialize Bedrock client client = boto3.client("bedrock-runtime") # Define S3 path for your dataset dataset_path = 's3://study-materials/my-educational-dataset.json' # Fine-tune the model response = client.start_training( modelName="GPT-3", datasetLocation=dataset_path, trainingParameters={"batch_size": 16, "epochs": 5} ) print(response)
Vous pouvez héberger cette application Streamlit sur AWS EC2 ou Elastic Beanstalk.
Si tout fonctionne bien, félicitations. Vous venez de devenir votre compagnon d'étude. Si je devais évaluer ce projet, je pourrais ajouter quelques exemples supplémentaires pour mes données synthétiques (duh ??) ou obtenir un autre ensemble de données pédagogiques qui correspond parfaitement à mes objectifs.
Merci d'avoir lu ! Dites-moi ce que vous en pensez !
Ce qui précède est le contenu détaillé de. pour plus d'informations, suivez d'autres articles connexes sur le site Web de PHP en chinois!

Outils d'IA chauds

Undresser.AI Undress
Application basée sur l'IA pour créer des photos de nu réalistes

AI Clothes Remover
Outil d'IA en ligne pour supprimer les vêtements des photos.

Undress AI Tool
Images de déshabillage gratuites

Clothoff.io
Dissolvant de vêtements AI

Video Face Swap
Échangez les visages dans n'importe quelle vidéo sans effort grâce à notre outil d'échange de visage AI entièrement gratuit !

Article chaud

Outils chauds

Bloc-notes++7.3.1
Éditeur de code facile à utiliser et gratuit

SublimeText3 version chinoise
Version chinoise, très simple à utiliser

Envoyer Studio 13.0.1
Puissant environnement de développement intégré PHP

Dreamweaver CS6
Outils de développement Web visuel

SublimeText3 version Mac
Logiciel d'édition de code au niveau de Dieu (SublimeText3)

Sujets chauds










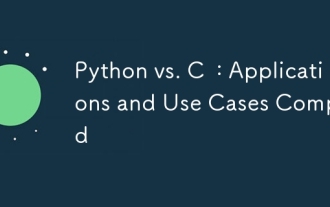
Python convient à la science des données, au développement Web et aux tâches d'automatisation, tandis que C convient à la programmation système, au développement de jeux et aux systèmes intégrés. Python est connu pour sa simplicité et son écosystème puissant, tandis que C est connu pour ses capacités de contrôle élevées et sous-jacentes.
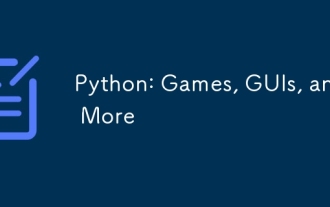
Python excelle dans les jeux et le développement de l'interface graphique. 1) Le développement de jeux utilise Pygame, fournissant des fonctions de dessin, audio et d'autres fonctions, qui conviennent à la création de jeux 2D. 2) Le développement de l'interface graphique peut choisir Tkinter ou Pyqt. Tkinter est simple et facile à utiliser, PYQT a des fonctions riches et convient au développement professionnel.
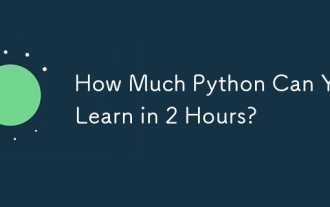
Vous pouvez apprendre les bases de Python dans les deux heures. 1. Apprenez les variables et les types de données, 2. Structures de contrôle maître telles que si les instructions et les boucles, 3. Comprenez la définition et l'utilisation des fonctions. Ceux-ci vous aideront à commencer à écrire des programmes Python simples.
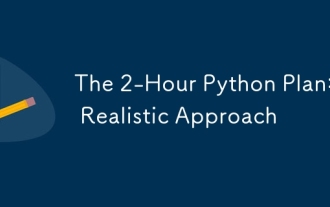
Vous pouvez apprendre les concepts de programmation de base et les compétences de Python dans les 2 heures. 1. Apprenez les variables et les types de données, 2. Flux de contrôle maître (instructions et boucles conditionnelles), 3. Comprenez la définition et l'utilisation des fonctions, 4. Démarrez rapidement avec la programmation Python via des exemples simples et des extraits de code.
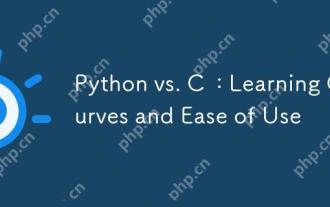
Python est plus facile à apprendre et à utiliser, tandis que C est plus puissant mais complexe. 1. La syntaxe Python est concise et adaptée aux débutants. Le typage dynamique et la gestion automatique de la mémoire le rendent facile à utiliser, mais peuvent entraîner des erreurs d'exécution. 2.C fournit des fonctionnalités de contrôle de bas niveau et avancées, adaptées aux applications haute performance, mais a un seuil d'apprentissage élevé et nécessite une gestion manuelle de la mémoire et de la sécurité.
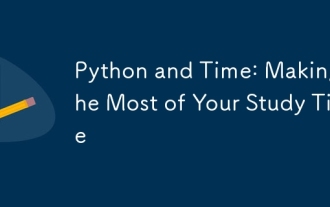
Pour maximiser l'efficacité de l'apprentissage de Python dans un temps limité, vous pouvez utiliser les modules DateTime, Time et Schedule de Python. 1. Le module DateTime est utilisé pour enregistrer et planifier le temps d'apprentissage. 2. Le module de temps aide à définir l'étude et le temps de repos. 3. Le module de planification organise automatiquement des tâches d'apprentissage hebdomadaires.
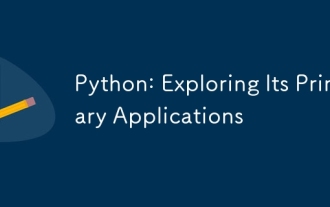
Python est largement utilisé dans les domaines du développement Web, de la science des données, de l'apprentissage automatique, de l'automatisation et des scripts. 1) Dans le développement Web, les cadres Django et Flask simplifient le processus de développement. 2) Dans les domaines de la science des données et de l'apprentissage automatique, les bibliothèques Numpy, Pandas, Scikit-Learn et Tensorflow fournissent un fort soutien. 3) En termes d'automatisation et de script, Python convient aux tâches telles que les tests automatisés et la gestion du système.
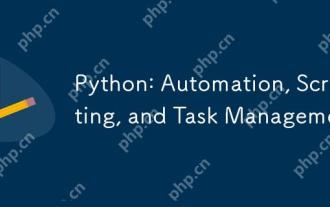
Python excelle dans l'automatisation, les scripts et la gestion des tâches. 1) Automatisation: La sauvegarde du fichier est réalisée via des bibliothèques standard telles que le système d'exploitation et la fermeture. 2) Écriture de script: utilisez la bibliothèque PSUTIL pour surveiller les ressources système. 3) Gestion des tâches: utilisez la bibliothèque de planification pour planifier les tâches. La facilité d'utilisation de Python et la prise en charge de la bibliothèque riche en font l'outil préféré dans ces domaines.
