Python で OpenSearch を使用した CRUD 操作をマスターする: 実践ガイド
OpenSearch は、Elasticsearch のオープンソース代替品であり、大規模なデータセット 簡単に。このブログでは、Python を使用して OpenSearch で基本的な CRUD (作成、読み取り、更新、削除) 操作を実行する方法をデモします。
前提条件:
- Python 3.7+
- Docker を使用してローカルにインストールされた OpenSearch
- RESTful API に関する知識
ステップ 1: Docker を使用して OpenSearch をローカルにセットアップする
始めるには、ローカル OpenSearch インスタンスが必要です。以下は、OpenSearch と OpenSearch Dashboards を起動する単純な docker-compose.yml ファイルです。
version: '3' services: opensearch-test-node-1: image: opensearchproject/opensearch:2.13.0 container_name: opensearch-test-node-1 environment: - cluster.name=opensearch-test-cluster - node.name=opensearch-test-node-1 - discovery.seed_hosts=opensearch-test-node-1,opensearch-test-node-2 - cluster.initial_cluster_manager_nodes=opensearch-test-node-1,opensearch-test-node-2 - bootstrap.memory_lock=true - "OPENSEARCH_JAVA_OPTS=-Xms512m -Xmx512m" - "DISABLE_INSTALL_DEMO_CONFIG=true" - "DISABLE_SECURITY_PLUGIN=true" ulimits: memlock: soft: -1 hard: -1 nofile: soft: 65536 hard: 65536 volumes: - opensearch-test-data1:/usr/share/opensearch/data ports: - 9200:9200 - 9600:9600 networks: - opensearch-test-net opensearch-test-node-2: image: opensearchproject/opensearch:2.13.0 container_name: opensearch-test-node-2 environment: - cluster.name=opensearch-test-cluster - node.name=opensearch-test-node-2 - discovery.seed_hosts=opensearch-test-node-1,opensearch-test-node-2 - cluster.initial_cluster_manager_nodes=opensearch-test-node-1,opensearch-test-node-2 - bootstrap.memory_lock=true - "OPENSEARCH_JAVA_OPTS=-Xms512m -Xmx512m" - "DISABLE_INSTALL_DEMO_CONFIG=true" - "DISABLE_SECURITY_PLUGIN=true" ulimits: memlock: soft: -1 hard: -1 nofile: soft: 65536 hard: 65536 volumes: - opensearch-test-data2:/usr/share/opensearch/data networks: - opensearch-test-net opensearch-test-dashboards: image: opensearchproject/opensearch-dashboards:2.13.0 container_name: opensearch-test-dashboards ports: - 5601:5601 expose: - "5601" environment: - 'OPENSEARCH_HOSTS=["http://opensearch-test-node-1:9200","http://opensearch-test-node-2:9200"]' - "DISABLE_SECURITY_DASHBOARDS_PLUGIN=true" networks: - opensearch-test-net volumes: opensearch-test-data1: opensearch-test-data2: networks: opensearch-test-net:
次のコマンドを実行して OpenSearch インスタンスを起動します:docker-compose up
OpenSearch には http://localhost:9200 からアクセスできます。
ステップ 2: Python 環境をセットアップする
python -m venv .venv source .venv/bin/activate pip install opensearch-py
また、プロジェクトを次のように構造化します:
├── interfaces.py ├── main.py ├── searchservice.py ├── docker-compose.yml
ステップ 3: インターフェイスとリソースの定義 (interfaces.py)
interfaces.py ファイルで、Resource クラスと Resources クラスを定義します。これらは、OpenSearch でさまざまなリソース タイプ (この場合はユーザー) を動的に処理するのに役立ちます。
from dataclasses import dataclass, field @dataclass class Resource: name: str def __post_init__(self) -> None: self.name = self.name.lower() @dataclass class Resources: users: Resource = field(default_factory=lambda: Resource("Users"))
ステップ 4: OpenSearch (searchservice.py) を使用した CRUD 操作
searchservice.py では、必要な操作の概要を示す抽象クラス SearchService を定義します。次に、HTTPOpenSearchService クラスはこれらの CRUD メソッドを実装し、OpenSearch クライアントと対話します。
# coding: utf-8 import abc import logging import typing as t from dataclasses import dataclass from uuid import UUID from interfaces import Resource, Resources from opensearchpy import NotFoundError, OpenSearch resources = Resources() class SearchService(abc.ABC): def search( self, kinds: t.List[Resource], tenants_id: UUID, companies_id: UUID, query: t.Dict[str, t.Any], ) -> t.Dict[t.Literal["hits"], t.Dict[str, t.Any]]: raise NotImplementedError def delete_index( self, kind: Resource, tenants_id: UUID, companies_id: UUID, data: t.Dict[str, t.Any], ) -> None: raise NotImplementedError def index( self, kind: Resource, tenants_id: UUID, companies_id: UUID, data: t.Dict[str, t.Any], ) -> t.Dict[str, t.Any]: raise NotImplementedError def delete_document( self, kind: Resource, tenants_id: UUID, companies_id: UUID, document_id: str, ) -> t.Optional[t.Dict[str, t.Any]]: raise NotImplementedError def create_index( self, kind: Resource, tenants_id: UUID, companies_id: UUID, data: t.Dict[str, t.Any], ) -> None: raise NotImplementedError @dataclass(frozen=True) class HTTPOpenSearchService(SearchService): client: OpenSearch def _gen_index( self, kind: Resource, tenants_id: UUID, companies_id: UUID, ) -> str: return ( f"tenant_{str(UUID(str(tenants_id)))}" f"_company_{str(UUID(str(companies_id)))}" f"_kind_{kind.name}" ) def index( self, kind: Resource, tenants_id: UUID, companies_id: UUID, data: t.Dict[str, t.Any], ) -> t.Dict[str, t.Any]: self.client.index( index=self._gen_index(kind, tenants_id, companies_id), body=data, id=data.get("id"), ) return data def delete_index( self, kind: Resource, tenants_id: UUID, companies_id: UUID, ) -> None: try: index = self._gen_index(kind, tenants_id, companies_id) if self.client.indices.exists(index): self.client.indices.delete(index) except NotFoundError: pass def create_index( self, kind: Resource, tenants_id: UUID, companies_id: UUID, ) -> None: body: t.Dict[str, t.Any] = {} self.client.indices.create( index=self._gen_index(kind, tenants_id, companies_id), body=body, ) def search( self, kinds: t.List[Resource], tenants_id: UUID, companies_id: UUID, query: t.Dict[str, t.Any], ) -> t.Dict[t.Literal["hits"], t.Dict[str, t.Any]]: return self.client.search( index=",".join( [self._gen_index(kind, tenants_id, companies_id) for kind in kinds] ), body={"query": query}, ) def delete_document( self, kind: Resource, tenants_id: UUID, companies_id: UUID, document_id: str, ) -> t.Optional[t.Dict[str, t.Any]]: try: response = self.client.delete( index=self._gen_index(kind, tenants_id, companies_id), id=document_id, ) return response except Exception as e: logging.error(f"Error deleting document: {e}") return None
ステップ 5: メイン (main.py) に CRUD を実装する
main.py では、次の方法を示します。
OpenSearch で
main.py- インデックス を作成します。
- サンプル ユーザー データを使用してドキュメントにインデックスを付けます。
クエリに基づいてドキュメントを- 検索します。
ID を使用してドキュメントを- 削除します。
# coding=utf-8 import logging import os import typing as t from uuid import uuid4 import searchservice from interfaces import Resources from opensearchpy import OpenSearch resources = Resources() logging.basicConfig(level=logging.INFO) search_service = searchservice.HTTPOpenSearchService( client=OpenSearch( hosts=[ { "host": os.getenv("OPENSEARCH_HOST", "localhost"), "port": os.getenv("OPENSEARCH_PORT", "9200"), } ], http_auth=( os.getenv("OPENSEARCH_USERNAME", ""), os.getenv("OPENSEARCH_PASSWORD", ""), ), use_ssl=False, verify_certs=False, ), ) tenants_id: str = "f0835e2d-bd68-406c-99a7-ad63a51e9ef9" companies_id: str = "bf58c749-c90a-41e2-b66f-6d98aae17a6c" search_str: str = "frank" document_id_to_delete: str = str(uuid4()) fake_data: t.List[t.Dict[str, t.Any]] = [ {"id": document_id_to_delete, "name": "Franklin", "tech": "python,node,golang"}, {"id": str(uuid4()), "name": "Jarvis", "tech": "AI"}, {"id": str(uuid4()), "name": "Parry", "tech": "Golang"}, {"id": str(uuid4()), "name": "Steve", "tech": "iOS"}, {"id": str(uuid4()), "name": "Frank", "tech": "node"}, ] search_service.delete_index( kind=resources.users, tenants_id=tenants_id, companies_id=companies_id ) search_service.create_index( kind=resources.users, tenants_id=tenants_id, companies_id=companies_id, ) for item in fake_data: search_service.index( kind=resources.users, tenants_id=tenants_id, companies_id=companies_id, data=dict(tenants_id=tenants_id, companies_id=companies_id, **item), ) search_query: t.Dict[str, t.Any] = { "bool": { "must": [], "must_not": [], "should": [], "filter": [ {"term": {"tenants_id.keyword": tenants_id}}, {"term": {"companies_id.keyword": companies_id}}, ], } } search_query["bool"]["must"].append( { "multi_match": { "query": search_str, "type": "phrase_prefix", "fields": ["name", "tech"], } } ) search_results = search_service.search( kinds=[resources.users], tenants_id=tenants_id, companies_id=companies_id, query=search_query, ) final_result = search_results.get("hits", {}).get("hits", []) for item in final_result: logging.info(["Item -> ", item.get("_source", {})]) deleted_result = search_service.delete_document( kind=resources.users, tenants_id=tenants_id, companies_id=companies_id, document_id=document_id_to_delete, ) logging.info(["Deleted result -> ", deleted_result])
ステップ 6: プロジェクトの実行
ドッカー構成Python main.py
結果:
見つかったレコードと削除されたレコードの情報を出力する必要があります。
ステップ 7: 結論
このブログでは、Docker を使用してローカルで OpenSearch をセットアップし、CRUD 操作を実行する方法をデモしました。 🎜>パイソン。 OpenSearch は、大規模なデータセットの管理とクエリを実行するための強力でスケーラブルなソリューションを提供します。このガイドは OpenSearch と ダミー データ の統合に焦点を当てていますが、実際のアプリケーションでは、OpenSearch は の高速化のための 読み取り最適化ストア としてよく使用されます。 データの取得。このような場合、プライマリ データベースと OpenSearch の両方を同時に更新することでデータの一貫性を確保するために、さまざまな インデックス付け戦略 を実装するのが一般的です。 これにより、OpenSearch
がプライマリ データ ソースと同期した状態を維持し、パフォーマンス と 精度最適化 します。 > データ取得中。 参考文献:
https://github.com/FranklinThaker/opensearch-integration-example
以上がPython で OpenSearch を使用した CRUD 操作をマスターする: 実践ガイドの詳細内容です。詳細については、PHP 中国語 Web サイトの他の関連記事を参照してください。

ホットAIツール

Undresser.AI Undress
リアルなヌード写真を作成する AI 搭載アプリ

AI Clothes Remover
写真から衣服を削除するオンライン AI ツール。

Undress AI Tool
脱衣画像を無料で

Clothoff.io
AI衣類リムーバー

Video Face Swap
完全無料の AI 顔交換ツールを使用して、あらゆるビデオの顔を簡単に交換できます。

人気の記事

ホットツール

メモ帳++7.3.1
使いやすく無料のコードエディター

SublimeText3 中国語版
中国語版、とても使いやすい

ゼンドスタジオ 13.0.1
強力な PHP 統合開発環境

ドリームウィーバー CS6
ビジュアル Web 開発ツール

SublimeText3 Mac版
神レベルのコード編集ソフト(SublimeText3)

ホットトピック










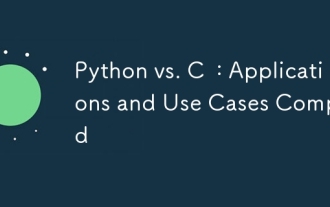
Pythonは、データサイエンス、Web開発、自動化タスクに適していますが、Cはシステムプログラミング、ゲーム開発、組み込みシステムに適しています。 Pythonは、そのシンプルさと強力なエコシステムで知られていますが、Cは高性能および基礎となる制御機能で知られています。
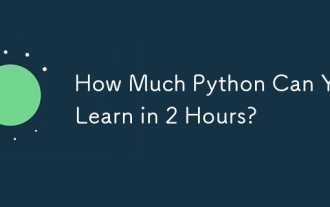
2時間以内にPythonの基本を学ぶことができます。 1。変数とデータ型を学習します。2。ステートメントやループの場合などのマスター制御構造、3。関数の定義と使用を理解します。これらは、簡単なPythonプログラムの作成を開始するのに役立ちます。
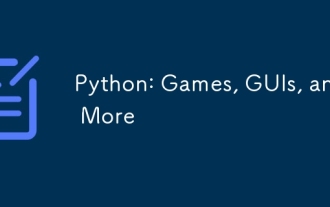
PythonはゲームとGUI開発に優れています。 1)ゲーム開発は、2Dゲームの作成に適した図面、オーディオ、その他の機能を提供し、Pygameを使用します。 2)GUI開発は、TKINTERまたはPYQTを選択できます。 TKINTERはシンプルで使いやすく、PYQTは豊富な機能を備えており、専門能力開発に適しています。
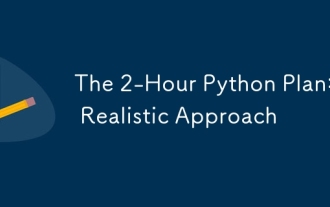
2時間以内にPythonの基本的なプログラミングの概念とスキルを学ぶことができます。 1.変数とデータ型、2。マスターコントロールフロー(条件付きステートメントとループ)、3。機能の定義と使用を理解する4。
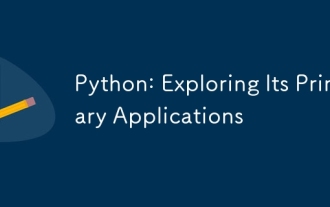
Pythonは、Web開発、データサイエンス、機械学習、自動化、スクリプトの分野で広く使用されています。 1)Web開発では、DjangoおよびFlask Frameworksが開発プロセスを簡素化します。 2)データサイエンスと機械学習の分野では、Numpy、Pandas、Scikit-Learn、Tensorflowライブラリが強力なサポートを提供します。 3)自動化とスクリプトの観点から、Pythonは自動テストやシステム管理などのタスクに適しています。
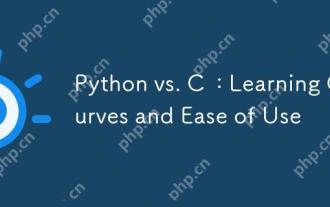
Pythonは学習と使用が簡単ですが、Cはより強力ですが複雑です。 1。Python構文は簡潔で初心者に適しています。動的なタイピングと自動メモリ管理により、使いやすくなりますが、ランタイムエラーを引き起こす可能性があります。 2.Cは、高性能アプリケーションに適した低レベルの制御と高度な機能を提供しますが、学習しきい値が高く、手動メモリとタイプの安全管理が必要です。
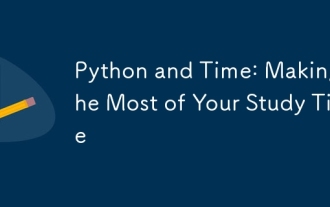
限られた時間でPythonの学習効率を最大化するには、PythonのDateTime、時間、およびスケジュールモジュールを使用できます。 1. DateTimeモジュールは、学習時間を記録および計画するために使用されます。 2。時間モジュールは、勉強と休息の時間を設定するのに役立ちます。 3.スケジュールモジュールは、毎週の学習タスクを自動的に配置します。
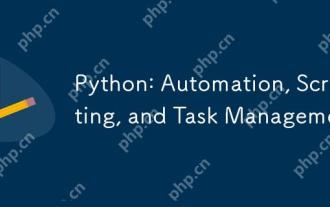
Pythonは、自動化、スクリプト、およびタスク管理に優れています。 1)自動化:OSやShutilなどの標準ライブラリを介してファイルバックアップが実現されます。 2)スクリプトの書き込み:Psutilライブラリを使用してシステムリソースを監視します。 3)タスク管理:スケジュールライブラリを使用してタスクをスケジュールします。 Pythonの使いやすさと豊富なライブラリサポートにより、これらの分野で優先ツールになります。
