条件付きチェーンを使用したインテリジェントな LLM アプリケーションの構築 - 詳細
TL;DR
- LLM アプリケーションで動的ルーティング戦略をマスターする
- 堅牢なエラー処理メカニズムを実装する
- 実用的な多言語コンテンツ処理システムを構築する
- 劣化戦略のベスト プラクティスを学ぶ
動的ルーティングについて
複雑な LLM アプリケーションでは、異なる入力に異なる処理パスが必要になることがよくあります。動的ルーティングは次のことに役立ちます:
- リソース使用率を最適化する
- 応答精度の向上
- システムの信頼性を向上させる
- 処理コストの管理
ルーティング戦略の設計
1. コアコンポーネント
from langchain.chains import LLMChain from langchain.prompts import ChatPromptTemplate from langchain.output_parsers import PydanticOutputParser from pydantic import BaseModel, Field from typing import Optional, List import asyncio class RouteDecision(BaseModel): route: str = Field(description="The selected processing route") confidence: float = Field(description="Confidence score of the decision") reasoning: str = Field(description="Explanation for the routing decision") class IntelligentRouter: def __init__(self, routes: List[str]): self.routes = routes self.parser = PydanticOutputParser(pydantic_object=RouteDecision) self.route_prompt = ChatPromptTemplate.from_template( """Analyze the following input and decide the best processing route. Available routes: {routes} Input: {input} {format_instructions} """ )
2. ルート選択ロジック
async def decide_route(self, input_text: str) -> RouteDecision: prompt = self.route_prompt.format( routes=self.routes, input=input_text, format_instructions=self.parser.get_format_instructions() ) chain = LLMChain( llm=self.llm, prompt=self.route_prompt ) result = await chain.arun(input=input_text) return self.parser.parse(result)
実践例: 多言語コンテンツシステム
1. システムアーキテクチャ
class MultiLangProcessor: def __init__(self): self.router = IntelligentRouter([ "translation", "summarization", "sentiment_analysis", "content_moderation" ]) self.processors = { "translation": TranslationChain(), "summarization": SummaryChain(), "sentiment_analysis": SentimentChain(), "content_moderation": ModerationChain() } async def process(self, content: str) -> Dict: try: route = await self.router.decide_route(content) if route.confidence < 0.8: return await self.handle_low_confidence(content, route) processor = self.processors[route.route] result = await processor.run(content) return { "status": "success", "route": route.route, "result": result } except Exception as e: return await self.handle_error(e, content)
2. エラー処理の実装
class ErrorHandler: def __init__(self): self.fallback_llm = ChatOpenAI( model_name="gpt-3.5-turbo", temperature=0.3 ) self.retry_limit = 3 self.backoff_factor = 1.5 async def handle_error( self, error: Exception, context: Dict ) -> Dict: error_type = type(error).__name__ if error_type in self.error_strategies: return await self.error_strategies[error_type]( error, context ) return await self.default_error_handler(error, context) async def retry_with_backoff( self, func, *args, **kwargs ): for attempt in range(self.retry_limit): try: return await func(*args, **kwargs) except Exception as e: if attempt == self.retry_limit - 1: raise e await asyncio.sleep( self.backoff_factor ** attempt )
劣化戦略の例
1. フォールバック チェーンのモデル化
class ModelFallbackChain: def __init__(self): self.models = [ ChatOpenAI(model_name="gpt-4"), ChatOpenAI(model_name="gpt-3.5-turbo"), ChatOpenAI(model_name="gpt-3.5-turbo-16k") ] async def run_with_fallback( self, prompt: str ) -> Optional[str]: for model in self.models: try: return await self.try_model(model, prompt) except Exception as e: continue return await self.final_fallback(prompt)
2. コンテンツチャンク戦略
class ChunkingStrategy: def __init__(self, chunk_size: int = 1000): self.chunk_size = chunk_size def chunk_content( self, content: str ) -> List[str]: # Implement smart content chunking return [ content[i:i + self.chunk_size] for i in range(0, len(content), self.chunk_size) ] async def process_chunks( self, chunks: List[str] ) -> List[Dict]: results = [] for chunk in chunks: try: result = await self.process_single_chunk(chunk) results.append(result) except Exception as e: results.append(self.handle_chunk_error(e, chunk)) return results
ベストプラクティスと推奨事項
-
ルート設計の原則
- ルートを重点的かつ具体的に保つ
- 明確なフォールバック パスを実装する
- ルートのパフォーマンス指標を監視する
-
エラー処理ガイドライン
- 段階的フォールバック戦略を実装する
- エラーを包括的にログに記録します
- 重大な障害に対するアラートを設定する
-
パフォーマンスの最適化
- 共通のルーティング決定をキャッシュします
- 可能な場合は同時処理を実装します
- ルーティングのしきい値を監視および調整します
結論
条件付きチェーンは、堅牢な LLM アプリケーションを構築するために重要です。重要なポイント:
- 明確なルーティング戦略を設計する
- 包括的なエラー処理を実装する
- 劣化シナリオを計画する
- パフォーマンスを監視して最適化する
以上が条件付きチェーンを使用したインテリジェントな LLM アプリケーションの構築 - 詳細の詳細内容です。詳細については、PHP 中国語 Web サイトの他の関連記事を参照してください。

ホットAIツール

Undresser.AI Undress
リアルなヌード写真を作成する AI 搭載アプリ

AI Clothes Remover
写真から衣服を削除するオンライン AI ツール。

Undress AI Tool
脱衣画像を無料で

Clothoff.io
AI衣類リムーバー

Video Face Swap
完全無料の AI 顔交換ツールを使用して、あらゆるビデオの顔を簡単に交換できます。

人気の記事

ホットツール

メモ帳++7.3.1
使いやすく無料のコードエディター

SublimeText3 中国語版
中国語版、とても使いやすい

ゼンドスタジオ 13.0.1
強力な PHP 統合開発環境

ドリームウィーバー CS6
ビジュアル Web 開発ツール

SublimeText3 Mac版
神レベルのコード編集ソフト(SublimeText3)

ホットトピック










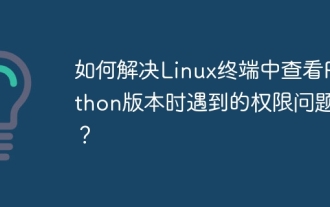
LinuxターミナルでPythonバージョンを表示する際の許可の問題の解決策PythonターミナルでPythonバージョンを表示しようとするとき、Pythonを入力してください...
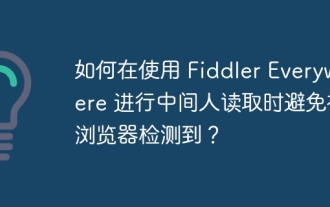
fiddlereveryversings for the-middleの測定値を使用するときに検出されないようにする方法
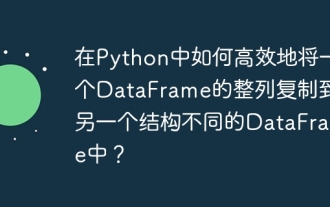
PythonのPandasライブラリを使用する場合、異なる構造を持つ2つのデータフレーム間で列全体をコピーする方法は一般的な問題です。 2つのデータがあるとします...
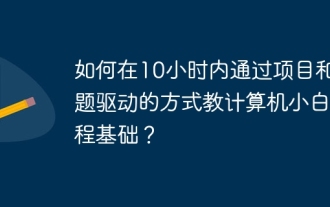
10時間以内にコンピューター初心者プログラミングの基本を教える方法は?コンピューター初心者にプログラミングの知識を教えるのに10時間しかない場合、何を教えることを選びますか...
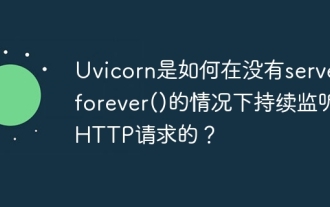
UvicornはどのようにしてHTTPリクエストを継続的に聞きますか? Uvicornは、ASGIに基づく軽量のWebサーバーです。そのコア機能の1つは、HTTPリクエストを聞いて続行することです...
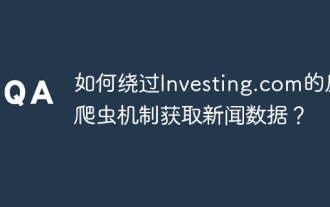
Investing.comの反クラウリング戦略を理解する多くの人々は、Investing.com(https://cn.investing.com/news/latest-news)からのニュースデータをクロールしようとします。
