MongoDB 設計におけるアルゴリズムの概念
1. スライディングウィンドウのコンセプト
MongoDB でのアプリケーション
// Sliding Window for Time-Series Data db.userActivity.aggregate([ // Sliding window for last 30 days of user engagement { $match: { timestamp: { $gte: new Date(Date.now() - 30 * 24 * 60 * 60 * 1000) } } }, { $group: { _id: { // Group by day day: { $dateToString: { format: "%Y-%m-%d", date: "$timestamp" }} }, dailyActiveUsers: { $addToSet: "$userId" }, totalEvents: { $sum: 1 } } }, // Sliding window aggregation to track trends { $setWindowFields: { sortBy: { "_id.day": 1 }, output: { movingAverageUsers: { $avg: "$dailyActiveUsers.length", window: { range: [-7, 0], unit: "day" } } } } } ])
主な利点
- ローリング指標を追跡します
- 時間ベースの傾向を分析する
- メモリの効率的な使用
2. ツーポインターテクニック
スキーマ設計例
// Optimized Social Graph Schema { _id: ObjectId("user1"), followers: [ { userId: ObjectId("user2"), followedAt: ISODate(), interaction: { // Two-pointer like tracking mutualFollows: Boolean, lastInteractionScore: Number } } ], following: [ { userId: ObjectId("user3"), followedAt: ISODate() } ] } // Efficient Friend Recommendation function findPotentialConnections(userId) { return db.users.aggregate([ { $match: { _id: userId } }, // Expand followers and following { $project: { potentialConnections: { $setIntersection: [ "$followers.userId", "$following.userId" ] } } } ]); }
最適化手法
- 計算の複雑さを軽減します
- 効率的な関係追跡
- 完全なコレクションのスキャンを最小限に抑える
3. ダイナミック プログラミング (DP) アプローチ
キャッシュとメモ化
// DP-Inspired Caching Strategy { _id: "user_analytics_cache", userId: ObjectId("user1"), // Memoized computation results cachedMetrics: { last30DaysEngagement: { computedAt: ISODate(), totalViews: 1000, avgSessionDuration: 5.5 }, yearlyTrends: { // Cached computation results computedAt: ISODate(), metrics: { /* pre-computed data */ } } }, // Invalidation timestamp lastUpdated: ISODate() } // DP-like Incremental Computation function updateUserAnalytics(userId) { // Check if cached result is valid const cachedResult = db.analyticsCache.findOne({ userId }); if (shouldRecompute(cachedResult)) { const newMetrics = computeComplexMetrics(userId); // Atomic update with incremental computation db.analyticsCache.updateOne( { userId }, { $set: { cachedMetrics: newMetrics, lastUpdated: new Date() } }, { upsert: true } ); } }
4. インデックス作成における貪欲なアプローチ
インデックス戦略
// Greedy Index Selection db.products.createIndex( { category: 1, price: -1, soldCount: -1 }, { // Greedy optimization partialFilterExpression: { inStock: true, price: { $gt: 100 } } } ) // Query Optimization Example function greedyQueryOptimization(filters) { // Dynamically select best index const indexes = db.products.getIndexes(); const bestIndex = indexes.reduce((best, current) => { // Greedy selection of most selective index const selectivityScore = computeIndexSelectivity(current, filters); return selectivityScore > best.selectivityScore ? { index: current, selectivityScore } : best; }, { selectivityScore: -1 }); return bestIndex.index; }
5. ヒープ/プライオリティキューの概念
分散型ランキングシステム
// Priority Queue-like Document Structure { _id: "global_leaderboard", topUsers: [ // Maintained like a min-heap { userId: ObjectId("user1"), score: 1000, lastUpdated: ISODate() }, // Continuously maintained top K users ], updateStrategy: { maxSize: 100, evictionPolicy: "lowest_score" } } // Efficient Leaderboard Management function updateLeaderboard(userId, newScore) { db.leaderboards.findOneAndUpdate( { _id: "global_leaderboard" }, { $push: { topUsers: { $each: [{ userId, score: newScore }], $sort: { score: -1 }, $slice: 100 // Maintain top 100 } } } ); }
6. グラフアルゴリズムのインスピレーション
ソーシャルネットワークスキーマ
// Graph-like User Connections { _id: ObjectId("user1"), connections: [ { userId: ObjectId("user2"), type: "friend", strength: 0.85, // Inspired by PageRank-like scoring connectionScore: { mutualFriends: 10, interactions: 25 } } ] } // Connection Recommendation function recommendConnections(userId) { return db.users.aggregate([ { $match: { _id: userId } }, // Graph traversal-like recommendation { $graphLookup: { from: "users", startWith: "$connections.userId", connectFromField: "connections.userId", connectToField: "_id", as: "potentialConnections", maxDepth: 2, restrictSearchWithMatch: { // Avoid already connected users _id: { $nin: existingConnections } } } } ]); }
スケーラビリティに関する考慮事項
重要な原則
-
アルゴリズムの効率
- 収集スキャンを最小限に抑える
- インデックス作成を戦略的に使用する
- 効率的な集約を実装する
-
分散コンピューティング
- シャーディングを活用する
- スマートパーティショニングを実装する
- 分散コンピューティングに集約パイプラインを使用する
-
キャッシュとメモ化
- 複雑な計算をキャッシュします
- 時間ベースの無効化を使用する
- 増分更新を実装する
主要なスキル
- データ アクセス パターンを理解する
- インデックス作成戦略を理解する
- クエリの複雑さを認識する
- 水平スケーリングについて考える
以上がMongoDB 設計におけるアルゴリズムの概念の詳細内容です。詳細については、PHP 中国語 Web サイトの他の関連記事を参照してください。

ホットAIツール

Undresser.AI Undress
リアルなヌード写真を作成する AI 搭載アプリ

AI Clothes Remover
写真から衣服を削除するオンライン AI ツール。

Undress AI Tool
脱衣画像を無料で

Clothoff.io
AI衣類リムーバー

Video Face Swap
完全無料の AI 顔交換ツールを使用して、あらゆるビデオの顔を簡単に交換できます。

人気の記事

ホットツール

メモ帳++7.3.1
使いやすく無料のコードエディター

SublimeText3 中国語版
中国語版、とても使いやすい

ゼンドスタジオ 13.0.1
強力な PHP 統合開発環境

ドリームウィーバー CS6
ビジュアル Web 開発ツール

SublimeText3 Mac版
神レベルのコード編集ソフト(SublimeText3)

ホットトピック










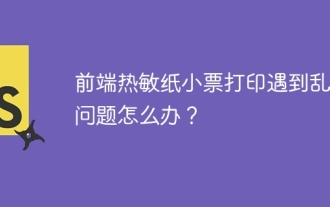
フロントエンドのサーマルペーパーチケット印刷のためのよくある質問とソリューションフロントエンド開発におけるチケット印刷は、一般的な要件です。しかし、多くの開発者が実装しています...
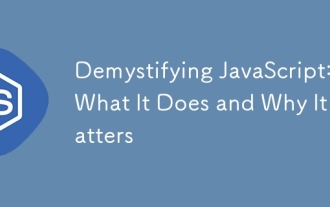
JavaScriptは現代のWeb開発の基礎であり、その主な機能には、イベント駆動型のプログラミング、動的コンテンツ生成、非同期プログラミングが含まれます。 1)イベント駆動型プログラミングにより、Webページはユーザー操作に応じて動的に変更できます。 2)動的コンテンツ生成により、条件に応じてページコンテンツを調整できます。 3)非同期プログラミングにより、ユーザーインターフェイスがブロックされないようにします。 JavaScriptは、Webインタラクション、シングルページアプリケーション、サーバー側の開発で広く使用されており、ユーザーエクスペリエンスとクロスプラットフォーム開発の柔軟性を大幅に改善しています。
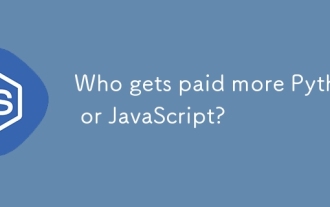
スキルや業界のニーズに応じて、PythonおよびJavaScript開発者には絶対的な給与はありません。 1. Pythonは、データサイエンスと機械学習でさらに支払われる場合があります。 2。JavaScriptは、フロントエンドとフルスタックの開発に大きな需要があり、その給与もかなりです。 3。影響要因には、経験、地理的位置、会社の規模、特定のスキルが含まれます。
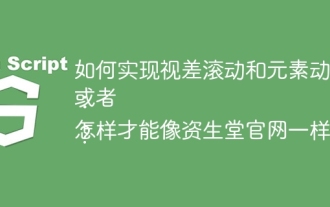
この記事の視差スクロールと要素のアニメーション効果の実現に関する議論では、Shiseidoの公式ウェブサイト(https://www.shisido.co.co.jp/sb/wonderland/)と同様の達成方法について説明します。
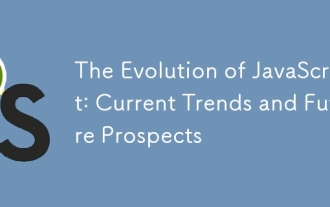
JavaScriptの最新トレンドには、TypeScriptの台頭、最新のフレームワークとライブラリの人気、WebAssemblyの適用が含まれます。将来の見通しは、より強力なタイプシステム、サーバー側のJavaScriptの開発、人工知能と機械学習の拡大、およびIoTおよびEDGEコンピューティングの可能性をカバーしています。
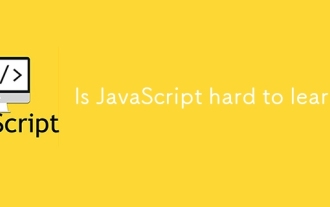
JavaScriptを学ぶことは難しくありませんが、挑戦的です。 1)変数、データ型、関数などの基本概念を理解します。2)非同期プログラミングをマスターし、イベントループを通じて実装します。 3)DOM操作を使用し、非同期リクエストを処理することを約束します。 4)一般的な間違いを避け、デバッグテクニックを使用します。 5)パフォーマンスを最適化し、ベストプラクティスに従ってください。
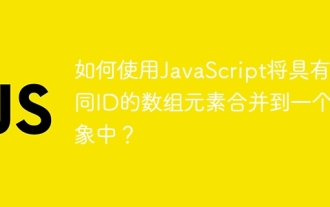
同じIDを持つ配列要素をJavaScriptの1つのオブジェクトにマージする方法は?データを処理するとき、私たちはしばしば同じIDを持つ必要性に遭遇します...
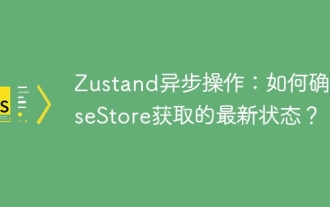
Zustand非同期操作のデータの更新問題。 Zustand State Management Libraryを使用する場合、非同期操作を不当にするデータ更新の問題に遭遇することがよくあります。 �...
