LeCun の好きなところ: Apple M1/M2 チップで LLaMA を実行! 130 億パラメータのモデルに必要なメモリはわずか 4GB
少し前、Meta がオープンソースの大規模言語モデル LLaMA をリリースした後、ネチズンはしきい値なしのダウンロード リンクを公開しましたが、それは「悲惨なことに」オープンでした。
このニュースが出るとすぐに、サークルはすぐに活気に満ち、皆がダウンロードしてテストし始めました。
しかし、トップレベルのグラフィックスカードを持っていない友人たちは、モデルを見てため息をつくことしかできません。
しかし、それは大きな問題ではありません。 Georgi Gerganov は最近、「llama.cpp」というプロジェクトを作成しました。LLaMA は GPU なしで実行できます。はい、これには Apple チップを搭載した Mac も含まれます。また、LeCun から転送サポートも受けました。
M1/M2 Mac で LLaMA を実行する 現在、それぞれ Apple の M1 プロセッサと M2 プロセッサに基づいた 2 つの比較的包括的なチュートリアルがあります。
早速、最初に効果を見てみましょう。
たとえば、最小の 7B モデルで、「最初に月に着陸したのは誰ですか?」と尋ねます。
とても早く、数秒で結果が出ました。
-p 『最初に月に足を踏み入れた人類は』
初めて月に足を踏み入れた人類は、38歳の宇宙飛行士ニール・A・アームストロングでした。 1969年7月20日、アポロ11号は月面に着陸しました。
アポロ 11 号は、月面に着陸した最初の有人ミッションであり、宇宙開発競争のクライマックスでした。 1969 年 7 月、ニール アームストロングとエドウィン "バズ" アルドリンは人類で初めて月面に着陸しました。 アポロ11号は7月16日にケネディ宇宙センターから打ち上げられました。
アームストロングの年齢、ミドルネーム、月面着陸日には明らかな事実誤認はありません。これほど小さなモデルではこれは簡単ではありません。
次のヒントを使用すると、実用的な Python コードを生成できます。
-p 'def open_and_return_content(filename):'
def open_and_return_content(filename): """Opens file (returning the content) and performs basic sanity checks"""if os.path.isfile(filename): with open(filename) as f: content = f.read() return contentelse: print('WARNING: file "{}" does not exist'.format(filename), file=sys.stderr) return ''def get_file_info(filename, fullpath): """Get file information (i.e., permission, owner, group, size)"""
接下来,我们就来看看具体是如何实现的。
最初に行うことは、LLaMA モデルをダウンロードすることです。
公式フォームを通じてメタに申請を送信することも、ネチズンが共有するリンクから直接申請を取得することもできます。
とにかく、完了すると、次のものの山が表示されます:
ご覧のとおり、異なるモデルが異なるフォルダーにあります。各モデルには、モデルに関する詳細を含む params.json があります。例:
ステップ 2: 依存関係をインストールする
首先,你需要安装Xcode来编译C++项目。
xcode-select --install
接下来,是构建C++项目的依赖项(pkgconfig和cmake)。
brew install pkgconfig cmake
在环境的配置上,假如你用的是Python 3.11,则可以创建一个虚拟环境:
/opt/homebrew/bin/python3.11 -m venv venv
然后激活venv。(如果是fish以外的shell,只要去掉.fish后缀即可)
. venv/bin/activate.fish
最后,安装Torch。
pip3 install --pre torch torchvision --extra-index-url https://download.pytorch.org/whl/nightly/cpu
如果你对利用新的Metal性能着色器(MPS)后端进行GPU训练加速感兴趣,可以通过运行以下程序来进行验证。但这不是在M1上运行LLaMA的必要条件。
python Python 3.11.2 (main, Feb 16 2023, 02:55:59) [Clang 14.0.0 (clang-1400.0.29.202)] on darwin Type "help", "copyright", "credits" or "license" for more information. >>> import torch; torch.backends.mps.is_available()True
第三步:编译LLaMA CPP
git clone git@github.com:ggerganov/llama.cpp.git
在安装完所有的依赖项后,你可以运行make:
make I llama.cpp build info: I UNAME_S:Darwin I UNAME_P:arm I UNAME_M:arm64 I CFLAGS: -I.-O3 -DNDEBUG -std=c11 -fPIC -pthread -DGGML_USE_ACCELERATE I CXXFLAGS: -I. -I./examples -O3 -DNDEBUG -std=c++11 -fPIC -pthread I LDFLAGS: -framework Accelerate I CC: Apple clang version 14.0.0 (clang-1400.0.29.202)I CXX:Apple clang version 14.0.0 (clang-1400.0.29.202) cc-I.-O3 -DNDEBUG -std=c11 -fPIC -pthread -DGGML_USE_ACCELERATE -c ggml.c -o ggml.o c++ -I. -I./examples -O3 -DNDEBUG -std=c++11 -fPIC -pthread -c utils.cpp -o utils.o c++ -I. -I./examples -O3 -DNDEBUG -std=c++11 -fPIC -pthread main.cpp ggml.o utils.o -o main-framework Accelerate ./main -h usage: ./main [options] options: -h, --helpshow this help message and exit -s SEED, --seed SEEDRNG seed (default: -1) -t N, --threads N number of threads to use during computation (default: 4) -p PROMPT, --prompt PROMPT prompt to start generation with (default: random) -n N, --n_predict N number of tokens to predict (default: 128) --top_k N top-k sampling (default: 40) --top_p N top-p sampling (default: 0.9) --temp Ntemperature (default: 0.8) -b N, --batch_size Nbatch size for prompt processing (default: 8) -m FNAME, --model FNAME model path (default: models/llama-7B/ggml-model.bin) c++ -I. -I./examples -O3 -DNDEBUG -std=c++11 -fPIC -pthread quantize.cpp ggml.o utils.o -o quantize-framework Accelerate
第四步:转换模型
假设你已经把模型放在llama.cpp repo中的models/下。
python convert-pth-to-ggml.py models/7B 1
那么,应该会看到像这样的输出:
{'dim': 4096, 'multiple_of': 256, 'n_heads': 32, 'n_layers': 32, 'norm_eps': 1e-06, 'vocab_size': 32000}n_parts =1Processing part0Processing variable: tok_embeddings.weight with shape:torch.Size([32000, 4096])and type:torch.float16 Processing variable: norm.weight with shape:torch.Size([4096])and type:torch.float16 Converting to float32 Processing variable: output.weight with shape:torch.Size([32000, 4096])and type:torch.float16 Processing variable: layers.0.attention.wq.weight with shape:torch.Size([4096, 4096])and type:torch.f loat16 Processing variable: layers.0.attention.wk.weight with shape:torch.Size([4096, 4096])and type:torch.f loat16 Processing variable: layers.0.attention.wv.weight with shape:torch.Size([4096, 4096])and type:torch.f loat16 Processing variable: layers.0.attention.wo.weight with shape:torch.Size([4096, 4096])and type:torch.f loat16 Processing variable: layers.0.feed_forward.w1.weight with shape:torch.Size([11008, 4096])and type:tor ch.float16 Processing variable: layers.0.feed_forward.w2.weight with shape:torch.Size([4096, 11008])and type:tor ch.float16 Processing variable: layers.0.feed_forward.w3.weight with shape:torch.Size([11008, 4096])and type:tor ch.float16 Processing variable: layers.0.attention_norm.weight with shape:torch.Size([4096])and type:torch.float 16... Done. Output file: models/7B/ggml-model-f16.bin, (part0 )
下一步将是进行量化处理:
./quantize ./models/7B/ggml-model-f16.bin ./models/7B/ggml-model-q4_0.bin 2
输出如下:
llama_model_quantize: loading model from './models/7B/ggml-model-f16.bin'llama_model_quantize: n_vocab = 32000llama_model_quantize: n_ctx = 512llama_model_quantize: n_embd= 4096llama_model_quantize: n_mult= 256llama_model_quantize: n_head= 32llama_model_quantize: n_layer = 32llama_model_quantize: f16 = 1... layers.31.attention_norm.weight - [ 4096, 1], type =f32 size =0.016 MB layers.31.ffn_norm.weight - [ 4096, 1], type =f32 size =0.016 MB llama_model_quantize: model size= 25705.02 MB llama_model_quantize: quant size=4017.27 MB llama_model_quantize: hist: 0.000 0.022 0.019 0.033 0.053 0.078 0.104 0.125 0.134 0.125 0.104 0.078 0.053 0.033 0.019 0.022 main: quantize time = 29389.45 ms main:total time = 29389.45 ms
第五步:运行模型
./main -m ./models/7B/ggml-model-q4_0.bin -t 8 -n 128 -p 'The first president of the USA was '
main: seed = 1678615879llama_model_load: loading model from './models/7B/ggml-model-q4_0.bin' - please wait ... llama_model_load: n_vocab = 32000llama_model_load: n_ctx = 512llama_model_load: n_embd= 4096llama_model_load: n_mult= 256llama_model_load: n_head= 32llama_model_load: n_layer = 32llama_model_load: n_rot = 128llama_model_load: f16 = 2llama_model_load: n_ff= 11008llama_model_load: n_parts = 1llama_model_load: ggml ctx size = 4529.34 MB llama_model_load: memory_size = 512.00 MB, n_mem = 16384llama_model_load: loading model part 1/1 from './models/7B/ggml-model-q4_0.bin'llama_model_load: .................................... donellama_model_load: model size =4017.27 MB / num tensors = 291 main: prompt: 'The first president of the USA was 'main: number of tokens in prompt = 9 1 -> ''1576 -> 'The' 937 -> ' first'6673 -> ' president' 310 -> ' of' 278 -> ' the'8278 -> ' USA' 471 -> ' was' 29871 -> ' ' sampling parameters: temp = 0.800000, top_k = 40, top_p = 0.950000 The first president of the USA was 57 years old when he assumed office (George Washington). Nowadays, the US electorate expects the new president to be more young at heart. President Donald Trump was 70 years old when he was inaugurated. In contrast to his predecessors, he is physically fit, healthy and active. And his fitness has been a prominent theme of his presidency. During the presidential campaign, he famously said he would be the “most active president ever” — a statement Trump has not yet achieved, but one that fits his approach to the office. His tweets demonstrate his physical activity. main: mem per token = 14434244 bytes main: load time =1311.74 ms main: sample time = 278.96 ms main:predict time =7375.89 ms / 54.23 ms per token main:total time =9216.61 ms
资源使用情况
第二位博主表示,在运行时,13B模型使用了大约4GB的内存,以及748%的CPU。(设定的就是让模型使用8个CPU核心)
没有指令微调
GPT-3和ChatGPT效果如此之好的关键原因之一是,它们都经过了指令微调,
这种额外的训练使它们有能力对人类的指令做出有效的反应。比如「总结一下这个」或「写一首关于水獭的诗」或「从这篇文章中提取要点」。
撰写教程的博主表示,据他观察,LLaMA并没有这样的能力。
也就是说,给LLaMA的提示需要采用经典的形式:「一些将由......完成的文本」。这也让提示工程变得更加困难。
举个例子,博主至今都还没有想出一个正确的提示,从而让LLaMA实现文本的总结。
以上がLeCun の好きなところ: Apple M1/M2 チップで LLaMA を実行! 130 億パラメータのモデルに必要なメモリはわずか 4GBの詳細内容です。詳細については、PHP 中国語 Web サイトの他の関連記事を参照してください。

ホットAIツール

Undresser.AI Undress
リアルなヌード写真を作成する AI 搭載アプリ

AI Clothes Remover
写真から衣服を削除するオンライン AI ツール。

Undress AI Tool
脱衣画像を無料で

Clothoff.io
AI衣類リムーバー

AI Hentai Generator
AIヘンタイを無料で生成します。

人気の記事

ホットツール

メモ帳++7.3.1
使いやすく無料のコードエディター

SublimeText3 中国語版
中国語版、とても使いやすい

ゼンドスタジオ 13.0.1
強力な PHP 統合開発環境

ドリームウィーバー CS6
ビジュアル Web 開発ツール

SublimeText3 Mac版
神レベルのコード編集ソフト(SublimeText3)

ホットトピック








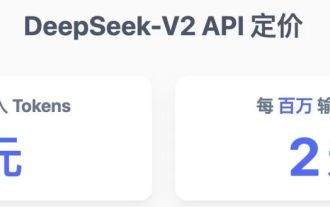
従来のコンピューティングを超える能力を備えているだけでなく、より低コストでより効率的なパフォーマンスを実現する人工知能モデルを想像してみてください。これは SF ではありません。世界で最も強力なオープンソース MoE モデルである DeepSeek-V2[1] が登場しました。 DeepSeek-V2 は、経済的なトレーニングと効率的な推論の特徴を備えた強力な専門家混合 (MoE) 言語モデルです。これは 236B のパラメータで構成されており、そのうち 21B は各マーカーをアクティブにするために使用されます。 DeepSeek67B と比較して、DeepSeek-V2 はパフォーマンスが優れていると同時に、トレーニング コストを 42.5% 節約し、KV キャッシュを 93.3% 削減し、最大生成スループットを 5.76 倍に高めます。 DeepSeek は一般的な人工知能を研究する会社です
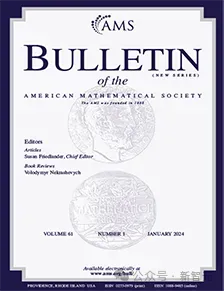
AI は確かに数学を変えつつあります。最近、この問題に細心の注意を払っている陶哲軒氏が『米国数学協会会報』(米国数学協会会報)の最新号を送ってくれた。 「機械は数学を変えるのか?」というテーマを中心に、多くの数学者が意見を述べ、そのプロセス全体は火花に満ち、ハードコアで刺激的でした。著者には、フィールズ賞受賞者のアクシャイ・ベンカテシュ氏、中国の数学者鄭楽軍氏、ニューヨーク大学のコンピューター科学者アーネスト・デイビス氏、その他業界で著名な学者を含む強力な顔ぶれが揃っている。 AI の世界は劇的に変化しています。これらの記事の多くは 1 年前に投稿されたものです。
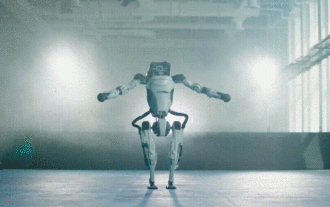
Boston Dynamics Atlas は正式に電動ロボットの時代に突入します!昨日、油圧式アトラスが歴史の舞台から「涙ながらに」撤退したばかりですが、今日、ボストン・ダイナミクスは電動式アトラスが稼働することを発表しました。ボストン・ダイナミクス社は商用人型ロボットの分野でテスラ社と競争する決意を持っているようだ。新しいビデオが公開されてから、わずか 10 時間ですでに 100 万人以上が視聴しました。古い人が去り、新しい役割が現れるのは歴史的な必然です。今年が人型ロボットの爆発的な年であることは間違いありません。ネットユーザーは「ロボットの進歩により、今年の開会式は人間のように見え、人間よりもはるかに自由度が高い。しかし、これは本当にホラー映画ではないのか?」とコメントした。ビデオの冒頭では、アトラスは仰向けに見えるように地面に静かに横たわっています。次に続くのは驚くべきことです
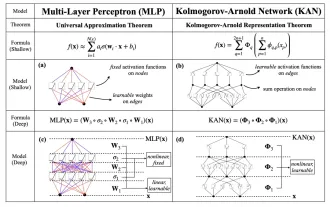
今月初め、MIT やその他の機関の研究者らは、MLP に代わる非常に有望な代替案である KAN を提案しました。 KAN は、精度と解釈可能性の点で MLP よりも優れています。また、非常に少数のパラメーターを使用して、多数のパラメーターを使用して実行する MLP よりも優れたパフォーマンスを発揮できます。たとえば、著者らは、KAN を使用して、より小規模なネットワークと高度な自動化で DeepMind の結果を再現したと述べています。具体的には、DeepMind の MLP には約 300,000 個のパラメーターがありますが、KAN には約 200 個のパラメーターしかありません。 KAN は、MLP が普遍近似定理に基づいているのに対し、KAN はコルモゴロフ-アーノルド表現定理に基づいているのと同様に、強力な数学的基礎を持っています。以下の図に示すように、KAN は
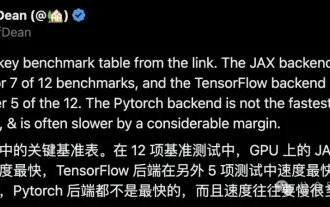
Google が推進する JAX のパフォーマンスは、最近のベンチマーク テストで Pytorch や TensorFlow のパフォーマンスを上回り、7 つの指標で 1 位にランクされました。また、テストは最高の JAX パフォーマンスを備えた TPU では行われませんでした。ただし、開発者の間では、依然として Tensorflow よりも Pytorch の方が人気があります。しかし、将来的には、おそらくより大規模なモデルが JAX プラットフォームに基づいてトレーニングされ、実行されるようになるでしょう。モデル 最近、Keras チームは、ネイティブ PyTorch 実装を使用して 3 つのバックエンド (TensorFlow、JAX、PyTorch) をベンチマークし、TensorFlow を使用して Keras2 をベンチマークしました。まず、主流のセットを選択します
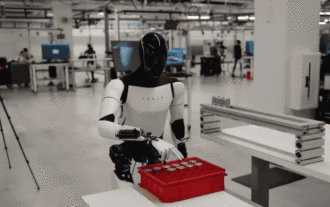
テスラのロボット「オプティマス」の最新映像が公開され、すでに工場内で稼働可能となっている。通常の速度では、バッテリー(テスラの4680バッテリー)を次のように分類します:公式は、20倍の速度でどのように見えるかも公開しました - 小さな「ワークステーション」上で、ピッキング、ピッキング、ピッキング:今回は、それがリリースされたハイライトの1つビデオの内容は、オプティマスが工場内でこの作業を完全に自律的に行い、プロセス全体を通じて人間の介入なしに完了するというものです。そして、オプティマスの観点から見ると、自動エラー修正に重点を置いて、曲がったバッテリーを拾い上げたり配置したりすることもできます。オプティマスのハンドについては、NVIDIA の科学者ジム ファン氏が高く評価しました。オプティマスのハンドは、世界の 5 本指ロボットの 1 つです。最も器用。その手は触覚だけではありません
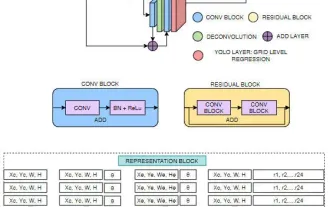
目標検出は自動運転システムにおいて比較的成熟した問題であり、その中でも歩行者検出は最も初期に導入されたアルゴリズムの 1 つです。ほとんどの論文では非常に包括的な研究が行われています。ただし、サラウンドビューに魚眼カメラを使用した距離認識については、あまり研究されていません。放射状の歪みが大きいため、標準のバウンディング ボックス表現を魚眼カメラに実装するのは困難です。上記の説明を軽減するために、拡張バウンディング ボックス、楕円、および一般的な多角形の設計を極/角度表現に探索し、これらの表現を分析するためのインスタンス セグメンテーション mIOU メトリックを定義します。提案された多角形モデルの FisheyeDetNet は、他のモデルよりも優れたパフォーマンスを示し、同時に自動運転用の Valeo 魚眼カメラ データセットで 49.5% の mAP を達成しました。
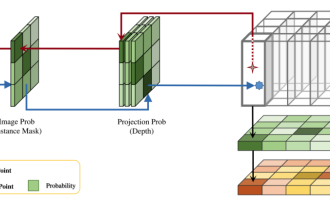
この論文では、自動運転においてさまざまな視野角 (遠近法や鳥瞰図など) から物体を正確に検出するという問題、特に、特徴を遠近法 (PV) 空間から鳥瞰図 (BEV) 空間に効果的に変換する方法について検討します。 Visual Transformation (VT) モジュールを介して実装されます。既存の手法は、2D から 3D への変換と 3D から 2D への変換という 2 つの戦略に大別されます。 2D から 3D への手法は、深さの確率を予測することで高密度の 2D フィーチャを改善しますが、特に遠方の領域では、深さ予測に固有の不確実性により不正確さが生じる可能性があります。 3D から 2D への方法では通常、3D クエリを使用して 2D フィーチャをサンプリングし、Transformer を通じて 3D と 2D フィーチャ間の対応のアテンション ウェイトを学習します。これにより、計算時間と展開時間が増加します。
