Python の視覚化ツールの使用方法
データセットの探索
データの視覚化について説明する前に、これから扱うデータセットについて簡単に見てみましょう。使用するデータは openlights から取得したものです。ルート データ セット、空港データ セット、航空会社データ セットを使用します。このうち、経路データの各行は 2 つの空港間の飛行経路に対応し、空港データの各行は世界の空港に対応し、関連情報を提供します。航空会社データの各行は各航空会社の情報を提供します。
最初にデータを読み取ります:
# Import the pandas library. import pandas # Read in the airports data. airports = pandas.read_csv("airports.csv", header=None, dtype=str) airports.columns = ["id", "name", "city", "country", "code", "icao", "latitude", "longitude", "altitude", "offset", "dst", "timezone"] # Read in the airlines data. airlines = pandas.read_csv("airlines.csv", header=None, dtype=str) airlines.columns = ["id", "name", "alias", "iata", "icao", "callsign", "country", "active"] # Read in the routes data. routes = pandas.read_csv("routes.csv", header=None, dtype=str) routes.columns = ["airline", "airline_id", "source", "source_id", "dest", "dest_id", "codeshare", "stops", "equipment"]
これらのデータには列の最初の項目がないため、列を割り当てることで列の最初の項目を追加します。属性 。各列を文字列として読み取ると、行 ID を一致として使用して異なるデータ フレームを比較する後続のステップが簡素化されるためです。これは、データの読み取り時に dtype 属性値を設定することで実現します。
その前に、データのクリーニング作業を行う必要があります。
routes = Routes[routes["airline_id"] != "//N"]
このコマンド行により、airline_id 列に数値データのみが含まれるようになります。
ヒストグラムの作成
データの構造を理解したので、さらに点の描画を開始して、この問題の調査を続けることができます。まず、ツール matplotlib を使用します。matplotlib は Python スタックの比較的低レベルのプロット ライブラリであるため、見栄えの良い曲線を作成するには、他のツール ライブラリよりも多くのコマンドが必要です。一方、matplotlib は非常に柔軟性が高いため、ほぼすべての曲線を作成できますが、柔軟性の代償として使用が非常に難しくなります。
まず、ヒストグラムを作成して、さまざまな航空会社の路線の長さの分布を示します。ヒストグラムは、すべてのルートの長さをさまざまな値の範囲に分割し、さまざまな値の範囲内にあるルートをカウントします。これから、どの航空会社が長いルートを持っているか、どの航空会社が短いルートを持っているかを知ることができます。
これを達成するには、まずルートの長さを計算する必要があります。最初のステップは、距離公式を使用することです。コサイン・セミサイン距離公式を使用して、次のように描かれた 2 点間の距離を計算します。経度と緯度、距離。
import math def haversine(lon1, lat1, lon2, lat2): # Convert coordinates to floats. lon1, lat1, lon2, lat2 = [float(lon1), float(lat1), float(lon2), float(lat2)] # Convert to radians from degrees. lon1, lat1, lon2, lat2 = map(math.radians, [lon1, lat1, lon2, lat2]) # Compute distance. dlon = lon2 - lon1 dlat = lat2 - lat1 a = math.sin(dlat/2)**2 + math.cos(lat1) * math.cos(lat2) * math.sin(dlon/2)**2 c = 2 * math.asin(math.sqrt(a)) km = 6367 * c return km
次に、関数を使用して、出発地空港と目的地空港間の片道距離を計算できます。ルート データ フレームから空港データ フレームに対応するsource_id と dest_id を取得し、それらを空港データ セットの id 列と照合する必要があります。後は計算するだけです。この関数は次のようになります:
def calc_dist(row): dist = 0 try: # Match source and destination to get coordinates. source = airports[airports["id"] == row["source_id"]].iloc[0] dest = airports[airports["id"] == row["dest_id"]].iloc[0] # Use coordinates to compute distance. dist = haversine(dest["longitude"], dest["latitude"], source["longitude"], source["latitude"]) except (ValueError, IndexError): pass return dist
If source_id 列と dest_id 列に有効な値がない場合、この関数はエラーを報告します。したがって、この無効な状況を捕捉するために try/catch モジュールを追加する必要があります。
*** では、パンダを使用して距離計算関数をルート データ フレームに適用します。これにより、すべてのルートの長さをキロメートル単位で含むパンダ シーケンスが得られます。
route_lengths = Route.apply(calc_dist, axis=1)
これで一連のルート距離が得られたので、データを対応する範囲に分類するヒストグラムを作成します。次に、それぞれの異なる範囲に該当するルートの数を数えます。
import matplotlib.pyplot as plt %matplotlib inline plt.hist(route_lengths, bins=20)
import matplotlib.pyplot as plt を使用して、matplotlib ポイント プロット関数をインポートします。次に、%matplotlib inline を使用して、ipython ノートブックに点を描画するように matplotlib を設定し、最後に、plt.hist(route_lengths, bins=20) を使用してヒストグラムを取得しました。これまで見てきたように、航空会社は長距離の長距離路線ではなく、近距離の短距離路線を運航する傾向があります。
seaborn の使用
seaborn は Python の高レベル ライブラリであり、同様の点描画を行うために使用できます。 Seaborn は matplotlib に基づいて構築されており、単純な統計処理に関連するいくつかのタイプのプロットを実行します。関数 distplot を使用して、コア確率密度の期待値に基づいてヒストグラムをプロットできます。コア密度の期待値は曲線です。本質的には、ヒストグラムよりも滑らかでルールが理解しやすい曲線です。
import seaborn seaborn.distplot(route_lengths, bins=20)
seaborn には、より美しいデフォルト スタイルもあります。 seaborn には matplotlib の各バージョンに対応するバージョンは含まれていませんが、確かに点を素早く描画する優れたツールであり、matplotlib のデフォルトのチャートと比較して、データの背後にある意味を理解するのに役立ちます。より詳細な統計作業を行いたい場合は、seaborn も優れたライブラリです。 ############棒グラフ######
柱状图也虽然很好,但是有时候我们会需要航空公司的平均路线长度。这时候我们可以使用条形图--每条航线都会有一个单独的状态条,显示航空公司航线 的平均长度。从中我们可以看出哪家是国内航空公司哪家是国际航空公司。我们可以使用pandas,一个python的数据分析库,来酸楚每个航空公司的平 均航线长度。
import numpy # Put relevant columns into a dataframe. route_length_df = pandas.DataFrame({"length": route_lengths, "id": routes["airline_id"]}) # Compute the mean route length per airline. airline_route_lengths = route_length_df.groupby("id").aggregate(numpy.mean) # Sort by length so we can make a better chart. airline_route_lengths = airline_route_lengths.sort("length", ascending=False)
我们首先用航线长度和航空公司的id来搭建一个新的数据框架。我们基于airline_id把route_length_df拆分成组,为每个航空 公司建立一个大体的数据框架。然后我们调用pandas的aggregate函数来获取航空公司数据框架中长度列的均值,然后把每个获取到的值重组到一个 新的数据模型里。之后把数据模型进行排序,这样就使得拥有最多航线的航空公司拍到了前面。
这样就可以使用matplotlib把结果画出来。
plt.bar(range(airline_route_lengths.shape[0]), airline_route_lengths["length"])
Matplotlib的plt.bar方法根据每个数据模型的航空公司平均航线长度(airline_route_lengths["length"])来做图。
问题是我们想看出哪家航空公司拥有的航线长度是什么并不容易。为了解决这个问题,我们需要能够看到坐标轴标签。这有点难,毕竟有这么多的航空公司。 一个能使问题变得简单的方法是使图表具有交互性,这样能实现放大跟缩小来查看轴标签。我们可以使用bokeh库来实现这个--它能便捷的实现交互性,作出 可缩放的图表。
要使用booked,我们需要先对数据进行预处理:
def lookup_name(row): try: # Match the row id to the id in the airlines dataframe so we can get the name. name = airlines["name"][airlines["id"] == row["id"]].iloc[0] except (ValueError, IndexError): name = "" return name # Add the index (the airline ids) as a column. airline_route_lengths["id"] = airline_route_lengths.index.copy() # Find all the airline names. airline_route_lengths["name"] = airline_route_lengths.apply(lookup_name, axis=1) # Remove duplicate values in the index. airline_route_lengths.index = range(airline_route_lengths.shape[0])
上面的代码会获取airline_route_lengths中每列的名字,然后添加到name列上,这里存贮着每个航空公司的名字。我们也添加到id列上以实现查找(apply函数不传index)。
***,我们重置索引序列以得到所有的特殊值。没有这一步,Bokeh 无法正常运行。
现在,我们可以继续说图表问题:
import numpy as np from bokeh.io import output_notebook from bokeh.charts import Bar, show output_notebook() p = Bar(airline_route_lengths, 'name', values='length', title="Average airline route lengths") show(p)
用 output_notebook 创建背景虚化,在 iPython 的 notebook 里画出图。然后,使用数据帧和特定序列制作条形图。***,显示功能会显示出该图。
这个图实际上不是一个图像--它是一个 JavaScript 插件。因此,我们在下面展示的是一幅屏幕截图,而不是真实的表格。
有了它,我们可以放大,看哪一趟航班的飞行路线最长。上面的图像让这些表格看起来挤在了一起,但放大以后,看起来就方便多了。
水平条形图
Pygal 是一个能快速制作出有吸引力表格的数据分析库。我们可以用它来按长度分解路由。首先把我们的路由分成短、中、长三个距离,并在 route_lengths 里计算出它们各占的百分比。
long_routes = len([k for k in route_lengths if k > 10000]) / len(route_lengths) medium_routes = len([k for k in route_lengths if k < 10000 and k > 2000]) / len(route_lengths) short_routes = len([k for k in route_lengths if k < 2000]) / len(route_lengths)
然后我们可以在 Pygal 的水平条形图里把每一个都绘成条形图:
import pygal from IPython.display import SVG chart = pygal.HorizontalBar() chart.title = 'Long, medium, and short routes' chart.add('Long', long_routes * 100) chart.add('Medium', medium_routes * 100) chart.add('Short', short_routes * 100) chart.render_to_file('routes.svg') SVG(filename='routes.svg')
首先,我们使用 pandasapplymethod 计算每个名称的长度。它将找到每个航空公司的名字字符的数量。然后,我们使用 matplotlib 做一个散点图来比较航空 id 的长度。当我们绘制时,我们把 theidcolumn of airlines 转换为整数类型。如果我们不这样做是行不通的,因为它需要在 x 轴上的数值。我们可以看到不少的长名字都出现在早先的 id 中。这可能意味着航空公司在成立前往往有较长的名字。
我们可以使用 seaborn 验证这个直觉。Seaborn 增强版的散点图,一个联合的点,它显示了两个变量是相关的,并有着类似地分布。
data = pandas.DataFrame({"lengths": name_lengths, "ids": airlines["id"].astype(int)})
seaborn.jointplot(x="ids", y="lengths", data=data)
画弧线
在地图上看到所有的航空路线是很酷的,幸运的是,我们可以使用 basemap 来做这件事。我们将画弧线连接所有的机场出发地和目的地。每个弧线想展示一个段都航线的路径。不幸的是,展示所有的线路又有太多的路由,这将会是一团糟。 替代,我们只现实前 3000 个路由。
# Make a base map with a mercator projection. Draw the coastlines. m = Basemap(projection='merc',llcrnrlat=-80,urcrnrlat=80,llcrnrlon=-180,urcrnrlon=180,lat_ts=20,resolution='c') m.drawcoastlines() # Iterate through the first 3000 rows. for name, row in routes[:3000].iterrows(): try: # Get the source and dest airports. source = airports[airports["id"] == row["source_id"]].iloc[0] dest = airports[airports["id"] == row["dest_id"]].iloc[0] # Don't draw overly long routes. if abs(float(source["longitude"]) - float(dest["longitude"])) < 90: # Draw a great circle between source and dest airports. m.drawgreatcircle(float(source["longitude"]), float(source["latitude"]), float(dest["longitude"]), float(dest["latitude"]),linewidth=1,color='b') except (ValueError, IndexError): pass # Show the map. plt.show()
我们将做的最终的探索是画一个机场网络图。每个机场将会是网络中的一个节点,并且如果两点之间有路由将划出节点之间的连线。如果有多重路由,将添加线的权重,以显示机场连接的更多。将使用 networkx 库来做这个功能。
首先,计算机场之间连线的权重。
# Initialize the weights dictionary. weights = {} # Keep track of keys that have been added once -- we only want edges with a weight of more than 1 to keep our network size manageable. added_keys = [] # Iterate through each route. for name, row in routes.iterrows(): # Extract the source and dest airport ids. source = row["source_id"] dest = row["dest_id"] # Create a key for the weights dictionary. # This corresponds to one edge, and has the start and end of the route. key = "{0}_{1}".format(source, dest) # If the key is already in weights, increment the weight. if key in weights: weights[key] += 1 # If the key is in added keys, initialize the key in the weights dictionary, with a weight of 2. elif key in added_keys: weights[key] = 2 # If the key isn't in added_keys yet, append it. # This ensures that we aren't adding edges with a weight of 1. else: added_keys.append(key)
一旦上面的代码运行,这个权重字典就包含了每两个机场之间权重大于或等于 2 的连线。所以任何机场有两个或者更多连接的路由将会显示出来。
# Import networkx and initialize the graph. import networkx as nx graph = nx.Graph() # Keep track of added nodes in this set so we don't add twice. nodes = set() # Iterate through each edge. for k, weight in weights.items(): try: # Split the source and dest ids and convert to integers. source, dest = k.split("_") source, dest = [int(source), int(dest)] # Add the source if it isn't in the nodes. if source not in nodes: graph.add_node(source) # Add the dest if it isn't in the nodes. if dest not in nodes: graph.add_node(dest) # Add both source and dest to the nodes set. # Sets don't allow duplicates. nodes.add(source) nodes.add(dest) # Add the edge to the graph. graph.add_edge(source, dest, weight=weight) except (ValueError, IndexError): pass pos=nx.spring_layout(graph) # Draw the nodes and edges. nx.draw_networkx_nodes(graph,pos, node_color='red', node_size=10, alpha=0.8) nx.draw_networkx_edges(graph,pos,width=1.0,alpha=1) # Show the plot. plt.show()
以上がPython の視覚化ツールの使用方法の詳細内容です。詳細については、PHP 中国語 Web サイトの他の関連記事を参照してください。

ホットAIツール

Undresser.AI Undress
リアルなヌード写真を作成する AI 搭載アプリ

AI Clothes Remover
写真から衣服を削除するオンライン AI ツール。

Undress AI Tool
脱衣画像を無料で

Clothoff.io
AI衣類リムーバー

Video Face Swap
完全無料の AI 顔交換ツールを使用して、あらゆるビデオの顔を簡単に交換できます。

人気の記事

ホットツール

メモ帳++7.3.1
使いやすく無料のコードエディター

SublimeText3 中国語版
中国語版、とても使いやすい

ゼンドスタジオ 13.0.1
強力な PHP 統合開発環境

ドリームウィーバー CS6
ビジュアル Web 開発ツール

SublimeText3 Mac版
神レベルのコード編集ソフト(SublimeText3)

ホットトピック








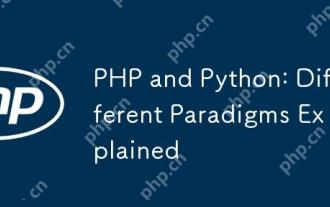
PHPは主に手順プログラミングですが、オブジェクト指向プログラミング(OOP)もサポートしています。 Pythonは、OOP、機能、手続き上のプログラミングなど、さまざまなパラダイムをサポートしています。 PHPはWeb開発に適しており、Pythonはデータ分析や機械学習などのさまざまなアプリケーションに適しています。
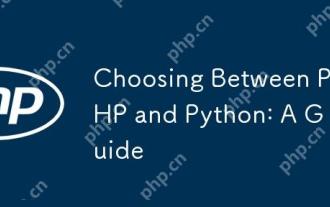
PHPはWeb開発と迅速なプロトタイピングに適しており、Pythonはデータサイエンスと機械学習に適しています。 1.PHPは、単純な構文と迅速な開発に適した動的なWeb開発に使用されます。 2。Pythonには簡潔な構文があり、複数のフィールドに適しており、強力なライブラリエコシステムがあります。
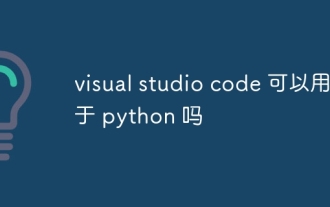
VSコードはPythonの書き込みに使用でき、Pythonアプリケーションを開発するための理想的なツールになる多くの機能を提供できます。ユーザーは以下を可能にします。Python拡張機能をインストールして、コードの完了、構文の強調表示、デバッグなどの関数を取得できます。デバッガーを使用して、コードを段階的に追跡し、エラーを見つけて修正します。バージョンコントロールのためにGitを統合します。コードフォーマットツールを使用して、コードの一貫性を維持します。糸くずツールを使用して、事前に潜在的な問題を発見します。
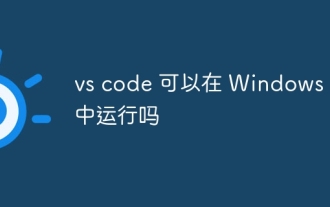
VSコードはWindows 8で実行できますが、エクスペリエンスは大きくない場合があります。まず、システムが最新のパッチに更新されていることを確認してから、システムアーキテクチャに一致するVSコードインストールパッケージをダウンロードして、プロンプトとしてインストールします。インストール後、一部の拡張機能はWindows 8と互換性があり、代替拡張機能を探すか、仮想マシンで新しいWindowsシステムを使用する必要があることに注意してください。必要な拡張機能をインストールして、適切に動作するかどうかを確認します。 Windows 8ではVSコードは実行可能ですが、開発エクスペリエンスとセキュリティを向上させるために、新しいWindowsシステムにアップグレードすることをお勧めします。
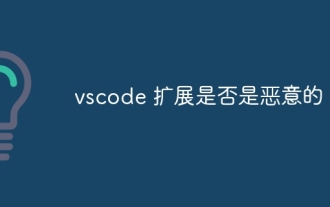
VSコード拡張機能は、悪意のあるコードの隠れ、脆弱性の活用、合法的な拡張機能としての自慰行為など、悪意のあるリスクを引き起こします。悪意のある拡張機能を識別する方法には、パブリッシャーのチェック、コメントの読み取り、コードのチェック、およびインストールに注意してください。セキュリティ対策には、セキュリティ認識、良好な習慣、定期的な更新、ウイルス対策ソフトウェアも含まれます。
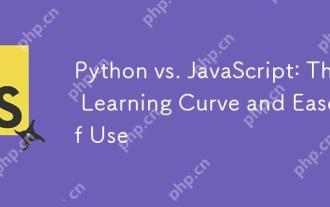
Pythonは、スムーズな学習曲線と簡潔な構文を備えた初心者により適しています。 JavaScriptは、急な学習曲線と柔軟な構文を備えたフロントエンド開発に適しています。 1。Python構文は直感的で、データサイエンスやバックエンド開発に適しています。 2。JavaScriptは柔軟で、フロントエンドおよびサーバー側のプログラミングで広く使用されています。
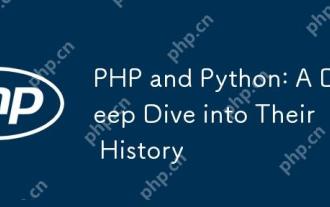
PHPは1994年に発信され、Rasmuslerdorfによって開発されました。もともとはウェブサイトの訪問者を追跡するために使用され、サーバー側のスクリプト言語に徐々に進化し、Web開発で広く使用されていました。 Pythonは、1980年代後半にGuidovan Rossumによって開発され、1991年に最初にリリースされました。コードの読みやすさとシンプルさを強調し、科学的コンピューティング、データ分析、その他の分野に適しています。
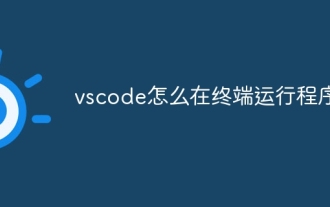
VSコードでは、次の手順を通じて端末でプログラムを実行できます。コードを準備し、統合端子を開き、コードディレクトリが端末作業ディレクトリと一致していることを確認します。プログラミング言語(pythonのpython your_file_name.pyなど)に従って実行コマンドを選択して、それが正常に実行されるかどうかを確認し、エラーを解決します。デバッガーを使用して、デバッグ効率を向上させます。
