テクスチャ コントラスト検出を使用した AI 生成画像の検出
この記事では、人工知能によって生成された画像を検出するためのディープラーニング モデルを開発する方法を紹介します。
AI が生成した画像を検出するための多くの深層学習手法は、画像の生成方法や画像の特性/セマンティクスに基づいており、通常は次のようなものです。このモデルは、人、顔、車など、人工知能によって生成された特定のオブジェクトのみを認識できます。
しかし、この研究で提案された「リッチなテクスチャ コントラストと貧しいテクスチャ コントラスト: AI 生成の画像検出のためのシンプルかつ効果的なアプローチ」というタイトルの方法は、これらの課題を克服し、より幅広い適用可能性を備えています。この研究論文を詳しく見て、AI が生成した画像を検出する他の方法が直面する問題を効果的に解決する方法を説明します。
一般化問題
モデル (ResNet-50 など) を使用して人工知能によって生成された画像を認識すると、モデルは学習します。画像のセマンティクスに基づいています。実際の画像と AI が生成したさまざまな車の画像をトレーニングに使用して、AI が生成した車の画像を認識するようにモデルをトレーニングした場合、モデルはこれらのデータから車に関する情報のみを取得できますが、他のオブジェクトについては取得できません。識別。
トレーニングはさまざまなオブジェクトのデータに対して実行できますが、この方法では時間がかかり、未知のデータに対しては約 72% の精度しか達成できません。学習回数やデータ量を増やすことで精度は向上しますが、無制限に学習データを取得できるわけではありません。
つまり、電流検出モデルの一般化には大きな問題があり、この問題を解決するために、本論文では次のような手法を提案します。
Smash&Reconstructionこの論文では、トレーニング中に画像の形状から AI が生成した特徴をモデルが学習するのを防ぐ独自の方法を紹介します。著者はこの目標を達成するためにSmash&Reconstructionと呼ばれる手法を提案しています。
この方法では、画像を所定のサイズの小さなブロックに分割し、再配置して新しい画像を生成します。生成モデルの最終入力画像を形成する前に追加の手順が必要なため、これは簡単な概要にすぎません。
#画像を小さな部分に分割した後、その小さな部分を 2 つのグループに分割します。1 つのグループはテクスチャが豊富な小さな部分であり、もう一つは、質感の悪い小さな破片です。
#画像内のオブジェクトや対照的な色の 2 つの領域間の境界などの詳細な領域は、リッチ テクスチャ ブロックになります。豊かなテクスチャ領域は、空や静止した水など、主に背景であるテクスチャ領域と比較して、ピクセルのばらつきが大きくなります。
テクスチャリッチメトリクスの計算
まず、上の画像に示すように、画像を所定のサイズの小さなチャンクに分割します。次に、これらの画像パッチのピクセル グラデーションを見つけて (つまり、水平、対角、反対角方向のピクセル値の差を見つけてそれらを加算します)、それらをリッチ テクスチャ パッチと不十分なテクスチャ パッチに分離します。
テクスチャの少ないブロックと比較して、テクスチャが豊富なブロックのピクセル グラデーション値は高くなります。画像のグラデーション値の計算式は次のとおりです。
ピクセル コントラストに基づいて画像を分離し、2 つの合成画像を取得します。このプロセスは、この記事で「Smash&Reconstruction」と呼ぶ完全なプロセスです。
#これにより、モデルはオブジェクトのコンテンツ表現ではなく、テクスチャの詳細を学習できるようになります
fingerprint
指紋ベースの方法のほとんどは画像生成テクノロジによって制限されており、これらのモデル/アルゴリズムは、拡散、GAN などの特定の方法/類似の方法によって生成された画像のみを検出できます。または他の CNN ベースの画像生成方法)。
この問題を正確に解決するために、論文ではこれらの画像パッチをリッチなテクスチャと貧弱なテクスチャに分割しました。そこで著者は、人工知能によって生成された画像内の指紋を識別する新しい方法を提案しました。これが論文のタイトルです。彼らは、30 個のハイパス フィルターを適用した後、画像内のテクスチャの豊富なパッチとテクスチャの少ないパッチの間のコントラストを見つけることを提案しました。
リッチなテクスチャ パッチと貧弱なテクスチャ パッチのコントラストはどのように役立ちますか?
理解を深めるために、実際の画像と AI で生成された画像を並べて比較します。
It is difficult to view these two images with the naked eye, right?
The paper first uses the Smash&Reconstruction process :
Contrast between each image after applying 30 high-pass filters on them:
From these results we can see that the contrast between the AI-generated images and the real images is comparable Than, the contrast between rich and poor texture patches is much higher.
In this way, we can see the difference with the naked eye, so we can put the contrast results into the trainable model and input the result data into the classifier. This is the purpose of our paper. Model architecture:
The structure of the classifier is as follows:
The paper mentions 30 high-pass filters, which were originally introduced for steganalysis.
Note: There are many ways to steganographically image. Broadly speaking, as long as information is hidden in a picture in some way and is difficult to discover through ordinary means, it can be called picture steganography. There are many related studies on steganalysis, and those who are interested can check the relevant information.
The filter here is applied to the matrix values of the image using a convolution method. The filter used is a high-pass filter, which only allows the high-frequency features of the image to pass through it. High-frequency features typically include edges, fine details, and rapid changes in intensity or color.
All filters except (f) and (g) are rotated at an angle before being reapplied to the image, thus forming a total of 30 filter. The rotation of these matrices is done using affine transformations, which are done using SciPy.
Summary
The results of the paper have reached a verification accuracy of 92%, and it is said that if more training is done, there will be better results As a result, this is a very interesting research. I also found the training code. If you are interested, you can study it in depth:
Paper: https://arxiv.org/abs/2311.12397
Code: https://github.com/hridayK/Detection-of-AI-generated-images
以上がテクスチャ コントラスト検出を使用した AI 生成画像の検出の詳細内容です。詳細については、PHP 中国語 Web サイトの他の関連記事を参照してください。

ホットAIツール

Undresser.AI Undress
リアルなヌード写真を作成する AI 搭載アプリ

AI Clothes Remover
写真から衣服を削除するオンライン AI ツール。

Undress AI Tool
脱衣画像を無料で

Clothoff.io
AI衣類リムーバー

AI Hentai Generator
AIヘンタイを無料で生成します。

人気の記事

ホットツール

メモ帳++7.3.1
使いやすく無料のコードエディター

SublimeText3 中国語版
中国語版、とても使いやすい

ゼンドスタジオ 13.0.1
強力な PHP 統合開発環境

ドリームウィーバー CS6
ビジュアル Web 開発ツール

SublimeText3 Mac版
神レベルのコード編集ソフト(SublimeText3)

ホットトピック
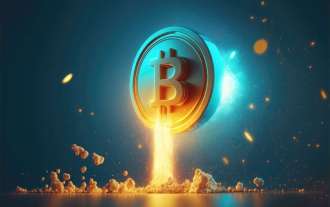
暗号通貨の人気により、仮想通貨取引プラットフォームが登場しています。世界の上位10の仮想通貨取引プラットフォームは、トランザクションの量と市場シェアに従って次のようにランク付けされています:Binance、Coinbase、FTX、Kucoin、Crypto.com、Kraken、Huobi、Gate.io、Bitfinex、Gemini。これらのプラットフォームは、幅広い暗号通貨の選択から、さまざまなレベルのトレーダーに適したデリバティブ取引に至るまで、幅広いサービスを提供しています。
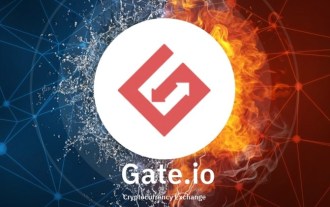
ゴマのオープンエクスチェンジを中国語に調整する方法は?このチュートリアルでは、コンピューターとAndroidの携帯電話の詳細な手順、予備的な準備から運用プロセスまで、そして一般的な問題を解決するために、セサミのオープン交換インターフェイスを中国に簡単に切り替え、取引プラットフォームをすばやく開始するのに役立ちます。
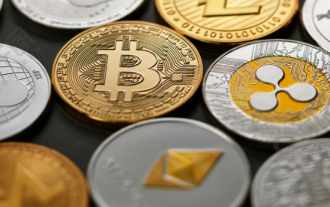
上位10の暗号通貨取引プラットフォームには、1。Okx、2。Binance、3。Gate.io、4。Kraken、5。Huobi、6。Coinbase、7。Kucoin、8。Crypto.com、9。Bitfinex、10。Gemini。プラットフォームを選択する際には、セキュリティ、流動性、処理料、通貨選択、ユーザーインターフェイス、カスタマーサポートを考慮する必要があります。
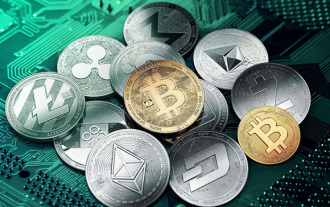
トップ10仮想通貨取引プラットフォーム2025:1。OKX、2。BINANCE、3。GATE.IO、4。Kraken、5。Huobi、6。Coinbase、7。Kucoin、8。Crypto.com、9。Bitfinex、10。Gemini。プラットフォームを選択する際には、セキュリティ、流動性、処理料、通貨選択、ユーザーインターフェイス、カスタマーサポートを考慮する必要があります。
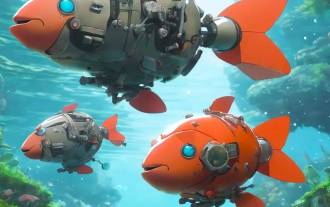
編集者 | ScienceAI 1年前、GoogleのTransformer論文の最後の著者であるLlion Jones氏は起業するために退職し、元Google研究者のDavid Ha氏と人工知能会社SakanaAIを共同設立した。 SakanaAI は、自然からインスピレーションを得たインテリジェンスに基づいて新しい基本モデルを作成すると主張しています。さて、SakanaAIは解答用紙を提出しました。 SakanaAI は、自動化された科学研究とオープンディスカバリのための世界初の AI システムである AIScientist のリリースを発表します。 AIScientist は、着想、コードの作成、実験の実行、結果の要約から、論文全体の執筆、査読の実施まで、AI 主導の科学研究と加速を可能にします。
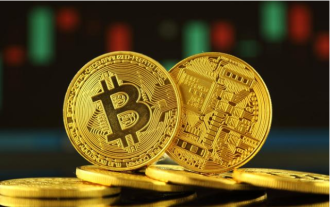
安全で信頼できるデジタル通貨プラットフォーム:1。OKX、2。Binance、3。Gate.io、4。Kraken、5。Huobi、6。Coinbase、7。Kucoin、8。Crypto.com、9。Bitfinex、10。Gemini。プラットフォームを選択する際には、セキュリティ、流動性、処理料、通貨選択、ユーザーインターフェイス、カスタマーサポートを考慮する必要があります。
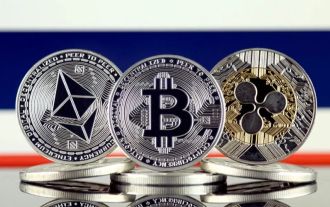
トップ10仮想通貨取引アプリのランキング:1。OKX、2。Binance、3。Gate.io、4。Kraken、5。Huobi、6。Coinbase、7。Kucoin、8。Crypto.com、9。Bitfinex、10。Gemini。プラットフォームを選択する際には、セキュリティ、流動性、処理料、通貨選択、ユーザーインターフェイス、カスタマーサポートを考慮する必要があります。
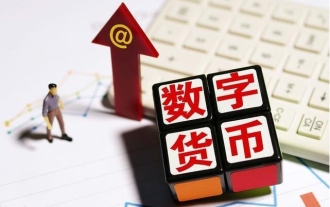
推奨される安全な仮想通貨ソフトウェアアプリ:1。Okx、2。Binance、3。Gate.io、4。Kraken、5。Huobi、6。Coinbase、7。Kucoin、8。Crypto.com、9。Bitfinex、10。Gemini。プラットフォームを選択する際には、セキュリティ、流動性、処理料、通貨選択、ユーザーインターフェイス、カスタマーサポートを考慮する必要があります。
