Python 프로그래밍 최적화 기술.
최적화된 코드는 소프트웨어의 효율성, 성능, 확장성에 직접적인 영향을 미치기 때문에 필수적입니다. 잘 작성된 코드는 더 빠르게 실행되고, 더 적은 리소스를 소비하며, 유지 관리가 더 용이하므로 더 큰 워크로드를 처리하고 사용자 경험을 개선하는 데 더 적합합니다. 또한 효율적인 코드에는 더 적은 처리 능력과 메모리가 필요하므로 운영 비용도 절감됩니다. 이는 임베디드 시스템이나 대규모 클라우드 애플리케이션과 같이 리소스가 제한된 환경에서 특히 중요합니다.
반면에 잘못 작성된 코드는 실행 시간이 느려지고 에너지 소비가 증가하며 인프라 비용이 높아질 수 있습니다. 예를 들어, 웹 애플리케이션에서 비효율적인 코드는 페이지 로드 속도를 늦추어 사용자 경험을 저하시키고 잠재적으로 사용자를 멀어지게 할 수 있습니다. 데이터 처리 작업에서 비효율적인 알고리즘은 대규모 데이터 세트를 처리하는 데 걸리는 시간을 크게 늘려 중요한 통찰력과 결정을 지연시킬 수 있습니다.
게다가 최적화된 코드는 유지 관리 및 확장이 더 간단한 경우가 많습니다. 최적화 모범 사례를 준수함으로써 개발자는 코드베이스를 깔끔하고 모듈식으로 유지하여 필요에 따라 애플리케이션을 더 쉽게 업데이트하거나 확장할 수 있습니다. 소프트웨어 프로젝트가 복잡해지고 시스템에 대한 요구가 증가함에 따라 이는 점점 더 중요해지고 있습니다.
보다 효율적이고 성능이 뛰어난 코드를 작성하는 데 도움이 될 수 있는 10가지 Python 프로그래밍 최적화 기술을 살펴보겠습니다. 이러한 기술은 시간이 지나도 확장성과 유지 관리가 가능하면서도 성능 요구 사항을 충족하는 강력한 애플리케이션을 개발하는 데 중요합니다. 이러한 기술은 모범 사례를 따르면 다른 프로그래밍 언어에도 적용할 수 있습니다.
1. 가변 패킹
가변 패킹은 여러 데이터 항목을 단일 구조로 그룹화하여 메모리 사용량을 최소화합니다. 이 기술은 대규모 데이터 처리와 같이 메모리 액세스 시간이 성능에 큰 영향을 미치는 시나리오에서 중요합니다. 관련 데이터를 함께 패킹하면 CPU 캐시를 보다 효율적으로 사용할 수 있어 데이터 검색 속도가 빨라집니다.
예:
import struct # Packing two integers into a binary format packed_data = struct.pack('ii', 10, 20) # Unpacking the packed binary data a, b = struct.unpack('ii', packed_data)
이 예에서는 struct 모듈을 사용하여 정수를 압축된 바이너리 형식으로 압축하여 데이터 처리를 더욱 효율적으로 만듭니다.
2. 스토리지 vs. 메모리
스토리지(디스크)와 메모리(RAM)의 차이점을 이해하는 것이 중요합니다. 메모리 작업은 더 빠르지만 휘발성인 반면, 스토리지는 지속적이지만 느립니다. 성능이 중요한 애플리케이션에서는 자주 액세스하는 데이터를 메모리에 유지하고 스토리지 I/O를 최소화하는 것이 속도를 위해 필수적입니다.
예:
import mmap # Memory-mapping a file with open("data.txt", "r+b") as f: mmapped_file = mmap.mmap(f.fileno(), 0) print(mmapped_file.readline()) mmapped_file.close()
메모리 매핑 파일을 사용하면 디스크 저장소를 메모리처럼 처리하여 대용량 파일의 액세스 시간을 단축할 수 있습니다.
3. 고정 길이 변수와 가변 길이 변수
고정 길이 변수는 인접한 메모리 블록에 저장되므로 액세스 및 조작이 더 빨라집니다. 반면 가변 길이 변수는 동적 메모리 할당을 관리하기 위해 추가 오버헤드가 필요하므로 특히 실시간 시스템에서 작업 속도가 느려질 수 있습니다.
예:
import array # Using fixed-length array for performance fixed_array = array.array('i', [1, 2, 3, 4, 5]) # Dynamic list (variable-length) dynamic_list = [1, 2, 3, 4, 5]
여기서 array.array는 고정 길이 배열을 제공하여 동적 목록보다 더 예측 가능한 성능을 제공합니다.
4. 내부 기능과 공개 기능
내부 기능은 정의된 모듈 내에서만 사용하도록 고안되었으며 속도와 효율성을 위해 최적화되는 경우가 많습니다. 공개 기능은 외부 사용을 위해 노출되며 추가 오류 처리 또는 로깅이 포함될 수 있어 효율성이 약간 떨어질 수 있습니다.
예:
def _private_function(data): # Optimized for internal use, with minimal error handling return data ** 2 def public_function(data): # Includes additional checks for external use if isinstance(data, int): return _private_function(data) raise ValueError("Input must be an integer")
과중한 계산을 비공개 함수에 유지함으로써 코드의 효율성을 최적화하고 외부 안전과 유용성을 위해 공개 함수를 예약합니다.
5. 기능 수정자
Python에서 데코레이터는 함수 수정자 역할을 하여 함수의 기본 실행 전후에 기능을 추가할 수 있습니다. 이는 여러 함수 호출에서 리소스 사용을 최적화할 수 있는 캐싱, 액세스 제어 또는 로깅과 같은 작업에 유용합니다.
예:
from functools import lru_cache @lru_cache(maxsize=100) def compute_heavy_function(x): # A computationally expensive operation return x ** x
lru_cache를 데코레이터로 사용하면 비용이 많이 드는 함수 호출의 결과를 캐시하여 중복 계산을 방지하여 성능을 향상시킵니다.
6. 라이브러리 사용
라이브러리를 활용하면 수레바퀴를 재발명하는 것을 피할 수 있습니다. NumPy와 같은 라이브러리는 C로 작성되고 성능을 위해 구축되었으므로 순수 Python 구현에 비해 과도한 수치 계산에 훨씬 더 효율적입니다.
예:
import numpy as np # Efficient matrix multiplication using NumPy matrix_a = np.random.rand(1000, 1000) matrix_b = np.random.rand(1000, 1000) result = np.dot(matrix_a, matrix_b)
Here, NumPy's dot function is enhanced for matrix operations, far outperforming nested loops in pure Python.
7. Short-Circuiting Conditionals
Short-circuiting reduces unnecessary evaluations, which is particularly valuable in complex condition checks or when involving resource-intensive operations. It prevents execution of conditions that don't need to be checked, saving both time and computational power.
Since conditional checks will stop the second they find the first value which satisfies the condition, you should put the variables most likely to validate/invalidate the condition first. In OR conditions (or), try to put the variable with the highest likelihood of being true first, and in AND conditions (and), try to put the variable with the highest likelihood of being false first. As soon as that variable is checked, the conditional can exit without needing to check the other values.
Example:
def complex_condition(x, y): return x != 0 and y / x > 2 # Stops evaluation if x is 0
In this example, Python’s logical operators ensure that the division is only executed if x is non-zero, preventing potential runtime errors and unnecessary computation.
8. Free Up Memory
In long-running applications, especially those dealing with large datasets, it’s essential to free up memory once it’s no longer needed. This can be done using del, gc.collect(), or by allowing objects to go out of scope.
Example:
import gc # Manual garbage collection to free up memory large_data = [i for i in range(1000000)] del large_data gc.collect() # Forces garbage collection
Using gc.collect() ensures that memory is reclaimed promptly, which is critical in memory-constrained environments.
9. Short Error Messages
In systems where memory or bandwidth is limited, such as embedded systems or logging in distributed applications, short error messages can reduce overhead. This practice also applies to scenarios where large-scale error logging is necessary.
Example:
try: result = 10 / 0 except ZeroDivisionError: print("Err: Div/0") # Short, concise error message
Short error messages are useful in environments where resource efficiency is crucial, such as IoT devices or high-frequency trading systems.
10. Optimize Loops
Loops are a common source of inefficiency, especially when processing large datasets. Optimising loops by reducing iterations, simplifying the logic, or using vectorised operations can significantly improve performance.
Example:
import numpy as np # Vectorised operation with NumPy array = np.array([1, 2, 3, 4, 5]) # Instead of looping through elements result = array * 2 # Efficient, vectorised operation
Vectorisation eliminates the need for explicit loops, leveraging low-level optimisations for faster execution.
By applying these techniques, you can ensure your Python or other programming language programs run faster, use less memory, and are more scalable, which is especially important for applications in data science, web and systems programming.
PS: you can use https://perfpy.com/#/ to check python code efficiency.
위 내용은 Python 프로그래밍 최적화 기술.의 상세 내용입니다. 자세한 내용은 PHP 중국어 웹사이트의 기타 관련 기사를 참조하세요!

핫 AI 도구

Undresser.AI Undress
사실적인 누드 사진을 만들기 위한 AI 기반 앱

AI Clothes Remover
사진에서 옷을 제거하는 온라인 AI 도구입니다.

Undress AI Tool
무료로 이미지를 벗다

Clothoff.io
AI 옷 제거제

Video Face Swap
완전히 무료인 AI 얼굴 교환 도구를 사용하여 모든 비디오의 얼굴을 쉽게 바꾸세요!

인기 기사

뜨거운 도구

메모장++7.3.1
사용하기 쉬운 무료 코드 편집기

SublimeText3 중국어 버전
중국어 버전, 사용하기 매우 쉽습니다.

스튜디오 13.0.1 보내기
강력한 PHP 통합 개발 환경

드림위버 CS6
시각적 웹 개발 도구

SublimeText3 Mac 버전
신 수준의 코드 편집 소프트웨어(SublimeText3)
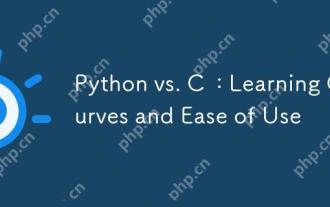
Python은 배우고 사용하기 쉽고 C는 더 강력하지만 복잡합니다. 1. Python Syntax는 간결하며 초보자에게 적합합니다. 동적 타이핑 및 자동 메모리 관리를 사용하면 사용하기 쉽지만 런타임 오류가 발생할 수 있습니다. 2.C는 고성능 응용 프로그램에 적합한 저수준 제어 및 고급 기능을 제공하지만 학습 임계 값이 높고 수동 메모리 및 유형 안전 관리가 필요합니다.
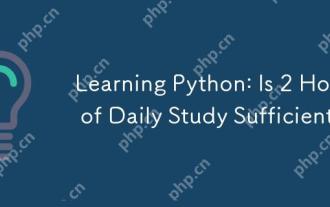
하루에 2 시간 동안 파이썬을 배우는 것으로 충분합니까? 목표와 학습 방법에 따라 다릅니다. 1) 명확한 학습 계획을 개발, 2) 적절한 학습 자원 및 방법을 선택하고 3) 실습 연습 및 검토 및 통합 연습 및 검토 및 통합,이 기간 동안 Python의 기본 지식과 고급 기능을 점차적으로 마스터 할 수 있습니다.
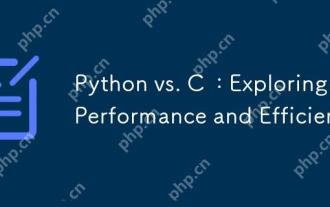
Python은 개발 효율에서 C보다 낫지 만 C는 실행 성능이 높습니다. 1. Python의 간결한 구문 및 풍부한 라이브러리는 개발 효율성을 향상시킵니다. 2.C의 컴파일 유형 특성 및 하드웨어 제어는 실행 성능을 향상시킵니다. 선택할 때는 프로젝트 요구에 따라 개발 속도 및 실행 효율성을 평가해야합니다.
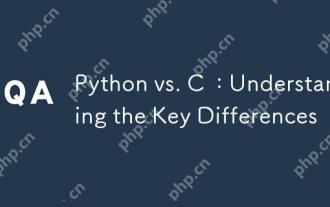
Python과 C는 각각 고유 한 장점이 있으며 선택은 프로젝트 요구 사항을 기반으로해야합니다. 1) Python은 간결한 구문 및 동적 타이핑으로 인해 빠른 개발 및 데이터 처리에 적합합니다. 2) C는 정적 타이핑 및 수동 메모리 관리로 인해 고성능 및 시스템 프로그래밍에 적합합니다.
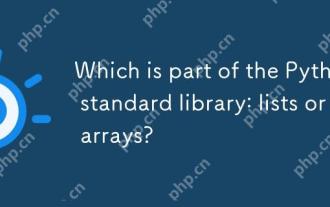
Pythonlistsarepartoftsandardlardlibrary, whileraysarenot.listsarebuilt-in, 다재다능하고, 수집 할 수있는 반면, arraysarreprovidedByTearRaymoduledlesscommonlyusedDuetolimitedFunctionality.
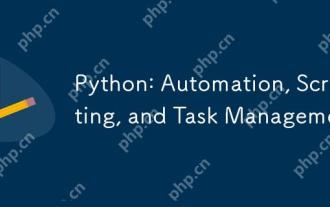
파이썬은 자동화, 스크립팅 및 작업 관리가 탁월합니다. 1) 자동화 : 파일 백업은 OS 및 Shutil과 같은 표준 라이브러리를 통해 실현됩니다. 2) 스크립트 쓰기 : PSUTIL 라이브러리를 사용하여 시스템 리소스를 모니터링합니다. 3) 작업 관리 : 일정 라이브러리를 사용하여 작업을 예약하십시오. Python의 사용 편의성과 풍부한 라이브러리 지원으로 인해 이러한 영역에서 선호하는 도구가됩니다.
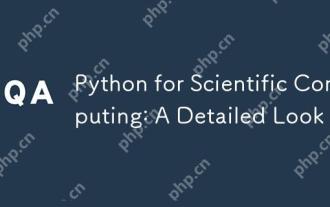
과학 컴퓨팅에서 Python의 응용 프로그램에는 데이터 분석, 머신 러닝, 수치 시뮬레이션 및 시각화가 포함됩니다. 1.numpy는 효율적인 다차원 배열 및 수학적 함수를 제공합니다. 2. Scipy는 Numpy 기능을 확장하고 최적화 및 선형 대수 도구를 제공합니다. 3. 팬더는 데이터 처리 및 분석에 사용됩니다. 4. matplotlib는 다양한 그래프와 시각적 결과를 생성하는 데 사용됩니다.
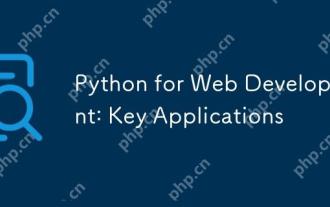
웹 개발에서 Python의 주요 응용 프로그램에는 Django 및 Flask 프레임 워크 사용, API 개발, 데이터 분석 및 시각화, 머신 러닝 및 AI 및 성능 최적화가 포함됩니다. 1. Django 및 Flask 프레임 워크 : Django는 복잡한 응용 분야의 빠른 개발에 적합하며 플라스크는 소형 또는 고도로 맞춤형 프로젝트에 적합합니다. 2. API 개발 : Flask 또는 DjangorestFramework를 사용하여 RESTFULAPI를 구축하십시오. 3. 데이터 분석 및 시각화 : Python을 사용하여 데이터를 처리하고 웹 인터페이스를 통해 표시합니다. 4. 머신 러닝 및 AI : 파이썬은 지능형 웹 애플리케이션을 구축하는 데 사용됩니다. 5. 성능 최적화 : 비동기 프로그래밍, 캐싱 및 코드를 통해 최적화
