실제 LLM 병렬 처리: 성능 향상을 위한 핵심 기술
핵심 사항
- LLM 애플리케이션의 병렬 처리 전략 마스터
- 효율적인 일괄 처리 메커니즘 구현
- 확장 가능한 문서 처리 시스템 구축
- 시스템 성능 및 리소스 활용 최적화
병렬 처리 사용 사례
LLM 응용 프로그램에서 병렬 처리는 특히 다음과 같은 경우에 적합합니다.
- 문서일괄처리
- 다중 모델 병렬 추론
- 대규모 데이터 분석
- 실시간 스트림 처리
일괄 처리 전략 설계
1. 기본 아키텍처
from typing import List, Dict, Any from dataclasses import dataclass import asyncio from langchain.chat_models import ChatOpenAI from langchain.callbacks import AsyncCallbackHandler @dataclass class BatchConfig: """Batch processing configuration""" batch_size: int = 5 max_concurrent_tasks: int = 3 timeout_seconds: int = 30 retry_attempts: int = 2 class BatchProcessor: def __init__(self, config: BatchConfig): self.config = config self.llm = ChatOpenAI( temperature=0, request_timeout=config.timeout_seconds ) self.semaphore = asyncio.Semaphore( config.max_concurrent_tasks ) async def process_batch( self, items: List[Any] ) -> List[Dict]: """Main batch processing function""" batches = self._create_batches(items) results = [] for batch in batches: batch_results = await self._process_batch_with_semaphore( batch ) results.extend(batch_results) return results
2. 비동기 처리 구현
class AsyncBatchProcessor(BatchProcessor): async def _process_single_item( self, item: Any ) -> Dict: """Process single item""" async with self.semaphore: for attempt in range(self.config.retry_attempts): try: return await self._execute_processing(item) except Exception as e: if attempt == self.config.retry_attempts - 1: return self._create_error_response(item, e) await asyncio.sleep(2 ** attempt) async def _execute_processing( self, item: Any ) -> Dict: """Execute specific processing logic""" task = asyncio.create_task( self.llm.agenerate([item]) ) try: result = await asyncio.wait_for( task, timeout=self.config.timeout_seconds ) return { "status": "success", "input": item, "result": result } except asyncio.TimeoutError: task.cancel() raise
실제 사례: 일괄 문서 처리 시스템
1. 시스템 아키텍처
class DocumentBatchProcessor: def __init__(self): self.config = BatchConfig( batch_size=10, max_concurrent_tasks=5 ) self.processor = AsyncBatchProcessor(self.config) self.results_manager = ResultsManager() async def process_documents( self, documents: List[str] ) -> Dict: """Process document batches""" try: preprocessed = await self._preprocess_documents( documents ) results = await self.processor.process_batch( preprocessed ) return await self.results_manager.merge_results( results ) except Exception as e: return self._handle_batch_error(e, documents)
2. 자원 제어 메커니즘
class ResourceController: def __init__(self): self.token_limit = 4096 self.request_limit = 100 self._request_count = 0 self._token_count = 0 self._reset_time = None async def check_limits(self) -> bool: """Check resource limits""" await self._update_counters() return ( self._request_count < self.request_limit and self._token_count < self.token_limit ) async def track_usage( self, tokens_used: int ): """Track resource usage""" self._token_count += tokens_used self._request_count += 1 async def wait_if_needed(self): """Wait for resource release if necessary""" if not await self.check_limits(): wait_time = self._calculate_wait_time() await asyncio.sleep(wait_time)
3. 결과 병합 전략
class ResultsManager: def __init__(self): self.merge_strategies = { "text": self._merge_text_results, "embeddings": self._merge_embedding_results, "classifications": self._merge_classification_results } async def merge_results( self, results: List[Dict] ) -> Dict: """Merge processing results""" merged = { "success_count": 0, "error_count": 0, "results": [] } for result in results: if result["status"] == "success": merged["success_count"] += 1 merged["results"].append( await self._process_result(result) ) else: merged["error_count"] += 1 return merged
성능 최적화 가이드
1. 메모리 관리
class MemoryManager: def __init__(self, max_memory_mb: int = 1024): self.max_memory = max_memory_mb * 1024 * 1024 self.current_usage = 0 async def monitor_memory(self): """Monitor memory usage""" import psutil process = psutil.Process() memory_info = process.memory_info() if memory_info.rss > self.max_memory: await self._trigger_memory_cleanup() async def _trigger_memory_cleanup(self): """Trigger memory cleanup""" import gc gc.collect()
2. 성능 모니터링
class PerformanceMonitor: def __init__(self): self.metrics = { "processing_times": [], "error_rates": [], "throughput": [] } async def record_metrics( self, batch_size: int, duration: float, errors: int ): """Record performance metrics""" self.metrics["processing_times"].append(duration) self.metrics["error_rates"].append(errors / batch_size) self.metrics["throughput"].append( batch_size / duration )
모범 사례
-
일괄 처리 최적화
- 시스템 리소스에 따라 배치 크기를 동적으로 조정
- 지능형 재시도 메커니즘 구현
- 메모리 사용량 모니터링 및 최적화
-
동시성 제어
- 세마포어를 사용하여 동시성 제한
- 요청 비율 제한 구현
- 합리적인 시간 초과 값 설정
-
오류 처리
- 계층형 오류 처리 구현
- 자세한 오류 정보 기록
- 우아한 성능 저하 옵션 제공
성능 조정 포인트
-
시스템 수준
- 시스템 리소스 사용량 모니터링
- 메모리 관리 최적화
- 로드 밸런싱 구현
-
적용수준
- 일괄 처리 전략 최적화
- 동시성 매개변수 조정
- 캐싱 메커니즘 구현
요약
병렬 처리는 고성능 LLM 애플리케이션을 구축하는 데 매우 중요합니다. 주요 내용:
- 효율적인 일괄 처리 전략 설계
- 강력한 자원 관리 구현
- 시스템 성능 모니터링 및 최적화
- 오류를 적절하게 처리
위 내용은 실제 LLM 병렬 처리: 성능 향상을 위한 핵심 기술의 상세 내용입니다. 자세한 내용은 PHP 중국어 웹사이트의 기타 관련 기사를 참조하세요!

핫 AI 도구

Undresser.AI Undress
사실적인 누드 사진을 만들기 위한 AI 기반 앱

AI Clothes Remover
사진에서 옷을 제거하는 온라인 AI 도구입니다.

Undress AI Tool
무료로 이미지를 벗다

Clothoff.io
AI 옷 제거제

Video Face Swap
완전히 무료인 AI 얼굴 교환 도구를 사용하여 모든 비디오의 얼굴을 쉽게 바꾸세요!

인기 기사

뜨거운 도구

메모장++7.3.1
사용하기 쉬운 무료 코드 편집기

SublimeText3 중국어 버전
중국어 버전, 사용하기 매우 쉽습니다.

스튜디오 13.0.1 보내기
강력한 PHP 통합 개발 환경

드림위버 CS6
시각적 웹 개발 도구

SublimeText3 Mac 버전
신 수준의 코드 편집 소프트웨어(SublimeText3)
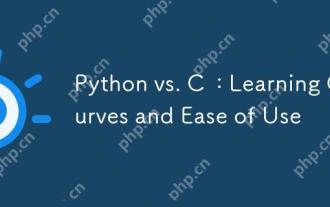
Python은 배우고 사용하기 쉽고 C는 더 강력하지만 복잡합니다. 1. Python Syntax는 간결하며 초보자에게 적합합니다. 동적 타이핑 및 자동 메모리 관리를 사용하면 사용하기 쉽지만 런타임 오류가 발생할 수 있습니다. 2.C는 고성능 응용 프로그램에 적합한 저수준 제어 및 고급 기능을 제공하지만 학습 임계 값이 높고 수동 메모리 및 유형 안전 관리가 필요합니다.
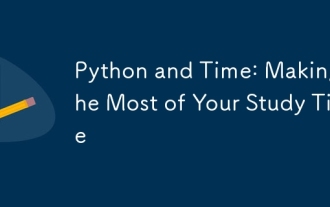
제한된 시간에 Python 학습 효율을 극대화하려면 Python의 DateTime, Time 및 Schedule 모듈을 사용할 수 있습니다. 1. DateTime 모듈은 학습 시간을 기록하고 계획하는 데 사용됩니다. 2. 시간 모듈은 학습과 휴식 시간을 설정하는 데 도움이됩니다. 3. 일정 모듈은 주간 학습 작업을 자동으로 배열합니다.
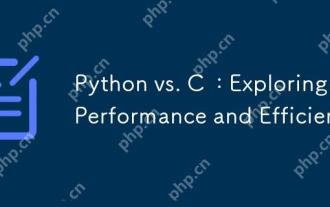
Python은 개발 효율에서 C보다 낫지 만 C는 실행 성능이 높습니다. 1. Python의 간결한 구문 및 풍부한 라이브러리는 개발 효율성을 향상시킵니다. 2.C의 컴파일 유형 특성 및 하드웨어 제어는 실행 성능을 향상시킵니다. 선택할 때는 프로젝트 요구에 따라 개발 속도 및 실행 효율성을 평가해야합니다.
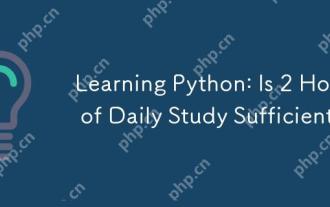
하루에 2 시간 동안 파이썬을 배우는 것으로 충분합니까? 목표와 학습 방법에 따라 다릅니다. 1) 명확한 학습 계획을 개발, 2) 적절한 학습 자원 및 방법을 선택하고 3) 실습 연습 및 검토 및 통합 연습 및 검토 및 통합,이 기간 동안 Python의 기본 지식과 고급 기능을 점차적으로 마스터 할 수 있습니다.
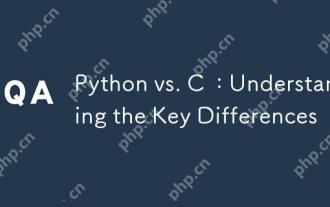
Python과 C는 각각 고유 한 장점이 있으며 선택은 프로젝트 요구 사항을 기반으로해야합니다. 1) Python은 간결한 구문 및 동적 타이핑으로 인해 빠른 개발 및 데이터 처리에 적합합니다. 2) C는 정적 타이핑 및 수동 메모리 관리로 인해 고성능 및 시스템 프로그래밍에 적합합니다.
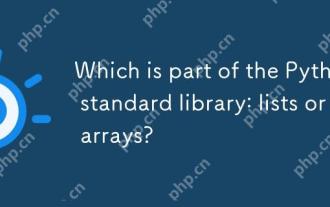
Pythonlistsarepartoftsandardlardlibrary, whileraysarenot.listsarebuilt-in, 다재다능하고, 수집 할 수있는 반면, arraysarreprovidedByTearRaymoduledlesscommonlyusedDuetolimitedFunctionality.
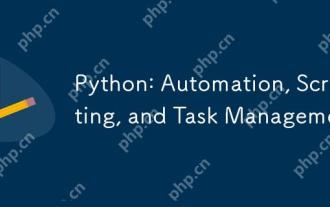
파이썬은 자동화, 스크립팅 및 작업 관리가 탁월합니다. 1) 자동화 : 파일 백업은 OS 및 Shutil과 같은 표준 라이브러리를 통해 실현됩니다. 2) 스크립트 쓰기 : PSUTIL 라이브러리를 사용하여 시스템 리소스를 모니터링합니다. 3) 작업 관리 : 일정 라이브러리를 사용하여 작업을 예약하십시오. Python의 사용 편의성과 풍부한 라이브러리 지원으로 인해 이러한 영역에서 선호하는 도구가됩니다.
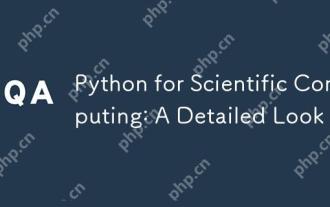
과학 컴퓨팅에서 Python의 응용 프로그램에는 데이터 분석, 머신 러닝, 수치 시뮬레이션 및 시각화가 포함됩니다. 1.numpy는 효율적인 다차원 배열 및 수학적 함수를 제공합니다. 2. Scipy는 Numpy 기능을 확장하고 최적화 및 선형 대수 도구를 제공합니다. 3. 팬더는 데이터 처리 및 분석에 사용됩니다. 4. matplotlib는 다양한 그래프와 시각적 결과를 생성하는 데 사용됩니다.
