PyTorch의 Caltech
커피 한잔 사주세요😄
*내 게시물은 Caltech 101에 대해 설명합니다.
Caltech101()은 아래와 같이 Caltech 101 데이터 세트를 사용할 수 있습니다.
*메모:
- 첫 번째 인수는 루트(필수 유형:str 또는 pathlib.Path)입니다. *절대경로, 상대경로 모두 가능합니다.
- 두 번째 인수는 target_type(Optional-Default:"category"-Type:str 또는 tuple 또는 str 목록)입니다. *"카테고리" 및/또는 "주석"을 설정할 수 있습니다.
- 세 번째 인수는 변환(Optional-Default:None-Type:callable)입니다.
- 네 번째 인수는 target_transform(Optional-Default:None-Type:callable)입니다.
- 다섯 번째 인수는 download(Optional-Default:False-Type:bool)입니다.
*메모:
- True인 경우 데이터 세트가 인터넷에서 다운로드되어 루트에 추출(압축 해제)됩니다.
- True이고 데이터세트가 이미 다운로드된 경우 추출됩니다.
- True이고 데이터 세트가 이미 다운로드되어 추출된 경우 아무 일도 일어나지 않습니다.
- 데이터 세트가 이미 다운로드되어 추출된 경우 더 빠르므로 False여야 합니다.
- 여기에서 데이터 세트(101_ObjectCategories.tar.gz 및 Annotations.tar)를 data/caltech101/에 수동으로 다운로드하고 추출할 수 있습니다.
- 이미지 인덱스의 카테고리는 Faces(0)는 0~434, Faces_easy(1)은 435~869, Leopards(2)입니다. )는 870~1069이고, 오토바이(3)는 1070~1867, 아코디언(4)은 1868~1922, 비행기(5)는 1923~2722, 앵커(6)은 2723~2764, ant(7)은 2765~2806, barrel(8)은 2807~2853, bass(9)는 2854~2907 등 .
from torchvision.datasets import Caltech101 category_data = Caltech101( root="data" ) category_data = Caltech101( root="data", target_type="category", transform=None, target_transform=None, download=False ) annotation_data = Caltech101( root="data", target_type="annotation" ) all_data = Caltech101( root="data", target_type=["category", "annotation"] ) len(category_data), len(annotation_data), len(all_data) # (8677, 8677, 8677) category_data # Dataset Caltech101 # Number of datapoints: 8677 # Root location: data\caltech101 # Target type: ['category'] category_data.root # 'data/caltech101' category_data.target_type # ['category'] print(category_data.transform) # None print(category_data.target_transform) # None category_data.download # <bound method Caltech101.download of Dataset Caltech101 # Number of datapoints: 8677 # Root location: data\caltech101 # Target type: ['category']> len(category_data.categories) # 101 category_data.categories # ['Faces', 'Faces_easy', 'Leopards', 'Motorbikes', 'accordion', # 'airplanes', 'anchor', 'ant', 'barrel', 'bass', 'beaver', # 'binocular', 'bonsai', 'brain', 'brontosaurus', 'buddha', # 'butterfly', 'camera', 'cannon', 'car_side', 'ceiling_fan', # 'cellphone', 'chair', 'chandelier', 'cougar_body', 'cougar_face', ...] len(category_data.annotation_categories) # 101 category_data.annotation_categories # ['Faces_2', 'Faces_3', 'Leopards', 'Motorbikes_16', 'accordion', # 'Airplanes_Side_2', 'anchor', 'ant', 'barrel', 'bass', # 'beaver', 'binocular', 'bonsai', 'brain', 'brontosaurus', # 'buddha', 'butterfly', 'camera', 'cannon', 'car_side', # 'ceiling_fan', 'cellphone', 'chair', 'chandelier', 'cougar_body', ...] category_data[0] # (<PIL.JpegImagePlugin.JpegImageFile image mode=RGB size=510x337>, 0) category_data[1] # (<PIL.JpegImagePlugin.JpegImageFile image mode=RGB size=519x343>, 0) category_data[2] # (<PIL.JpegImagePlugin.JpegImageFile image mode=RGB size=492x325>, 0) category_data[435] # (<PIL.JpegImagePlugin.JpegImageFile image mode=RGB size=290x334>, 1) category_data[870] # (<PIL.JpegImagePlugin.JpegImageFile image mode=RGB size=192x128>, 2) annotation_data[0] # (<PIL.JpegImagePlugin.JpegImageFile image mode=RGB size=510x337>, # array([[10.00958466, 8.18210863, 8.18210863, 10.92332268, ...], # [132.30670927, 120.42811502, 103.52396166, 90.73162939, ...]])) annotation_data[1] # (<PIL.JpegImagePlugin.JpegImageFile image mode=RGB size=519x343>, # array([[15.19298246, 13.71929825, 15.19298246, 19.61403509, ...], # [121.5877193, 103.90350877, 80.81578947, 64.11403509, ...]])) annotation_data[2] # (<PIL.JpegImagePlugin.JpegImageFile image mode=RGB size=492x325>, # array([[10.40789474, 7.17807018, 5.79385965, 9.02368421, ...], # [131.30789474, 120.69561404, 102.23947368, 86.09035088, ...]])) annotation_data[435] # (<PIL.JpegImagePlugin.JpegImageFile image mode=RGB size=290x334>, # array([[64.52631579, 95.31578947, 123.26315789, 149.31578947, ...], # [15.42105263, 8.31578947, 10.21052632, 28.21052632, ...]])) annotation_data[870] # (<PIL.JpegImagePlugin.JpegImageFile image mode=RGB size=192x128>, # array([[2.96536524, 7.55604534, 19.45780856, 33.73992443, ...], # [23.63413098, 32.13539043, 33.83564232, 8.84193955, ...]])) all_data[0] # (<PIL.JpegImagePlugin.JpegImageFile image mode=RGB size=510x337>, # (0, array([[10.00958466, 8.18210863, 8.18210863, 10.92332268, ...], # [132.30670927, 120.42811502, 103.52396166, 90.73162939, ...]])) all_data[1] # (<PIL.JpegImagePlugin.JpegImageFile image mode=RGB size=519x343>, # (0, array([[15.19298246, 13.71929825, 15.19298246, 19.61403509, ...], # [121.5877193, 103.90350877, 80.81578947, 64.11403509, ...]])) all_data[2] # (<PIL.JpegImagePlugin.JpegImageFile image mode=RGB size=492x325>, # (0, array([[10.40789474, 7.17807018, 5.79385965, 9.02368421, ...], # [131.30789474, 120.69561404, 102.23947368, 86.09035088, ...]])) all_data[3] # (<PIL.JpegImagePlugin.JpegImageFile image mode=RGB size=538x355>, # (0, array([[19.54035088, 18.57894737, 26.27017544, 38.2877193, ...], # [131.49122807, 100.24561404, 74.2877193, 49.29122807, ...]])) all_data[4] # (<PIL.JpegImagePlugin.JpegImageFile image mode=RGB size=528x349>, # (0, array([[11.87982456, 11.87982456, 13.86578947, 15.35526316, ...], # [128.34649123, 105.50789474, 91.60614035, 76.71140351, ...]])) import matplotlib.pyplot as plt def show_images(data, main_title=None): plt.figure(figsize=(10, 5)) plt.suptitle(t=main_title, y=1.0, fontsize=14) ims = (0, 1, 2, 435, 870, 1070, 1868, 1923, 2723, 2765, 2807, 2854) for i, j in enumerate(ims, start=1): plt.subplot(2, 5, i) if len(data.target_type) == 1: if data.target_type[0] == "category": im, lab = data[j] plt.title(label=lab) elif data.target_type[0] == "annotation": im, (px, py) = data[j] plt.scatter(x=px, y=py) plt.imshow(X=im) elif len(data.target_type) == 2: if data.target_type[0] == "category": im, (lab, (px, py)) = data[j] elif data.target_type[0] == "annotation": im, ((px, py), lab) = data[j] plt.title(label=lab) plt.imshow(X=im) plt.scatter(x=px, y=py) if i == 10: break plt.tight_layout() plt.show() show_images(data=category_data, main_title="category_data") show_images(data=annotation_data, main_title="annotation_data") show_images(data=all_data, main_title="all_data")
위 내용은 PyTorch의 Caltech의 상세 내용입니다. 자세한 내용은 PHP 중국어 웹사이트의 기타 관련 기사를 참조하세요!

핫 AI 도구

Undresser.AI Undress
사실적인 누드 사진을 만들기 위한 AI 기반 앱

AI Clothes Remover
사진에서 옷을 제거하는 온라인 AI 도구입니다.

Undress AI Tool
무료로 이미지를 벗다

Clothoff.io
AI 옷 제거제

Video Face Swap
완전히 무료인 AI 얼굴 교환 도구를 사용하여 모든 비디오의 얼굴을 쉽게 바꾸세요!

인기 기사

뜨거운 도구

메모장++7.3.1
사용하기 쉬운 무료 코드 편집기

SublimeText3 중국어 버전
중국어 버전, 사용하기 매우 쉽습니다.

스튜디오 13.0.1 보내기
강력한 PHP 통합 개발 환경

드림위버 CS6
시각적 웹 개발 도구

SublimeText3 Mac 버전
신 수준의 코드 편집 소프트웨어(SublimeText3)

뜨거운 주제










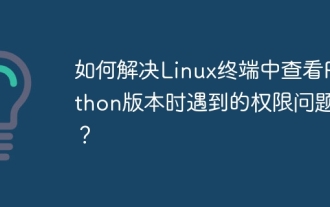
Linux 터미널에서 Python 버전을 보려고 할 때 Linux 터미널에서 Python 버전을 볼 때 권한 문제에 대한 솔루션 ... Python을 입력하십시오 ...
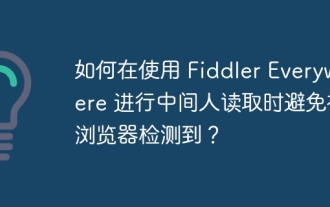
Fiddlerevery Where를 사용할 때 Man-in-the-Middle Reading에 Fiddlereverywhere를 사용할 때 감지되는 방법 ...
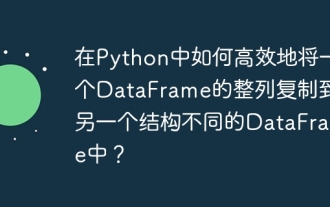
Python의 Pandas 라이브러리를 사용할 때는 구조가 다른 두 데이터 프레임 사이에서 전체 열을 복사하는 방법이 일반적인 문제입니다. 두 개의 dats가 있다고 가정 해
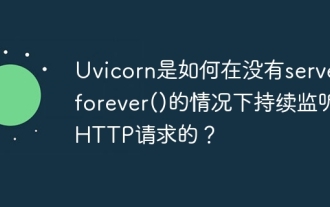
Uvicorn은 HTTP 요청을 어떻게 지속적으로 듣습니까? Uvicorn은 ASGI를 기반으로 한 가벼운 웹 서버입니다. 핵심 기능 중 하나는 HTTP 요청을 듣고 진행하는 것입니다 ...
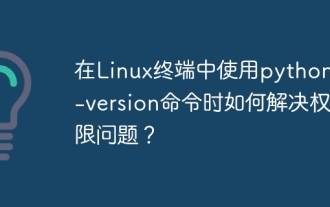
Linux 터미널에서 Python 사용 ...
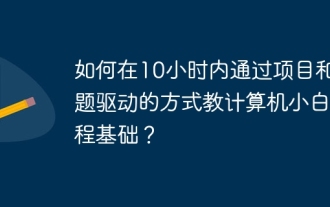
10 시간 이내에 컴퓨터 초보자 프로그래밍 기본 사항을 가르치는 방법은 무엇입니까? 컴퓨터 초보자에게 프로그래밍 지식을 가르치는 데 10 시간 밖에 걸리지 않는다면 무엇을 가르치기로 선택 하시겠습니까?
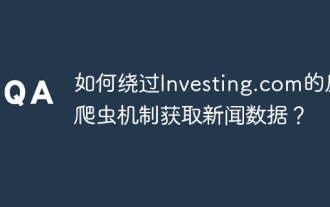
Investing.com의 크롤링 전략 이해 많은 사람들이 종종 Investing.com (https://cn.investing.com/news/latest-news)에서 뉴스 데이터를 크롤링하려고합니다.
