AWS Bedrock을 사용하여 AI 트래픽 혼잡 예측기 배포: 전체 개요
우리 모두 교통을 좋아하죠? 내가 발표를 완전히 망쳤다는 생각이 드는 유일한 시간입니다(과도한 생각은 고통입니다).
농담은 제쳐두고 PoC로서 실시간으로 트래픽을 확인할 수 있는 프로젝트를 만들어서 앞으로 더욱 발전시키고 싶었습니다. 교통 정체 예측기를 만나보세요.
AWS Bedrock을 사용하여 Traffic Congestion Predictor를 배포하는 과정을 살펴보겠습니다. AWS Bedrock은 기초 모델을 위한 완전 관리형 서비스를 제공하므로 AI 애플리케이션 배포에 적합합니다. 초기 설정부터 최종 배포 및 테스트까지 모든 것을 다룹니다.
이제 전제조건은
- 적절한 권한이 있는 AWS 계정(직불 카드를 특정 한도 내에서 무료로 사용할 수 있다고 가정했기 때문에 확인을 위해 직불 카드를 사용해야 했습니다. 어려움).
- 파이썬 3.8
- 교통 혼잡 예측 코드(이전 개발)
- AWS CLI 설치 및 구성
- Python 및 AWS 서비스에 대한 기본 지식만으로도 충분합니다.
1단계: 환경 준비
먼저 개발 환경을 설정하세요.
# Create a new virtual environment python -m venv bedrock-env source bedrock-env/bin/activate # On Windows use: bedrock-env\Scripts\activate # Install required packages pip install boto3 pandas numpy scikit-learn streamlit plotly
2단계: AWS Bedrock 설정
AWS 콘솔로 이동하여 AWS Bedrock을 활성화합니다
Bedrock에서 새 모델 만들기:
- AWS Bedrock 콘솔로 이동
- "모델 액세스"를 선택하세요
- 클로드 모델 패밀리 접근 권한 요청
- 승인을 기다립니다(대개는 즉각적이지만 어떤 일이 일어날 수 있음)
3단계: Bedrock 통합을 위한 코드 수정
새 파일 만들기 "bedrock_integration.py":
import boto3 import json import numpy as np import pandas as pd from typing import Dict, Any class TrafficPredictor: def __init__(self): self.bedrock = boto3.client( service_name='bedrock-runtime', region_name='us-east-1' # Change to your region ) def prepare_features(self, input_data: Dict[str, Any]) -> pd.DataFrame: # Convert input data to model features hour = input_data['hour'] day = input_data['day'] features = pd.DataFrame({ 'hour_sin': [np.sin(2 * np.pi * hour/24)], 'hour_cos': [np.cos(2 * np.pi * hour/24)], 'day_sin': [np.sin(2 * np.pi * day/7)], 'day_cos': [np.cos(2 * np.pi * day/7)], 'temperature': [input_data['temperature']], 'precipitation': [input_data['precipitation']], 'special_event': [input_data['special_event']], 'road_work': [input_data['road_work']], 'vehicle_count': [input_data['vehicle_count']] }) return features def predict(self, input_data: Dict[str, Any]) -> float: features = self.prepare_features(input_data) # Prepare prompt for Claude prompt = f""" Based on the following traffic conditions, predict the congestion level (0-10): - Time: {input_data['hour']}:00 - Day of week: {input_data['day']} - Temperature: {input_data['temperature']}°C - Precipitation: {input_data['precipitation']}mm - Special event: {'Yes' if input_data['special_event'] else 'No'} - Road work: {'Yes' if input_data['road_work'] else 'No'} - Vehicle count: {input_data['vehicle_count']} Return only the numerical prediction. """ # Call Bedrock response = self.bedrock.invoke_model( modelId='anthropic.claude-v2', body=json.dumps({ "prompt": prompt, "max_tokens": 10, "temperature": 0 }) ) # Parse response response_body = json.loads(response['body'].read()) prediction = float(response_body['completion'].strip()) return np.clip(prediction, 0, 10)
4단계: FastAPI 백엔드 생성
"api.py:"
만들기
from fastapi import FastAPI, HTTPException from pydantic import BaseModel from bedrock_integration import TrafficPredictor from typing import Dict, Any app = FastAPI() predictor = TrafficPredictor() class PredictionInput(BaseModel): hour: int day: int temperature: float precipitation: float special_event: bool road_work: bool vehicle_count: int @app.post("/predict") async def predict_traffic(input_data: PredictionInput) -> Dict[str, float]: try: prediction = predictor.predict(input_data.dict()) return {"congestion_level": prediction} except Exception as e: raise HTTPException(status_code=500, detail=str(e))
5단계: AWS 인프라 생성
"infrastructure.py" 만들기:
import boto3 import json def create_infrastructure(): # Create ECR repository ecr = boto3.client('ecr') try: ecr.create_repository(repositoryName='traffic-predictor') except ecr.exceptions.RepositoryAlreadyExistsException: pass # Create ECS cluster ecs = boto3.client('ecs') ecs.create_cluster(clusterName='traffic-predictor-cluster') # Create task definition task_def = { 'family': 'traffic-predictor', 'containerDefinitions': [{ 'name': 'traffic-predictor', 'image': f'{ecr.describe_repositories()["repositories"][0]["repositoryUri"]}:latest', 'memory': 512, 'cpu': 256, 'essential': True, 'portMappings': [{ 'containerPort': 8000, 'hostPort': 8000, 'protocol': 'tcp' }] }], 'requiresCompatibilities': ['FARGATE'], 'networkMode': 'awsvpc', 'cpu': '256', 'memory': '512' } ecs.register_task_definition(**task_def)
6단계: 애플리케이션 컨테이너화
"Dockerfile:"
만들기
FROM python:3.9-slim WORKDIR /app COPY requirements.txt . RUN pip install --no-cache-dir -r requirements.txt COPY . . CMD ["uvicorn", "api:app", "--host", "0.0.0.0", "--port", "8000"]
"requirements.txt:"
만들기
fastapi uvicorn boto3 pandas numpy scikit-learn
7단계: AWS에 배포
다음 명령을 실행하세요.
# Build and push Docker image aws ecr get-login-password --region us-east-1 | docker login --username AWS --password-stdin $AWS_ACCOUNT_ID.dkr.ecr.us-east-1.amazonaws.com docker build -t traffic-predictor . docker tag traffic-predictor:latest $AWS_ACCOUNT_ID.dkr.ecr.us-east-1.amazonaws.com/traffic-predictor:latest docker push $AWS_ACCOUNT_ID.dkr.ecr.us-east-1.amazonaws.com/traffic-predictor:latest # Create infrastructure python infrastructure.py
8단계: Streamlit 프런트엔드 업데이트
"app.py"를 수정하여 API에 연결하세요.
import streamlit as st import requests import plotly.graph_objects as go import plotly.express as px API_ENDPOINT = "your-api-endpoint" def predict_traffic(input_data): response = requests.post(f"{API_ENDPOINT}/predict", json=input_data) return response.json()["congestion_level"] # Rest of the Streamlit code remains the same, but replace direct model calls # with API calls using predict_traffic()
9단계: 테스트 및 모니터링
API 엔드포인트 테스트:
curl -X POST "your-api-endpoint/predict" \ -H "Content-Type: application/json" \ -d '{"hour":12,"day":1,"temperature":25,"precipitation":0,"special_event":false,"road_work":false,"vehicle_count":1000}'
AWS CloudWatch를 사용하여 모니터링:
- CloudWatch 대시보드 설정
- 오류율 및 지연 시간에 대한 경보 생성
- API 사용량 및 비용 모니터링
모든 일이 잘 된다면. 축하해요! 교통 혼잡 예측기를 성공적으로 배포했습니다. 그 일을 위해 등을 대십시오! 비용과 성능을 모니터링하고, 정기적으로 모델을 업데이트하고, CI/CD 파이프라인을 구현해야 합니다. 다음 단계는 사용자 인증 추가, 모니터링 및 경고 강화, 모델 성능 최적화, 사용자 피드백을 기반으로 더 많은 기능 추가입니다.
이 글을 읽어주셔서 감사합니다. 생각이나 질문, 관찰 사항이 있으면 알려주세요!
위 내용은 AWS Bedrock을 사용하여 AI 트래픽 혼잡 예측기 배포: 전체 개요의 상세 내용입니다. 자세한 내용은 PHP 중국어 웹사이트의 기타 관련 기사를 참조하세요!

핫 AI 도구

Undresser.AI Undress
사실적인 누드 사진을 만들기 위한 AI 기반 앱

AI Clothes Remover
사진에서 옷을 제거하는 온라인 AI 도구입니다.

Undress AI Tool
무료로 이미지를 벗다

Clothoff.io
AI 옷 제거제

AI Hentai Generator
AI Hentai를 무료로 생성하십시오.

인기 기사

뜨거운 도구

메모장++7.3.1
사용하기 쉬운 무료 코드 편집기

SublimeText3 중국어 버전
중국어 버전, 사용하기 매우 쉽습니다.

스튜디오 13.0.1 보내기
강력한 PHP 통합 개발 환경

드림위버 CS6
시각적 웹 개발 도구

SublimeText3 Mac 버전
신 수준의 코드 편집 소프트웨어(SublimeText3)

뜨거운 주제










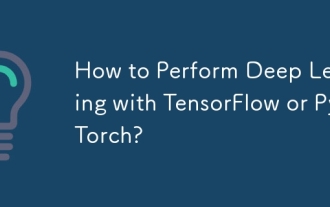
Tensorflow 또는 Pytorch로 딥 러닝을 수행하는 방법은 무엇입니까?
