Efficient Indexing in MongoDB 2.6
By Osmar Olivo, Product Manager at MongoDB One of the most powerful features of MongoDB is its rich indexing functionality. Users can specify secondary indexes on any field, compound indexes, geospatial, text, sparse, TTL, and others. Havi
By Osmar Olivo, Product Manager at MongoDB
One of the most powerful features of MongoDB is its rich indexing functionality. Users can specify secondary indexes on any field, compound indexes, geospatial, text, sparse, TTL, and others. Having extensive indexing functionality makes it easier for developers to build apps that provide rich functionality and low latency.
MongoDB 2.6 introduces a new query planner, including the ability to perform index intersection. Prior to 2.6 the query planner could only make use of a single index for most queries. That meant that if you wanted to query on multiple fields together, you needed to create a compound index. It also meant that if there were several different combinations of fields you wanted to query on, you might need several different compound indexes.
Each index adds overhead to your deployment - indexes consume space, on disk and in RAM, and indexes are maintained during updates, which adds disk IO. In other words, indexes improve the efficiency of many operations, but they also come at a cost. For many applications, index intersection will allow users to reduce the number of indexes they need while still providing rich features and low latency.
In the following sections we will take a deep dive into index intersection and how it can be applied to applications.
An Example - The Phone Book
Let’s take the example of a phone book with the following schema.
{ FirstName LastName Phone_Number Address }
If I were to search for “Smith, John” how would I index the following query to be as efficient as possible?
db.phonebook.find({ FirstName : “John”, LastName : “Smith” })
I could use an individual index on FirstName and search for all of the “Johns”.
This would look something like ensureIndex( { FirstName : 1 } )
We run this query and we get back 200,000 John Smiths. Looking at the explain() output below however, we see that we scanned 1,000,000 “Johns” in the process of finding 200,000 “John Smiths”.
> db.phonebook.find({ FirstName : "John", LastName : "Smith"}).explain() { "cursor" : "BtreeCursor FirstName_1", "isMultiKey" : false, "n" : 200000, "nscannedObjects" : 1000000, "nscanned" : 1000000, "nscannedObjectsAllPlans" : 1000101, "nscannedAllPlans" : 1000101, "scanAndOrder" : false, "indexOnly" : false, "nYields" : 2, "nChunkSkips" : 0, "millis" : 2043, "indexBounds" : { "FirstName" : [ [ "John", "John" ] ] }, "server" : "Oz-Olivo-MacBook-Pro.local:27017" }
How about creating an individual index on LastName?
This would look something like ensureIndex( { LastName : 1 } )
Running this query we get back 200,000 “John Smiths” but our explain output says that we now scanned 400,000 “Smiths”. How can we make this better?
db.phonebook.find({ FirstName : "John", LastName : "Smith"}).explain() { "cursor" : "BtreeCursor LastName_1", "isMultiKey" : false, "n" : 200000, "nscannedObjects" : 400000, "nscanned" : 400000, "nscannedObjectsAllPlans" : 400101, "nscannedAllPlans" : 400101, "scanAndOrder" : false, "indexOnly" : false, "nYields" : 1, "nChunkSkips" : 0, "millis" : 852, "indexBounds" : { "LastName" : [ [ "Smith", "Smith" ] ] }, "server" : "Oz-Olivo-MacBook-Pro.local:27017" }
So we know that there are 1,000,000 “John” entries, 400,000 “Smith” entries, and 200,000 “John Smith” entries in our phonebook. Is there a way that we can scan just the 200,000 we need?
In the case of a phone book this is somewhat simple; since we know that we want it to be sorted by Lastname, Firstname we can create a compound index on them, like the below.
ensureIndex( { LastName : true, FirstName : 1 } ) db.phonebook.find({ FirstName : "John", LastName : "Smith"}).explain() { "cursor" : "BtreeCursor LastName_1_FirstName_1", "isMultiKey" : false, "n" : 200000, "nscannedObjects" : 200000, "nscanned" : 200000, "nscannedObjectsAllPlans" : 200000, "nscannedAllPlans" : 200000, "scanAndOrder" : false, "indexOnly" : false, "nYields" : 0, "nChunkSkips" : 0, "millis" : 370, "indexBounds" : { "LastName" : [ [ "Smith", "Smith" ] ], "FirstName" : [ [ "John", "John" ] ] }, "server" : "Oz-Olivo-MacBook-Pro.local:27017" }
Looking at the explain on this, we see that the index only scanned the 200,000 documents that matched, so we got a perfect hit.
Beyond Compound Indexes
The compound index is a great solution in the case of a phonebook in which we always know how we are going to be querying our data. Now what if we have an application in which users can arbitrarily query for different fields together? We can’t possibly create a compound index for every possible combination because of the overhead imposed by indexes, as we discussed above, and because MongoDB limits you to 64 indexes per collection. Index intersection can really help.
Imagine the case of a medical application which doctors use to filter through patients. At a high level, omitting several details, a basic schema may look something like the below.
{ Fname LName SSN Age Blood_Type Conditions : [] Medications : [ ] ... ... }
Some sample searches that a doctor/nurse may run on this system would look something like the below.
Find me a Patient with Blood_Type = O under the age of 50
db.patients.find( { Blood_Type : “O”, Age : { $lt : 50 } } )
Find me all patients over the age of 60 on Medication X
db.patients.find( { Medications : “X” , Age : { $gt : 60} })
Find me all Diabetic patients on medication Y
db.patients.find( { Conditions : “Diabetes”, Medications : “Y” } )
With all of the unstructured data in modern applications, along with the desire to be able to search for things as needed in an ad-hoc way, it can become very difficult to predict usage patterns. Since we can’t possibly create compound indexes for every combination of fields, because we don’t necessarily know what those will be ahead of time, we can try indexing individual fields to try to salvage some performance. But as shown above in our phone book application, this can lead to performance issues in which we pull documents into memory that are not matches.
To avoid the paging of unnecessary data, the new index intersection feature in 2.6 increases the overall efficiency of these types of ad-hoc queries by processing the indexes involved individually and then intersecting the result set to find the matching documents. This means you only pull the final matching documents into memory and everything else is processed using the indexes. This processing will utilize more CPU, but should greatly reduce the amount of IO done for queries where all of the data is not in memory as well as allow you to utilize your memory more efficiently.
For example, looking at the earlier example:
db.patients.find( { Blood_Type : “O”, Age : { $lt : 50 } } )
It is inefficient to find all patients with BloodType: O (which could be millions) and then pull into memory each document to find the ones with age
Instead, the query planner finds all patients with bloodType: O using the index on BloodType, and all patients with age
Index intersection allows for much more efficient use of existing RAM so less total memory will usually be required to fit the working set then previously. Also, if you had several compound indices that were made up of different combinations of fields, then you can reduce the total number of indexes on the system. This means storing less indices in memory as well as achieving better insert/update performance since fewer indices must be updated.
As of version 2.6.0, you cannot intersect with geo or text indices and you can intersect at most 2 separate indices with each other. These limitations are likely to change in a future release.
Optimizing Multi-key Indexes It is also possible to intersect an index with itself in the case of multi-key indexes. Consider the below query:
Find me all patients with Diabetes & High Blood Pressure
db.patients.find( { Conditions : { $all : [ “Diabetes”, “High Blood Pressure” ] } } )
In this case we will find the result set of all Patients with Diabetes, and the result set of all patients with High blood pressure, and intersect the two to get all patients with both. Again, this requires less memory and disk speed for better overall performance. As of the 2.6.0 release, an index can intersect with itself up to 10 times.
Do We Still Need Compound Indexes?
To be clear, compound indexing will ALWAYS be more performant IF you know what you are going to be querying on and can create one ahead of time. Furthermore, if your working set is entirely in memory, then you will not reap any of the benefits of Index Intersection as it is primarily based on reducing IO. But in a more ad-hoc case where one cannot predict the shape of the queries and the working set is much larger than available memory, index intersection will automatically take over and choose the most performant path.
- Download MongoDB 2.6 Today
- Learn about all of the key new features in MongoDB 2.6 by downloading the whitepaper
原文地址:Efficient Indexing in MongoDB 2.6, 感谢原作者分享。

Alat AI Hot

Undresser.AI Undress
Apl berkuasa AI untuk mencipta foto bogel yang realistik

AI Clothes Remover
Alat AI dalam talian untuk mengeluarkan pakaian daripada foto.

Undress AI Tool
Gambar buka pakaian secara percuma

Clothoff.io
Penyingkiran pakaian AI

Video Face Swap
Tukar muka dalam mana-mana video dengan mudah menggunakan alat tukar muka AI percuma kami!

Artikel Panas

Alat panas

Notepad++7.3.1
Editor kod yang mudah digunakan dan percuma

SublimeText3 versi Cina
Versi Cina, sangat mudah digunakan

Hantar Studio 13.0.1
Persekitaran pembangunan bersepadu PHP yang berkuasa

Dreamweaver CS6
Alat pembangunan web visual

SublimeText3 versi Mac
Perisian penyuntingan kod peringkat Tuhan (SublimeText3)

Topik panas










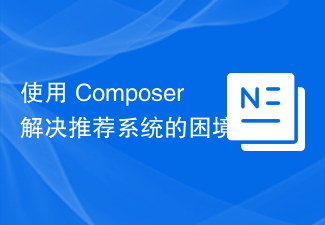
Apabila membangunkan laman web e-dagang, saya menghadapi masalah yang sukar: bagaimana menyediakan pengguna dengan cadangan produk yang diperibadikan. Pada mulanya, saya mencuba beberapa algoritma cadangan mudah, tetapi hasilnya tidak sesuai, dan kepuasan pengguna juga terjejas. Untuk meningkatkan ketepatan dan kecekapan sistem cadangan, saya memutuskan untuk menggunakan penyelesaian yang lebih profesional. Akhirnya, saya memasang Andres-Montanez/Cadangan-Bundle melalui komposer, yang bukan sahaja menyelesaikan masalah saya, tetapi juga meningkatkan prestasi sistem cadangan. Anda boleh belajar komposer melalui alamat berikut:
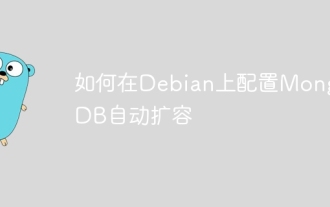
Artikel ini memperkenalkan cara mengkonfigurasi MongoDB pada sistem Debian untuk mencapai pengembangan automatik. Langkah -langkah utama termasuk menubuhkan set replika MongoDB dan pemantauan ruang cakera. 1. Pemasangan MongoDB Pertama, pastikan MongoDB dipasang pada sistem Debian. Pasang menggunakan arahan berikut: SudoaptDateSudoaptInstall-ImongoDB-Org 2. Mengkonfigurasi set replika replika MongoDB MongoDB Set memastikan ketersediaan dan kelebihan data yang tinggi, yang merupakan asas untuk mencapai pengembangan kapasiti automatik. Mula MongoDB Service: sudosystemctlstartmongodsudosys
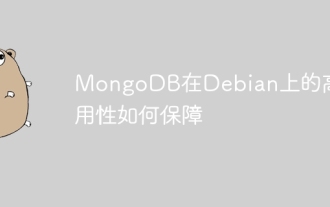
Artikel ini menerangkan cara membina pangkalan data MongoDB yang sangat tersedia pada sistem Debian. Kami akan meneroka pelbagai cara untuk memastikan keselamatan data dan perkhidmatan terus beroperasi. Strategi Utama: Replicaset: Replicaset: Gunakan replika untuk mencapai redundansi data dan failover automatik. Apabila nod induk gagal, set replika secara automatik akan memilih nod induk baru untuk memastikan ketersediaan perkhidmatan yang berterusan. Sandaran dan Pemulihan Data: Secara kerap Gunakan perintah Mongodump untuk membuat sandaran pangkalan data dan merumuskan strategi pemulihan yang berkesan untuk menangani risiko kehilangan data. Pemantauan dan penggera: Menyebarkan alat pemantauan (seperti Prometheus, Grafana) untuk memantau status MongoDB dalam masa nyata, dan
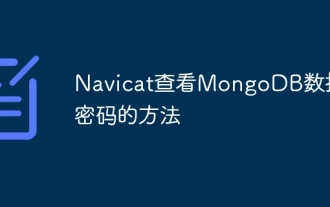
Tidak mustahil untuk melihat kata laluan MongoDB secara langsung melalui Navicat kerana ia disimpan sebagai nilai hash. Cara mendapatkan kata laluan yang hilang: 1. Tetapkan semula kata laluan; 2. Periksa fail konfigurasi (mungkin mengandungi nilai hash); 3. Semak Kod (boleh kata laluan Hardcode).
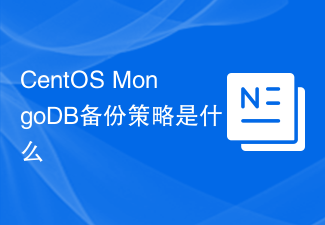
Penjelasan terperinci mengenai strategi sandaran yang cekap MongoDB di bawah sistem CentOS Artikel ini akan memperkenalkan secara terperinci pelbagai strategi untuk melaksanakan sandaran MongoDB pada sistem CentOS untuk memastikan kesinambungan data dan kesinambungan perniagaan. Kami akan merangkumi sandaran manual, sandaran masa, sandaran skrip automatik, dan kaedah sandaran dalam persekitaran kontena Docker, dan menyediakan amalan terbaik untuk pengurusan fail sandaran. Sandaran Manual: Gunakan perintah Mongodump untuk melakukan sandaran penuh manual, contohnya: Mongodump-Hlocalhost: 27017-U Pengguna-P Password-D Database Data-O/Backup Direktori Perintah ini akan mengeksport data dan metadata pangkalan data yang ditentukan ke direktori sandaran yang ditentukan.
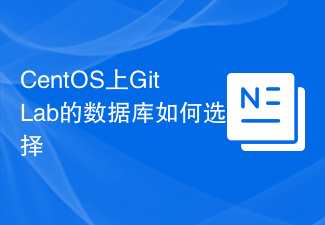
Panduan Penyebaran Pangkalan Data Gitlab pada sistem CentOS Memilih pangkalan data yang betul adalah langkah utama dalam berjaya menggunakan GitLab. Gitlab serasi dengan pelbagai pangkalan data, termasuk MySQL, PostgreSQL, dan MongoDB. Artikel ini akan menerangkan secara terperinci bagaimana untuk memilih dan mengkonfigurasi pangkalan data ini. Cadangan Pemilihan Pangkalan Data MySQL: Sistem Pengurusan Pangkalan Data Relasi yang digunakan secara meluas (RDBMS), dengan prestasi yang stabil dan sesuai untuk kebanyakan senario penempatan GitLab. PostgreSQL: RDBMS sumber terbuka yang kuat, menyokong pertanyaan kompleks dan ciri -ciri canggih, sesuai untuk mengendalikan set data yang besar. MongoDB: Pangkalan Data NoSQL Popular, Bagus Mengendalikan Laut
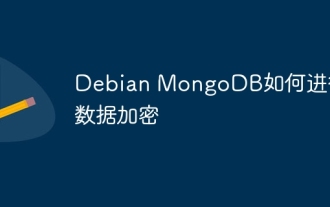
Menyulitkan pangkalan data MongoDB pada sistem Debian memerlukan langkah berikut: Langkah 1: Pasang MongoDB terlebih dahulu, pastikan sistem Debian anda dipasang MongoDB. Jika tidak, sila rujuk kepada dokumen MongoDB rasmi untuk pemasangan: https://docs.mongodb.com/manual/tutorial/install-mongodb-on-debian/step 2: menghasilkan fail kunci penyulitan Buat fail yang mengandungi kunci penyulitan dan tetapkan kebenaran yang betul:
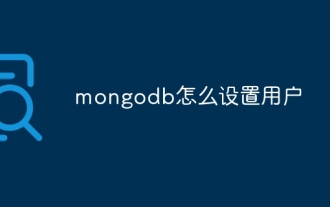
Untuk menyediakan pengguna MongoDB, ikuti langkah -langkah ini: 1. Sambungkan ke pelayan dan buat pengguna pentadbir. 2. Buat pangkalan data untuk memberikan akses pengguna. 3. Gunakan arahan CreateUser untuk membuat pengguna dan menentukan hak dan hak akses pangkalan data mereka. 4. Gunakan perintah getusers untuk memeriksa pengguna yang dibuat. 5. Secara pilihan menetapkan keizinan lain atau memberi kebenaran kepada pengguna ke koleksi tertentu.
