Amalan Penting untuk Membina Aplikasi LLM Teguh
pengenalan
Saya telah membina aplikasi LLM dalam awan. Saya juga telah melihat banyak pembangun membuat apl LLM yang sangat bagus untuk MVP atau prototaip dan memerlukan sedikit usaha untuk menyediakannya pengeluaran. Satu atau lebih daripada amalan yang disenaraikan apabila digunakan boleh membantu aplikasi anda meningkat dengan cara yang berkesan. Artikel ini tidak merangkumi keseluruhan aspek kejuruteraan perisian pembangunan aplikasi tetapi hanya pada aplikasi pembalut LLM. Juga, coretan kod adalah dalam python dan logik yang sama boleh digunakan pada bahasa lain juga.
1. Manfaatkan Middleware untuk Fleksibiliti
Gunakan perisian tengah seperti LiteLLM atau LangChain untuk mengelakkan kunci masuk vendor dan beralih antara model dengan mudah semasa ia berkembang.
Python:
from litellm import completion response = completion( model="gpt-3.5-turbo", messages=[{"role": "user", "content": "Hello, how are you?"}] )
Penyelesaian perisian tengah seperti LiteLLM dan LangChain menyediakan lapisan abstraksi antara aplikasi anda dan pelbagai penyedia LLM. Abstraksi ini membolehkan anda bertukar dengan mudah antara model atau pembekal yang berbeza tanpa mengubah kod aplikasi teras anda. Apabila landskap AI berkembang pesat, model baharu kerap dikeluarkan dengan keupayaan yang dipertingkatkan. Dengan menggunakan perisian tengah, anda boleh menggunakan model baharu ini atau menukar penyedia dengan pantas berdasarkan prestasi, kos atau keperluan ciri, memastikan aplikasi anda kekal terkini dan berdaya saing.
2. Laksanakan Mekanisme Cuba Semula
Elakkan isu had kadar dengan melaksanakan logik cuba semula dalam panggilan API anda.
Python:
import time from openai import OpenAI client = OpenAI() def retry_api_call(max_retries=3, delay=1): for attempt in range(max_retries): try: response = client.chat.completions.create( model="gpt-3.5-turbo", messages=[{"role": "user", "content": "Hello!"}] ) return response except Exception as e: if attempt == max_retries - 1: raise e time.sleep(delay * (2 ** attempt)) # Exponential backoff
Pembekal LLM sering mengenakan had kadar untuk mengelakkan penyalahgunaan dan memastikan penggunaan yang adil. Melaksanakan mekanisme percubaan semula dengan pengunduran eksponen membantu aplikasi anda menangani kegagalan sementara atau ralat had kadar dengan anggun. Pendekatan ini meningkatkan kebolehpercayaan aplikasi anda dengan mencuba semula permintaan yang gagal secara automatik, mengurangkan kemungkinan gangguan perkhidmatan disebabkan isu sementara. Strategi penyingkiran eksponen (meningkatkan kelewatan antara percubaan semula) membantu menghalang API melampau dengan permintaan semula serta-merta, yang boleh memburukkan lagi isu pengehadan kadar.
3. Sediakan Pengunduran Penyedia LLM
Jangan bergantung pada satu pembekal LLM. Laksanakan sandaran untuk mengendalikan isu kuota atau gangguan perkhidmatan.
from litellm import completion def get_llm_response(prompt): providers = ['openai/gpt-3.5-turbo', 'anthropic/claude-2', 'cohere/command-nightly'] for provider in providers: try: response = completion(model=provider, messages=[{"role": "user", "content": prompt}]) return response except Exception as e: print(f"Error with {provider}: {str(e)}") continue raise Exception("All LLM providers failed")
Bergantung pada penyedia LLM tunggal boleh menyebabkan gangguan perkhidmatan jika penyedia tersebut mengalami masa henti atau jika anda mencapai had kuota. Dengan melaksanakan pilihan sandaran, anda memastikan operasi berterusan aplikasi anda. Pendekatan ini juga membolehkan anda memanfaatkan kekuatan pembekal atau model yang berbeza untuk pelbagai tugas. LiteLLM memudahkan proses ini dengan menyediakan antara muka bersatu untuk berbilang pembekal, menjadikannya lebih mudah untuk bertukar antara mereka atau melaksanakan logik sandaran.
4. Melaksanakan Kebolehmerhatian
Gunakan alatan seperti Langfuse atau Helicone untuk pengesanan dan penyahpepijatan LLM.
from langfuse.openai import OpenAI client = OpenAI( api_key="your-openai-api-key", langfuse_public_key="your-langfuse-public-key", langfuse_secret_key="your-langfuse-secret-key" ) response = client.chat.completions.create( model="gpt-3.5-turbo", messages=[{"role": "user", "content": "Hello, AI!"}] )
Kelebihan melaksanakan kebolehmerhatian:
- Penyahpepijatan dipertingkatkan: Kesan dan mainkan semula perbualan dengan mudah untuk mengenal pasti isu.
- Pengoptimuman prestasi: Dapatkan cerapan tentang masa tindak balas dan prestasi model.
- Pengurusan kos: Jejaki penggunaan token dan kos yang berkaitan untuk kawalan belanjawan yang lebih baik.
- Jaminan kualiti: Pantau kualiti respons dan kenal pasti bidang untuk penambahbaikan.
- Analisis pengalaman pengguna: Fahami interaksi pengguna dan optimumkan gesaan dengan sewajarnya.
- Pematuhan dan pengauditan: Kekalkan log untuk pematuhan kawal selia dan audit dalaman.
- Pengesanan anomali: Kenal pasti dan tindak balas dengan pantas kepada corak atau gelagat luar biasa.
Alat kebolehmerhatian memberikan cerapan penting tentang prestasi aplikasi LLM anda, corak penggunaan dan potensi isu. Ia membolehkan anda memantau dan menganalisis interaksi dengan LLM dalam masa nyata, membantu anda mengoptimumkan gesaan, mengenal pasti kesesakan dan memastikan kualiti respons yang dijana oleh AI. Tahap keterlihatan ini penting untuk mengekalkan, menyahpepijat dan menambah baik aplikasi anda dari semasa ke semasa.
5. Urus Gesaan dengan Berkesan
Gunakan alatan pengurusan segera dengan versi bukannya gesaan pengekodan keras dalam kod atau fail teks anda.
from promptflow import PromptFlow pf = PromptFlow() prompt_template = pf.get_prompt("greeting_prompt", version="1.2") response = openai.ChatCompletion.create( model="gpt-3.5-turbo", messages=[{"role": "user", "content": prompt_template.format(name="Alice")}] )
Pengurusan segera yang berkesan adalah penting untuk mengekalkan dan menambah baik aplikasi LLM anda. Dengan menggunakan alatan pengurusan segera yang khusus, anda boleh mengawal versi gesaan anda, A/B menguji variasi yang berbeza dan mengemas kininya dengan mudah merentas aplikasi anda. Pendekatan ini memisahkan logik segera daripada kod aplikasi, menjadikannya lebih mudah untuk mengulangi gesaan tanpa mengubah aplikasi teras. Ia juga membolehkan ahli pasukan bukan teknikal menyumbang kepada peningkatan segera dan membolehkan kerjasama yang lebih baik dalam memperhalusi interaksi AI.
6. Store Conversation History Persistently
Use a persistent cache like Redis for storing conversation history instead of in-memory cache which is not adapted for distributed systems.
from langchain.memory import RedisChatMessageHistory from langchain.chains import ConversationChain from langchain.llms import OpenAI # Initialize Redis chat message history message_history = RedisChatMessageHistory(url="redis://localhost:6379/0", ttl=600, session_id="user-123") # Create a conversation chain with Redis memory conversation = ConversationChain( llm=OpenAI(), memory=message_history, verbose=True ) # Use the conversation response = conversation.predict(input="Hi there!") print(response) # The conversation history is automatically stored in Redis
Storing conversation history is essential for maintaining context in ongoing interactions and providing personalized experiences. Using a persistent cache like Redis, especially in distributed systems, ensures that conversation history is reliably stored and quickly accessible. This approach allows your application to scale horizontally while maintaining consistent user experiences across different instances or servers. The use of Redis with LangChain simplifies the integration of persistent memory into your conversational AI system, making it easier to build stateful, context-aware applications.
7. Use JSON Mode whenever possible
Whenever possible like extracting structured information, provide a JSON schema instead of relying on raw text output.
import openai response = openai.ChatCompletion.create( model="gpt-3.5-turbo-1106", response_format={"type": "json_object"}, messages=[ {"role": "system", "content": "Extract the name and age from the user's input."}, {"role": "user", "content": "My name is John and I'm 30 years old."} ] ) print(response.choices[0].message.content) # Output: {"name": "John", "age": 30}
Using JSON mode for information extraction provides a structured and consistent output format, making it easier to parse and process the LLM's responses in your application. This approach reduces the need for complex post-processing of free-form text and minimizes the risk of misinterpretation. It's particularly useful for tasks like form filling, data extraction from unstructured text, or any scenario where you need to integrate AI-generated content into existing data structures or databases.
8. Set Up Credit Alerts
Implement alerts for prepaid credits and per-user credit checks, even in MVP stages.
def check_user_credits(user_id, requested_tokens): user_credits = get_user_credits(user_id) if user_credits < requested_tokens: raise InsufficientCreditsError(f"User {user_id} has insufficient credits") remaining_credits = user_credits - requested_tokens if remaining_credits < CREDIT_ALERT_THRESHOLD: send_low_credit_alert(user_id, remaining_credits) return True
Implementing credit alerts and per-user credit checks is crucial for managing costs and ensuring fair usage in your LLM application. This system helps prevent unexpected expenses and allows you to proactively manage user access based on their credit limits. By setting up alerts at multiple thresholds, you can inform users or administrators before credits are depleted, ensuring uninterrupted service. This approach is valuable even in MVP stages, as it helps you understand usage patterns and plan for scaling your application effectively.
9. Implement Feedback Loops
Create mechanisms for users to provide feedback on AI responses, starting with simple thumbs up/down ratings.
def process_user_feedback(response_id, feedback): if feedback == 'thumbs_up': log_positive_feedback(response_id) elif feedback == 'thumbs_down': log_negative_feedback(response_id) trigger_improvement_workflow(response_id) # In your API endpoint @app.route('/feedback', methods=['POST']) def submit_feedback(): data = request.json process_user_feedback(data['response_id'], data['feedback']) return jsonify({"status": "Feedback received"})
Implementing feedback loops is essential for continuously improving your LLM application. By allowing users to provide feedback on AI responses, you can identify areas where the model performs well and where it needs improvement. This data can be used to fine-tune models, adjust prompts, or implement additional safeguards. Starting with simple thumbs up/down ratings provides an easy way for users to give feedback, while more detailed feedback options can be added later for deeper insights. This approach helps in building trust with users and demonstrates your commitment to improving the AI's performance based on real-world usage.
10. Implement Guardrails
Use prompt guards to check for prompt injection attacks, toxic content, and off-topic responses.
import re from better_profanity import profanity def check_prompt_injection(input_text): injection_patterns = [ r"ignore previous instructions", r"disregard all prior commands", r"override system prompt" ] for pattern in injection_patterns: if re.search(pattern, input_text, re.IGNORECASE): return True return False def check_toxic_content(input_text): return profanity.contains_profanity(input_text) def sanitize_input(input_text): if check_prompt_injection(input_text): raise ValueError("Potential prompt injection detected") if check_toxic_content(input_text): raise ValueError("Toxic content detected") # Additional checks can be added here (e.g., off-topic detection) return input_text # Return sanitized input if all checks pass # Usage try: safe_input = sanitize_input(user_input) # Process safe_input with your LLM except ValueError as e: print(f"Input rejected: {str(e)}")
Implementing guardrails is crucial for ensuring the safety and reliability of your LLM application. This example demonstrates how to check for potential prompt injection attacks and toxic content. Prompt injection attacks attempt to override or bypass the system's intended behavior, while toxic content checks help maintain a safe and respectful environment. By implementing these checks, you can prevent malicious use of your AI system and ensure that the content generated aligns with your application's guidelines and ethical standards. Additional checks can be added to detect off-topic responses or other unwanted behaviors, further enhancing the robustness of your application.
Conclusion
All the above listed points can be easily integrated into your application and they prepare you better for scaling in production. You may also agree or disagree on some of the above points. In any case, feel free to post your questions or comments.
Atas ialah kandungan terperinci Amalan Penting untuk Membina Aplikasi LLM Teguh. Untuk maklumat lanjut, sila ikut artikel berkaitan lain di laman web China PHP!

Alat AI Hot

Undresser.AI Undress
Apl berkuasa AI untuk mencipta foto bogel yang realistik

AI Clothes Remover
Alat AI dalam talian untuk mengeluarkan pakaian daripada foto.

Undress AI Tool
Gambar buka pakaian secara percuma

Clothoff.io
Penyingkiran pakaian AI

Video Face Swap
Tukar muka dalam mana-mana video dengan mudah menggunakan alat tukar muka AI percuma kami!

Artikel Panas

Alat panas

Notepad++7.3.1
Editor kod yang mudah digunakan dan percuma

SublimeText3 versi Cina
Versi Cina, sangat mudah digunakan

Hantar Studio 13.0.1
Persekitaran pembangunan bersepadu PHP yang berkuasa

Dreamweaver CS6
Alat pembangunan web visual

SublimeText3 versi Mac
Perisian penyuntingan kod peringkat Tuhan (SublimeText3)

Topik panas










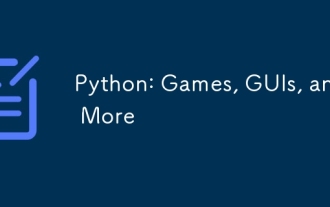
Python cemerlang dalam permainan dan pembangunan GUI. 1) Pembangunan permainan menggunakan pygame, menyediakan lukisan, audio dan fungsi lain, yang sesuai untuk membuat permainan 2D. 2) Pembangunan GUI boleh memilih tkinter atau pyqt. TKInter adalah mudah dan mudah digunakan, PYQT mempunyai fungsi yang kaya dan sesuai untuk pembangunan profesional.
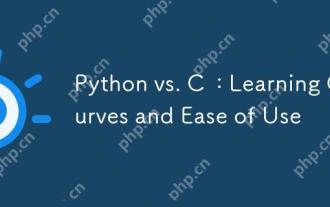
Python lebih mudah dipelajari dan digunakan, manakala C lebih kuat tetapi kompleks. 1. Sintaks Python adalah ringkas dan sesuai untuk pemula. Penaipan dinamik dan pengurusan memori automatik menjadikannya mudah digunakan, tetapi boleh menyebabkan kesilapan runtime. 2.C menyediakan kawalan peringkat rendah dan ciri-ciri canggih, sesuai untuk aplikasi berprestasi tinggi, tetapi mempunyai ambang pembelajaran yang tinggi dan memerlukan memori manual dan pengurusan keselamatan jenis.
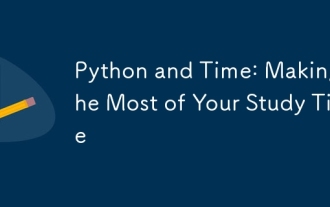
Untuk memaksimumkan kecekapan pembelajaran Python dalam masa yang terhad, anda boleh menggunakan modul, masa, dan modul Python. 1. Modul DateTime digunakan untuk merakam dan merancang masa pembelajaran. 2. Modul Masa membantu menetapkan kajian dan masa rehat. 3. Modul Jadual secara automatik mengatur tugas pembelajaran mingguan.
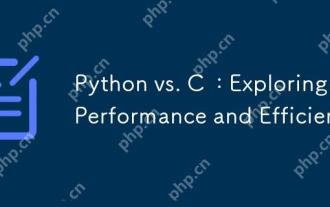
Python lebih baik daripada C dalam kecekapan pembangunan, tetapi C lebih tinggi dalam prestasi pelaksanaan. 1. Sintaks ringkas Python dan perpustakaan yang kaya meningkatkan kecekapan pembangunan. 2. Ciri-ciri jenis kompilasi dan kawalan perkakasan meningkatkan prestasi pelaksanaan. Apabila membuat pilihan, anda perlu menimbang kelajuan pembangunan dan kecekapan pelaksanaan berdasarkan keperluan projek.
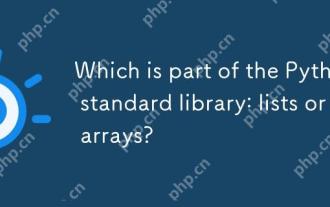
Pythonlistsarepartofthestandardlibrary, sementara
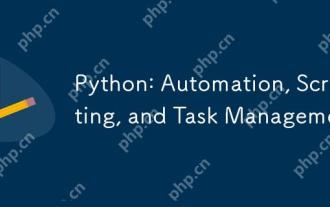
Python cemerlang dalam automasi, skrip, dan pengurusan tugas. 1) Automasi: Sandaran fail direalisasikan melalui perpustakaan standard seperti OS dan Shutil. 2) Penulisan Skrip: Gunakan Perpustakaan Psutil untuk memantau sumber sistem. 3) Pengurusan Tugas: Gunakan perpustakaan jadual untuk menjadualkan tugas. Kemudahan penggunaan Python dan sokongan perpustakaan yang kaya menjadikannya alat pilihan di kawasan ini.
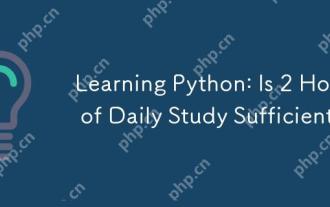
Adakah cukup untuk belajar Python selama dua jam sehari? Ia bergantung pada matlamat dan kaedah pembelajaran anda. 1) Membangunkan pelan pembelajaran yang jelas, 2) Pilih sumber dan kaedah pembelajaran yang sesuai, 3) mengamalkan dan mengkaji semula dan menyatukan amalan tangan dan mengkaji semula dan menyatukan, dan anda secara beransur-ansur boleh menguasai pengetahuan asas dan fungsi lanjutan Python dalam tempoh ini.
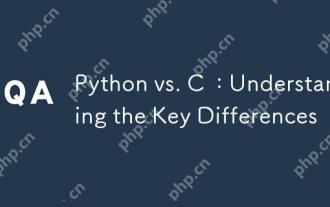
Python dan C masing -masing mempunyai kelebihan sendiri, dan pilihannya harus berdasarkan keperluan projek. 1) Python sesuai untuk pembangunan pesat dan pemprosesan data kerana sintaks ringkas dan menaip dinamik. 2) C sesuai untuk prestasi tinggi dan pengaturcaraan sistem kerana menaip statik dan pengurusan memori manual.
