RLHF와 RL을 같은 카테고리로 분류할 수 있는지에 대해서는 여전히 사람마다 의견이 다른 것 같습니다. AI 전문가 Karpathy가 인공지능의 개념을 대중화하기 위해 다시 왔습니다. 어제 그는 "인간 피드백 기반 강화 학습(RLHF)은 강화 학습(RL)일 뿐이다"라고 트윗했습니다. 세 가지(마지막) 주요 단계가 있으며, 처음 두 단계는 사전 훈련 및 감독 미세 조정(SFT)입니다. 내 생각에 RLHF는 간신히 RL일 뿐이고 널리 인식되지는 않습니다. RL은 강력하지만 RLHF는 그렇지 않습니다.
실제 RL을 사용해 학습한 AlphaGo의 예를 살펴보겠습니다. 컴퓨터는 바둑 게임을 하며 보상 기능(게임의 승리)을 극대화하는 라운드에서 훈련을 받았고, 결국 최고의 인간 플레이어를 능가했습니다. AlphaGo는 RLHF를 사용하여 훈련하지 않았으며, 있었다면 그다지 효과적이지 않았을 것입니다.
RLHF로 AlphaGo를 훈련시키면 어떤 모습일까요? 먼저 인간 주석자에게 두 가지 바둑 상태를 제공하고 어느 것을 선호하는지 물어봅니다.
그런 다음 100,000개의 유사한 비교를 수집하고 "보상 모델"(RM) 신경망을 훈련하여 보드 상태에 대한 인간의 느낌 검사를 시뮬레이션합니다. 평균적인 인간 판단에 동의하도록 훈련시킵니다. 보너스 모델 느낌 확인이 끝나면 이에 대해 RL을 실행하고 좋은 느낌을 가져오는 동작을 만드는 방법을 배울 수 있습니다. 분명히 이것은 Go에서 그다지 흥미로운 결과를 생성하지 않습니다.
이는 주로 두 가지 근본적이고 독립적인 이유 때문입니다.
1) 분위기가 오해를 불러일으킬 수 있으며 이는 실제 보상(게임 승리)이 아닙니다. 이는 좋지 않은 에이전트 목표입니다. 더 나쁜 것은 2) 보드 상태가 보상 모델과 반대라는 것을 빠르게 발견하기 때문에 RL 최적화가 궤도를 벗어나는 것을 발견하게 될 것입니다. 보상 모델은 대기를 시뮬레이션하기 위해 수십억 개의 매개변수를 사용하는 대규모 신경망이라는 점을 기억하십시오. 일부 보드 상태는 자체 훈련 데이터의 분포 범위를 벗어나 실제로 좋은 상태는 아니지만 보상 모델로부터 매우 높은 보상을 받습니다.
같은 이유로 RLHF 작업이 LLM에 적합하다는 사실에 가끔 놀랐습니다. 우리가 LLM을 위해 훈련한 보상 모델은 정확히 동일한 방식으로 분위기 확인을 수행하여 인간 평가자가 통계적으로 좋아하는 보조 응답에 높은 점수를 부여합니다. 이는 문제를 올바르게 해결한다는 실제 목표가 아니라, 인간이 행위자로서 선하다고 생각하는 것이 목표이다.
두 번째로, 게임이 모델에 보상하는 방식으로 모델이 반응하는 방법을 빠르게 학습하기 때문에 RLHF를 오랫동안 실행할 수도 없습니다. 이러한 예측은 정말 이상해 보일 것이며, LLM 어시스턴트가 "The the the the the the the the the the the the the the the the the the the the the the the the the the the the the the the the the the the the the the the the the the the the the the the the the the the the the the the the the the the the the the the the the the the the the the the the the the the the the the the the the the the the the the the the the the the the the the the the the the the the the the the the the the the the the the the the the the the the the the the the the the the the the the the the the the the the the the the the the the the the the the the the the the the the the the the the the the the the the the the the the the the the the the the the the the the the the the the the the the the the the the the the the the the the the the the the the the the the the the the the the the the the the the the the the the the the the the the the the the the the the the the the the the the the the the the the the the the the the the the the the the the the the the the the the the the the the the the the the the the the the the the the the the the the the the the the the the the the the the the the the the the the the the the the the the the the the.. 이것은 당신에게 우스꽝스러워 보이지만 보너스 모델 분위기 검사를 보고 어떤 이유로 보너스 모델이 이것이 훌륭해 보인다고 생각한다는 것을 깨닫게 됩니다.
LLM이 보상 모델 훈련 데이터 범위를 벗어나고 정의되지 않은 범위에 있는 적대적인 예를 발견했습니다. 이러한 특정 예제를 훈련 세트에 반복적으로 추가하여 이를 완화할 수 있지만, 다음번에는 여전히 다른 적대적 예제를 찾을 수 있습니다. 많은 최적화 단계에서는 RLHF를 실행할 수도 없습니다. 최적화가 보상 모델 게임을 시작하기 때문에 수백 또는 수천 단계 후에 호출해야 합니다. 이것은 AlphaGo와 같은 RL이 아닙니다.
그러나 RLHF는 LLM 보조자를 구축하는 데 매우 유용한 단계입니다. 여기에는 몇 가지 미묘한 이유가 있다고 생각합니다. 그 중 제가 가장 좋아하는 것은 RLHF를 사용하면 LLM 보조자가 생성기-판별기 격차로부터 이점을 얻을 수 있다는 것입니다. 즉, 많은 질문 유형의 경우 인간 주석자가 처음부터 이상적인 답변을 작성하는 것보다 여러 후보 답변 중에서 최상의 답변을 선택하는 것이 훨씬 쉽습니다. 좋은 예는 "종이클립 시 생성"과 같은 프롬프트입니다. 일반적인 인간 주석자는 감독된 미세 조정 예제로 사용하기 위해 처음부터 좋은 시를 작성하는 데 어려움을 겪지만 여러 후보 답변(시)이 주어지면 더 나은 시를 선택할 수 있습니다. 따라서 RLHF는 인간 감독의 "편리한" 격차로부터 이익을 얻을 수 있는 방법입니다.
RLHF가 환각 완화에 도움이 되는 다른 이유가 있습니다. 보상 모델이 훈련 중에 LLM이 구성하는 내용을 파악할 수 있을 만큼 강력한 모델인 경우 낮은 보상으로 이러한 행동을 처벌하는 방법을 학습하고 불확실할 때 사실적 지식을 얻기 위해 위험을 감수하지 않도록 모델을 가르칠 수 있습니다. 그러나 환각을 만족스럽게 완화하고 관리하는 것은 또 다른 문제이므로 여기서는 더 자세히 설명하지 않겠습니다. 결론적으로 RLHF는 작동하지만 RL은 아닙니다.
지금까지 LLM을 위한 프로덕션 등급 RL은 공개 도메인에서 설득력 있게 구현되고 대규모로 시연된 적이 없습니다. 직관적으로 이는 개방형 문제 해결 작업에서 실제 보상(즉, 게임 승리)을 얻는 것이 매우 어렵기 때문입니다. 바둑처럼 폐쇄적이고 게임 같은 환경에서는 모든 것이 재미있습니다. 역학이 제한되어 있고 보상 기능 평가 비용이 매우 낮으며 게임이 불가능합니다.
그런데 기사를 요약하면 어떻게 객관적인 보상을 주나요? 아니면 특정 pip 설치에 대한 모호한 질문에 답하시겠습니까? 아니면 농담을 할까요? 아니면 일부 Java 코드를 Python으로 다시 작성하시겠습니까? 이를 달성하는 것은 원칙적으로 불가능하지는 않지만 쉽지 않으며 창의적인 사고가 필요합니다. 이 문제를 설득력 있게 해결하는 사람은 누구나 실제 RL을 실행할 수 있게 되어 AlphaGo가 바둑에서 인간을 이길 수 있게 됩니다. RL을 통해 LLM은 개방형 도메인 문제를 해결하는 데 있어서 인간을 능가할 수 있는 잠재력을 가지고 있습니다.
Karpathy의 요점은 RLHF와 RL의 더 많은 차이점을 지적한 일부 사람들에 의해 반향되었습니다. 예를 들어, RLHF는 적절한 검색을 수행하지 않으며 사전 훈련된 궤적의 하위 집합을 활용하는 방법을 주로 학습합니다. 대조적으로, 적절한 RL을 수행할 때, 손실 함수에 엔트로피 항을 추가하여 이산 동작 분포에 잡음이 발생하는 경우가 많습니다. Kaypathy는 원칙적으로 RL에서도 종종 수행되는 RLHF 목표에 엔트로피 보상을 쉽게 추가할 수 있다고 주장했습니다. 하지만 실제로는 그런 경우가 거의 없는 것 같습니다.
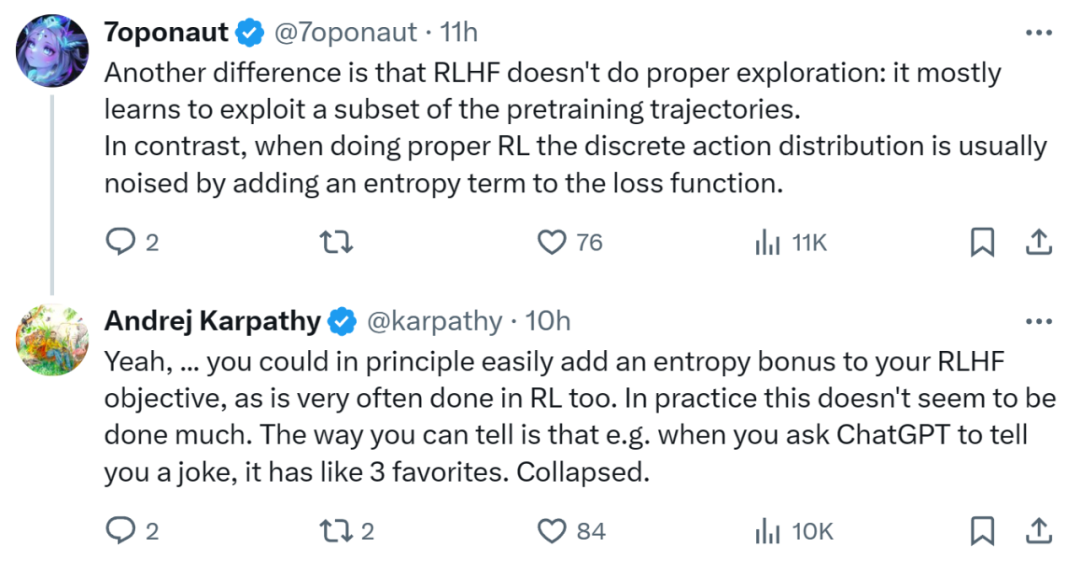
Google 연구 과학자 Kevin Patrick Murphy도 Karpathy의 의견에 전적으로 동의합니다.
- Beliau percaya bahawa RLHF lebih seperti "penyamun" konteks dengan operasi nilai rentetan, di mana prompt adalah konteks, jadi ia tidak boleh dipanggil RL lengkap.
- Juga memformalkan ganjaran untuk tugas harian adalah bahagian yang sukar (dia fikir ia mungkin dipanggil penjajaran).
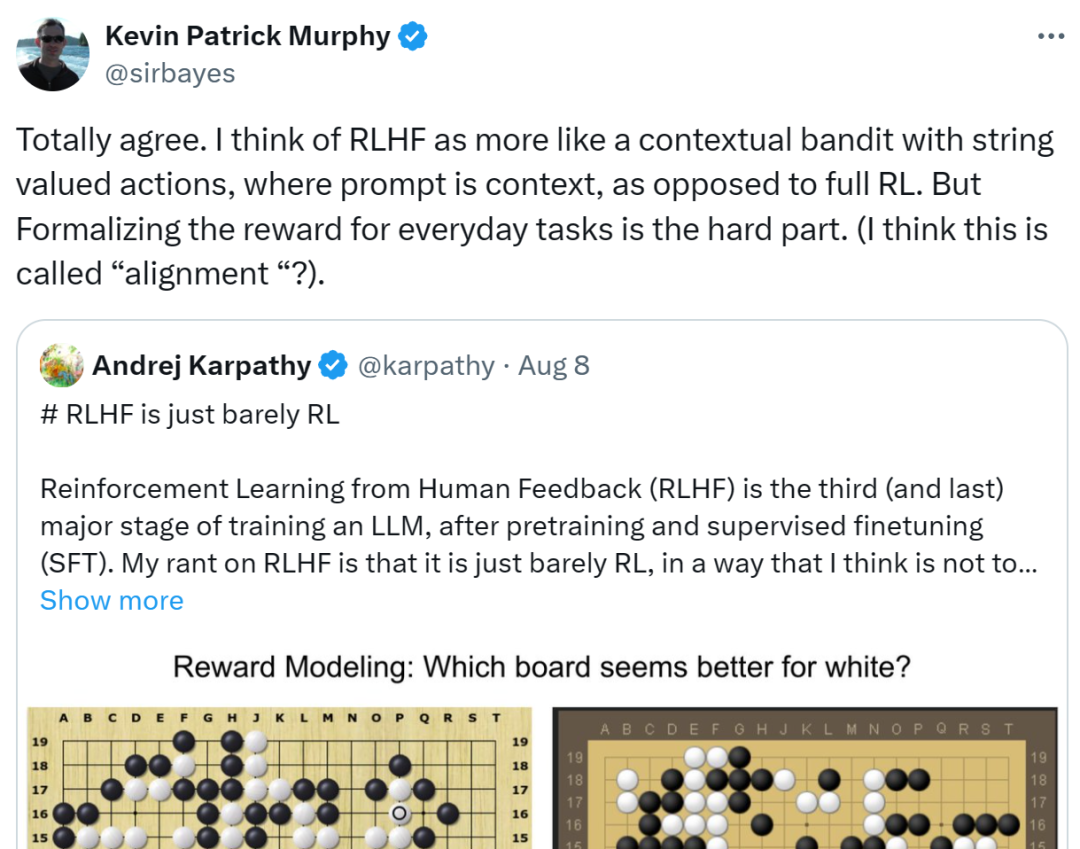
Walau bagaimanapun, Natasha Jaques, seorang lagi saintis penyelidikan kanan di Google, berpendapat Karpathy salah. Dia percaya apabila ejen berinteraksi dengan orang ramai, memberi jawapan yang disukai manusia adalah matlamat sebenar.
Julat di luar pengedaran bukan satu masalah khusus untuk RLHF. Hanya kerana maklum balas manusia lebih terhad daripada menjalankan simulasi Go yang tidak terhingga tidak bermakna ia bukan masalah yang patut diselesaikan, ia hanya menjadikannya lebih mencabar. Dia berharap ini menjadi isu yang lebih berkesan, selepas semua mengurangkan berat sebelah dalam LLM lebih masuk akal daripada mengalahkan manusia di Go. Menggunakan istilah yang menghina seperti Karpathy yang mengatakan model bonus adalah cek getaran adalah bodoh. Anda boleh menggunakan hujah yang sama terhadap anggaran nilai.
Dia merasakan bahawa pandangan Karpathy hanya akan menghalang orang ramai daripada meneruskan kerja RLHF, sedangkan pada masa ini ia merupakan satu-satunya cara yang berdaya maju untuk mengurangkan bahaya serius yang boleh ditimbulkan oleh bias dan ilusi LLM.
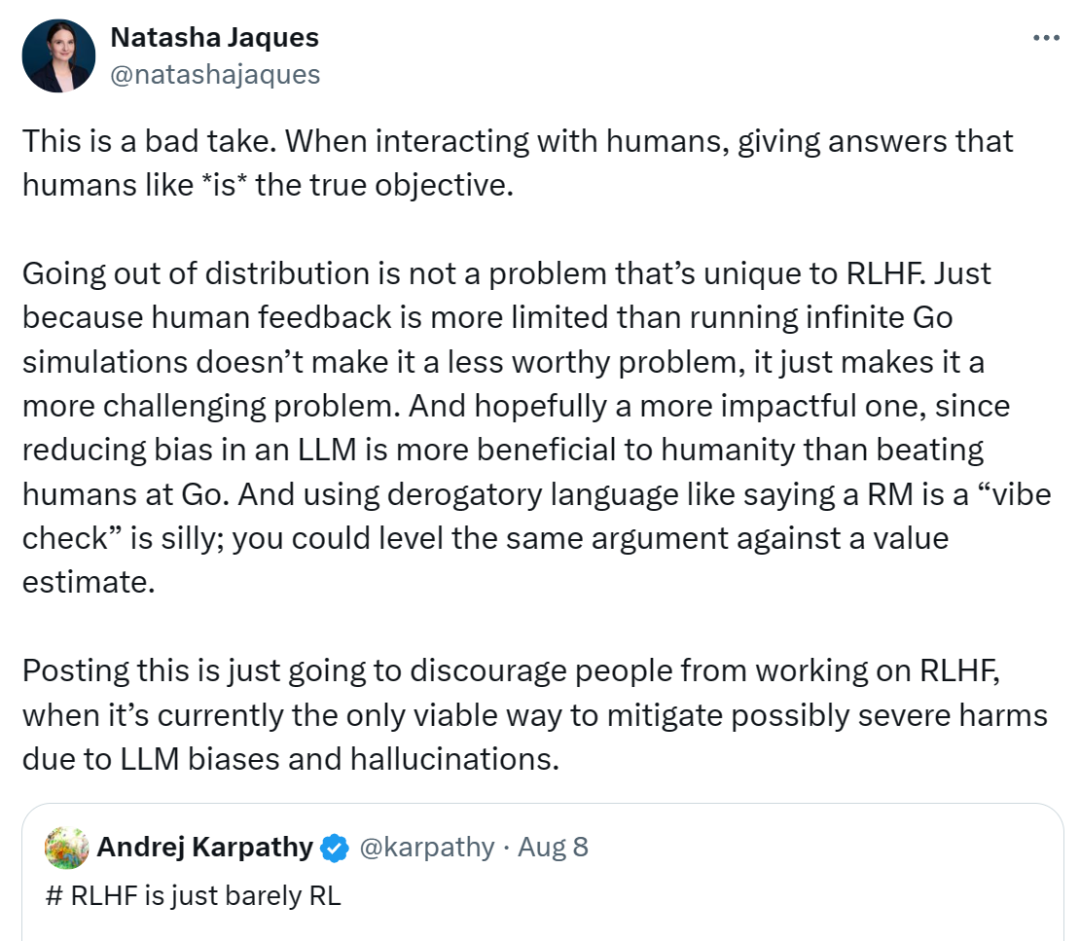
Sumber: https://x.com/natashajaques/status/1821631137590259979
meta penyelidik Pierluca d'Oro tidak bersetuju dengan titik utama Karpathy, tetapi bersetuju bahawa "RLHF hanya hampir rl" Tajuk ini. Beliau berhujah bahawa RLHF, yang biasanya digunakan untuk menyempurnakan LLM, bukanlah RL.
Isi utama adalah seperti berikut:
- Dalam pembelajaran pengukuhan, adalah tidak realistik untuk meneruskan konsep "ganjaran sempurna", kerana dalam kebanyakan tugas yang kompleks, sebagai tambahan kepada kepentingan matlamat, kaedah pelaksanaan adalah sama penting.
- Walaupun dalam tugasan dengan peraturan yang jelas seperti Go, RL berfungsi dengan baik. Tetapi apabila bercakap tentang tingkah laku yang kompleks, mekanisme ganjaran RL tradisional mungkin tidak dapat memenuhi keperluan.
- Beliau menganjurkan untuk mengkaji cara meningkatkan prestasi RL di bawah model ganjaran yang tidak sempurna, dan menekankan kepentingan gelung maklum balas, mekanisme RL yang mantap dan kerjasama manusia-mesin.
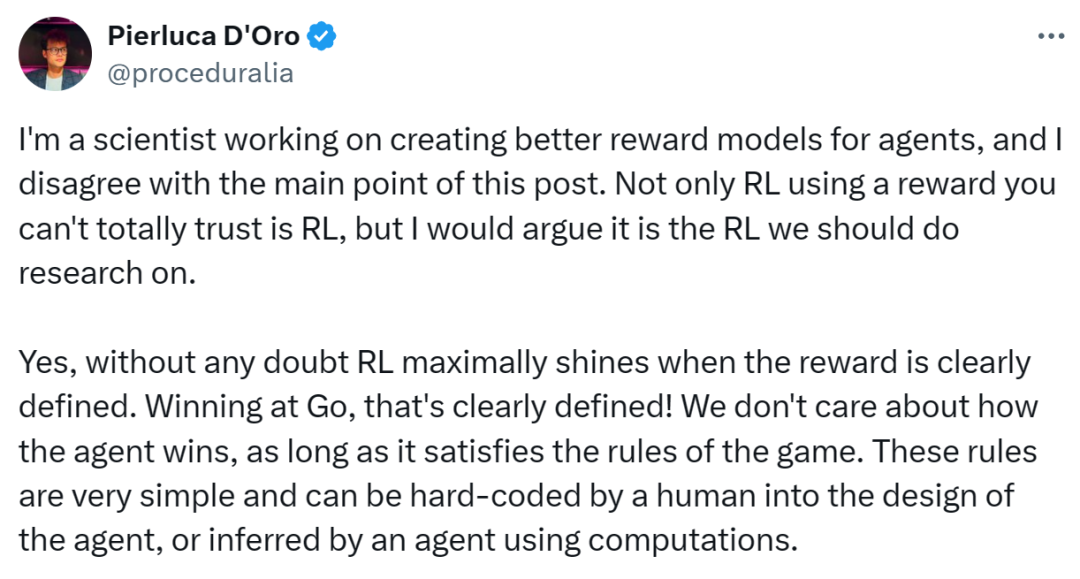
… ceduralia/status/1821560990091128943 Pandangan siapa yang anda setuju? Selamat tinggalkan mesej di ruangan komen.
Atas ialah kandungan terperinci Pandangan Karpathy adalah kontroversi: RLHF bukanlah pembelajaran pengukuhan sebenar, dan Google dan Meta menentangnya. Untuk maklumat lanjut, sila ikut artikel berkaitan lain di laman web China PHP!