


Meringkaskan Teks Menggunakan Model BART Memeluk Muka
Dalam dunia yang serba pantas hari ini, memekatkan kandungan bentuk panjang kepada ringkasan ringkas adalah penting, sama ada untuk mengimbas artikel dengan pantas atau menyerlahkan perkara utama dalam kertas penyelidikan. Memeluk Wajah menawarkan alat yang berkuasa untuk ringkasan teks: model BART. Dalam artikel ini, kami akan meneroka cara anda boleh memanfaatkan model terlatih Hugging Face, khususnya model facebook/bart-large-cnn, untuk meringkaskan artikel dan teks yang panjang.
Bermula dengan Model BART Memeluk Wajah
Memeluk Wajah menyediakan pelbagai model untuk tugasan NLP seperti klasifikasi teks, terjemahan dan ringkasan. Salah satu model ringkasan yang paling popular ialah BART (Pengubah Dua Arah dan Auto-Regresif), yang dilatih untuk menjana ringkasan yang koheren daripada dokumen besar.
Langkah 1: Pasang Perpustakaan Transformers Wajah Memeluk
Untuk bermula dengan model Hugging Face, anda perlu memasang pustaka transformer. Anda boleh melakukan ini menggunakan pip:
pip install transformers
Langkah 2: Mengimport Talian Paip Summarization
Setelah pustaka dipasang, anda boleh memuatkan model pra-latihan untuk ringkasan dengan mudah. API saluran paip Hugging Face menyediakan antara muka peringkat tinggi untuk menggunakan model seperti facebook/bart-large-cnn, yang telah diperhalusi untuk tugasan ringkasan.
from transformers import pipeline # Load the summarization model summarizer = pipeline("summarization", model="facebook/bart-large-cnn")
Langkah 3: Menjalankan Summarizer
Sekarang anda telah menyediakan ringkasan, anda boleh suapan dalam mana-mana teks panjang untuk menjana ringkasan. Di bawah ialah contoh menggunakan contoh artikel tentang Dame Maggie Smith, seorang pelakon terkenal Britain.
ARTICLE = """ Dame Margaret Natalie Smith (28 December 1934 – 27 September 2024) was a British actress. Known for her wit in both comedic and dramatic roles, she had an extensive career on stage and screen for over seven decades and was one of Britain's most recognisable and prolific actresses. She received numerous accolades, including two Academy Awards, five BAFTA Awards, four Emmy Awards, three Golden Globe Awards and a Tony Award, as well as nominations for six Olivier Awards. Smith is one of the few performers to earn the Triple Crown of Acting. Smith began her stage career as a student, performing at the Oxford Playhouse in 1952, and made her professional debut on Broadway in New Faces of '56. Over the following decades Smith established herself alongside Judi Dench as one of the most significant British theatre performers, working for the National Theatre and the Royal Shakespeare Company. On Broadway, she received the Tony Award for Best Actress in a Play for Lettice and Lovage (1990). She was Tony-nominated for Noël Coward's Private Lives (1975) and Tom Stoppard's Night and Day (1979). Smith won Academy Awards for Best Actress for The Prime of Miss Jean Brodie (1969) and Best Supporting Actress for California Suite (1978). She was Oscar-nominated for Othello (1965), Travels with My Aunt (1972), A Room with a View (1985) and Gosford Park (2001). She portrayed Professor Minerva McGonagall in the Harry Potter film series (2001–2011). She also acted in Death on the Nile (1978), Hook (1991), Sister Act (1992), The Secret Garden (1993), The Best Exotic Marigold Hotel (2012), Quartet (2012) and The Lady in the Van (2015). Smith received newfound attention and international fame for her role as Violet Crawley in the British period drama Downton Abbey (2010–2015). The role earned her three Primetime Emmy Awards; she had previously won one for the HBO film My House in Umbria (2003). Over the course of her career she was the recipient of numerous honorary awards, including the British Film Institute Fellowship in 1993, the BAFTA Fellowship in 1996 and the Society of London Theatre Special Award in 2010. Smith was made a dame by Queen Elizabeth II in 1990. """ # Generate the summary summary = summarizer(ARTICLE, max_length=130, min_length=30, do_sample=False) # Print the summary print(summary)
Output:
[{'summary_text': 'Dame Margaret Natalie Smith (28 December 1934 – 27 September 2024) was a British actress. Known for her wit in both comedic and dramatic roles, she had an extensive career on stage and screen for over seven decades. She received numerous accolades, including two Academy Awards, five BAFTA Awards, four Emmy Awards, three Golden Globe Awards and a Tony Award.'}]
Seperti yang anda boleh lihat daripada output, ringkasan meringkaskan perkara utama artikel ke dalam format yang ringkas dan boleh dibaca, menyerlahkan fakta penting seperti umur panjang kerjaya dan pujiannya.
Satu lagi Pendekatan: Merumuskan Teks daripada Fail
Dalam sesetengah kes penggunaan, anda mungkin mahu membaca teks daripada fail dan bukannya rentetan kod keras. Di bawah ialah skrip Python yang dikemas kini yang membaca artikel daripada fail teks dan menjana ringkasan.
from transformers import pipeline # Load the summarizer pipeline summarizer = pipeline("summarization", model="facebook/bart-large-cnn") # Function to read the article from a text file def read_article_from_file(file_path): with open(file_path, 'r') as file: return file.read() # Path to the text file containing the article file_path = 'article.txt' # Change this to your file path # Read the article from the file ARTICLE = read_article_from_file(file_path) # Get the summary summary = summarizer(ARTICLE, max_length=130, min_length=30, do_sample=False) # Print the summary print(summary)
Input Fail:
Dalam kes ini, anda perlu menyimpan artikel ke fail teks (article.txt dalam contoh), dan skrip akan membaca kandungan dan meringkaskannya.
Kesimpulan
Model BART Memeluk Wajah ialah alat yang hebat untuk ringkasan teks automatik. Sama ada anda sedang memproses artikel panjang, kertas penyelidikan atau mana-mana badan teks yang besar, model ini boleh membantu anda menyaring maklumat menjadi ringkasan yang ringkas.
Artikel ini menunjukkan cara anda boleh menyepadukan model ringkasan pra-latihan Hugging Face ke dalam projek anda, kedua-duanya dengan teks berkod keras dan input fail. Dengan hanya beberapa baris kod, anda boleh menyediakan saluran ringkasan yang cekap dan berjalan dalam projek Python anda.
Atas ialah kandungan terperinci Meringkaskan Teks Menggunakan Model BART Memeluk Muka. Untuk maklumat lanjut, sila ikut artikel berkaitan lain di laman web China PHP!

Alat AI Hot

Undresser.AI Undress
Apl berkuasa AI untuk mencipta foto bogel yang realistik

AI Clothes Remover
Alat AI dalam talian untuk mengeluarkan pakaian daripada foto.

Undress AI Tool
Gambar buka pakaian secara percuma

Clothoff.io
Penyingkiran pakaian AI

Video Face Swap
Tukar muka dalam mana-mana video dengan mudah menggunakan alat tukar muka AI percuma kami!

Artikel Panas

Alat panas

Notepad++7.3.1
Editor kod yang mudah digunakan dan percuma

SublimeText3 versi Cina
Versi Cina, sangat mudah digunakan

Hantar Studio 13.0.1
Persekitaran pembangunan bersepadu PHP yang berkuasa

Dreamweaver CS6
Alat pembangunan web visual

SublimeText3 versi Mac
Perisian penyuntingan kod peringkat Tuhan (SublimeText3)

Topik panas
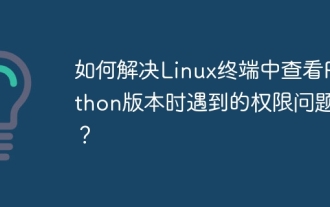
Penyelesaian kepada Isu Kebenaran Semasa Melihat Versi Python di Terminal Linux Apabila anda cuba melihat versi Python di Terminal Linux, masukkan Python ...
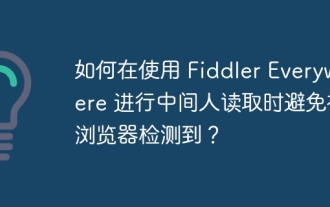
Cara mengelakkan dikesan semasa menggunakan fiddlerevery di mana untuk bacaan lelaki-dalam-pertengahan apabila anda menggunakan fiddlerevery di mana ...
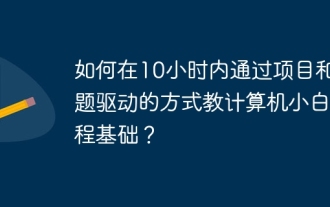
Bagaimana Mengajar Asas Pengaturcaraan Pemula Komputer Dalam masa 10 jam? Sekiranya anda hanya mempunyai 10 jam untuk mengajar pemula komputer beberapa pengetahuan pengaturcaraan, apa yang akan anda pilih untuk mengajar ...
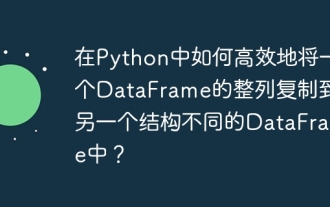
Apabila menggunakan Perpustakaan Pandas Python, bagaimana untuk menyalin seluruh lajur antara dua data data dengan struktur yang berbeza adalah masalah biasa. Katakan kita mempunyai dua DAT ...
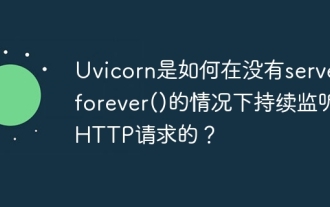
Bagaimanakah Uvicorn terus mendengar permintaan HTTP? Uvicorn adalah pelayan web ringan berdasarkan ASGI. Salah satu fungsi terasnya ialah mendengar permintaan HTTP dan teruskan ...
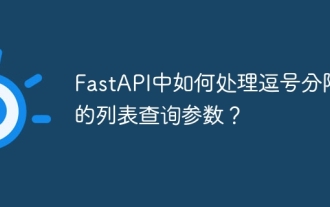
Fastapi ...
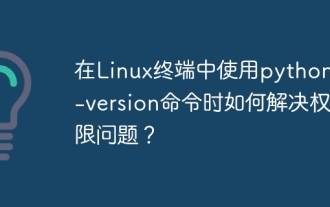
Menggunakan Python di Terminal Linux ...
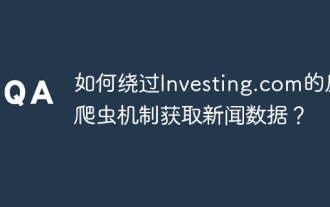
Memahami Strategi Anti-Crawling of Investing.com Ramai orang sering cuba merangkak data berita dari Investing.com (https://cn.investing.com/news/latest-news) ...
