


How Freshdesk Scaled Its Technology (Part I) – Before Shard_MySQL
[Edit Notes: Three year old startup Freshdesk built out of Chennai, is now clocking 70 million app views per week. The company is growing fast. In this post, its operations head Kiran talks about how they scaled the technology backend.]
Every startup’s fondest dream is to somehow grow exponentially but still stay nimble and super efficient. However that’s easier said than done. The 32GB RAM that is more than capable of handling the load today is going to look like a joke a week later. And with the financial freedom of a startup, you can only take one step at a time.
At Freshdesk, our customer base grew by 400 percent in the last year. And the number of requests boomed from 2 million to 65 million.
These are really cool numbers for a 3-year-old startup but from an engineering perspective, it’s closer to nightmare than dream come true. We scaled left right and center (but mostly upwards) in a really short amount of time, using a whole bunch of vertical techniques. Sure, we eventually had to shard our databases just to keep up, but some of these techniques helped us stay afloat, for quite a while.
Moore’s way
We tried to scale in the most straightforward way there is, by increasing the RAM, CPU and I/O. We travelled from Medium Instance Amazon EC2 First Generation to High Memory Quadruple Extra Large. It effectively increased our RAM from 3.75 GB to 64 GB. Then we figured that the amount of RAM we add and the CPU cycles do not correlate with the workload we get out of the instance. So we stayed put at 64GB.
The Read/write split
Since Freshdesk is a heavy read application (4:1; end user portals, APIs and loads of third party integrations tend to do that to you), we used MYSQL replication and distributed the reads between master and slave to accommodate them. Initially, we had different slaves getting selected for different queries using a round robin algorithm, but that quickly proved ineffective as we had no control over which query hit which DB. We worked around this by marking dedicated roles for each slave. For example, we used a slave for background processing jobs and another for report generation and so on (Seamless Database Pool, a Rails plugin, should do the job but if you’re an Engineyard user, I’d suggest you check out this cookbook).
As expected, the R/W split increased the number of I/Os we performed on our DBs but it didn’t do much good for the number of writes per second.
MySQL Partitioning
MySQL 5 has a built-in partitioning capability so all you have to do is, just choose the partition key and the number of partitions and the table will be partitioned, for you, automatically. However, if you’re thinking about going for MySQL partitioning, here are a couple of things you should keep in mind:
1. You need to choose the partition key carefully or alter the current schema to follow the MySQL partition rules.
2. The number of partitions you start with will affect the I/O operations on the disk directly.
3. If you use a hash-based algorithm with hash-based keys, you cannot control who goes where. This means you’ll be in trouble if two or more noisy customers fall within the same partition.
4. You need to make sure that every query contains the MySQL partition key. A query without the partition key ends up scanning all the partitions. Performance takes a dive as expected.
Post-partitioning, our read performance increased dramatically but, as expected, our number of writes didn’t increase much.
Caching
Some objects like support agent details change only 3-4 times in their lifetime. So, we started caching ActiveRecord objects and as well as html partials (bits and pieces of HTML) using Memcached. We chose Memcached because it scales well with multiple clusters. The Memcached client you use actually makes a lot of difference in the response time so we ended up going with dalli.
Distributed functions
Another way we try to keep the response time low is by using different storage engines for different purposes. For example, we use Amazon RedShift for analytics and data mining and Redis, to store state information and background jobs for Resque. But because Redis can’t scale or fallback, we don’t use it for atomic operations.
Scaling vertically can only get you so far. Even as we tried various techniques, we knew it was only a matter of time before we scaled horizontally. And the rate at which we were growing didn’t give us much time to ponder over whether it was a good decision or not. So before our app response times could sky rocket and the status quo changed, we sharded our databases. But that story’s for another day.
Further reading
About MySQL partitioning
Scaling of Basecamp
Mr.Moore gets to punt on Sharding
[About the Author:Kiran is the Director of Operations atFreshdesk. He calls himself the guy you should be mad at when the application is down. Reproduced with permission fromFreshdesk blog.]

Alat AI Hot

Undresser.AI Undress
Apl berkuasa AI untuk mencipta foto bogel yang realistik

AI Clothes Remover
Alat AI dalam talian untuk mengeluarkan pakaian daripada foto.

Undress AI Tool
Gambar buka pakaian secara percuma

Clothoff.io
Penyingkiran pakaian AI

AI Hentai Generator
Menjana ai hentai secara percuma.

Artikel Panas

Alat panas

Notepad++7.3.1
Editor kod yang mudah digunakan dan percuma

SublimeText3 versi Cina
Versi Cina, sangat mudah digunakan

Hantar Studio 13.0.1
Persekitaran pembangunan bersepadu PHP yang berkuasa

Dreamweaver CS6
Alat pembangunan web visual

SublimeText3 versi Mac
Perisian penyuntingan kod peringkat Tuhan (SublimeText3)

Topik panas


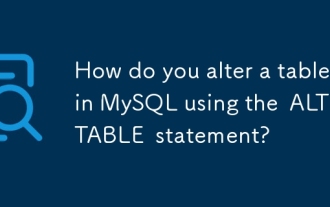
Artikel ini membincangkan menggunakan pernyataan jadual Alter MySQL untuk mengubah suai jadual, termasuk menambah/menjatuhkan lajur, menamakan semula jadual/lajur, dan menukar jenis data lajur.
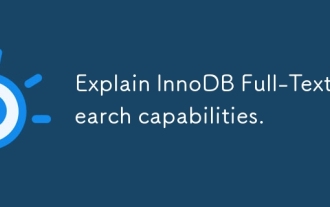
Keupayaan carian teks penuh InnoDB sangat kuat, yang dapat meningkatkan kecekapan pertanyaan pangkalan data dan keupayaan untuk memproses sejumlah besar data teks. 1) InnoDB melaksanakan carian teks penuh melalui pengindeksan terbalik, menyokong pertanyaan carian asas dan maju. 2) Gunakan perlawanan dan terhadap kata kunci untuk mencari, menyokong mod boolean dan carian frasa. 3) Kaedah pengoptimuman termasuk menggunakan teknologi segmentasi perkataan, membina semula indeks dan menyesuaikan saiz cache untuk meningkatkan prestasi dan ketepatan.
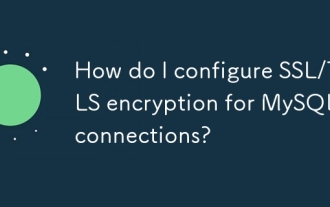
Artikel membincangkan mengkonfigurasi penyulitan SSL/TLS untuk MySQL, termasuk penjanaan sijil dan pengesahan. Isu utama menggunakan implikasi keselamatan sijil yang ditandatangani sendiri. [Kira-kira aksara: 159]
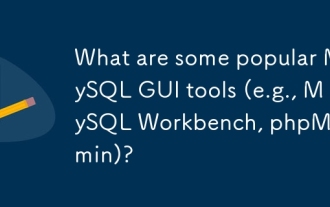
Artikel membincangkan alat MySQL GUI yang popular seperti MySQL Workbench dan PHPMyAdmin, membandingkan ciri dan kesesuaian mereka untuk pemula dan pengguna maju. [159 aksara]
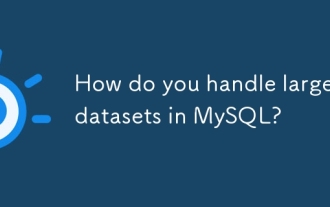
Artikel membincangkan strategi untuk mengendalikan dataset besar di MySQL, termasuk pembahagian, sharding, pengindeksan, dan pengoptimuman pertanyaan.
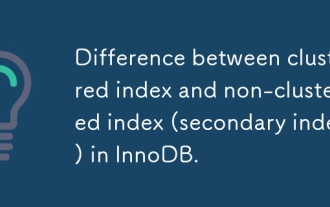
Perbezaan antara indeks clustered dan indeks bukan cluster adalah: 1. Klustered Index menyimpan baris data dalam struktur indeks, yang sesuai untuk pertanyaan oleh kunci dan julat utama. 2. Indeks Indeks yang tidak berkumpul indeks nilai utama dan penunjuk kepada baris data, dan sesuai untuk pertanyaan lajur utama bukan utama.
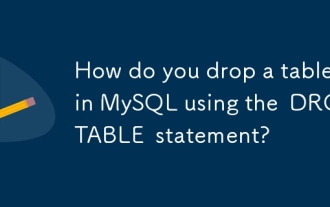
Artikel ini membincangkan jadual menjatuhkan di MySQL menggunakan pernyataan Jadual Drop, menekankan langkah berjaga -jaga dan risiko. Ia menyoroti bahawa tindakan itu tidak dapat dipulihkan tanpa sandaran, memperincikan kaedah pemulihan dan bahaya persekitaran pengeluaran yang berpotensi.
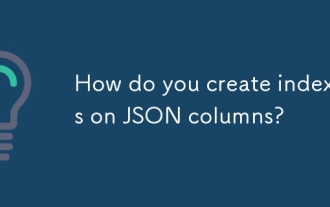
Artikel ini membincangkan membuat indeks pada lajur JSON dalam pelbagai pangkalan data seperti PostgreSQL, MySQL, dan MongoDB untuk meningkatkan prestasi pertanyaan. Ia menerangkan sintaks dan faedah mengindeks laluan JSON tertentu, dan menyenaraikan sistem pangkalan data yang disokong.
