


Detailed explanation of string processing in python data cleaning series
Preface
Data cleaning is a complex and tedious (kubi) task, and it is also the most important link in the entire data analysis process. Some people say that 80% of the time of an analysis project is cleaning data. This sounds strange, but it is true in actual work. There are two purposes of data cleaning. The first is to make the data available through cleaning. The second is to make the data more suitable for subsequent analysis. In other words, there is "dirty" data that needs to be washed, and clean data that needs to be washed as well.
In data analysis, especially text analysis, character processing requires a lot of energy, so understanding character processing is also a very important ability for data analysis.
String processing methods
First of all, let’s understand what the basic methods are.
First of all, let’s understand The split method of string below
str='i like apple,i like bananer' print(str.split(','))
The result of splitting the character str with commas:
['i like apple', 'i like bananer']
print(str.split(' '))
The result of splitting based on spaces:
['i', 'like', 'apple,i', 'like', 'bananer']
print(str.index(',')) print(str.find(','))
Both search results are:
12
If the index cannot be found, the index returns an error, and find returns -1
print(str.count('i'))
The result is:
4
connt is used to count the frequency of the target string
print(str.replace(',', ' ').split(' '))
The result is:
['i', 'like', 'apple', 'i', 'like', 'bananer']
replace here after replacing commas with spaces , using spaces to split the string, just enough to take out each word.
In addition to conventional methods, the most powerful character processing tool is regular expressions.
Regular expression
Before using regular expressions, we need to understand the many methods in regular expressions.
Let me look at the use of the next method. First understand the difference between the match and search methods
str = "Cats are smarter than dogs" pattern=re.compile(r'(.*) are (.*?) .*') result=re.match(pattern,str) for i in range(len(result.groups())+1): print(result.group(i))
The result is:
Cats are smarter than dogs
Cats
smarter
Under this form of pettern matching rules, the match and search methods The return result is the same
At this time, if the pattern is changed to
pattern=re.compile(r'are (.*?) .*')
match will return none, and the search will return the result For:
are smarter than dogs
smarter
Next let’s learn about the use of other methods
str = "138-9592-5592 # number" pattern=re.compile(r'#.*$') number=re.sub(pattern,'',str) print(number)
The result is:
138-9592-5592
The above is to extract the number by replacing the content after the # sign with nothing.
We can also further replace the crossbar of the number
print(re.sub(r'-*','',number))
The result is:
13895925592
We can also use the find method to print out the found string
str = "138-9592-5592 # number" pattern=re.compile(r'5') print(pattern.findall(str))
The result is:
['5', '5', '5']
The overall content of regular expressions is relatively large, and we need to have a sufficient understanding of the rules for matching strings. The following is Specific matching rules.
Vectorized string function
When cleaning up the scattered data to be analyzed, it is often necessary to do some string regularization work.
data = pd.Series({'li': '120@qq.com','wang':'5632@qq.com', 'chen': '8622@xinlang.com','zhao':np.nan,'sun':'5243@gmail.com'}) print(data)
The result is:
You can make preliminary judgments on the data through some integrated methods. , for example, use contains to determine whether each data contains the keyword
print(data.str.contains('@'))
. The result is:
You can also split the string and extract the required string
data = pd.Series({'li': '120@qq.com','wang':'5632@qq.com', 'chen': '8622@xinlang.com','zhao':np.nan,'sun':'5243@gmail.com'}) pattern=re.compile(r'(\d*)@([a-z]+)\.([a-z]{2,4})') result=data.str.match(pattern) #这里用fillall的方法也可以result=data.str.findall(pattern) print(result)
结果为:
chen [(8622, xinlang, com)]
li [(120, qq, com)]
sun [(5243, gmail, com)]
wang [(5632, qq, com)]
zhao NaN
dtype: object
此时加入我们需要提取邮箱前面的名称
print(result.str.get(0))
结果为:
或者需要邮箱所属的域名
print(result.str.get(1))
结果为:
当然也可以用切片的方式进行提取,不过提取的数据准确性不高
data = pd.Series({'li': '120@qq.com','wang':'5632@qq.com', 'chen': '8622@xinlang.com','zhao':np.nan,'sun':'5243@gmail.com'}) print(data.str[:6])
结果为:
最后我们了解下矢量化的字符串方法
更多Detailed explanation of string processing in python data cleaning series相关文章请关注PHP中文网!

Hot AI Tools

Undresser.AI Undress
AI-powered app for creating realistic nude photos

AI Clothes Remover
Online AI tool for removing clothes from photos.

Undress AI Tool
Undress images for free

Clothoff.io
AI clothes remover

Video Face Swap
Swap faces in any video effortlessly with our completely free AI face swap tool!

Hot Article

Hot Tools

Notepad++7.3.1
Easy-to-use and free code editor

SublimeText3 Chinese version
Chinese version, very easy to use

Zend Studio 13.0.1
Powerful PHP integrated development environment

Dreamweaver CS6
Visual web development tools

SublimeText3 Mac version
God-level code editing software (SublimeText3)

Hot Topics










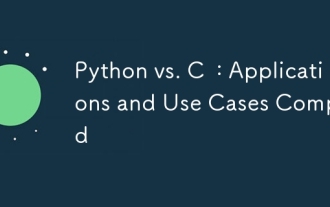
Python is suitable for data science, web development and automation tasks, while C is suitable for system programming, game development and embedded systems. Python is known for its simplicity and powerful ecosystem, while C is known for its high performance and underlying control capabilities.
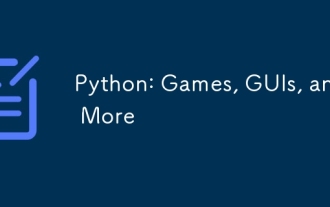
Python excels in gaming and GUI development. 1) Game development uses Pygame, providing drawing, audio and other functions, which are suitable for creating 2D games. 2) GUI development can choose Tkinter or PyQt. Tkinter is simple and easy to use, PyQt has rich functions and is suitable for professional development.
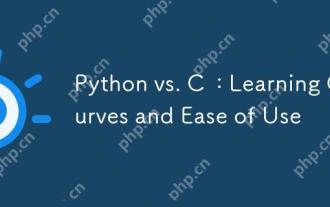
Python is easier to learn and use, while C is more powerful but complex. 1. Python syntax is concise and suitable for beginners. Dynamic typing and automatic memory management make it easy to use, but may cause runtime errors. 2.C provides low-level control and advanced features, suitable for high-performance applications, but has a high learning threshold and requires manual memory and type safety management.
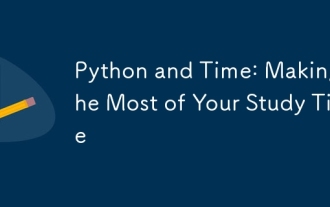
To maximize the efficiency of learning Python in a limited time, you can use Python's datetime, time, and schedule modules. 1. The datetime module is used to record and plan learning time. 2. The time module helps to set study and rest time. 3. The schedule module automatically arranges weekly learning tasks.
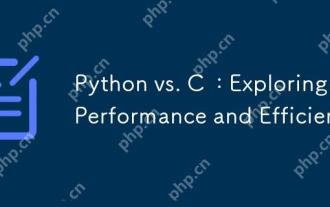
Python is better than C in development efficiency, but C is higher in execution performance. 1. Python's concise syntax and rich libraries improve development efficiency. 2.C's compilation-type characteristics and hardware control improve execution performance. When making a choice, you need to weigh the development speed and execution efficiency based on project needs.
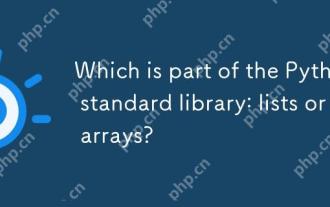
Pythonlistsarepartofthestandardlibrary,whilearraysarenot.Listsarebuilt-in,versatile,andusedforstoringcollections,whereasarraysareprovidedbythearraymoduleandlesscommonlyusedduetolimitedfunctionality.
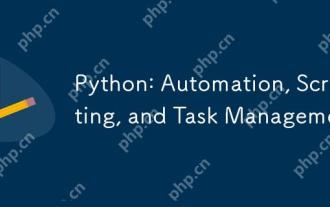
Python excels in automation, scripting, and task management. 1) Automation: File backup is realized through standard libraries such as os and shutil. 2) Script writing: Use the psutil library to monitor system resources. 3) Task management: Use the schedule library to schedule tasks. Python's ease of use and rich library support makes it the preferred tool in these areas.
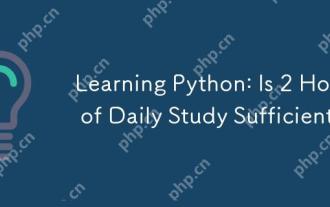
Is it enough to learn Python for two hours a day? It depends on your goals and learning methods. 1) Develop a clear learning plan, 2) Select appropriate learning resources and methods, 3) Practice and review and consolidate hands-on practice and review and consolidate, and you can gradually master the basic knowledge and advanced functions of Python during this period.
