KALDI语音识别工具包运行TIMIT数据库实例
TIMIT数据库介绍: TIMIT数据库由630个话者组成,每个人讲10句,美式英语的8种主要方言。 TIMIT S5实例: 首先,将TIMIT.ISO中的TIMIT复制到主文件夹。 1.进入对应的目录,进行如下操作: zhangju@ubuntu :~$ cd kaldi-trunk/egs/timit/s5/zhangju@ubuntu :~
TIMIT数据库介绍:
TIMIT数据库由630个话者组成,每个人讲10句,美式英语的8种主要方言。
TIMIT S5实例:
首先,将TIMIT.ISO中的TIMIT复制到主文件夹。
1.进入对应的目录,进行如下操作:
zhangju@ubuntu :~$ cd kaldi-trunk/egs/timit/s5/ zhangju@ubuntu :~/kaldi-trunk/egs/timit/s5$ sudo local/timit_data_prep.sh /home/zhangju/TIMIT
会看到如下显示:
Creating coretest set.
MDAB0 MWBT0 FELC0 MTAS1 MWEW0 FPAS0 MJMP0 MLNT0 FPKT0 MLLL0 MTLS0 FJLM0 MBPM0 MKLT0 FNLP0 MCMJ0 MJDH0 FMGD0 MGRT0 MNJM0 FDHC0 MJLN0 MPAM0 FMLD0
# of utterances in coretest set = 192
Creating dev set.
FAKS0 FDAC1 FJEM0 MGWT0 MJAR0 MMDB1 MMDM2 MPDF0 FCMH0 FKMS0 MBDG0 MBWM0 MCSH0 FADG0 FDMS0 FEDW0 MGJF0 MGLB0 MRTK0 MTAA0 MTDT0 MTHC0 MWJG0 FNMR0 FREW0 FSEM0 MBNS0 MMJR0 MDLS0 MDLF0 MDVC0 MERS0 FMAH0 FDRW0 MRCS0 MRJM4 FCAL1 MMWH0 FJSJ0 MAJC0 MJSW0 MREB0 FGJD0 FJMG0 MROA0 MTEB0 MJFC0 MRJR0 FMML0 MRWS1
# of utterances in dev set = 400
Finalizing test
Finalizing dev
timit_data_prep succeeded.
于是在/home/zhangju/kaldi-trunk/egs/timit/s5文件夹下新生成data文件夹,其内包含local文件夹以及相关内容。
2.在终端输入:
local/timit_train_lms.sh data/local(下载、计算文本,用以建立语言模型) local/timit_format_data.sh(处理与fst有关的东西)
3.创建train的mfcc:
sudo steps/make_mfcc.sh data/train exp/make_mfcc/train mfccs 4
(要对train,dev,test创建)
会看到:
Succeeded creating MFCC features for train
sudo steps/make_mfcc.sh data/test exp/make_mfcc/test mfccs 4
会看到:
Succeeded creating MFCC features for test
sudo steps/make_mfcc.sh data/dev exp/make_mfcc/dev mfccs 4
会看到:
Succeeded creating MFCC features for dev
4.训练单音素系统(monophone systom)
sudo steps/train_mono.sh data/train data/lang exp/mono
会显示:
Computing cepstral mean and variance statistics
Initializing monophone system.
Compiling training graphs
Pass 0
Pass 1
Aligning data
Pass 2
Aligning data
Pass 3
Aligning data
Pass 4
Aligning data
Pass 5
Aligning data
Pass 6
Aligning data
Pass 7
Aligning data
Pass 8
Aligning data
Pass 9
Aligning data
Pass 10
Aligning data
Pass 11
Pass 12
Aligning data
Pass 13
Pass 14
Pass 15
Aligning data
Pass 16
Pass 17
Pass 18
Pass 19
Pass 20
Aligning data
Pass 21
Pass 22
Pass 23
Pass 24
Pass 25
Aligning data
Pass 26
Pass 27
Pass 28
Pass 29
于是,新建了exp/mono文件夹
scripts/mkgraph.sh --mono data/lang exp/mono exp/mono/graph(制图)
会显示:
fsttablecompose data/lang/L.fst data/lang/G.fst
fstdeterminizestar --use-log=true
fstminimizeencoded
fstisstochastic data/lang/tmp/LG.fst
-0.000244359 -0.0912761
warning: LG not stochastic.
fstcomposecontext --context-size=1 --central-position=0 --read-disambig-syms=data/lang/tmp/disambig_phones.list --write-disambig-syms=data/lang/tmp/disambig_ilabels_1_0.list data/lang/tmp/ilabels_1_0
fstisstochastic data/lang/tmp/CLG_1_0.fst
-0.000244359 -0.0912761
warning: CLG not stochastic.
make-h-transducer --disambig-syms-out=exp/mono/graph/disambig_tid.list --transition-scale=1.0 data/lang/tmp/ilabels_1_0 exp/mono/tree exp/mono/final.mdl
fstminimizeencoded
fstdeterminizestar --use-log=true
fsttablecompose exp/mono/graph/Ha.fst data/lang/tmp/CLG_1_0.fst
fstrmsymbols exp/mono/graph/disambig_tid.list
fstrmepslocal
fstisstochastic exp/mono/graph/HCLGa.fst
0.000331581 -0.091291
HCLGa is not stochastic
add-self-loops --self-loop-scale=0.1 --reorder=true exp/mono/final.mdl
5.
for test in dev test ; do steps/decode_deltas.sh exp/mono data/$test data/lang exp/mono/decode_$test & done(解码test数据集(test是*/s5/data中dev、test文件夹中的test文件夹))
终端输出结果是:[1] 2307
[2] 2308
6.
scripts/average_wer.sh exp/mono/decode_*/wer > exp/mono/wer
会显示:
[1]- 完成 steps/decode_deltas.sh exp/mono data/$test data/lang exp/mono/decode_$test
[2]+ 完成 steps/decode_deltas.sh exp/mono data/$test data/lang exp/mono/decode_$test
7.从单音素系统中获得alignments:(分别从mono文件夹中的train,dev,test中获得)(用以训练其他系统)
steps/align_deltas.sh data/train data/lang exp/mono exp/mono_ali_train
会显示:
Computing cepstral mean and variance statistics
Aligning all training data
Done.
方法二:修改run.sh中的timit路径,但后直接运行run.sh
TIMIT S3实例
1 数据准备,输入:
local/timit_data_prep.sh /home/zhangju/TIMIT
终端显示:
Creating coretest set.
MDAB0 MWBT0 FELC0 MTAS1 MWEW0 FPAS0 MJMP0 MLNT0 FPKT0 MLLL0 MTLS0 FJLM0 MBPM0 MKLT0 FNLP0 MCMJ0 MJDH0 FMGD0 MGRT0 MNJM0 FDHC0 MJLN0 MPAM0 FMLD0 (这是说话人的名字,前面加M,F分别表示男性和女性)
# of utterances in coretest set = 192 (核心测试集中有192句话)
Creating dev set.
FAKS0 FDAC1 FJEM0 MGWT0 MJAR0 MMDB1 MMDM2 MPDF0 FCMH0 FKMS0 MBDG0 MBWM0 MCSH0 FADG0 FDMS0 FEDW0 MGJF0 MGLB0 MRTK0 MTAA0 MTDT0 MTHC0 MWJG0 FNMR0 FREW0 FSEM0 MBNS0 MMJR0 MDLS0 MDLF0 MDVC0 MERS0 FMAH0 FDRW0 MRCS0 MRJM4 FCAL1 MMWH0 FJSJ0 MAJC0 MJSW0 MREB0 FGJD0 FJMG0 MROA0 MTEB0 MJFC0 MRJR0 FMML0 MRWS1
# of utterances in dev set = 400 (设备集中有400句话)
Finalizing test (完成test)
Finalizing dev (完成dev)
timit_data_prep succeeded.
输入:
local/timit_train_lms.sh data/local
终端显示为
Not installing the kaldi_lm toolkit since it is already there.
(kaldi_lm工具箱里有:
compute_perplexity计算复杂度(用于对语言模型作评估,复杂度越低越好)
discount_ngrams给n阶语法模型作平滑处理(留出频率给实际会出现的但ngram中没出现的词语组合)
get_raw_ngrams(得到原始n阶语法模型)
get_word_map.pl*(得到词语的映射表)
interpolate_ngrams(补充(修改)n阶语法模型)
finalize_arpa.pl(完成arpa(arpa是一种格式,协议),是interpolate_ngrams程序中调用的)
map_words_in_arpa.pl(得到arpa格式的词语)
merge_ngrams(合并、融合n阶语法模型)
merge_ngrams_online(在线合并、融合n阶语法模型)
optimize_alpha.pl(使alpha最优化)
prune_lm.sh(删去出现频率较低的数据)
prune_ngrams(删去出现频率较低的数据)
scale_configs.pl
train_lm.sh(训练语言模型)
uniq_to_ngrams)
Creating phones file, and monophone lexicon (mapping phones to itself). (创建音子文件及单音素词典)
Creating biphone model(创建双音子模型)
Training biphone language model in folder data/local/lm (训练双音子语言模型)
Creating directory data/local/lm/biphone (创建目录data/local/lm/biphone )
Getting raw N-gram counts ()
Iteration 1/7 of optimizing discounting parameters
discount_ngrams: for n-gram order 1, D=0.400000, tau=0.900000 phi=2.000000
interpolate_ngrams: 60 words in wordslist
discount_ngrams: for n-gram order 2, D=0.600000, tau=0.900000 phi=2.000000
discount_ngrams: for n-gram order 3, D=0.800000, tau=1.100000 phi=2.000000
discount_ngrams: for n-gram order 1, D=0.400000, tau=0.675000 phi=2.000000
discount_ngrams: for n-gram order 2, D=0.600000, tau=0.675000 phi=2.000000
discount_ngrams: for n-gram order 3, D=0.800000, tau=0.825000 phi=2.000000
interpolate_ngrams: 60 words in wordslist
discount_ngrams: for n-gram order 1, D=0.400000, tau=1.215000 phi=2.000000
discount_ngrams: for n-gram order 2, D=0.600000, tau=1.215000 phi=2.000000
discount_ngrams: for n-gram order 3, D=0.800000, tau=1.485000 phi=2.000000
interpolate_ngrams: 60 words in wordslist
Perplexity over 11412.000000 words is 17.013357
Perplexity over 10833.000000 words (excluding 579.000000 OOVs) is 17.460842
real 0m0.021s
user 0m0.012s
sys 0m0.000s
Perplexity over 11412.000000 words is 17.016472
Perplexity over 10833.000000 words (excluding 579.000000 OOVs) is 17.464985
real 0m0.020s
user 0m0.012s
sys 0m0.000s
Perplexity over 11412.000000 words is 17.021475
Perplexity over 10833.000000 words (excluding 579.000000 OOVs) is 17.471402
real 0m0.025s
user 0m0.012s
sys 0m0.000s
optimize_alpha.pl: alpha=-2.1628504673 is too negative, limiting it to -0.5
Projected perplexity change from setting alpha=-0.5 is 17.016472->17.0106241428571, reduction of 0.00584785714286085
Alpha value on iter 1 is -0.5
Iteration 2/7 of optimizing discounting parameters
discount_ngrams: for n-gram order 1, D=0.400000, tau=0.450000 phi=2.000000
discount_ngrams: for n-gram order 2, D=0.600000, tau=0.450000 phi=2.000000
discount_ngrams: for n-gram order 3, D=0.600000, tau=0.550000 phi=2.000000
interpolate_ngrams: 60 words in wordslist
interpolate_ngrams: 60 words in wordslist
discount_ngrams: for n-gram order 1, D=0.400000, tau=0.450000 phi=2.000000
discount_ngrams: for n-gram order 2, D=0.600000, tau=0.450000 phi=2.000000
discount_ngrams: for n-gram order 3, D=0.800000, tau=0.550000 phi=2.000000
interpolate_ngrams: 60 words in wordslist
discount_ngrams: for n-gram order 1, D=0.400000, tau=0.450000 phi=2.000000
discount_ngrams: for n-gram order 2, D=0.600000, tau=0.450000 phi=2.000000
discount_ngrams: for n-gram order 3, D=1.080000, tau=0.550000 phi=2.000000
Perplexity over 11412.000000 words is 17.011355
Perplexity over 10833.000000 words (excluding 579.000000 OOVs) is 17.457880
real 0m0.018s
user 0m0.004s
sys 0m0.008s
Perplexity over 11412.000000 words is 17.011355
Perplexity over 10833.000000 words (excluding 579.000000 OOVs) is 17.457880
real 0m0.022s
user 0m0.012s
sys 0m0.000s
Perplexity over 11412.000000 words is 17.011355
Perplexity over 10833.000000 words (excluding 579.000000 OOVs) is 17.457880
real 0m0.019s
user 0m0.008s
sys 0m0.004s
optimize_alpha.pl: objective function is not convex; returning alpha=0.7
Projected perplexity change from setting alpha=0.7 is 17.011355->17.011355, reduction of 0
Alpha value on iter 2 is 0.7
Iteration 3/7 of optimizing discounting parameters
discount_ngrams: for n-gram order 1, D=0.400000, tau=0.450000 phi=2.000000
discount_ngrams: for n-gram order 2, D=0.600000, tau=0.450000 phi=2.000000
discount_ngrams: for n-gram order 3, D=1.360000, tau=0.412500 phi=2.000000
interpolate_ngrams: 60 words in wordslist
discount_ngrams: for n-gram order 1, D=0.400000, tau=0.450000 phi=2.000000
discount_ngrams: for n-gram order 2, D=0.600000, tau=0.450000 phi=2.000000
discount_ngrams: for n-gram order 3, D=1.360000, tau=0.550000 phi=2.000000
interpolate_ngrams: 60 words in wordslist
discount_ngrams: for n-gram order 1, D=0.400000, tau=0.450000 phi=2.000000
discount_ngrams: for n-gram order 2, D=0.600000, tau=0.450000 phi=2.000000
discount_ngrams: for n-gram order 3, D=1.360000, tau=0.742500 phi=2.000000
interpolate_ngrams: 60 words in wordslist
Perplexity over 11412.000000 words is 17.011355
Perplexity over 10833.000000 words (excluding 579.000000 OOVs) is 17.457880
real 0m0.020s
user 0m0.012s
sys 0m0.000s
Perplexity over 11412.000000 words is 17.011355
Perplexity over 10833.000000 words (excluding 579.000000 OOVs) is 17.457880
real 0m0.019s
user 0m0.008s
sys 0m0.004s
Perplexity over 11412.000000 words is 17.011355
Perplexity over 10833.000000 words (excluding 579.000000 OOVs) is 17.457880
real 0m0.021s
user 0m0.012s
sys 0m0.000s
optimize_alpha.pl: objective function is not convex; returning alpha=0.7
Projected perplexity change from setting alpha=0.7 is 17.011355->17.011355, reduction of 0
Alpha value on iter 3 is 0.7
Iteration 4/7 of optimizing discounting parameters
discount_ngrams: for n-gram order 1, D=0.400000, tau=0.450000 phi=2.000000
discount_ngrams: for n-gram order 2, D=0.600000, tau=0.450000 phi=2.000000
discount_ngrams: for n-gram order 3, D=1.360000, tau=0.935000 phi=1.750000
interpolate_ngrams: 60 words in wordslist
discount_ngrams: for n-gram order 1, D=0.400000, tau=0.450000 phi=2.000000
discount_ngrams: for n-gram order 2, D=0.600000, tau=0.450000 phi=2.000000
discount_ngrams: for n-gram order 3, D=1.360000, tau=0.935000 phi=2.000000
interpolate_ngrams: 60 words in wordslist
discount_ngrams: for n-gram order 1, D=0.400000, tau=0.450000 phi=2.000000
discount_ngrams: for n-gram order 2, D=0.600000, tau=0.450000 phi=2.000000
discount_ngrams: for n-gram order 3, D=1.360000, tau=0.935000 phi=2.350000
interpolate_ngrams: 60 words in wordslist
Perplexity over 11412.000000 words is 17.011355
Perplexity over 10833.000000 words (excluding 579.000000 OOVs) is 17.457880
real 0m0.018s
user 0m0.012s
sys 0m0.000s
Perplexity over 11412.000000 words is 17.011355
Perplexity over 10833.000000 words (excluding 579.000000 OOVs) is 17.457880
real 0m0.018s
user 0m0.012s
sys 0m0.000s
Perplexity over 11412.000000 words is 17.011355
Perplexity over 10833.000000 words (excluding 579.000000 OOVs) is 17.457880
real 0m0.023s
user 0m0.012s
sys 0m0.000s
optimize_alpha.pl: objective function is not convex; returning alpha=0.7
Projected perplexity change from setting alpha=0.7 is 17.011355->17.011355, reduction of 0
Alpha value on iter 4 is 0.7
Iteration 5/7 of optimizing discounting parameters
discount_ngrams: for n-gram order 1, D=0.400000, tau=0.450000 phi=2.000000
discount_ngrams: for n-gram order 2, D=0.450000, tau=0.450000 phi=2.000000
discount_ngrams: for n-gram order 3, D=1.360000, tau=0.935000 phi=2.700000
interpolate_ngrams: 60 words in wordslist
interpolate_ngrams: 60 words in wordslist
discount_ngrams: for n-gram order 1, D=0.400000, tau=0.450000 phi=2.000000
discount_ngrams: for n-gram order 2, D=0.600000, tau=0.450000 phi=2.000000
discount_ngrams: for n-gram order 3, D=1.360000, tau=0.935000 phi=2.700000
interpolate_ngrams: 60 words in wordslist
discount_ngrams: for n-gram order 1, D=0.400000, tau=0.450000 phi=2.000000
discount_ngrams: for n-gram order 2, D=0.810000, tau=0.450000 phi=2.000000
discount_ngrams: for n-gram order 3, D=1.360000, tau=0.935000 phi=2.700000
Perplexity over 11412.000000 words is 17.008195
Perplexity over 10833.000000 words (excluding 579.000000 OOVs) is 17.454326
real 0m0.019s
user 0m0.008s
sys 0m0.004s
Perplexity over 11412.000000 words is 17.011355
Perplexity over 10833.000000 words (excluding 579.000000 OOVs) is 17.457880
real 0m0.019s
user 0m0.012s
sys 0m0.000s
Perplexity over 11412.000000 words is 17.018212
Perplexity over 10833.000000 words (excluding 579.000000 OOVs) is 17.465417
real 0m0.021s
user 0m0.012s
sys 0m0.000s
optimize_alpha.pl: alpha=-0.670499383475985 is too negative, limiting it to -0.5
Projected perplexity change from setting alpha=-0.5 is 17.011355->17.0064832142857, reduction of 0.00487178571427904
Alpha value on iter 5 is -0.5
Iteration 6/7 of optimizing discounting parameters
interpolate_ngrams: 60 words in wordslist
discount_ngrams: for n-gram order 1, D=0.400000, tau=0.450000 phi=2.000000
discount_ngrams: for n-gram order 1, D=0.400000, tau=0.450000 phi=2.000000
discount_ngrams: for n-gram order 2, D=0.300000, tau=0.337500 phi=2.000000
discount_ngrams: for n-gram order 3, D=1.360000, tau=0.935000 phi=2.700000
interpolate_ngrams: 60 words in wordslist
discount_ngrams: for n-gram order 2, D=0.300000, tau=0.450000 phi=2.000000
discount_ngrams: for n-gram order 3, D=1.360000, tau=0.935000 phi=2.700000
interpolate_ngrams: 60 words in wordslist
discount_ngrams: for n-gram order 1, D=0.400000, tau=0.450000 phi=2.000000
discount_ngrams: for n-gram order 2, D=0.300000, tau=0.607500 phi=2.000000
discount_ngrams: for n-gram order 3, D=1.360000, tau=0.935000 phi=2.700000
Perplexity over 11412.000000 words is 17.008198
Perplexity over 10833.000000 words (excluding 579.000000 OOVs) is 17.454134
real 0m0.019s
user 0m0.012s
sys 0m0.000s
Perplexity over 11412.000000 words is 17.006972
Perplexity over 10833.000000 words (excluding 579.000000 OOVs) is 17.452861
real 0m0.020s
user 0m0.012s
sys 0m0.000s
Perplexity over 11412.000000 words is 17.006526
Perplexity over 10833.000000 words (excluding 579.000000 OOVs) is 17.452349
real 0m0.022s
user 0m0.012s
sys 0m0.000s
Projected perplexity change from setting alpha=0.280321158690507 is 17.006972->17.0064966287094, reduction of 0.000475371290633575
Alpha value on iter 6 is 0.280321158690507
Iteration 7/7 of optimizing discounting parameters
discount_ngrams: for n-gram order 1, D=0.400000, tau=0.450000 phi=2.000000
discount_ngrams: for n-gram order 2, D=0.300000, tau=0.576145 phi=1.750000
discount_ngrams: for n-gram order 3, D=1.360000, tau=0.935000 phi=2.700000
interpolate_ngrams: 60 words in wordslist
discount_ngrams: for n-gram order 1, D=0.400000, tau=0.450000 phi=2.000000
discount_ngrams: for n-gram order 2, D=0.300000, tau=0.576145 phi=2.350000
discount_ngrams: for n-gram order 3, D=1.360000, tau=0.935000 phi=2.700000
discount_ngrams: for n-gram order 1, D=0.400000, tau=0.450000 phi=2.000000
discount_ngrams: for n-gram order 2, D=0.300000, tau=0.576145 phi=2.000000
discount_ngrams: for n-gram order 3, D=1.360000, tau=0.935000 phi=2.700000
interpolate_ngrams: 60 words in wordslist
interpolate_ngrams: 60 words in wordslist
Perplexity over 11412.000000 words is 17.006845
Perplexity over 10833.000000 words (excluding 579.000000 OOVs) is 17.452750
real 0m0.019s
user 0m0.012s
sys 0m0.000s
Perplexity over 11412.000000 words is 17.006575
Perplexity over 10833.000000 words (excluding 579.000000 OOVs) is 17.452414
real 0m0.021s
user 0m0.012s
sys 0m0.000s
Perplexity over 11412.000000 words is 17.006336
Perplexity over 10833.000000 words (excluding 579.000000 OOVs) is 17.452127
real 0m0.022s
user 0m0.012s
sys 0m0.000s
Projected perplexity change from setting alpha=0.690827338145686 is 17.006575->17.0062591109755, reduction of 0.000315889024498972
Alpha value on iter 7 is 0.690827338145686
Final config is:
D=0.4 tau=0.45 phi=2.0
D=0.3 tau=0.576144521410728 phi=2.69082733814569
D=1.36 tau=0.935 phi=2.7
Discounting N-grams.
discount_ngrams: for n-gram order 1, D=0.400000, tau=0.450000 phi=2.000000
discount_ngrams: for n-gram order 2, D=0.300000, tau=0.576145 phi=2.690827
discount_ngrams: for n-gram order 3, D=1.360000, tau=0.935000 phi=2.700000
Computing final perplexity
Building ARPA LM (perplexity computation is in background)
interpolate_ngrams: 60 words in wordslist
interpolate_ngrams: 60 words in wordslist
Perplexity over 11412.000000 words is 17.006029
Perplexity over 10833.000000 words (excluding 579.000000 OOVs) is 17.451754
17.006029
输入
local/timit_format_data.sh
终端显示:
Creating L.fst
Done creating L.fst
Creating L_disambig.fst
Done creating L_disambig.fst
Creating G.fst
arpa2fst -
\data\
Processing 1-grams
Processing 2-grams
Connected 0 states without outgoing arcs.
remove_oovs.pl: removed 0 lines.
G.fst created. How stochastic is it ?
fstisstochastic data/lang_test/G.fst
0 -0.0900995
fsttablecompose data/lang_test/L_disambig.fst data/lang_test/G.fst
How stochastic is LG.fst.
fstisstochastic data/lang_test/G.fst
0 -0.0900995
fstisstochastic
fsttablecompose data/lang/L.fst data/lang_test/G.fst
0 -0.0900994
How stochastic is LG_disambig.fst.
fsttablecompose data/lang_test/L_disambig.fst data/lang_test/G.fst
fstisstochastic
0 -0.0900994
First few lines of lexicon FST:
0 1
0 1 sil
1 2 aa AA 1.20397282
1 1 aa AA 0.356674939
1 1 ae AE 0.356674939
1 2 ae AE 1.20397282
1 1 ah AH 0.356674939
1 2 ah AH 1.20397282
1 1 ao AO 0.356674939
1 2 ao AO 1.20397282
timit_format_data succeeded.
输入:
mfccdir=mfccs for test in train test dev ; do > steps/make_mfcc.sh data/$test exp/make_mfcc/$test $mfccdir 4 > done
终端显示:
Succeeded creating MFCC features for train
Succeeded creating MFCC features for test
Succeeded creating MFCC features for dev
2 训练单音素系统,终端输入:
steps/train_mono.sh data/train data/lang exp/mono
终端显示:
Computing cepstral mean and variance statistics
Initializing monophone system.
Compiling training graphs
Pass 0
Pass 1
Aligning data
Pass 2
Aligning data
Pass 3
Aligning data
Pass 4
Aligning data
Pass 5
Aligning data
Pass 6
Aligning data
Pass 7
Aligning data
Pass 8
Aligning data
Pass 9
Aligning data
Pass 10
Aligning data
Pass 11
Pass 12
Aligning data
Pass 13
Pass 14
Pass 15
Aligning data
Pass 16
Pass 17
Pass 18
Pass 19
Pass 20
Aligning data
Pass 21
Pass 22
Pass 23
Pass 24
Pass 25
Aligning data
Pass 26
Pass 27
Pass 28
Pass 29
scripts/mkgraph.sh --mono data/lang_test exp/mono exp/mono/graph(制图)
终端显示:
fsttablecompose data/lang_test/L_disambig.fst data/lang_test/G.fst
fstminimizeencoded
fstdeterminizestar --use-log=true
fstisstochastic data/lang_test/tmp/LG.fst
0 -0.0901494
warning: LG not stochastic.
fstcomposecontext --context-size=1 --central-position=0 --read-disambig-syms=data/lang_test/tmp/disambig_phones.list --write-disambig-syms=data/lang_test/tmp/disambig_ilabels_1_0.list data/lang_test/tmp/ilabels_1_0
fstisstochastic data/lang_test/tmp/CLG_1_0.fst
0 -0.0901494
warning: CLG not stochastic.
make-h-transducer --disambig-syms-out=exp/mono/graph/disambig_tid.list --transition-scale=1.0 data/lang_test/tmp/ilabels_1_0 exp/mono/tree exp/mono/final.mdl
fsttablecompose exp/mono/graph/Ha.fst data/lang_test/tmp/CLG_1_0.fst
fstdeterminizestar --use-log=true
fstminimizeencoded
fstrmsymbols exp/mono/graph/disambig_tid.list
fstrmepslocal
fstisstochastic exp/mono/graph/HCLGa.fst
0 -0.0901494
HCLGa is not stochastic
add-self-loops --self-loop-scale=0.1 --reorder=true exp/mono/final.mdl
3 解码测试的数据集,输入
for test in dev test ; do steps/decode_deltas.sh exp/mono data/$test data/lang exp/mono/decode_$test & done
终端显示:
[1] 16368
[2] 16369
3.1计算结果,输入:
scripts/average_wer.sh exp/mono/decode_*/wer > exp/mono/wer
终端显示:
[1]- 完成 steps/decode_deltas.sh exp/mono data/$test data/lang exp/mono/decode_$test
[2]+ 完成 steps/decode_deltas.sh exp/mono data/$test data/lang exp/mono/decode_$test
4 从单音素系统中获得排列
创建排列用以训练其他系统,如ANN-HMM。
输入:
steps/align_deltas.sh data/train data/lang exp/mono exp/mono_ali_train
终端显示:
Computing cepstral mean and variance statistics
Aligning all training data
Done.
steps/align_deltas.sh data/dev data/lang exp/mono exp/mono_ali_dev
方法二:修改相应的TIMIT路径之后,直接运行run.sh
TIMIT S4实例此脚本是用于构建一个音位识别器
WORKDIR=/home/zhangju/ss4(自己找个有空间的路径作为WORKDIR)
mkdir -p $WORKDIR
cp -r conf local utils steps path.sh $WORKDIR
cd $WORKDIR
. path.sh(此文件中的环境变量KALDIROOT要自己修改路径,改到自己裝的kaldi文件中。KALDIROOT=/home/mayuan/kaldi-trunk(我用nano改的。))
local/timit_data_prep.sh --config-dir=$PWD/conf --corpus-dir=/home/zhangju/TIMIT --work-dir=$WORKDIR

熱AI工具

Undresser.AI Undress
人工智慧驅動的應用程序,用於創建逼真的裸體照片

AI Clothes Remover
用於從照片中去除衣服的線上人工智慧工具。

Undress AI Tool
免費脫衣圖片

Clothoff.io
AI脫衣器

Video Face Swap
使用我們完全免費的人工智慧換臉工具,輕鬆在任何影片中換臉!

熱門文章

熱工具

記事本++7.3.1
好用且免費的程式碼編輯器

SublimeText3漢化版
中文版,非常好用

禪工作室 13.0.1
強大的PHP整合開發環境

Dreamweaver CS6
視覺化網頁開發工具

SublimeText3 Mac版
神級程式碼編輯軟體(SublimeText3)
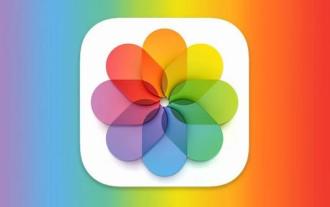
蘋果公司最新發布的iOS18、iPadOS18以及macOSSequoia系統為Photos應用程式增添了一項重要功能,旨在幫助用戶輕鬆恢復因各種原因遺失或損壞的照片和影片。這項新功能在Photos應用的"工具"部分引入了一個名為"已恢復"的相冊,當用戶設備中存在未納入其照片庫的圖片或影片時,該相冊將自動顯示。 "已恢復"相簿的出現為因資料庫損壞、相機應用未正確保存至照片庫或第三方應用管理照片庫時照片和視頻丟失提供了解決方案。使用者只需簡單幾步
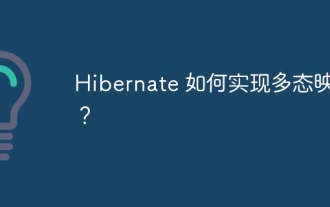
Hibernate多態映射可映射繼承類別到資料庫,提供以下映射類型:joined-subclass:為子類別建立單獨表,包含父類別所有欄位。 table-per-class:為子類別建立單獨資料表,僅包含子類別特有列。 union-subclass:類似joined-subclass,但父類別表聯合所有子類別列。
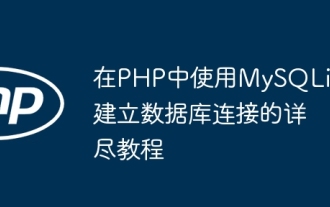
如何在PHP中使用MySQLi建立資料庫連線:包含MySQLi擴充(require_once)建立連線函數(functionconnect_to_db)呼叫連線函數($conn=connect_to_db())執行查詢($result=$conn->query())關閉連線( $conn->close())
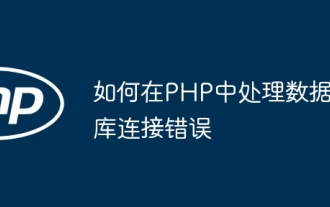
PHP處理資料庫連線報錯,可以使用下列步驟:使用mysqli_connect_errno()取得錯誤代碼。使用mysqli_connect_error()取得錯誤訊息。透過擷取並記錄這些錯誤訊息,可以輕鬆識別並解決資料庫連接問題,確保應用程式的順暢運作。
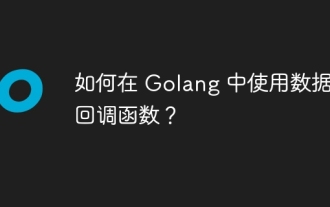
在Golang中使用資料庫回呼函數可以實現:在指定資料庫操作完成後執行自訂程式碼。透過單獨的函數新增自訂行為,無需編寫額外程式碼。回調函數可用於插入、更新、刪除和查詢操作。必須使用sql.Exec、sql.QueryRow或sql.Query函數才能使用回呼函數。
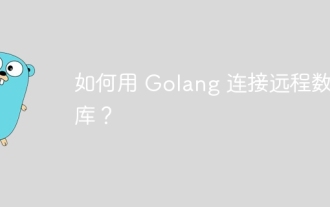
透過Go標準庫database/sql包,可以連接到MySQL、PostgreSQL或SQLite等遠端資料庫:建立包含資料庫連接資訊的連接字串。使用sql.Open()函數開啟資料庫連線。執行SQL查詢和插入操作等資料庫操作。使用defer關閉資料庫連線以釋放資源。
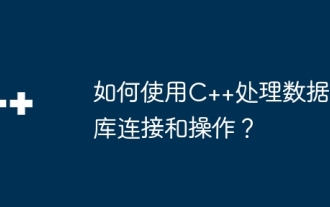
在C++中使用DataAccessObjects(DAO)函式庫連接和操作資料庫,包括建立資料庫連線、執行SQL查詢、插入新記錄和更新現有記錄。具體步驟為:1.包含必要的函式庫語句;2.開啟資料庫檔案;3.建立Recordset物件執行SQL查詢或操作資料;4.遍歷結果或依照特定需求更新記錄。
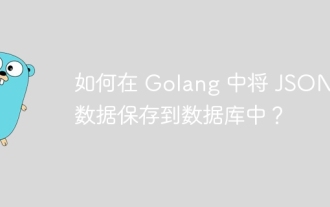
可以透過使用gjson函式庫或json.Unmarshal函數將JSON資料儲存到MySQL資料庫中。 gjson函式庫提供了方便的方法來解析JSON字段,而json.Unmarshal函數需要一個目標類型指標來解組JSON資料。這兩種方法都需要準備SQL語句和執行插入操作來將資料持久化到資料庫中。
