C++中有哪些適用於先進資料處理的函式庫或框架?
C++ 中有各種函式庫和框架可簡化高階資料處理任務:Eigen:用於線性代數運算,針對速度和效率最佳化。 Armadillo:類似於 Eigen,提供更友善的語法和便捷的函數調用,擅長處理稀疏矩陣。 TensorFlow:用於機器學習和深度學習,支援大量資料集並提供用於建立和訓練神經網路模型的工具。
C++ 高階資料處理函式庫和框架
C++ 中存在著大量的函式庫和框架,可以大幅簡化高階資料處理任務。本文將介紹幾個流行且功能強大的選項。
Eigen
Eigen 是一個用於線性代數運算的 C++ 範本函式庫。它提供了廣泛的矩陣和向量操作,包括求逆、求特徵值和線性求解器。 Eigen 針對速度和效率進行了最佳化,是大型資料集處理的理想選擇。
實戰案例:
#include <Eigen/Dense> int main() { // 创建一个 3x3 矩阵 Eigen::Matrix3d A; A << 1, 2, 3, 4, 5, 6, 7, 8, 9; // 求矩阵的特征值 Eigen::EigenSolver<Eigen::Matrix3d> es(A); Eigen::VectorXd eigenvalues = es.eigenvalues().real(); // 打印特征值 std::cout << "特征值:" << eigenvalues << std::endl; return 0; }
Armadillo
Armadillo 是另一個用於線性代數運算的 C++ 模板函式庫。它與 Eigen 類似,但提供了更友善的語法和更方便的函數呼叫。 Armadillo 特別擅長處理稀疏矩陣。
實戰案例:
#include <armadillo> int main() { // 创建一个 3x3 矩阵 arma::mat A = { {1, 2, 3}, {4, 5, 6}, {7, 8, 9} }; // 求矩阵的行列式 double det = arma::det(A); // 打印行列式 std::cout << "行列式:" << det << std::endl; return 0; }
TensorFlow
TensorFlow 是機器學習和深度學習的開源函式庫。它提供了一組用於建立和訓練神經網路模型的工具。 TensorFlow 具有可擴展性和效率,即使在處理大量資料集時也能提供出色的效能。
實戰案例:
#include <tensorflow/core/public/session.h> #include <tensorflow/core/public/tensor.h> int main() { // 创建一个 TensorFlow 会话 tensorflow::Session session; // 定义一个简单的线性回归模型 tensorflow::GraphDef graph; tensorflow::Tensor w(tensorflow::DT_FLOAT, tensorflow::TensorShape({1})); tensorflow::Tensor b(tensorflow::DT_FLOAT, tensorflow::TensorShape({1})); auto node1 = graph.add_node(); node1.set_op("Placeholder"); node1.add_attr("dtype", tensorflow::DT_FLOAT); node1.add_attr("shape", tensorflow::TensorShape({1}).AsProto()); auto node2 = graph.add_node(); node2.set_op("Variable"); node2.add_attr("dtype", tensorflow::DT_FLOAT); node2.add_attr("shape", tensorflow::TensorShape({1}).AsProto()); node2.add_attr("variable_name", "w"); auto node3 = graph.add_node(); node3.set_op("Variable"); node3.add_attr("dtype", tensorflow::DT_FLOAT); node3.add_attr("shape", tensorflow::TensorShape({1}).AsProto()); node3.add_attr("variable_name", "b"); auto node4 = graph.add_node(); node4.set_op("MatMul"); node4.add_input(node1.name()); node4.add_input(node2.name()); auto node5 = graph.add_node(); node5.set_op("BiasAdd"); node5.add_input(node4.name()); node5.add_input(node3.name()); // 加载模型到会话中 tensorflow::Status status = session.Run(tensorflow::GraphDefRequest{}, {}, {"w", "b"}, &outputs); // 打印变量的值 std::cout << "w: " << outputs[0].scalar<float>()() << std::endl; std::cout << "b: " << outputs[1].scalar<float>()() << std::endl; return 0; }
結語
這些函式庫和框架只是 C++ 中用於高階資料處理的眾多選項中的一小部分。選擇最適合您需求的程式庫或框架取決於您正在處理的任務的具體性質和規模。
以上是C++中有哪些適用於先進資料處理的函式庫或框架?的詳細內容。更多資訊請關注PHP中文網其他相關文章!

熱AI工具

Undresser.AI Undress
人工智慧驅動的應用程序,用於創建逼真的裸體照片

AI Clothes Remover
用於從照片中去除衣服的線上人工智慧工具。

Undress AI Tool
免費脫衣圖片

Clothoff.io
AI脫衣器

Video Face Swap
使用我們完全免費的人工智慧換臉工具,輕鬆在任何影片中換臉!

熱門文章

熱工具

記事本++7.3.1
好用且免費的程式碼編輯器

SublimeText3漢化版
中文版,非常好用

禪工作室 13.0.1
強大的PHP整合開發環境

Dreamweaver CS6
視覺化網頁開發工具

SublimeText3 Mac版
神級程式碼編輯軟體(SublimeText3)
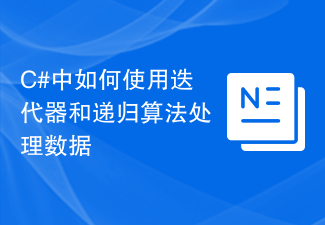
C#中如何使用迭代器和遞歸演算法處理數據,需要具體程式碼範例在C#中,迭代器和遞歸演算法是兩種常用的數據處理方法。迭代器可以幫助我們遍歷集合中的元素,而遞歸演算法則能夠有效地處理複雜的問題。本文將詳細介紹如何使用迭代器和遞歸演算法來處理數據,並提供具體的程式碼範例。使用迭代器處理資料在C#中,我們可以使用迭代器來遍歷集合中的元素,而無需事先知道集合的大小。透過迭代器,我
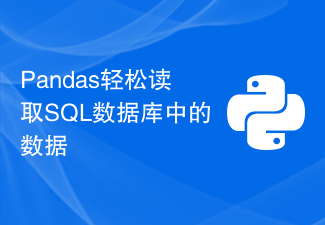
資料處理利器:Pandas讀取SQL資料庫中的數據,需要具體程式碼範例隨著資料量的不斷增長和複雜性的提高,資料處理成為了現代社會中一個重要的環節。在資料處理過程中,Pandas成為了許多資料分析師和科學家的首選工具之一。本文將介紹如何使用Pandas函式庫來讀取SQL資料庫中的數據,並提供一些具體的程式碼範例。 Pandas是基於Python的一個強大的數據處理和分
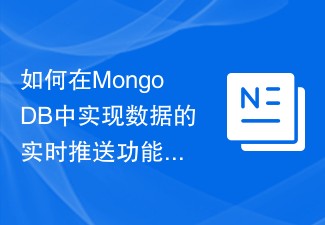
如何在MongoDB中實現資料的即時推送功能MongoDB是一種以文件為導向的NoSQL資料庫,其特點是具有高可擴展性和靈活的資料模型。在一些應用場景中,我們需要即時推送資料更新給客戶端,以便及時更新介面或做出相應的操作。本文將介紹如何在MongoDB中實現資料的即時推送功能,並給出具體的程式碼範例。實現即時推播功能的方法有很多種,例如使用輪詢、長輪詢、Web
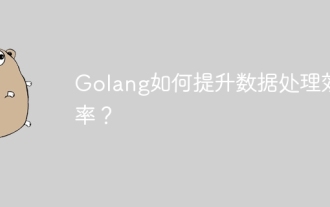
Golang透過並發性、高效能記憶體管理、原生資料結構和豐富的第三方函式庫,提升資料處理效率。具體優勢包括:並行處理:協程支援同時執行多個任務。高效率記憶體管理:垃圾回收機制自動管理記憶體。高效資料結構:切片、映射和通道等資料結構快速存取和處理資料。第三方函式庫:涵蓋fasthttp和x/text等各種資料處理庫。
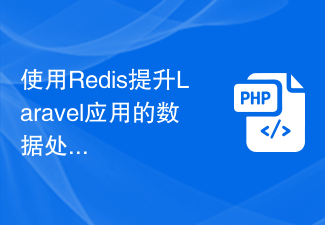
使用Redis提升Laravel應用的資料處理效率隨著網路應用的不斷發展,資料處理效率成為了開發者關注的重點之一。在開發基於Laravel框架的應用時,我們可以藉助Redis來提升資料處理效率,實現資料的快速存取和快取。本文將介紹如何使用Redis在Laravel應用中進行資料處理,並提供具體的程式碼範例。一、Redis簡介Redis是一種高效能的記憶體數據
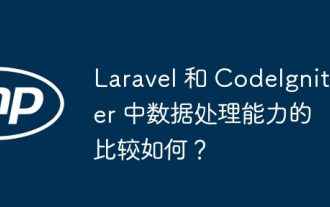
比較Laravel和CodeIgniter的資料處理能力:ORM:Laravel使用EloquentORM,提供類別物件關係映射,而CodeIgniter使用ActiveRecord,將資料庫模型表示為PHP類別的子類別。查詢建構器:Laravel具有靈活的鍊式查詢API,而CodeIgniter的查詢建構器更簡單,基於陣列。資料驗證:Laravel提供了一個Validator類,支援自訂驗證規則,而CodeIgniter的驗證功能內建較少,需要手動編碼自訂規則。實戰案例:用戶註冊範例展示了Lar
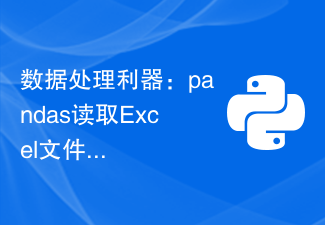
隨著數據處理的日益普及,越來越多人開始關注如何有效利用數據,讓數據為自己所用的。而在日常的資料處理中,Excel表格無疑是最常見的一種資料格式。然而,當需要處理大量資料時,手動操作Excel顯然會變得十分費時費力。因此,本文將介紹一個高效率的資料處理利器-pandas,以及如何利用該工具快速讀取Excel檔案並進行資料處理。一、pandas簡介pandas
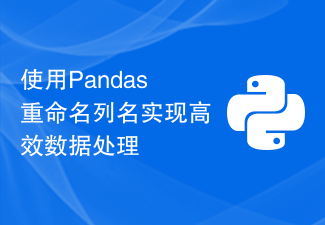
高效資料處理:使用Pandas修改列名,需要具體程式碼範例資料處理是資料分析中一個非常重要的環節,而在資料處理過程中,經常需要對資料的列名進行修改。 Pandas是一個強大的資料處理庫,提供了豐富的方法和函數來幫助我們快速且有效率地處理資料。本文將介紹如何使用Pandas修改列名,並提供具體的程式碼範例。在實際的資料分析中,原始資料的列名可能存在命名規範不統一、不易理解
