本網站AIxiv專欄是發布學術、技術內容的欄位。過去幾年,本站AIxiv專欄接收通報逾2000多篇內容,涵蓋全球各大大學與企業的頂級實驗室,有效促進了學術交流與傳播。如果您有優秀的工作想要分享,歡迎投稿或聯絡報道。投稿信箱:liyazhou@jiqizhixin.com;zhaoyunfeng@jiqizhixin.com。
在三維產生建模的研究領域,現行的兩大類3D 表示方法要麼基於擬合能力不足的隱式解碼器,要不是缺乏清晰定義的空間結構難以與主流的3D 擴散技術融合。來自中科大、清華和微軟亞洲研究院的研究人員提出了 GaussianCube,這是一種具有強大擬合能力的顯式結構化三維表示,並且可以無縫應用於目前主流的 3D 擴散模型中。 GaussianCube 首先採用新穎的密度約束高斯擬合演算法,該演算法能夠對 3D 資產進行高精度擬合,同時確保使用固定數量的自由高斯。隨後,借助最優傳輸演算法,這些高斯被重新排列到一個預先定義的體素網格之中。由於 GaussianCube 的結構化特性,研究者無需複雜的網路設計就能直接應用標準的 3D U-Net 作為擴散建模的主幹網路。 更為關鍵的是,本文提出的新型擬合演算法大大增強了表示的緊湊性,在3D 表示擬合品質相似的情況下所需的參數量僅是傳統結構化表示所需參數量的十分之一或百分之一。這種緊湊性大幅降低了 3D 生成建模的複雜性。研究人員在無條件和條件性 3D 物件生成、數位化身創建以及文本到 3D 內容合成等多個方面開展了廣泛的實驗。 數值結果表明,GaussianCube 相較之前的基準演算法實現了最高 74% 的效能提升。如下所示,GaussianCube 不僅能夠產生高品質的三維資產,而且還提供了極具吸引力的視覺效果,充分證明了其作為 3D 生成通用表示的巨大潛力。
之後上使用 2. 以輸入肖像來建立數位化身的結果。本文的方法可以極大程度上保留輸入肖像的身份特徵信息,並提供細緻的髮型、服裝建模。 
時使用文字本文的方法可以輸出與文字資訊一致的結果,並且可以建模複雜的幾何結構和細節材質。 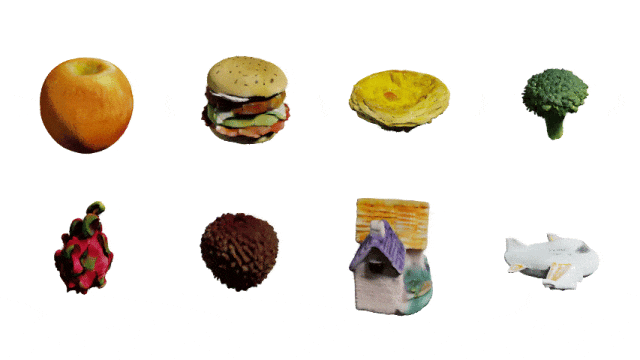
本文產生的三維資產語意明確,具有高品質的幾何結構和材質。
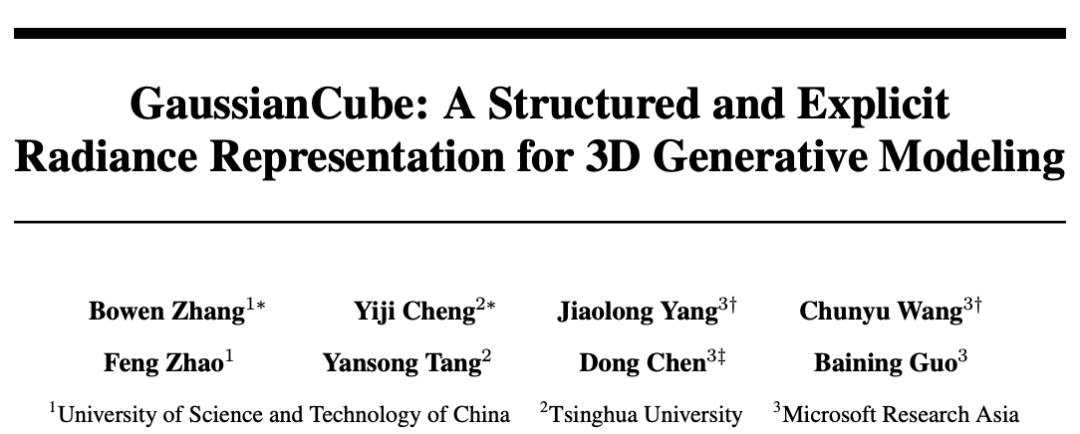
- 論文名稱:GaussianCube: A Structured and Explicit Radiance Representation for 3D Generative Modeling
- 專案首頁:https://gaussiancube.github.io/
- 論文連結:https://arxiv.org/pdf/2403.19655
- 程式碼開源:https://github.com/GaussianCube/ GaussianCube
- 示範影片:https://www.bilibili.com/video/BV1zy411h7wB/
大多數先前的3D 生成建模工作都使用了Neural Radiance Field (NeRF) 的變體作為其底層的3D表示,這些表示通常結合了一個明確的結構化特徵表示和一個隱式的特徵解碼器。然而在三維生成建模中,所有三維物體都必須共享同一個隱式特徵解碼器,這種做法在很大程度上削弱了 NeRF 的擬合能力。此外,NeRF 所依賴的體渲染技術具有非常高的運算複雜性,這導致了渲染速度緩慢,更需要消耗極高的 GPU 記憶體。近期,另一種三維表示方法高斯濺鍍(3D Gaussian Splatting,簡稱 3DGS)備受矚目。雖然 3DGS 擬合能力強大、運算效能高效,也具備完全顯式的特性,在三維重建任務中得到了廣泛應用。但是,3DGS 缺乏一個明確定義的空間結構,這使得其在無法直接應用於目前主流生成建模框架中。 因此,研究團隊提出了 GaussianCube。這是一種創新的三維表示方法,它既結構化又完全顯式,具備強大的擬合能力。本文介紹的方法首先確保透過固定數量的自由高斯實現高精度的擬合,然後將這些高斯有效地組織到一個結構化的體素網格中。這種顯式且結構化的表示方法讓研究者能夠無縫地採用標準的3D 網路架構,例如U-Net,無需進行使用非結構化或隱式解碼表示時所需的複雜和客製化的網絡設計。 同時,透過最優傳輸演算法進行的結構化組織最大程度地保持了相鄰高斯核之間的空間結構關係,使得研究者僅使用經典的3D 卷積網路就能有效率地提取特徵。更關鍵的是,鑑於先前研究中的發現,擴散模型在處理高維度資料分佈時表現不佳,本文提出的GaussianCube 在維持高品質重建的同時,顯著減少了所需的參數量,大大緩解了擴散模型在分佈建模上的壓力,為3D 生成建模領域帶來了顯著的建模能力和效率提升。 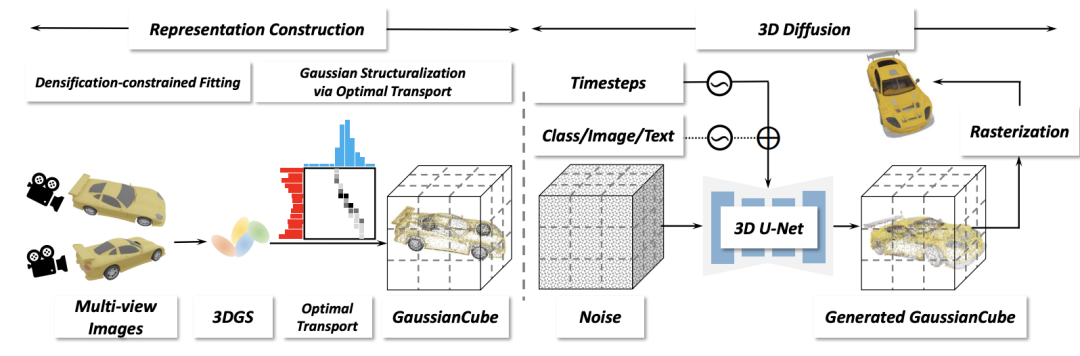
##本文的架構包括兩個主要階段:表示建構和三維擴散。在表示建構階段,給定三維資產的多視角渲染圖,對其進行密度約束的高斯擬合,以獲得具有固定數量的三維高斯。隨後,透過最優化傳輸將三維高斯結構化為 GaussianCube。在三維擴散階段,研究人員對三維擴散模型進行訓練,以便從高斯雜訊中產生 GaussianCube。 ##在表示建構階段,研究人員需要為每個三維資產創建適合生成建模的表示。考慮到生成領域往往需要建模的資料有統一的固定長度,而原始3DGS 擬合演算法中的自適應密度控制會導致擬合不同物體所使用的高斯核數量不同,這給生成建模帶來了極大挑戰。一個非常簡單的解決方案是直接去除自適應密度控制,但研究人員發現這會嚴重降低擬合的精確度。本文提出了一種新穎的密度約束擬合演算法,保留原始自適應密度控制中的剪枝操作,但對其中的分裂和克隆操作進行了新的約束處理。
具體來說,假設當前迭代包括
個高斯,研究人員透過選擇那些在視角空間位置梯度幅度超過預定義閾值τ 的高斯來識別分裂或克隆操作的候選對象,這些候選對象的數量記為
。為了防止超出預先定義的最大值個高斯,從候選物件中選擇
個具有最大視角空間位置梯度的高斯進行分割或克隆。在完成擬合過程後,研究人員以 α=0 的高斯填充以達到目標計數
而不影響渲染結果。由於此策略,可以實現了與類似品質的現有工作相比參數量減少了幾個量級的高品質表示,顯著降低了擴散模型的建模難度。 



#
The Gaussian obtained by the combined method still does not have a clear spatial arrangement structure, which makes subsequent diffusion models unable to efficiently model the data. To this end, researchers proposed to map Gaussians into a predefined structured voxel grid to make Gaussians have a clear spatial structure. Intuitively, the goal of this step is to “move” each Gaussian into a voxel while maintaining the spatial adjacency of the Gaussians as much as possible.
The researchers modeled it as an optimal transmission problem, used the Jonker-Volgenant algorithm to obtain the corresponding mapping relationship, and then organized the Gaussians are organized into corresponding voxels to obtain GaussianCube, and the position of the original Gaussian is replaced with the offset of the current voxel center to reduce the solution space of the diffusion model. The final GaussianCube representation is not only structured, but also maintains the structural relationship between adjacent Gaussians to the greatest extent, which provides strong support for efficient feature extraction for 3D generative modeling.
In the three-dimensional diffusion stage, this article uses a three-dimensional diffusion model to model the distribution of GaussianCube. Thanks to the spatially structured organization of GaussianCube, standard 3D convolution is sufficient to effectively extract and aggregate features of neighboring Gaussians without the need for complex network or training designs. Therefore, the researchers took advantage of standard U-Net network diffusion and directly replaced the original 2D operators (including convolution, attention, upsampling, and downsampling) with their 3D implementations.
The three-dimensional diffusion model of this article also supports a variety of condition signals to control the generation process, including category label condition generation, creating digital avatars based on image conditions, and generating three-dimensional digital assets based on text. . The generation capability based on multimodal conditions greatly expands the application scope of the model and provides a powerful tool for future 3D content creation. Experimental results
The researchers first verified GaussianCube on the ShapeNet Car data set fitting ability. Experimental results show that compared with baseline methods, GaussianCube can achieve high-precision three-dimensional object fitting at the fastest speed and with the smallest number of parameters. Table 1. Values of different three-dimensional representations on ShapeNet Car regarding spatial structure, fitting quality, relative fitting speed, and amount of parameters used Compare. ∗
Indicates that different objects share implicit feature decoders. All methods are evaluated with 30K iterations. 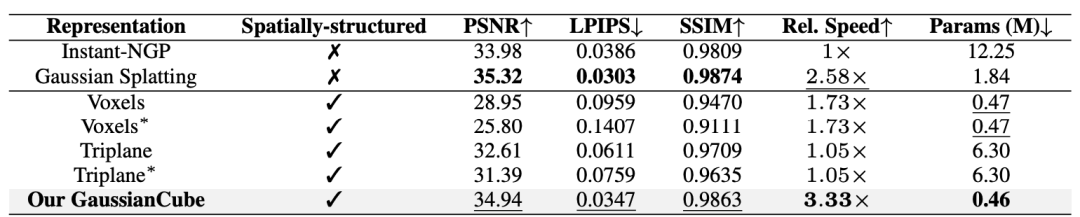
图 8. Different three -dimensional visual comparison on Shapenet CAR. ∗ indicates that different objects share implicit feature decoders. All methods are evaluated with 30K iterations.
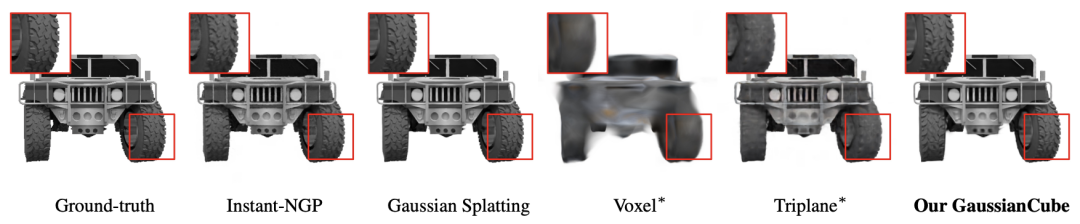
The researchers then verified the generation ability of the GaussianCube-based diffusion model on a large number of data sets, including ShapeNet, OmniObject3D, and synthetic digital avatar data sets. and Objaverse dataset. Experimental results show that our model achieves leading results in unconditional and category-conditional object generation, digital avatar creation, and text-to-3D synthesis, ranging from numerical metrics to visual quality. In particular, GaussianCube achieves a performance improvement of up to 74% compared to the previous baseline algorithm. 2 2. Do unconditional generation on Shapenet CAR, Chair, and quantitative comparison of category conditions on Omniobject3D. 
Figure 9. Qualitative comparison of unconditional generation on ShapeNet Car, Chair. The method in this article can generate accurate geometry and detailed materials. Figure 10. Qualitative comparison of category condition generation on OmniObject3D. This method can generate complex objects with clear semantics. Table 3. Quantitative comparison of digital avatar creation based on input portraits. Figure 11. Qualitative comparison of digital avatar creation based on input portrait. The method in this article can more accurately restore the identity features, expressions, accessories and hair details of the input portrait. 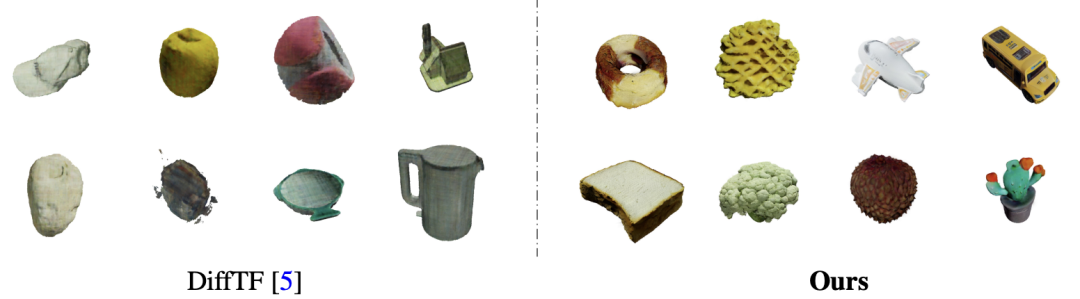
Table 4. Quantitative comparison of creating 3D assets based on input text. Inference time was tested using a single A100. Shap-E and LGM achieved similar CLIP Scores to the method in this article, but they respectively used millions of training data (this article only used 100,000 three-dimensional data for training), and a two-dimensional Vincentian graph diffusion model prior. 
图 12. Based on the qualitative comparison of creating three -dimensional assets in the input text. The method in this article can achieve high-quality 3D asset generation based on input text. 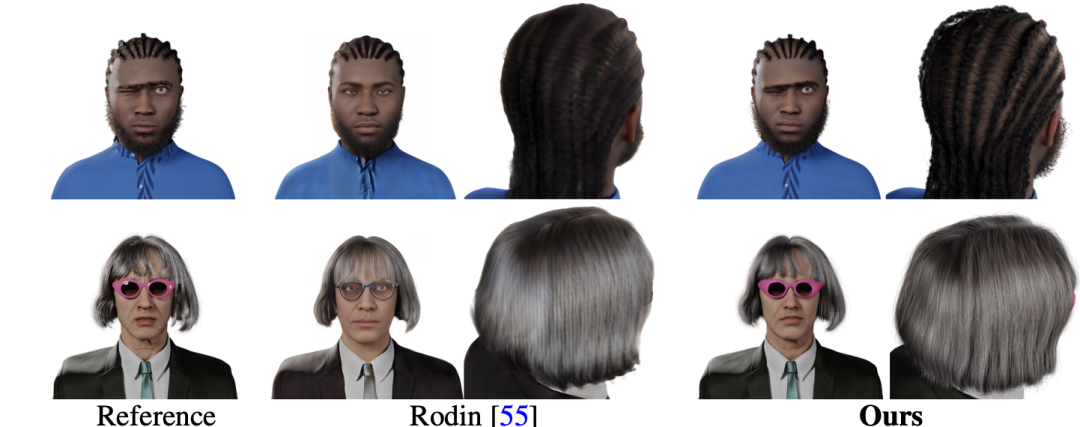
以上是高品質3D生成最有希望的一集? GaussianCube在三維生成中全面超越NeRF的詳細內容。更多資訊請關注PHP中文網其他相關文章!