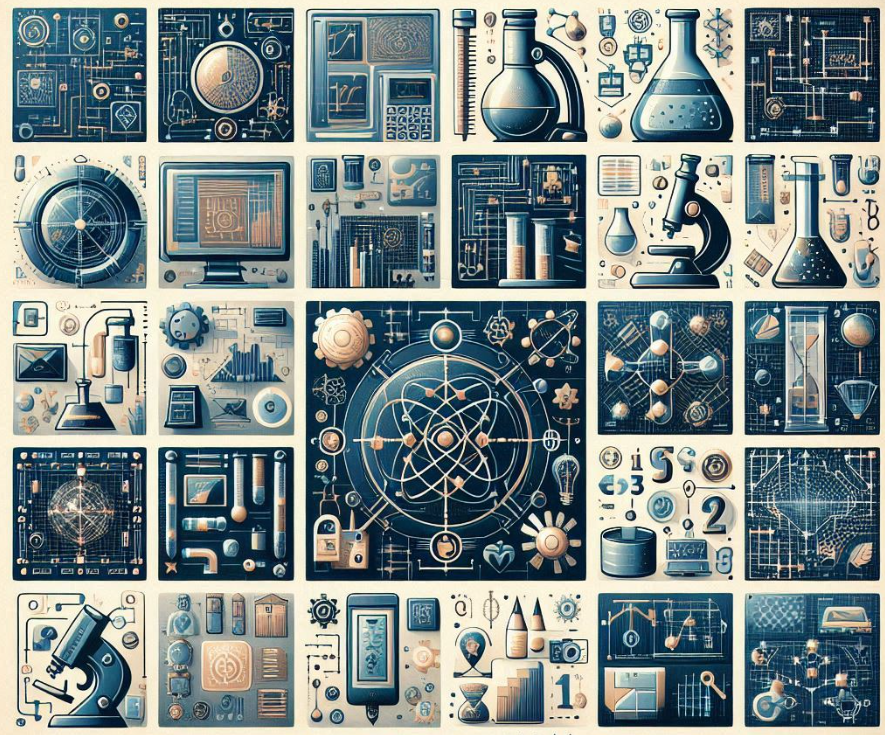
Editor | Radish skin dielectric materials can store and release charges and are widely used in capacitors, electronics and power systems. Due to their extremely high power density and fast response characteristics, they are used in fields such as hybrid electric vehicles, portable electronic devices and pulse power systems, but their energy density still needs to be further improved. High-entropy strategies have become an effective method to improve energy storage performance. However, discovering new high-entropy systems in high-dimensional composition spaces is a huge challenge for traditional trial-and-error experiments. Based on phase field simulations and limited experimental data, research teams from Wuhan University of Technology, Tsinghua University, and Pennsylvania State University proposed a generative learning method to accelerate the discovery of high-level learning in an infinite exploration space of more than
10^11combinations. Entropic dielectric materials (HED). This work provides an effective and innovative way to design high-entropy dielectric materials, significantly reducing the experimental cycle. The research was titled "Generative learning facilitated discovery of high-entropyceramic dielectrics for capacitive energy storage" and was published in "Nature Communications" on June 10, 2024.
Dielectric materials can store and release charges and are the core
component in capacitors. They are widely used in hybrid electric vehicles, portable electronic devices, and pulsed power systems due to their high power density and fast response characteristics. Additionally, dielectric materials are critical in modern electronic and power systems, supporting the
miniaturization
and high-efficiency operation of devices. However, traditional dielectric materials have limitations in energy density and thermal stability. The multi-entropy strategy can significantly improve these properties by introducing a variety of elements.
Illustration: Phase field simulation of the impact of configuration entropy (S
config) on energy storage performance. (Source: paper) High-entropy ceramics improve energy storage performance by forming diverse polarization structures with different valence states, ionic radii, and electronegativities, improving polarization response and breakdown strength. Currently, traditional experimental methods are inefficient and costly in discovering new high-entropy systems. To address these challenges, research teams from Tsinghua University, Wuhan University of Technology, and Pennsylvania State University built a generative learning-based framework based on small experimental data to accelerate the discovery of high energy density HED
. 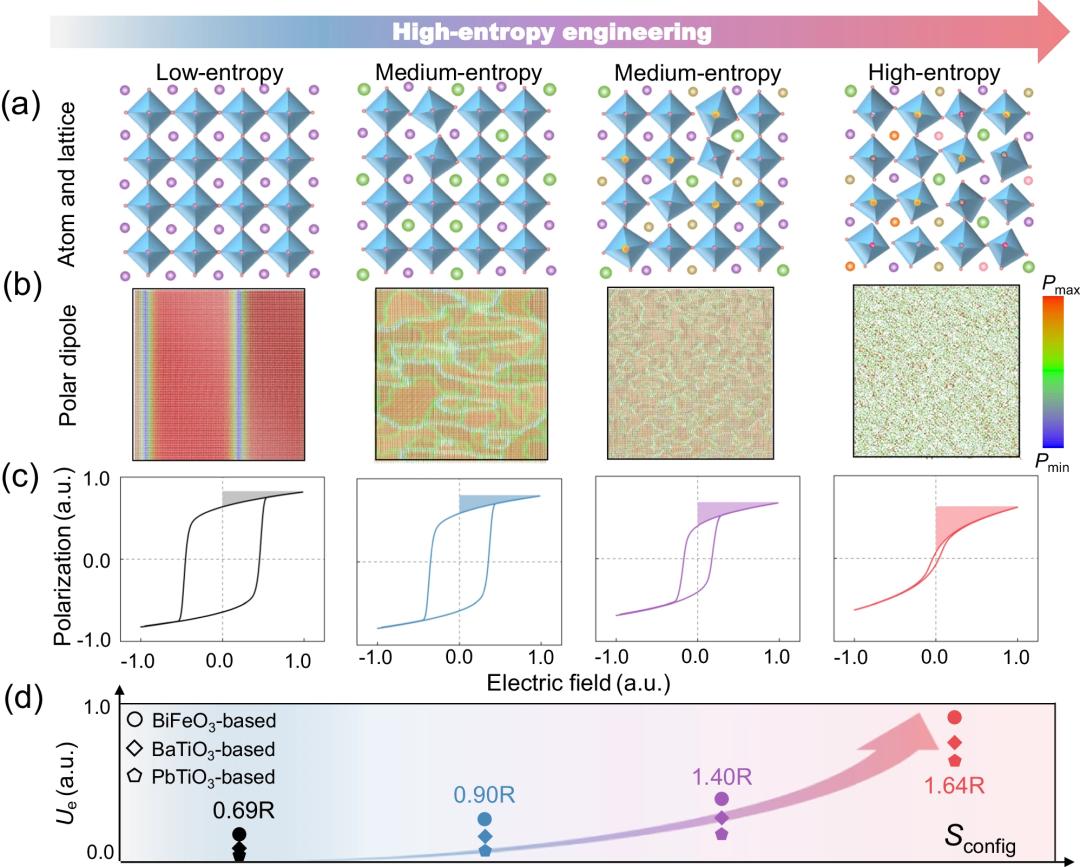
Illustration: Overview of the generative learning framework for high-entropy design. (Source: paper) In order to clarify the influence of configurational entropy on polarization response, the researchers performed phase field simulations to calculate the polarization-electric field (P-E) loop and corresponding energy density of HEDs with different entropy values. The results show that as the entropy value increases, the polarization region becomes more neutral and the energy density increases significantly. As an experimental example, the team selected Bi(Mg0.5Ti0.5)O3 (BMT) as the original matrix and designed the HED by simultaneously doping its A-site and B-site with multiple elements. Using 77 sets of experimental results as initial data, the researchers established a generative learning model based on encoding-decoding architecture, and combined data reconstruction and artificial neural network (ANN) to find potential optimal high-entropy combinations. Illustration: phase
structure
and electrical property analysis. (Source: paper) Then perform probability sampling on the existing small sample data, retain two decimal places for the element content of positions A and B, and set the sum of each position to 1 to construct a possibility of close to 10^11 combinations.
space
, looking for the optimal
combination that satisfies the high entropy criterion. Then, the top five combinations with predicted results were screened from more than 2,000 candidate materials, and five sets of targeted experiments were conducted to verify their potential in energy storage performance.
圖示:儲能效能和循環穩定性。 (資料來源:論文)實驗結果顯示,所製備的高熵陶瓷電介質薄膜在能量密度和擊穿強度方面均顯著優於傳統材料,特別是C-3薄膜在5104 kV/cm的電場下能量密度達到156 J/cm^3,是原始BMT(~18 J/cm^3)薄膜的八倍。此外,研究也探討了不同退火溫度對高熵薄膜性能的影響,發現適當的退火溫度能進一步提升材料的儲能特性。總而言之,高熵薄膜不僅具有優異的疲勞性能以及溫度和頻率穩定性,而且在儲能電容器中也顯示出廣泛應用的巨大潛力。基於機器學習驅動的模式,團隊利用非常稀疏的實驗數據有效地找到具有高儲能性能的所需高熵複合材料。該方法還使研究人員顯著縮短了整體實驗週期,並為設計具有複雜組件的材料系統開闢了新的途徑論文連結:https://www.nature.com/articles/s41467-024-49170-8
以上是比原始材料強8倍,清華、武漢理工團隊用AI篩選高熵電介質材料的詳細內容。更多資訊請關注PHP中文網其他相關文章!