重構 ReadmeGenie
Introduction
This week I was tasked to refactor the ReadmeGenie. If you just arrived here, ReadmeGenie is my open-source project that uses AI to generate readmes based on the files that the user inputs.
Initially, my thoughts were, "The program is working fine. I’ve been developing it in an organized way since day one... so why change it?"
Well, after taking a week-long break from the project, I opened it up again and immediately thought, "What is this?"
Why refactor?
To give you some context, here’s an example: One of my core functions, which I once thought was perfect, turned out to be much more complex than necessary. During the refactoring process, I broke it down into five separate functions—and guess what? The code is much cleaner and easier to manage now.
Take a look at the original version of this function:
def generate_readme(file_paths, api_key, base_url, output_filename, token_usage): try: load_dotenv() # Check if the api_key was provided either as an environment variable or as an argument if not api_key and not get_env(): logger.error(f"{Fore.RED}API key is required but not provided. Exiting.{Style.RESET_ALL}") sys.exit(1) # Concatenate content from multiple files file_content = "" try: for file_path in file_paths: with open(file_path, 'r') as file: file_content += file.read() + "\\n\\n" except FileNotFoundError as fnf_error: logger.error(f"{Fore.RED}File not found: {file_path}{Style.RESET_ALL}") sys.exit(1) # Get the base_url from arguments, environment, or use the default chosenModel = selectModel(base_url) try: if chosenModel == 'cohere': base_url = os.getenv("COHERE_BASE_URL", "https://api.cohere.ai/v1") response = cohereAPI(api_key, file_content) readme_content = response.generations[0].text.strip() + FOOTER_STRING else: base_url = os.getenv("GROQ_BASE_URL", "https://api.groq.com") response = groqAPI(api_key, base_url, file_content) readme_content = response.choices[0].message.content.strip() + FOOTER_STRING except AuthenticationError as auth_error: logger.error(f"{Fore.RED}Authentication failed: Invalid API key. Please check your API key and try again.{Style.RESET_ALL}") sys.exit(1) except Exception as api_error: logger.error(f"{Fore.RED}API request failed: {api_error}{Style.RESET_ALL}") sys.exit(1) # Process and save the generated README content if readme_content[0] != '*': readme_content = "\n".join(readme_content.split('\n')[1:]) try: with open(output_filename, 'w') as output_file: output_file.write(readme_content) logger.info(f"README.md file generated and saved as {output_filename}") logger.warning(f"This is your file's content:\n{readme_content}") except IOError as io_error: logger.error(f"{Fore.RED}Failed to write to output file: {output_filename}. Error: {io_error}{Style.RESET_ALL}") sys.exit(1) # Save API key if needed if not get_env() and api_key is not None: logger.warning("Would you like to save your API key and base URL in a .env file for future use? [y/n]") answer = input() if answer.lower() == 'y': create_env(api_key, base_url, chosenModel) elif get_env(): if chosenModel == 'cohere' and api_key != os.getenv("COHERE_API_KEY"): if api_key is not None: logger.warning("Would you like to save this API Key? [y/n]") answer = input() if answer.lower() == 'y': create_env(api_key, base_url, chosenModel) elif chosenModel == 'groq' and api_key != os.getenv("GROQ_API_KEY"): if api_key is not None: logger.warning("Would you like to save this API Key? [y/n]") answer = input() if answer.lower() == 'y': create_env(api_key, base_url, chosenModel) # Report token usage if the flag is set if token_usage: try: usage = response.usage logger.info(f"Token Usage Information: Prompt tokens: {usage.prompt_tokens}, Completion tokens: {usage.completion_tokens}, Total tokens: {usage.total_tokens}") except AttributeError: logger.warning(f"{Fore.YELLOW}Token usage information is not available for this response.{Style.RESET_ALL}") logger.info(f"{Fore.GREEN}File created successfully") sys.exit(0)
1. Eliminate Global Variables
Global variables can lead to unexpected side effects. Keep the state within the scope it belongs to, and pass values explicitly when necessary.
2. Use Functions for Calculations
Avoid storing intermediate values in variables where possible. Instead, use functions to perform calculations when needed—this keeps your code flexible and easier to debug.
3. Separate Responsibilities
A single function should do one thing, and do it well. Split tasks like command-line argument parsing, file reading, AI model management, and output generation into separate functions or classes. This separation allows for easier testing and modification in the future.
4. Improve Naming
Meaningful variable and function names are crucial. When revisiting your code after some time, clear names help you understand the flow without needing to re-learn everything.
5. Reduce Duplication
If you find yourself copying and pasting code, it’s a sign that you could benefit from shared functions or classes. Duplication makes maintenance harder, and small changes can easily result in bugs.
Commiting and pushing to GitHub
1. Create a branch
I started by creating a branch using:
git checkout -b <branch-name>
This command creates a new branch and switches to it.
2. Making a Series of Commits
Once on the new branch, I made incremental commits. Each commit represents a logical chunk of work, whether it was refactoring a function, fixing a bug, or adding a new feature. Making frequent, small commits helps track changes more effectively and makes it easier to review the history of the project.
git status git add <file_name> git commit -m "Refactored function"
3. Rebasing to Keep a Clean History
After making several commits, I rebased my branch to keep the history clean and linear. Rebasing allows me to reorder, combine, or modify commits before they are pushed to GitHub. This is especially useful if some of the commits are very small or if I want to avoid cluttering the commit history with too many incremental changes.
git rebase -i main
In this step, I initiated an interactive rebase on top of the main branch. The -i flag allows me to modify the commit history interactively. I could squash some of my smaller commits into one larger, cohesive commit. For instance, if I had a series of commits like:
Refactor part 1
Refactor part 2
Fix bug in refactor
I could squash them into a single commit with a clearer message
4. Pushing Changes to GitHub
Once I was satisfied with the commit history after the rebase, I pushed the changes to GitHub. If you’ve just created a new branch, you’ll need to push it to the remote repository with the -u flag, which sets the upstream branch for future pushes.
git push -u origin <branch-name>
5. Merging
In the last step I did a fast-forward merge to the main branch and pushed again
git checkout main # change to the main branch git merge --ff-only <branch-name> # make a fast-forward merge git push origin main # push to the main
Takeaways
Everything has room to improve. Refactoring may seem like a hassle, but it often results in cleaner, more maintainable, and more efficient code. So, the next time you feel hesitant about refactoring, remember: there’s always a better way to do things.
Even though I think it's perfect now, I will definitely have something to improve on my next commit.
以上是重構 ReadmeGenie的詳細內容。更多資訊請關注PHP中文網其他相關文章!

熱AI工具

Undresser.AI Undress
人工智慧驅動的應用程序,用於創建逼真的裸體照片

AI Clothes Remover
用於從照片中去除衣服的線上人工智慧工具。

Undress AI Tool
免費脫衣圖片

Clothoff.io
AI脫衣器

Video Face Swap
使用我們完全免費的人工智慧換臉工具,輕鬆在任何影片中換臉!

熱門文章

熱工具

記事本++7.3.1
好用且免費的程式碼編輯器

SublimeText3漢化版
中文版,非常好用

禪工作室 13.0.1
強大的PHP整合開發環境

Dreamweaver CS6
視覺化網頁開發工具

SublimeText3 Mac版
神級程式碼編輯軟體(SublimeText3)
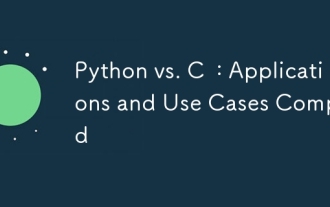
Python适合数据科学、Web开发和自动化任务,而C 适用于系统编程、游戏开发和嵌入式系统。Python以简洁和强大的生态系统著称,C 则以高性能和底层控制能力闻名。
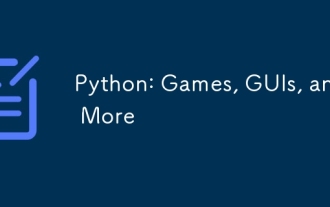
Python在遊戲和GUI開發中表現出色。 1)遊戲開發使用Pygame,提供繪圖、音頻等功能,適合創建2D遊戲。 2)GUI開發可選擇Tkinter或PyQt,Tkinter簡單易用,PyQt功能豐富,適合專業開發。
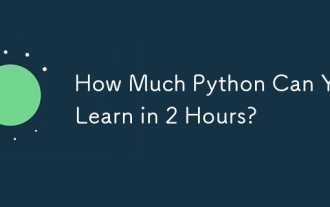
兩小時內可以學到Python的基礎知識。 1.學習變量和數據類型,2.掌握控制結構如if語句和循環,3.了解函數的定義和使用。這些將幫助你開始編寫簡單的Python程序。
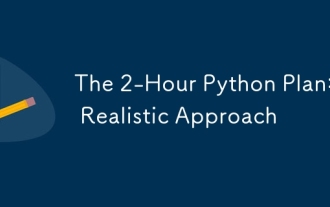
2小時內可以學會Python的基本編程概念和技能。 1.學習變量和數據類型,2.掌握控制流(條件語句和循環),3.理解函數的定義和使用,4.通過簡單示例和代碼片段快速上手Python編程。
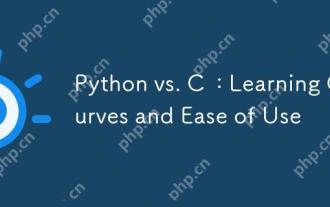
Python更易學且易用,C 則更強大但複雜。 1.Python語法簡潔,適合初學者,動態類型和自動內存管理使其易用,但可能導致運行時錯誤。 2.C 提供低級控制和高級特性,適合高性能應用,但學習門檻高,需手動管理內存和類型安全。
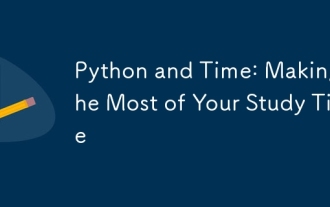
要在有限的時間內最大化學習Python的效率,可以使用Python的datetime、time和schedule模塊。 1.datetime模塊用於記錄和規劃學習時間。 2.time模塊幫助設置學習和休息時間。 3.schedule模塊自動化安排每週學習任務。
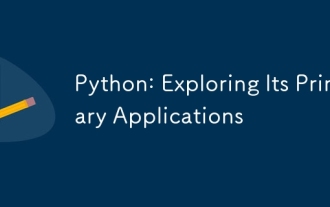
Python在web開發、數據科學、機器學習、自動化和腳本編寫等領域有廣泛應用。 1)在web開發中,Django和Flask框架簡化了開發過程。 2)數據科學和機器學習領域,NumPy、Pandas、Scikit-learn和TensorFlow庫提供了強大支持。 3)自動化和腳本編寫方面,Python適用於自動化測試和系統管理等任務。
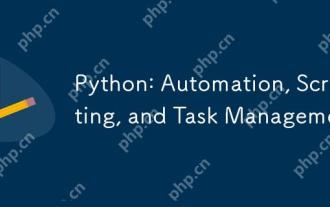
Python在自動化、腳本編寫和任務管理中表現出色。 1)自動化:通過標準庫如os、shutil實現文件備份。 2)腳本編寫:使用psutil庫監控系統資源。 3)任務管理:利用schedule庫調度任務。 Python的易用性和豐富庫支持使其在這些領域中成為首選工具。
