使用Pytorch構建變壓器模型的綜合指南
>本教程的目的是為如何使用Pytorch構建變壓器模型提供全面的理解。變壓器是現代機器學習中最強大的模型之一。他們徹底改變了該領域,特別是在自然語言處理(NLP)任務中,例如語言翻譯和文本摘要。長期的短期內存(LSTM)網絡由於能夠處理遠程依賴和並行計算的能力而被這些任務中的變壓器所取代。
構建變壓器指南中使用的工具是Pytorch,Pytorch是一個流行的開源機器學習庫,以其簡單,多功能性和效率而聞名。借助動態計算圖和廣泛的庫,Pytorch已成為機器學習和人工智能領域的研究人員和開發人員的首選。 對於那些不熟悉Pytorch的人來說,訪問Datacamp的課程,建議使用Pytorch進行深度學習介紹。 Vaswani等人所需要的全部所需的> Transformers之後,由於其獨特的設計和有效性,變形金剛已成為許多NLP任務的基石。
>。在變壓器的核心是注意機制,特別是“自我注意力”的概念,它允許模型稱重和優先級輸入數據。這種機制使變壓器能夠管理數據中的長期依賴性。從根本上講,這是一種加權方案,允許模型在產生輸出時專注於輸入的不同部分。
>這種機制允許模型考慮輸入序列中的不同單詞或特徵,分配每個單詞或一個“權重”,表示其對產生給定輸出的重要性。 例如,在句子翻譯任務中,在翻譯特定單詞的同時,該模型可能會將更高的注意力權重分配給語法或語義上與目標詞相關的單詞。這個過程允許變壓器在單詞或特徵之間捕獲依賴項,無論其序列與彼此之間的距離如何。
。變形金剛在NLP領域的影響不能被誇大。他們在許多任務中都表現出了傳統模型的表現,證明了以更細微的方式理解和產生人類語言的能力。
為了更深入地了解NLP,Datacamp在Python課程中的自然語言處理簡介是推薦的資源。設置Pytorch
>在構建變壓器之前,必須正確設置工作環境。首先,需要安裝Pytorch。 Pytorch(當前穩定版本-2.0.1)可以通過PIP或CONDA軟件包管理器輕鬆安裝。
對於PIP,請使用命令:
對於conda,請使用命令:
pip3 install torch torchvision torchaudio
>使用pytorch和cpu友善訪問pytorch文檔。
此外,對深度學習概念有基本的理解是有益的,因為這些理解對於理解變形金剛的操作至關重要。對於需要進修的人來說,python中的Datacamp課程深度學習是一個寶貴的資源,涵蓋了深度學習中的關鍵概念。>用pytorch
構建變壓器模型
要構建變壓器模型以下步驟是必要的:導入庫和模塊
- 定義基本構建塊 - 多頭注意力,位置饋送網絡,位置編碼
- 構建編碼器塊
- 構建解碼器塊
- >組合編碼器和解碼器層以創建完整的變壓器網絡
>我們將從導入Pytorch庫的核心功能,用於創建神經網絡的神經網絡模塊,用於培訓網絡的優化模塊以及用於處理數據的數據實用程序功能。此外,我們將導入用於數學操作的標準Python數學模塊和用於創建複雜對象副本的複制模塊。
這些工具為定義模型的體系結構,管理數據和建立培訓過程奠定了基礎。
2。定義基本構建塊:多頭注意,位置饋線網絡,位置編碼
conda install pytorch torchvision torchaudio pytorch-cuda=11.7 -c pytorch -c nvidia
多頭注意機制在序列中計算每對位置之間的注意力。它由捕獲輸入序列的不同方面的多個“注意力頭”組成。
要了解有關多頭注意的更多信息,請查看大語模型(LLMS)概念課程的此註意機制部分。
>
類定義和初始化:
import torch import torch.nn as nn import torch.optim as optim import torch.utils.data as data import math import copy
d_model:輸入的維度。
num_heads:將輸入拆分為。class MultiHeadAttention(nn.Module): def __init__(self, d_model, num_heads): super(MultiHeadAttention, self).__init__() # Ensure that the model dimension (d_model) is divisible by the number of heads assert d_model % num_heads == 0, "d_model must be divisible by num_heads" # Initialize dimensions self.d_model = d_model # Model's dimension self.num_heads = num_heads # Number of attention heads self.d_k = d_model // num_heads # Dimension of each head's key, query, and value # Linear layers for transforming inputs self.W_q = nn.Linear(d_model, d_model) # Query transformation self.W_k = nn.Linear(d_model, d_model) # Key transformation self.W_v = nn.Linear(d_model, d_model) # Value transformation self.W_o = nn.Linear(d_model, d_model) # Output transformation def scaled_dot_product_attention(self, Q, K, V, mask=None): # Calculate attention scores attn_scores = torch.matmul(Q, K.transpose(-2, -1)) / math.sqrt(self.d_k) # Apply mask if provided (useful for preventing attention to certain parts like padding) if mask is not None: attn_scores = attn_scores.masked_fill(mask == 0, -1e9) # Softmax is applied to obtain attention probabilities attn_probs = torch.softmax(attn_scores, dim=-1) # Multiply by values to obtain the final output output = torch.matmul(attn_probs, V) return output def split_heads(self, x): # Reshape the input to have num_heads for multi-head attention batch_size, seq_length, d_model = x.size() return x.view(batch_size, seq_length, self.num_heads, self.d_k).transpose(1, 2) def combine_heads(self, x): # Combine the multiple heads back to original shape batch_size, _, seq_length, d_k = x.size() return x.transpose(1, 2).contiguous().view(batch_size, seq_length, self.d_model) def forward(self, Q, K, V, mask=None): # Apply linear transformations and split heads Q = self.split_heads(self.W_q(Q)) K = self.split_heads(self.W_k(K)) V = self.split_heads(self.W_v(V)) # Perform scaled dot-product attention attn_output = self.scaled_dot_product_attention(Q, K, V, mask) # Combine heads and apply output transformation output = self.W_o(self.combine_heads(attn_output)) return output
初始化檢查d_model是否可以由num_heads除外,然後定義查詢,鍵,值和輸出的轉換權重。
- >計算注意分數:attn_scores = torch.matmul(q,k.transpose(-2,-1)) / Math.sqrt(self.d_k)。在這裡,注意分數是通過取查詢(q)和鍵(k)的點乘積來計算的,然後按鍵維(d_k)的平方根進行縮放。
> 施加蒙版:如果提供了掩碼,則將其應用於注意分數以掩蓋特定的值。
- >計算注意力的權重:注意分數通過SoftMax函數傳遞,以將其轉換為總和為1的概率 >計算輸出:通過將注意力權重乘以(v)。
- 拆分頭:
- 應用輸出轉換:最後,組合張量通過輸出線性轉換。 總而言之,
- 多頭類別封裝了變壓器模型中常用的多頭注意機制。它需要將輸入分為多個注意力頭,將注意力集中在每個頭上,然後將結果組合在一起。通過這樣做,模型可以在不同尺度的輸入數據中捕獲各種關係,從而提高模型的表達能力。 >位置饋電網絡
- 類定義:
- > d_model:模型輸入和輸出的維度。
> d_ff:饋送網絡中內層的維度。 - > self.fc1和self.fc2:兩個完全連接的(線性)層,具有輸入和輸出尺寸,由d_model和d_ff定義
- self.relu:relu(rectified Linear單元)激活函數,該功能引入了兩個線性層之間的非線性。
- self.fc1(x):輸入首先通過第一個線性層(FC1)。 self.relu(...):然後通過Relu激活函數傳遞FC1的輸出。 relu用零替換所有負值,將非線性引入模型。
- self.fc2(...):然後,激活的輸出通過第二線性層(FC2),產生最終輸出。 總而言之,positionWiseFeedForward類定義了由位置的饋電神經網絡,該網絡由兩個線性層組成,其中介於兩者之間。在變壓器模型的背景下,該饋送向前網絡分別且相同地應用於每個位置。它有助於改變變壓器內的注意機制所學的功能,作為註意輸出的附加處理步驟。
- >位置編碼
- >位置編碼用於在輸入序列中註入每個令牌的位置信息。它使用不同頻率的正弦和余弦函數來生成位置編碼。
- forward方法:
- 正向方法只需將位置編碼添加到輸入x。
- >它使用第一個X.Size(1)PE的元素來確保位置編碼匹配x。 的實際序列長度
- 摘要
-
位置編碼類添加了有關令牌在序列中的位置的信息。由於變壓器模型缺乏對代幣順序的固有知識(由於其自我發揮機制),因此該類別有助於該模型考慮令牌在序列中的位置。選擇使用的正弦函數以使模型可以輕鬆學習到相對位置,因為它們為序列中的每個位置都產生獨特而光滑的編碼。
3。構建編碼器塊pip3 install torch torchvision torchaudio
登入後複製登入後複製登入後複製登入後複製登入後複製登入後複製登入後複製登入後複製登入後複製該類被定義為Pytorch的Nn.模塊的子類,這意味著它可以用作Pytorch中神經網絡的構建塊。
初始化:conda install pytorch torchvision torchaudio pytorch-cuda=11.7 -c pytorch -c nvidia
登入後複製登入後複製登入後複製登入後複製登入後複製登入後複製登入後複製登入後複製>
參數:
import torch import torch.nn as nn import torch.optim as optim import torch.utils.data as data import math import copy
登入後複製登入後複製登入後複製登入後複製登入後複製登入後複製登入後複製d_model:輸入的維度。 num_heads:多頭注意力中註意力的數量。
>- d_ff:在位置前饋網絡中內部層的維度。
- 輟學:用於正則化的輟學率。
- >組件:
- >
self.self_attn:多頭注意機制。 self.feed_forward:位置上的饋送神經網絡。
- self.norm1和self.norm2:層歸一化,用於平滑層的輸入。
- self.dropout:輟學層,用於防止過度擬合,通過在訓練過程中隨機設置一些激活為零。
- forward方法:
>輸入:>
x:ecdoder層的輸入。class MultiHeadAttention(nn.Module): def __init__(self, d_model, num_heads): super(MultiHeadAttention, self).__init__() # Ensure that the model dimension (d_model) is divisible by the number of heads assert d_model % num_heads == 0, "d_model must be divisible by num_heads" # Initialize dimensions self.d_model = d_model # Model's dimension self.num_heads = num_heads # Number of attention heads self.d_k = d_model // num_heads # Dimension of each head's key, query, and value # Linear layers for transforming inputs self.W_q = nn.Linear(d_model, d_model) # Query transformation self.W_k = nn.Linear(d_model, d_model) # Key transformation self.W_v = nn.Linear(d_model, d_model) # Value transformation self.W_o = nn.Linear(d_model, d_model) # Output transformation def scaled_dot_product_attention(self, Q, K, V, mask=None): # Calculate attention scores attn_scores = torch.matmul(Q, K.transpose(-2, -1)) / math.sqrt(self.d_k) # Apply mask if provided (useful for preventing attention to certain parts like padding) if mask is not None: attn_scores = attn_scores.masked_fill(mask == 0, -1e9) # Softmax is applied to obtain attention probabilities attn_probs = torch.softmax(attn_scores, dim=-1) # Multiply by values to obtain the final output output = torch.matmul(attn_probs, V) return output def split_heads(self, x): # Reshape the input to have num_heads for multi-head attention batch_size, seq_length, d_model = x.size() return x.view(batch_size, seq_length, self.num_heads, self.d_k).transpose(1, 2) def combine_heads(self, x): # Combine the multiple heads back to original shape batch_size, _, seq_length, d_k = x.size() return x.transpose(1, 2).contiguous().view(batch_size, seq_length, self.d_model) def forward(self, Q, K, V, mask=None): # Apply linear transformations and split heads Q = self.split_heads(self.W_q(Q)) K = self.split_heads(self.W_k(K)) V = self.split_heads(self.W_v(V)) # Perform scaled dot-product attention attn_output = self.scaled_dot_product_attention(Q, K, V, mask) # Combine heads and apply output transformation output = self.W_o(self.combine_heads(attn_output)) return output
登入後複製登入後複製登入後複製登入後複製登入後複製>蒙版:可選的掩碼以忽略輸入的某些部分。
- 處理步驟:
- 自我注意力:輸入X通過多頭自我注意的機制傳遞。
>前進網絡:上一個步驟的輸出通過位置饋線向前網絡傳遞。 添加&歸一化(進率後):類似於步驟2,將饋送輸出添加到此階段的輸入(殘留連接),然後使用norm2。
>輸出:返回處理後的張量作為編碼層的輸出。- 摘要:
-
4。構建解碼器塊 類定義:pip3 install torch torchvision torchaudio
登入後複製登入後複製登入後複製登入後複製登入後複製登入後複製登入後複製登入後複製登入後複製初始化:conda install pytorch torchvision torchaudio pytorch-cuda=11.7 -c pytorch -c nvidia
登入後複製登入後複製登入後複製登入後複製登入後複製登入後複製登入後複製登入後複製>
參數:import torch import torch.nn as nn import torch.optim as optim import torch.utils.data as data import math import copy
登入後複製登入後複製登入後複製登入後複製登入後複製登入後複製登入後複製d_model:輸入的維度。
- num_heads:多頭注意力中註意力的數量。
- > > d_ff:進料向前網絡中內層的維度。
- > 輟學:正規化的輟學率。
- 組件
self.self_attn:目標序列的多頭自我注意機制。
self.cross_attn:參與編碼器輸出的多頭注意機制。- self.feed_forward:位置上的饋送神經網絡。
- self.norm1,self.norm2,self.norm3:層標準化組件。
- self.dropout:正規化的輟學層。
:
>輸入: x:解碼器層的輸入。class MultiHeadAttention(nn.Module): def __init__(self, d_model, num_heads): super(MultiHeadAttention, self).__init__() # Ensure that the model dimension (d_model) is divisible by the number of heads assert d_model % num_heads == 0, "d_model must be divisible by num_heads" # Initialize dimensions self.d_model = d_model # Model's dimension self.num_heads = num_heads # Number of attention heads self.d_k = d_model // num_heads # Dimension of each head's key, query, and value # Linear layers for transforming inputs self.W_q = nn.Linear(d_model, d_model) # Query transformation self.W_k = nn.Linear(d_model, d_model) # Key transformation self.W_v = nn.Linear(d_model, d_model) # Value transformation self.W_o = nn.Linear(d_model, d_model) # Output transformation def scaled_dot_product_attention(self, Q, K, V, mask=None): # Calculate attention scores attn_scores = torch.matmul(Q, K.transpose(-2, -1)) / math.sqrt(self.d_k) # Apply mask if provided (useful for preventing attention to certain parts like padding) if mask is not None: attn_scores = attn_scores.masked_fill(mask == 0, -1e9) # Softmax is applied to obtain attention probabilities attn_probs = torch.softmax(attn_scores, dim=-1) # Multiply by values to obtain the final output output = torch.matmul(attn_probs, V) return output def split_heads(self, x): # Reshape the input to have num_heads for multi-head attention batch_size, seq_length, d_model = x.size() return x.view(batch_size, seq_length, self.num_heads, self.d_k).transpose(1, 2) def combine_heads(self, x): # Combine the multiple heads back to original shape batch_size, _, seq_length, d_k = x.size() return x.transpose(1, 2).contiguous().view(batch_size, seq_length, self.d_model) def forward(self, Q, K, V, mask=None): # Apply linear transformations and split heads Q = self.split_heads(self.W_q(Q)) K = self.split_heads(self.W_k(K)) V = self.split_heads(self.W_v(V)) # Perform scaled dot-product attention attn_output = self.scaled_dot_product_attention(Q, K, V, mask) # Combine heads and apply output transformation output = self.W_o(self.combine_heads(attn_output)) return output
登入後複製登入後複製登入後複製登入後複製登入後複製> enc_output:來自相應的encoder的輸出(在跨注意步驟中使用)。
> src_mask:源蒙版忽略了編碼器輸出的某些部分。- > tgt_mask:目標蒙版忽略了解碼器輸入的某些部分。
- 處理步驟:
-
- 對目標序列的自我注意:輸入X是通過自我注意的機制來處理的。
- 添加和歸一化(自我注意力之後):自我注意的輸出被添加到原始X中,然後使用Norm1。 與編碼器輸出的交叉注意:上一步的歸一化輸出是通過跨意義機制處理的,該機制可用於Encoder的輸出ENC_OUTPUT。 添加和歸一化(跨注意事後):跨注意的輸出添加到此階段的輸入中,然後使用Norm2進行輟學和歸一化。
- >前進網絡:上一個步驟的輸出通過饋電網絡傳遞。 添加和歸一化(進率後):將進紙輸出輸出添加到此階段的輸入中,然後使用Norm3進行輟學和歸一化。
- >輸出:返回處理後的張量作為解碼器層的輸出。
- 摘要:
- 解碼器類定義了變壓器解碼器的單層。它由多頭自我發揮機制,一種多頭跨注意機制(符合編碼器的輸出),位置饋送前向前向神經網絡以及相應的殘留連接,層歸一化和輟學層組成。這種組合使解碼器可以根據目標序列和源序列來基於編碼器的表示產生有意義的輸出。與編碼器一樣,通常將多個解碼器層堆疊以形成變壓器模型的完整解碼器部分。 接下來,將編碼器和解碼器塊匯總在一起以構建綜合變壓器模型。
>
類定義:
初始化:
構造函數採用以下參數:> >pip3 install torch torchvision torchaudio
登入後複製登入後複製登入後複製登入後複製登入後複製登入後複製登入後複製登入後複製登入後複製> src_vocab_size:源詞彙大小。
> tgt_vocab_size:目標詞彙大小。conda install pytorch torchvision torchaudio pytorch-cuda=11.7 -c pytorch -c nvidia
登入後複製登入後複製登入後複製登入後複製登入後複製登入後複製登入後複製登入後複製d_model:模型嵌入的尺寸。 > num_heads:多頭注意機制中註意力頭的數量。
num_layers:編碼器和解碼器的層數。import torch import torch.nn as nn import torch.optim as optim import torch.utils.data as data import math import copy
登入後複製登入後複製登入後複製登入後複製登入後複製登入後複製登入後複製- >
- max_seq_length:位置編碼的最大序列長度。
- 輟學:正規化的輟學率。
- >它定義了以下組件:
- >
- self.encoder_embedding:源序列的嵌入層。
- self.decoder_embedding:目標序列的嵌入層。
- self.positional_encoding:位置編碼組件。
- self.encoder_layers:編碼層的列表。 >
- self.decoder_layers:解碼器層列表。
- self.fc:最終完全連接(線性)層映射到目標詞彙大小。
- self.dropout:輟學層。
生成蒙版方法:
該方法用於為源和目標序列創建掩碼,以確保忽略填充令牌,並且在訓練目標序列的訓練過程中看不到未來的令牌。> pip3 install torch torchvision torchaudio
登入後複製登入後複製登入後複製登入後複製登入後複製登入後複製登入後複製登入後複製登入後複製forward方法:
此方法定義了變壓器的正向通行證,採用源和目標序列並產生輸出預測。
>輸入嵌入和位置編碼:首先使用其各自的嵌入層嵌入源和目標序列,然後添加到其位置編碼中。conda install pytorch torchvision torchaudio pytorch-cuda=11.7 -c pytorch -c nvidia
登入後複製登入後複製登入後複製登入後複製登入後複製登入後複製登入後複製登入後複製>編碼器層:源序列通過編碼層傳遞,最終的編碼器輸出代表已處理的源序列。
>解碼器層:目標序列和編碼器的輸出通過解碼器層傳遞,從而導致解碼器的輸出。- 最終線性層:解碼器的輸出使用完全連接的(線性)層映射到目標詞彙大小。
- 輸出:
- 最終輸出是代表模型對目標序列的預測的張量。
變壓器類將變壓器模型的各個組件匯總在一起,包括嵌入,位置編碼,編碼器層和解碼器層。它提供了一個方便的界面,用於訓練和推理,封裝了多頭關注,進率向前網絡和層歸一化的複雜性。
>>此實現遵循標準變壓器體系結構,使其適合於序列到序列任務,例如機器翻譯,文本摘要等。包含掩蔽可確保該模型遵守序列中的因果關係,忽略填充令牌,並防止未來的代幣洩漏。
這些順序步驟使變壓器模型有效地處理輸入序列並產生相應的輸出序列。訓練Pytorch變壓器模型 樣本數據準備
出於說明目的,將在此示例中製作一個虛擬數據集。但是,在實際情況下,將採用更實質性的數據集,並且該過程將涉及文本預處理以及為源和目標語言創建詞彙映射。pip3 install torch torchvision torchaudio
登入後複製登入後複製登入後複製登入後複製登入後複製登入後複製登入後複製登入後複製登入後複製超參數:
這些值定義了變壓器模型的體系結構和行為:
- > src_vocab_size,tgt_vocab_size:源和目標序列的詞彙大小,都設置為5000。
d_model:模型嵌入的維度,設置為512。 - d_ff:饋線網絡中內層的維度,設置為2048。
>- max_seq_length:位置編碼的最大序列長度,設置為100。
輟學:正規化的輟學率,設置為0.1。- 創建一個變壓器實例:
>此行創建了變壓器類的實例,並用給定的超參數初始化它。該實例將具有這些超參數定義的架構和行為。 >
生成隨機示例數據:conda install pytorch torchvision torchaudio pytorch-cuda=11.7 -c pytorch -c nvidia
登入後複製登入後複製登入後複製登入後複製登入後複製登入後複製登入後複製登入後複製>
以下幾行生成隨機源和目標序列:> src_data:1和src_vocab_size之間的隨機整數,代表具有形狀的一批源序列(64,max_seq_length)。
> tgt_data:1和tgt_vocab_size之間的隨機整數,代表具有形狀的一批目標序列(64,max_seq_length)。
- 這些隨機序列可以用作變壓器模型的輸入,模擬了一批具有64個示例和長度序列的數據。
- 摘要:
- >代碼段演示瞭如何初始化變壓器模型並生成可以饋入模型的隨機源和目標序列。所選的超參數確定變壓器的特定結構和特性。此設置可能是較大腳本的一部分,其中對模型進行了對實際順序到序列任務進行訓練和評估,例如機器翻譯或文本摘要。
訓練模型 接下來,將使用上述樣本數據訓練該模型。但是,在現實世界中,將採用更大的數據集,通常將其劃分為不同的集合,以進行培訓和驗證目的。
>損失功能和優化器:
>>
優化器= optim.Adam(...):將優化器定義為ADAM,學習率為0.0001和特定的beta值。import torch import torch.nn as nn import torch.optim as optim import torch.utils.data as data import math import copy
登入後複製登入後複製登入後複製登入後複製登入後複製登入後複製登入後複製模型訓練模式:
- > transformer.train():將變壓器模型設置為訓練模式,從而實現只有在訓練期間適用的輟學等行為。
訓練環:
代碼代碼使用典型的訓練循環訓練100個時期的模型:
- 範圍(100)的時期:迭代100多個訓練時期。 態 output = transformer(src_data,tgt_data [:,:-1]):通過變壓器傳遞源數據和目標數據(每個序列中的最後一個令牌)。這在序列到序列任務中很常見,其中目標通過一個令牌移動。
- 損失=標準(...):計算模型的預測與目標數據之間的損失(不包括每個序列中的第一個令牌)。通過將數據重塑為一維張量並使用交叉滲透損失函數來計算損失。
- lose.backward():計算相對於模型參數的損失梯度。
- > importizer.step():使用計算的梯度更新模型的參數。
- print(f“ epoch:{epoch 1},損失:{loss.item()}”):打印當前的時期數和該時代的損失值。
- 摘要:
此代碼片段在100個時期的隨機生成源和目標序列上訓練變壓器模型。它使用ADAM優化器和橫向滲透損失函數。每個時期都打印損失,使您可以監視培訓進度。在現實世界中,您將用任務中的實際數據替換隨機源和目標序列,例如機器翻譯。 >變壓器模型性能評估
訓練模型後,可以在驗證數據集或測試數據集上評估其性能。以下是如何完成此操作的一個示例:
>評估模式:
pip3 install torch torchvision torchaudio
登入後複製登入後複製登入後複製登入後複製登入後複製登入後複製登入後複製登入後複製登入後複製transformer.eval():將變壓器模型置於評估模式。這很重要,因為它關閉了僅在訓練期間使用的某些行為(例如輟學)。
- 生成隨機驗證數據:
val_src_data:1和src_vocab_size之間的隨機整數,代表具有形狀的一批驗證源序列(64,max_seq_length)。 val_tgt_data:1和tgt_vocab_size之間的隨機整數,代表具有形狀的一批驗證目標序列(64,max_seq_length)。
- >驗證環:
-
>:禁用梯度計算,因為我們不需要在驗證過程中計算梯度。這可以減少記憶消耗並加快計算加速。
- > val_output =變形金剛(val_src_data,val_tgt_data [:,: - 1]):通過變壓器傳遞驗證源數據和驗證源數據和驗證目標數據(每個順序中的最後一個令牌)。 > val_loss = Criterion(...):計算模型的預測與驗證目標數據之間的損失(不包括每個序列中的第一個令牌)。通過將數據重塑為一維張量並使用先前定義的跨透明損失函數來計算損失。
- print(f“驗證損失:{val_loss.item()}”):打印驗證損失值。
- >
摘要:
此代碼段評估隨機生成的驗證數據集上的變壓器模型,計算驗證損失並打印它。在實際情況下,應從您正在處理的任務中替換隨機驗證數據。驗證損失可以使您表明您的模型在看不見的數據上的性能,這是對模型概括能力的關鍵衡量。>有關變壓器和擁抱面孔的更多詳細信息,我們的教程,使用變壓器和擁抱面的介紹是有用的。
結論和進一步的資源總之,該教程演示瞭如何使用Pytorch構建變壓器模型,Pytorch是最通用的深度學習工具之一。憑藉其並行的能力和捕獲數據中的長期依賴性的能力,變形金剛在各個領域都具有巨大的潛力,尤其是NLP任務,例如翻譯,摘要和情感分析。
渴望加深對先進深度學習概念和技術的理解的人,請考慮使用Datacamp上的Keras探索課程。您還可以在單獨的教程中使用Pytorch構建簡單的神經網絡。 >獲得頂級AI認證>證明您可以有效,負責任地使用AI。
pip3 install torch torchvision torchaudio
此方法將輸入X重塑為形狀(batch_size,num_heads,seq_length,d_k)。它使模型能夠同時處理多個注意力頭,從而可以進行並行計算。
組合頭:
conda install pytorch torchvision torchaudio pytorch-cuda=11.7 -c pytorch -c nvidia
分別將注意力應用於每個頭部後,此方法將結果結合回形狀的單個張量(batch_size,seq_length,d_model)。這為進一步處理的結果做準備。
forward方法:
import torch import torch.nn as nn import torch.optim as optim import torch.utils.data as data import math import copy
正向方法是實際計算發生的地方:
應用線性轉換:首先使用初始化中定義的權重通過線性轉換。 拆分頭:使用split_heads方法將變換後的Q,K,V分為多個頭。
class MultiHeadAttention(nn.Module): def __init__(self, d_model, num_heads): super(MultiHeadAttention, self).__init__() # Ensure that the model dimension (d_model) is divisible by the number of heads assert d_model % num_heads == 0, "d_model must be divisible by num_heads" # Initialize dimensions self.d_model = d_model # Model's dimension self.num_heads = num_heads # Number of attention heads self.d_k = d_model // num_heads # Dimension of each head's key, query, and value # Linear layers for transforming inputs self.W_q = nn.Linear(d_model, d_model) # Query transformation self.W_k = nn.Linear(d_model, d_model) # Key transformation self.W_v = nn.Linear(d_model, d_model) # Value transformation self.W_o = nn.Linear(d_model, d_model) # Output transformation def scaled_dot_product_attention(self, Q, K, V, mask=None): # Calculate attention scores attn_scores = torch.matmul(Q, K.transpose(-2, -1)) / math.sqrt(self.d_k) # Apply mask if provided (useful for preventing attention to certain parts like padding) if mask is not None: attn_scores = attn_scores.masked_fill(mask == 0, -1e9) # Softmax is applied to obtain attention probabilities attn_probs = torch.softmax(attn_scores, dim=-1) # Multiply by values to obtain the final output output = torch.matmul(attn_probs, V) return output def split_heads(self, x): # Reshape the input to have num_heads for multi-head attention batch_size, seq_length, d_model = x.size() return x.view(batch_size, seq_length, self.num_heads, self.d_k).transpose(1, 2) def combine_heads(self, x): # Combine the multiple heads back to original shape batch_size, _, seq_length, d_k = x.size() return x.transpose(1, 2).contiguous().view(batch_size, seq_length, self.d_model) def forward(self, Q, K, V, mask=None): # Apply linear transformations and split heads Q = self.split_heads(self.W_q(Q)) K = self.split_heads(self.W_k(K)) V = self.split_heads(self.W_v(V)) # Perform scaled dot-product attention attn_output = self.scaled_dot_product_attention(Q, K, V, mask) # Combine heads and apply output transformation output = self.W_o(self.combine_heads(attn_output)) return output
>
>組合頭:使用combine_heads方法將每個頭的結果組合回單個張量。類是Pytorch的NN.Module的子類,這意味著它將繼承使用神經網絡層所需的所有功能。
初始化:
>pip3 install torch torchvision torchaudio
conda install pytorch torchvision torchaudio pytorch-cuda=11.7 -c pytorch -c nvidia
類定義:
該類被定義為Pytorch的NN.模塊的子類,允許將其用作標準的Pytorch層。
import torch import torch.nn as nn import torch.optim as optim import torch.utils.data as data import math import copy
>
d_model:模型輸入的尺寸。class MultiHeadAttention(nn.Module): def __init__(self, d_model, num_heads): super(MultiHeadAttention, self).__init__() # Ensure that the model dimension (d_model) is divisible by the number of heads assert d_model % num_heads == 0, "d_model must be divisible by num_heads" # Initialize dimensions self.d_model = d_model # Model's dimension self.num_heads = num_heads # Number of attention heads self.d_k = d_model // num_heads # Dimension of each head's key, query, and value # Linear layers for transforming inputs self.W_q = nn.Linear(d_model, d_model) # Query transformation self.W_k = nn.Linear(d_model, d_model) # Key transformation self.W_v = nn.Linear(d_model, d_model) # Value transformation self.W_o = nn.Linear(d_model, d_model) # Output transformation def scaled_dot_product_attention(self, Q, K, V, mask=None): # Calculate attention scores attn_scores = torch.matmul(Q, K.transpose(-2, -1)) / math.sqrt(self.d_k) # Apply mask if provided (useful for preventing attention to certain parts like padding) if mask is not None: attn_scores = attn_scores.masked_fill(mask == 0, -1e9) # Softmax is applied to obtain attention probabilities attn_probs = torch.softmax(attn_scores, dim=-1) # Multiply by values to obtain the final output output = torch.matmul(attn_probs, V) return output def split_heads(self, x): # Reshape the input to have num_heads for multi-head attention batch_size, seq_length, d_model = x.size() return x.view(batch_size, seq_length, self.num_heads, self.d_k).transpose(1, 2) def combine_heads(self, x): # Combine the multiple heads back to original shape batch_size, _, seq_length, d_k = x.size() return x.transpose(1, 2).contiguous().view(batch_size, seq_length, self.d_model) def forward(self, Q, K, V, mask=None): # Apply linear transformations and split heads Q = self.split_heads(self.W_q(Q)) K = self.split_heads(self.W_k(K)) V = self.split_heads(self.W_v(V)) # Perform scaled dot-product attention attn_output = self.scaled_dot_product_attention(Q, K, V, mask) # Combine heads and apply output transformation output = self.W_o(self.combine_heads(attn_output)) return output
pe:一個充滿零的張量,將用位置編碼填充。
位置:一個張量,包含序列中每個位置的位置索引。
class MultiHeadAttention(nn.Module): def __init__(self, d_model, num_heads):
- >最後,PE被註冊為緩衝區,這意味著它將成為模塊狀態的一部分,但不會被視為可訓練的參數。
以上是使用Pytorch構建變壓器模型的綜合指南的詳細內容。更多資訊請關注PHP中文網其他相關文章!

熱AI工具

Undresser.AI Undress
人工智慧驅動的應用程序,用於創建逼真的裸體照片

AI Clothes Remover
用於從照片中去除衣服的線上人工智慧工具。

Undress AI Tool
免費脫衣圖片

Clothoff.io
AI脫衣器

Video Face Swap
使用我們完全免費的人工智慧換臉工具,輕鬆在任何影片中換臉!

熱門文章

熱工具

記事本++7.3.1
好用且免費的程式碼編輯器

SublimeText3漢化版
中文版,非常好用

禪工作室 13.0.1
強大的PHP整合開發環境

Dreamweaver CS6
視覺化網頁開發工具

SublimeText3 Mac版
神級程式碼編輯軟體(SublimeText3)
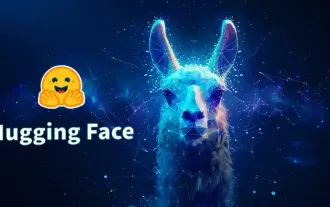
Meta的Llama 3.2:多模式和移動AI的飛躍 Meta最近公佈了Llama 3.2,這是AI的重大進步,具有強大的視覺功能和針對移動設備優化的輕量級文本模型。 以成功為基礎
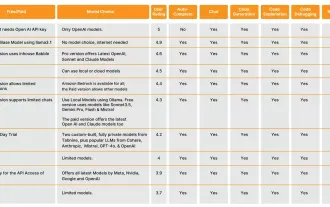
嘿,編碼忍者!您當天計劃哪些與編碼有關的任務?在您進一步研究此博客之前,我希望您考慮所有與編碼相關的困境,這是將其列出的。 完畢? - 讓&#8217
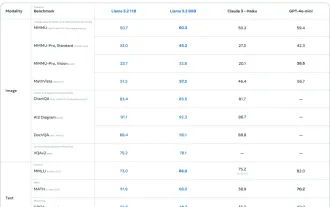
本週的AI景觀:進步,道德考慮和監管辯論的旋風。 OpenAI,Google,Meta和Microsoft等主要參與者已經釋放了一系列更新,從開創性的新車型到LE的關鍵轉變
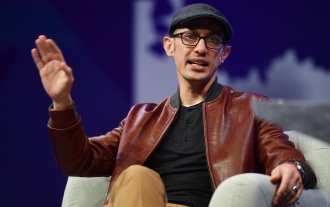
Shopify首席執行官TobiLütke最近的備忘錄大膽地宣布AI對每位員工的基本期望是公司內部的重大文化轉變。 這不是短暫的趨勢。這是整合到P中的新操作範式
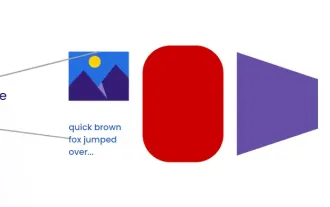
介紹 想像一下,穿過美術館,周圍是生動的繪畫和雕塑。現在,如果您可以向每一部分提出一個問題並獲得有意義的答案,該怎麼辦?您可能會問:“您在講什麼故事?
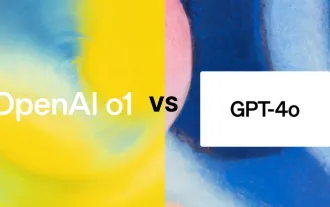
介紹 Openai已根據備受期待的“草莓”建築發布了其新模型。這種稱為O1的創新模型增強了推理能力,使其可以通過問題進行思考
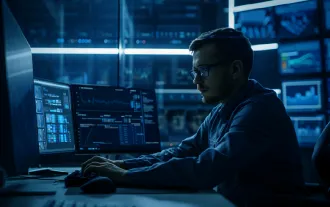
SQL的Alter表語句:動態地將列添加到數據庫 在數據管理中,SQL的適應性至關重要。 需要即時調整數據庫結構嗎? Alter表語句是您的解決方案。本指南的詳細信息添加了Colu
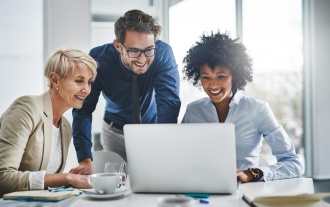
對於那些可能是我專欄新手的人,我廣泛探討了AI的最新進展,包括體現AI,AI推理,AI中的高科技突破,及時的工程,AI培訓,AI,AI RE RE等主題
