python基於Node2Vec怎麼實現節點分類及其視覺化
簡介
Node2vec是一種用於圖嵌入(Graph Embedding)的方法,可用於節點分類、社群發現和連結預測等任務。
實作過程
載入資料集
首先,讓我們載入所需的Python函式庫並執行以下程式碼以載入Cora資料集:
import networkx as nx import numpy as np import pandas as pd import matplotlib.pyplot as plt %matplotlib inline from sklearn.manifold import TSNE from node2vec import Node2Vec # 加载Cora数据集 cora = pd.read_csv('cora/cora.content', sep='\t', header=None) cited_in = pd.read_csv('cora/cora.cites', sep='\t', header=None, names=['target', 'source']) nodes, features = cora.iloc[:, :-1], cora.iloc[:, -1]
其中cora.content
包含了所有節點特徵訊息,總共具有2708個節點和1433個特徵;而cora.cites
透過引文映射分別針對所述每個節點建立一個節點間的有向邊關係,共有5429個邊。接下來,我們需要將節點特徵和引用資訊合併,建構圖結構。
# 定义函数:构造基于Cora数据集的图结构 def create_graph(nodes, features, cited_in): nodes.index = nodes.index.map(str) graph = nx.from_pandas_edgelist(cited_in, source='source', target='target') for index, row in nodes.iterrows(): node_id = str(row[0]) features = row.drop(labels=[0]) node_attrs = {f'attr_{i}': float(x) for i, x in enumerate(features)} if graph.has_node(node_id) == True: temp = graph.nodes[node_id] temp.update(node_attrs) graph.add_nodes_from([(node_id, temp)]) else: graph.add_nodes_from([(node_id, node_attrs)]) return graph # 构建图 graph = create_graph(nodes, features, cited_in)
此函數將 cora.content
中的節點特徵與 cora.cites
的有向邊整合,並在圖上標記它們。現在我們已經建立了一個圖形視圖,可以按想法視覺化。
使用Node2vec嵌入資料
為了進行節點特徵分類,我們需要從網路中提取一些資訊並將其作為輸入傳遞給分類器。一個範例是使用節點2向量方法將提取的資訊轉換為向量表達式,使每個節點至少具有一個維度。
透過隨機遊走樣本從起始節點到目標節點,Node2Vec模型學習代表每個節點的向量。節點2Vec模型定義隨機遊走過程中節點間的轉移機率。
我們將使用 node2vec 函式庫來產生圖形的嵌入表示,並採用神經網路進行節點分類。
# 定义函数:创建基于Cora数据集的嵌入 def create_embeddings(graph): # 初始化node2vec实例,指定相关超参数 n2v = Node2Vec(graph, dimensions=64, walk_length=30, num_walks=200, p=1, q=1, weight_key='attr_weight') # 基于指定参数训练得到嵌入向量表达式 model = n2v.fit(window=10, min_count=1, batch_words=4) # 获得所有图中节点的嵌入向量 embeddings = pd.DataFrame(model.wv.vectors) ids = list(map(str, model.wv.index2word)) # 将原有的特征和id与新获取到的嵌入向量按行合并 lookup_table = nodes.set_index(0).join(embeddings.set_index(embeddings.index)) return np.array(lookup_table.dropna().iloc[:, -64:]), np.array(list(range(1, lookup_table.shape[0] + 1))) # 创建嵌入向量 cora_embeddings, cora_labels = create_embeddings(graph)
透過以上程式碼,我們可以得到每個節點的64維節點嵌入表達。
訓練分類器
接下來我們將指定一些分類器並在Cora資料集上訓練它們,以期根據嵌入進行準確的節點分類操作。
from sklearn import svm, model_selection, metrics # 使用支持向量机作为示范的分类器 svm_model = svm.SVC(kernel='rbf', C=1, gamma=0.01) # 进行交叉验证和分类训练 scores = model_selection.cross_val_score( svm_model, cora_embeddings, cora_labels, cv=5) print(scores.mean())
為了獲得更好的效能,支援向量機作為分類器時,我們還需要對其進行相關調參操作。此處採取了5折交叉驗證的方式對其性能進行評估輸出。
視覺化節點嵌入
為了更好地理解,我們需要將人類難以理解的64維特徵表達進行降維處理以實現視覺化。 t-SNE是一種專門用於降低高維度資料複雜度的方法,我們在這裡使用它。它產生一個二維圖,相似節點之間緊密地聚集在一起,而這個圖是透過輸出僅包含兩個元素的機率分佈向量來實現的。
# 定义函数:可视化Nodes2Vec的结果 def visualize_results(embeddings, labels): # 使用t-SNE对数据进行降维并绘图 tsne = TSNE(n_components=2, verbose=1, perplexity=40, n_iter=300) tsne_results = tsne.fit_transform(embeddings) plt.figure(figsize=(10, 5)) plt.scatter(tsne_results[:,0], tsne_results[:,1], c=labels) plt.colorbar() plt.show() # 可视化结果 visualize_results(cora_embeddings, cora_labels)
Node2Vec產生的嵌入向量將被輸入到t-SNE中,其中t-SNE將64維向量表達進行了降維,並輸出我們可以使用 matplotlib 庫可視化的二維散點圖。大多數相關節點是否緊密聚集,可以在圖形介面中進行檢查。
以上是python基於Node2Vec怎麼實現節點分類及其視覺化的詳細內容。更多資訊請關注PHP中文網其他相關文章!

熱AI工具

Undresser.AI Undress
人工智慧驅動的應用程序,用於創建逼真的裸體照片

AI Clothes Remover
用於從照片中去除衣服的線上人工智慧工具。

Undress AI Tool
免費脫衣圖片

Clothoff.io
AI脫衣器

AI Hentai Generator
免費產生 AI 無盡。

熱門文章

熱工具

記事本++7.3.1
好用且免費的程式碼編輯器

SublimeText3漢化版
中文版,非常好用

禪工作室 13.0.1
強大的PHP整合開發環境

Dreamweaver CS6
視覺化網頁開發工具

SublimeText3 Mac版
神級程式碼編輯軟體(SublimeText3)

熱門話題
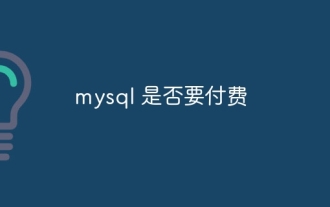
MySQL 有免費的社區版和收費的企業版。社區版可免費使用和修改,但支持有限,適合穩定性要求不高、技術能力強的應用。企業版提供全面商業支持,適合需要穩定可靠、高性能數據庫且願意為支持買單的應用。選擇版本時考慮的因素包括應用關鍵性、預算和技術技能。沒有完美的選項,只有最合適的方案,需根據具體情況謹慎選擇。

HadiDB:輕量級、高水平可擴展的Python數據庫HadiDB(hadidb)是一個用Python編寫的輕量級數據庫,具備高度水平的可擴展性。安裝HadiDB使用pip安裝:pipinstallhadidb用戶管理創建用戶:createuser()方法創建一個新用戶。 authentication()方法驗證用戶身份。 fromhadidb.operationimportuseruser_obj=user("admin","admin")user_obj.
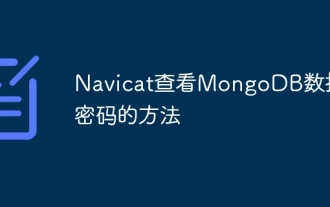
直接通過 Navicat 查看 MongoDB 密碼是不可能的,因為它以哈希值形式存儲。取回丟失密碼的方法:1. 重置密碼;2. 檢查配置文件(可能包含哈希值);3. 檢查代碼(可能硬編碼密碼)。
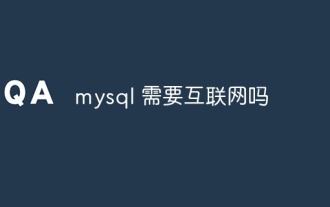
MySQL 可在無需網絡連接的情況下運行,進行基本的數據存儲和管理。但是,對於與其他系統交互、遠程訪問或使用高級功能(如復制和集群)的情況,則需要網絡連接。此外,安全措施(如防火牆)、性能優化(選擇合適的網絡連接)和數據備份對於連接到互聯網的 MySQL 數據庫至關重要。
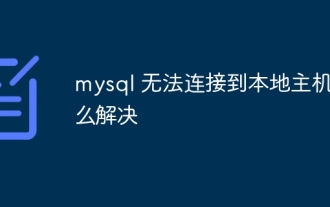
無法連接 MySQL 可能是由於以下原因:MySQL 服務未啟動、防火牆攔截連接、端口號錯誤、用戶名或密碼錯誤、my.cnf 中的監聽地址配置不當等。排查步驟包括:1. 檢查 MySQL 服務是否正在運行;2. 調整防火牆設置以允許 MySQL 監聽 3306 端口;3. 確認端口號與實際端口號一致;4. 檢查用戶名和密碼是否正確;5. 確保 my.cnf 中的 bind-address 設置正確。
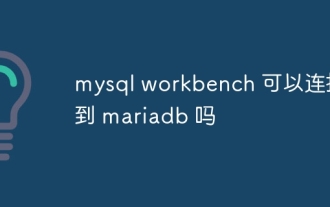
MySQL Workbench 可以連接 MariaDB,前提是配置正確。首先選擇 "MariaDB" 作為連接器類型。在連接配置中,正確設置 HOST、PORT、USER、PASSWORD 和 DATABASE。測試連接時,檢查 MariaDB 服務是否啟動,用戶名和密碼是否正確,端口號是否正確,防火牆是否允許連接,以及數據庫是否存在。高級用法中,使用連接池技術優化性能。常見錯誤包括權限不足、網絡連接問題等,調試錯誤時仔細分析錯誤信息和使用調試工具。優化網絡配置可以提升性能
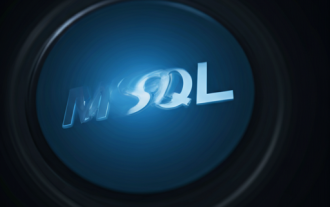
MySQL數據庫性能優化指南在資源密集型應用中,MySQL數據庫扮演著至關重要的角色,負責管理海量事務。然而,隨著應用規模的擴大,數據庫性能瓶頸往往成為製約因素。本文將探討一系列行之有效的MySQL性能優化策略,確保您的應用在高負載下依然保持高效響應。我們將結合實際案例,深入講解索引、查詢優化、數據庫設計以及緩存等關鍵技術。 1.數據庫架構設計優化合理的數據庫架構是MySQL性能優化的基石。以下是一些核心原則:選擇合適的數據類型選擇最小的、符合需求的數據類型,既能節省存儲空間,又能提升數據處理速度
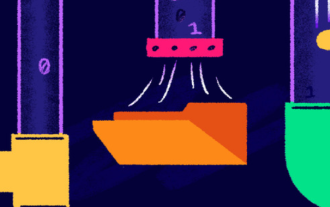
作為數據專業人員,您需要處理來自各種來源的大量數據。這可能會給數據管理和分析帶來挑戰。幸運的是,兩項 AWS 服務可以提供幫助:AWS Glue 和 Amazon Athena。
