在Python中進行矩陣和線性代數計算
在本文中,我們將學習如何使用Python進行矩陣和線性代數計算,例如矩陣乘法、求行列式、解線性方程式等。
從NumPy函式庫中可以使用一個矩陣物件來實現。在進行計算時,矩陣與數組物件相對可比。
線性代數是一個龐大的主題,超出了本文的範圍。
然而,如果你需要操作矩陣和向量,NumPy是一個很好的起點。
使用的方法
使用Numpy找到矩陣的轉置
使用Numpy找到矩陣的逆
矩陣與向量相乘
#使用numpy.linalg子包取得矩陣的行列式
使用numpy.linalg找出特徵值
使用numpy.linalg解方程式
方法1:使用Numpy找出矩陣的轉置
numpy.matrix.T 屬性 − 傳回給定矩陣的轉置。
Example
的中文翻譯為:範例
以下程式使用 numpy.matrix.T 屬性傳回矩陣的轉置 −
# importing NumPy module import numpy as np # input matrix inputMatrix = np.matrix([[6, 1, 5], [2, 0, 8], [1, 4, 3]]) # printing the input matrix print("Input Matrix:\n", inputMatrix) # printing the transpose of an input matrix # by applying the .T attribute of the NumPy matrix of the numpy Module print("Transpose of an input matrix\n", inputMatrix.T)
輸出
執行時,上述程式將產生以下輸出 -
Input Matrix: [[6 1 5] [2 0 8] [1 4 3]] Transpose of an input matrix [[6 2 1] [1 0 4] [5 8 3]]
方法2:使用Numpy找出矩陣的逆
numpy.matrix.I屬性 - 傳回給定矩陣的逆矩陣。
Example
的中文翻譯為:範例
以下程式使用 numpy.matrix.I 屬性傳回矩陣的逆矩陣 −
# importing NumPy module import numpy as np # input matrix inputMatrix = np.matrix([[6, 1, 5],[2, 0, 8],[1, 4, 3]]) # printing the input matrix print("Input Matrix:\n", inputMatrix) # printing the inverse of an input matrix # by applying the .I attribute of the NumPy matrix of the numpy Module print("Inverse of an input matrix:\n", inputMatrix.I)
輸出
執行時,上述程式將產生以下輸出 -
Input Matrix: [[6 1 5] [2 0 8] [1 4 3]] Inverse of an input matrix: [[ 0.21333333 -0.11333333 -0.05333333] [-0.01333333 -0.08666667 0.25333333] [-0.05333333 0.15333333 0.01333333]]
方法三:矩陣與向量相乘
Example
的中文翻譯為:範例
以下程式使用*運算子傳回輸入矩陣和向量的乘積 -
# importing numpy module import numpy as np # input matrix inputMatrix = np.matrix([[6, 1, 5],[2, 0, 8],[1, 4, 3]]) # printing the input matrix print("Input Matrix:\n", inputMatrix) # creating a vector using numpy.matrix() function inputVector = np.matrix([[1],[3],[5]]) # printing the multiplication of the input matrix and vector print("Multiplication of input matrix and vector:\n", inputMatrix*inputVector)
輸出
執行時,上述程式將產生以下輸出 -
Input Matrix: [[6 1 5] [2 0 8] [1 4 3]] Multiplication of input matrix and vector: [[34] [42] [28]]
方法四:使用numpy.linalg子包取得矩陣的行列式
numpy.linalg.det() 函數 − 計算一個方陣的行列式。
Example
的中文翻譯為:範例
下列程式使用 numpy.linalg.det() 函數傳回矩陣的行列式 −
# importing numpy module import numpy as np # input matrix inputMatrix = np.matrix([[6, 1, 5],[2, 0, 8],[1, 4, 3]]) # printing the input matrix print("Input Matrix:\n", inputMatrix) # getting the determinant of an input matrix outputDet = np.linalg.det(inputMatrix) # printing the determinant of an input matrix print("Determinant of an input matrix:\n", outputDet)
輸出
執行時,上述程式將產生以下輸出 -
Input Matrix: [[6 1 5] [2 0 8] [1 4 3]] Determinant of an input matrix: -149.99999999999997
使用numpy.linalg找到特徵值的第五種方法
numpy.linalg.eigvals() 函數 − 計算指定方陣/矩陣的特徵值和右特徵向量。
Example
的中文翻譯為:範例
The following program returns the Eigenvalues of an input matrix using the numpy.linalg.eigvals() function −
# importing NumPy module import numpy as np # input matrix inputMatrix = np.matrix([[6, 1, 5],[2, 0, 8],[1, 4, 3]]) # printing the input matrix print("Input Matrix:\n", inputMatrix) # getting Eigenvalues of an input matrix eigenValues = np.linalg.eigvals(inputMatrix) # printing Eigenvalues of an input matrix print("Eigenvalues of an input matrix:\n", eigenValues)
輸出
執行時,上述程式將產生以下輸出 -
Input Matrix: [[6 1 5] [2 0 8] [1 4 3]] Eigenvalues of an input matrix: [ 9.55480959 3.69447805 -4.24928765]
方法六:使用numpy.linalg解方程式
我們可以解決類似於找到 A*X = B 的 X 值的問題,
其中A是矩陣,B是向量。
Example
的中文翻譯為:範例
以下是使用solve()函數傳回x值的程式-
# importing NumPy module import numpy as np # input matrix inputMatrix = np.matrix([[6, 1, 5],[2, 0, 8],[1, 4, 3]]) # printing the input matrix print("Input Matrix:\n", inputMatrix) # creating a vector using np.matrix() function inputVector = np.matrix([[1],[3],[5]]) # getting the value of x in an equation inputMatrix * x = inputVector x_value = np.linalg.solve(inputMatrix, inputVector) # printing x value print("x value:\n", x_value) # multiplying input matrix with x values print("Multiplication of input matrix with x values:\n", inputMatrix * x_value)
輸出
執行時,上述程式將產生以下輸出 -
Input Matrix: [[6 1 5] [2 0 8] [1 4 3]] x value: [[-0.39333333] [ 0.99333333] [ 0.47333333]] Multiplication of input matrix with x values: [[1.] [3.] [5.]]
結論
在本文中,我們學習如何使用Python中的NumPy模組執行矩陣和線性代數運算。我們學會如何計算矩陣的轉置、逆和行列式。我們也學習如何在線性代數中進行一些計算,例如解方程式和確定特徵值。
以上是在Python中進行矩陣和線性代數計算的詳細內容。更多資訊請關注PHP中文網其他相關文章!

熱AI工具

Undresser.AI Undress
人工智慧驅動的應用程序,用於創建逼真的裸體照片

AI Clothes Remover
用於從照片中去除衣服的線上人工智慧工具。

Undress AI Tool
免費脫衣圖片

Clothoff.io
AI脫衣器

Video Face Swap
使用我們完全免費的人工智慧換臉工具,輕鬆在任何影片中換臉!

熱門文章

熱工具

記事本++7.3.1
好用且免費的程式碼編輯器

SublimeText3漢化版
中文版,非常好用

禪工作室 13.0.1
強大的PHP整合開發環境

Dreamweaver CS6
視覺化網頁開發工具

SublimeText3 Mac版
神級程式碼編輯軟體(SublimeText3)
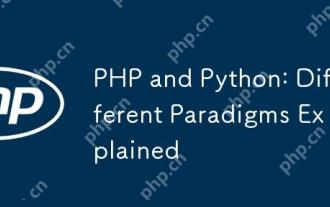
PHP主要是過程式編程,但也支持面向對象編程(OOP);Python支持多種範式,包括OOP、函數式和過程式編程。 PHP適合web開發,Python適用於多種應用,如數據分析和機器學習。
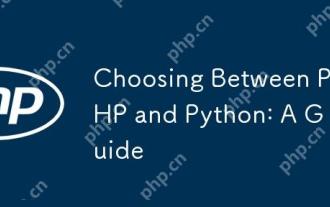
PHP適合網頁開發和快速原型開發,Python適用於數據科學和機器學習。 1.PHP用於動態網頁開發,語法簡單,適合快速開發。 2.Python語法簡潔,適用於多領域,庫生態系統強大。
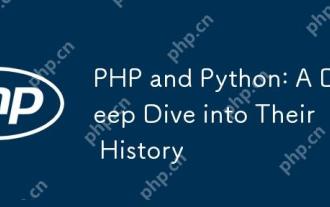
PHP起源於1994年,由RasmusLerdorf開發,最初用於跟踪網站訪問者,逐漸演變為服務器端腳本語言,廣泛應用於網頁開發。 Python由GuidovanRossum於1980年代末開發,1991年首次發布,強調代碼可讀性和簡潔性,適用於科學計算、數據分析等領域。
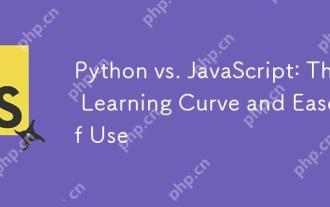
Python更適合初學者,學習曲線平緩,語法簡潔;JavaScript適合前端開發,學習曲線較陡,語法靈活。 1.Python語法直觀,適用於數據科學和後端開發。 2.JavaScript靈活,廣泛用於前端和服務器端編程。
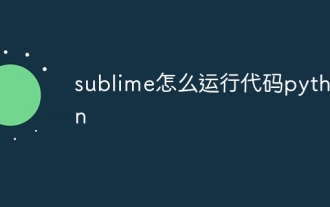
在 Sublime Text 中運行 Python 代碼,需先安裝 Python 插件,再創建 .py 文件並編寫代碼,最後按 Ctrl B 運行代碼,輸出會在控制台中顯示。
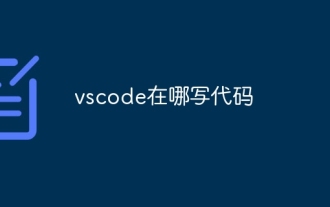
在 Visual Studio Code(VSCode)中編寫代碼簡單易行,只需安裝 VSCode、創建項目、選擇語言、創建文件、編寫代碼、保存並運行即可。 VSCode 的優點包括跨平台、免費開源、強大功能、擴展豐富,以及輕量快速。
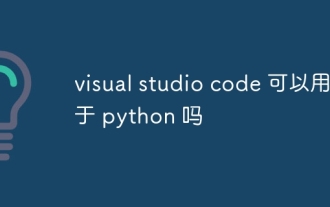
VS Code 可用於編寫 Python,並提供許多功能,使其成為開發 Python 應用程序的理想工具。它允許用戶:安裝 Python 擴展,以獲得代碼補全、語法高亮和調試等功能。使用調試器逐步跟踪代碼,查找和修復錯誤。集成 Git,進行版本控制。使用代碼格式化工具,保持代碼一致性。使用 Linting 工具,提前發現潛在問題。
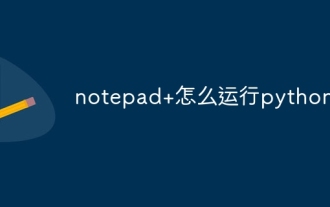
在 Notepad 中運行 Python 代碼需要安裝 Python 可執行文件和 NppExec 插件。安裝 Python 並為其添加 PATH 後,在 NppExec 插件中配置命令為“python”、參數為“{CURRENT_DIRECTORY}{FILE_NAME}”,即可在 Notepad 中通過快捷鍵“F6”運行 Python 代碼。
