使用Accelerate函式庫在多GPU上進行LLM推理
大型语言模型(llm)已经彻底改变了自然语言处理领域。随着这些模型在规模和复杂性上的增长,推理的计算需求也显著增加。为了应对这一挑战利用多个gpu变得至关重要。
因此,这篇文章将在多个GPU上同时进行推理,内容主要包括:介绍Accelerate库、简单的方法和工作代码示例,以及使用多个GPU进行性能基准测试
本文将使用多个3090将llama2-7b的推理扩展在多个GPU上
基本示例
我们首先介绍一个简单的示例来演示使用Accelerate进行多gpu“消息传递”。
from accelerate import Accelerator from accelerate.utils import gather_object accelerator = Accelerator() # each GPU creates a string message=[ f"Hello this is GPU {accelerator.process_index}" ] # collect the messages from all GPUs messages=gather_object(message) # output the messages only on the main process with accelerator.print() accelerator.print(messages)
输出如下:
['Hello this is GPU 0', 'Hello this is GPU 1', 'Hello this is GPU 2', 'Hello this is GPU 3', 'Hello this is GPU 4']
多GPU推理
下面是一个简单的、非批处理的推理方法。代码很简单,因为Accelerate库已经帮我们做了很多工作,我们直接使用就可以:
from accelerate import Accelerator from accelerate.utils import gather_object from transformers import AutoModelForCausalLM, AutoTokenizer from statistics import mean import torch, time, json accelerator = Accelerator() # 10*10 Prompts. Source: https://www.penguin.co.uk/articles/2022/04/best-first-lines-in-books prompts_all=["The King is dead. Long live the Queen.","Once there were four children whose names were Peter, Susan, Edmund, and Lucy.","The story so far: in the beginning, the universe was created.","It was a bright cold day in April, and the clocks were striking thirteen.","It is a truth universally acknowledged, that a single man in possession of a good fortune, must be in want of a wife.","The sweat wis lashing oafay Sick Boy; he wis trembling.","124 was spiteful. Full of Baby's venom.","As Gregor Samsa awoke one morning from uneasy dreams he found himself transformed in his bed into a gigantic insect.","I write this sitting in the kitchen sink.","We were somewhere around Barstow on the edge of the desert when the drugs began to take hold.", ] * 10 # load a base model and tokenizer model_path="models/llama2-7b" model = AutoModelForCausalLM.from_pretrained(model_path,device_map={"": accelerator.process_index},torch_dtype=torch.bfloat16, ) tokenizer = AutoTokenizer.from_pretrained(model_path) # sync GPUs and start the timer accelerator.wait_for_everyone() start=time.time() # divide the prompt list onto the available GPUs with accelerator.split_between_processes(prompts_all) as prompts:# store output of generations in dictresults=dict(outputs=[], num_tokens=0) # have each GPU do inference, prompt by promptfor prompt in prompts:prompt_tokenized=tokenizer(prompt, return_tensors="pt").to("cuda")output_tokenized = model.generate(**prompt_tokenized, max_new_tokens=100)[0] # remove prompt from output output_tokenized=output_tokenized[len(prompt_tokenized["input_ids"][0]):] # store outputs and number of tokens in result{}results["outputs"].append( tokenizer.decode(output_tokenized) )results["num_tokens"] += len(output_tokenized) results=[ results ] # transform to list, otherwise gather_object() will not collect correctly # collect results from all the GPUs results_gathered=gather_object(results) if accelerator.is_main_process:timediff=time.time()-startnum_tokens=sum([r["num_tokens"] for r in results_gathered ]) print(f"tokens/sec: {num_tokens//timediff}, time {timediff}, total tokens {num_tokens}, total prompts {len(prompts_all)}")
使用多个gpu会导致一些通信开销:性能在4个gpu时呈线性增长,然后在这种特定设置中趋于稳定。当然这里的性能取决于许多参数,如模型大小和量化、提示长度、生成的令牌数量和采样策略,所以我们只讨论一般的情况
1 GPU: 44个token /秒,时间:225.5s
2个GPU:每秒处理88个token,总共用时112.9秒
3个GPU:每秒处理128个令牌,总共耗时77.6秒
4 gpu: 137个token /秒,时间:72.7s
5个gpu:每秒处理119个token,总共需要83.8秒的时间
在多GPU上进行批处理
现实世界中,我们可以使用批处理推理来加快速度。这会减少GPU之间的通讯,加快推理速度。我们只需要增加prepare_prompts函数将一批数据而不是单条数据输入到模型即可:
from accelerate import Accelerator from accelerate.utils import gather_object from transformers import AutoModelForCausalLM, AutoTokenizer from statistics import mean import torch, time, json accelerator = Accelerator() def write_pretty_json(file_path, data):import jsonwith open(file_path, "w") as write_file:json.dump(data, write_file, indent=4) # 10*10 Prompts. Source: https://www.penguin.co.uk/articles/2022/04/best-first-lines-in-books prompts_all=["The King is dead. Long live the Queen.","Once there were four children whose names were Peter, Susan, Edmund, and Lucy.","The story so far: in the beginning, the universe was created.","It was a bright cold day in April, and the clocks were striking thirteen.","It is a truth universally acknowledged, that a single man in possession of a good fortune, must be in want of a wife.","The sweat wis lashing oafay Sick Boy; he wis trembling.","124 was spiteful. Full of Baby's venom.","As Gregor Samsa awoke one morning from uneasy dreams he found himself transformed in his bed into a gigantic insect.","I write this sitting in the kitchen sink.","We were somewhere around Barstow on the edge of the desert when the drugs began to take hold.", ] * 10 # load a base model and tokenizer model_path="models/llama2-7b" model = AutoModelForCausalLM.from_pretrained(model_path,device_map={"": accelerator.process_index},torch_dtype=torch.bfloat16, ) tokenizer = AutoTokenizer.from_pretrained(model_path) tokenizer.pad_token = tokenizer.eos_token # batch, left pad (for inference), and tokenize def prepare_prompts(prompts, tokenizer, batch_size=16):batches=[prompts[i:i + batch_size] for i in range(0, len(prompts), batch_size)]batches_tok=[]tokenizer.padding_side="left" for prompt_batch in batches:batches_tok.append(tokenizer(prompt_batch, return_tensors="pt", padding='longest', truncatinotallow=False, pad_to_multiple_of=8,add_special_tokens=False).to("cuda") )tokenizer.padding_side="right"return batches_tok # sync GPUs and start the timer accelerator.wait_for_everyone() start=time.time() # divide the prompt list onto the available GPUs with accelerator.split_between_processes(prompts_all) as prompts:results=dict(outputs=[], num_tokens=0) # have each GPU do inference in batchesprompt_batches=prepare_prompts(prompts, tokenizer, batch_size=16) for prompts_tokenized in prompt_batches:outputs_tokenized=model.generate(**prompts_tokenized, max_new_tokens=100) # remove prompt from gen. tokensoutputs_tokenized=[ tok_out[len(tok_in):] for tok_in, tok_out in zip(prompts_tokenized["input_ids"], outputs_tokenized) ] # count and decode gen. tokens num_tokens=sum([ len(t) for t in outputs_tokenized ])outputs=tokenizer.batch_decode(outputs_tokenized) # store in results{} to be gathered by accelerateresults["outputs"].extend(outputs)results["num_tokens"] += num_tokens results=[ results ] # transform to list, otherwise gather_object() will not collect correctly # collect results from all the GPUs results_gathered=gather_object(results) if accelerator.is_main_process:timediff=time.time()-startnum_tokens=sum([r["num_tokens"] for r in results_gathered ]) print(f"tokens/sec: {num_tokens//timediff}, time elapsed: {timediff}, num_tokens {num_tokens}")
可以看到批处理会大大加快速度。
需要重写的内容是:1个GPU:520个令牌/秒,时间:19.2秒
两张GPU的算力为每秒900个代币,计算时间为11.1秒
3 gpu: 1205个token /秒,时间:8.2s
四张GPU:1655个令牌/秒,所需时间为6.0秒
5个GPU: 每秒1658个令牌,时间:6.0秒
总结
截止到本文为止,llama.cpp,ctransformer还不支持多GPU推理,好像llama.cpp在6月有个多GPU的merge,但是我没看到官方更新,所以这里暂时确定不支持多GPU。如果有小伙伴确认可以支持多GPU请留言。
huggingface的Accelerate包则为我们使用多GPU提供了一个很方便的选择,使用多个GPU推理可以显着提高性能,但gpu之间通信的开销随着gpu数量的增加而显著增加。
以上是使用Accelerate函式庫在多GPU上進行LLM推理的詳細內容。更多資訊請關注PHP中文網其他相關文章!

熱AI工具

Undresser.AI Undress
人工智慧驅動的應用程序,用於創建逼真的裸體照片

AI Clothes Remover
用於從照片中去除衣服的線上人工智慧工具。

Undress AI Tool
免費脫衣圖片

Clothoff.io
AI脫衣器

Video Face Swap
使用我們完全免費的人工智慧換臉工具,輕鬆在任何影片中換臉!

熱門文章

熱工具

記事本++7.3.1
好用且免費的程式碼編輯器

SublimeText3漢化版
中文版,非常好用

禪工作室 13.0.1
強大的PHP整合開發環境

Dreamweaver CS6
視覺化網頁開發工具

SublimeText3 Mac版
神級程式碼編輯軟體(SublimeText3)
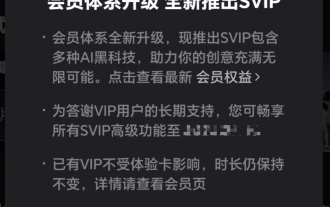
本站6月27日訊息,剪映是由位元組跳動旗下臉萌科技開發的一款影片剪輯軟體,依託於抖音平台且基本面向該平台用戶製作短影片內容,並相容於iOS、安卓、Windows 、MacOS等作業系統。剪映官方宣布會員體系升級,推出全新SVIP,包含多種AI黑科技,例如智慧翻譯、智慧劃重點、智慧包裝、數位人合成等。價格方面,剪映SVIP月費79元,年費599元(本站註:折合每月49.9元),連續包月則為59元每月,連續包年為499元每年(折合每月41.6元) 。此外,剪映官方也表示,為提升用戶體驗,向已訂閱了原版VIP
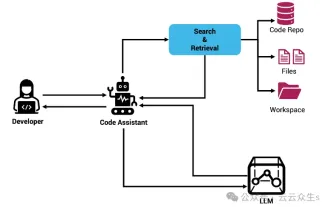
透過將檢索增強生成和語意記憶納入AI編碼助手,提升開發人員的生產力、效率和準確性。譯自EnhancingAICodingAssistantswithContextUsingRAGandSEM-RAG,作者JanakiramMSV。雖然基本AI程式設計助理自然有幫助,但由於依賴對軟體語言和編寫軟體最常見模式的整體理解,因此常常無法提供最相關和正確的程式碼建議。這些編碼助手產生的代碼適合解決他們負責解決的問題,但通常不符合各個團隊的編碼標準、慣例和風格。這通常會導致需要修改或完善其建議,以便將程式碼接受到應

想了解更多AIGC的內容,請造訪:51CTOAI.x社群https://www.51cto.com/aigc/譯者|晶顏審校|重樓不同於網路上隨處可見的傳統問題庫,這些問題需要跳脫常規思維。大語言模型(LLM)在數據科學、生成式人工智慧(GenAI)和人工智慧領域越來越重要。這些複雜的演算法提升了人類的技能,並在許多產業中推動了效率和創新性的提升,成為企業保持競爭力的關鍵。 LLM的應用範圍非常廣泛,它可以用於自然語言處理、文字生成、語音辨識和推薦系統等領域。透過學習大量的數據,LLM能夠產生文本
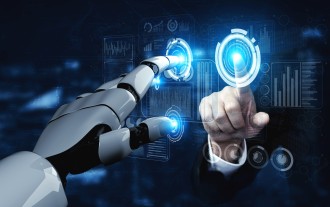
大型語言模型(LLM)是在龐大的文字資料庫上訓練的,在那裡它們獲得了大量的實際知識。這些知識嵌入到它們的參數中,然後可以在需要時使用。這些模型的知識在訓練結束時被「具體化」。在預訓練結束時,模型實際上停止學習。對模型進行對齊或進行指令調優,讓模型學習如何充分利用這些知識,以及如何更自然地回應使用者的問題。但是有時模型知識是不夠的,儘管模型可以透過RAG存取外部內容,但透過微調使用模型適應新的領域被認為是有益的。這種微調是使用人工標註者或其他llm創建的輸入進行的,模型會遇到額外的實際知識並將其整合
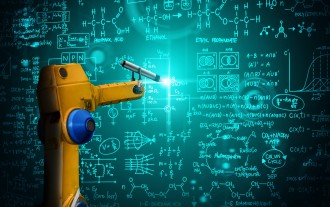
機器學習是人工智慧的重要分支,它賦予電腦從數據中學習的能力,並能夠在無需明確編程的情況下改進自身能力。機器學習在各個領域都有廣泛的應用,從影像辨識和自然語言處理到推薦系統和詐欺偵測,它正在改變我們的生活方式。機器學習領域存在著多種不同的方法和理論,其中最具影響力的五種方法被稱為「機器學習五大派」。這五大派分別為符號派、聯結派、進化派、貝葉斯派和類推學派。 1.符號學派符號學(Symbolism),又稱符號主義,強調利用符號進行邏輯推理和表達知識。該學派認為學習是一種逆向演繹的過程,透過現有的
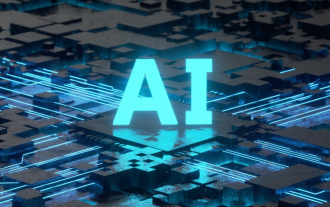
編輯|ScienceAI問答(QA)資料集在推動自然語言處理(NLP)研究中發揮著至關重要的作用。高品質QA資料集不僅可以用於微調模型,也可以有效評估大語言模型(LLM)的能力,尤其是針對科學知識的理解和推理能力。儘管目前已有許多科學QA數據集,涵蓋了醫學、化學、生物等領域,但這些數據集仍有一些不足之處。其一,資料形式較為單一,大多數為多項選擇題(multiple-choicequestions),它們易於進行評估,但限制了模型的答案選擇範圍,無法充分測試模型的科學問題解答能力。相比之下,開放式問答
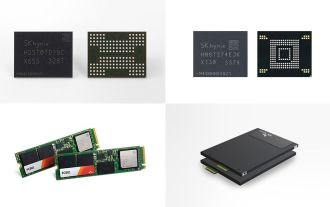
本站8月1日消息,SK海力士今天(8月1日)發布博文,宣布將出席8月6日至8日,在美國加州聖克拉拉舉行的全球半導體記憶體峰會FMS2024,展示諸多新一代產品。未來記憶體和儲存高峰會(FutureMemoryandStorage)簡介前身是主要面向NAND供應商的快閃記憶體高峰會(FlashMemorySummit),在人工智慧技術日益受到關注的背景下,今年重新命名為未來記憶體和儲存高峰會(FutureMemoryandStorage),以邀請DRAM和儲存供應商等更多參與者。新產品SK海力士去年在
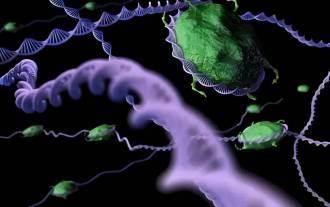
編輯|KX在藥物研發領域,準確有效地預測蛋白質與配體的結合親和力對於藥物篩選和優化至關重要。然而,目前的研究並沒有考慮到分子表面訊息在蛋白質-配體相互作用中的重要作用。基於此,來自廈門大學的研究人員提出了一種新穎的多模態特徵提取(MFE)框架,該框架首次結合了蛋白質表面、3D結構和序列的信息,並使用交叉注意機制進行不同模態之間的特徵對齊。實驗結果表明,該方法在預測蛋白質-配體結合親和力方面取得了最先進的性能。此外,消融研究證明了該框架內蛋白質表面資訊和多模態特徵對齊的有效性和必要性。相關研究以「S
