AI視訊生成框架測試競爭:Pika、Gen-2、ModelScope、SEINE,誰能勝出?
AI 影片生成,是最近最熱門的領域之一。各大學實驗室、網路巨頭 AI Lab、新創公司紛紛加入了 AI 影片生成的賽道。 Pika、Gen-2、Show-1、VideoCrafter、ModelScope、SEINE、LaVie、VideoLDM 等影片產生模型的發布,更是讓人眼睛一亮。 v⁽ⁱ⁾
大家一定對以下幾個問題感到好奇:
- ##到底哪個影片生成模型最牛?
- 每個模型有什麼專長?
- AI 影片產生領域目前還有哪些值得關注的問題待解決?
為此,我們推出了VBench,一個全面的「視訊生成模型的評測框架」,旨在向用戶提供關於各種視訊模型的優劣和特點。透過VBench,使用者可以了解不同視訊模型的強項和優勢。
- #論文:https://arxiv.org/abs /2311.17982
- #程式碼:https://github.com/Vchitect/VBench
- 網頁:https://vchitect.github.io /VBench-project/
- 論文標題:VBench: Comprehensive Benchmark Suite for Video Generative Models
#VBench不僅能全面、細緻地評估影片生成效果,也能提供符合人們感官體驗的評估,節省時間和精力。
- VBench 包含16 個分層和解耦的評測維度
- VBench 開源了用於文生視訊產生評測的Prompt List 系統
- VBench 每個維度的評測方案與人類的觀感與評估對齊
- VBench 提供了多視角的洞察,助力未來對於AI 視訊生成的探索
AI 影片產生模型- 評測結果
已開源的AI視訊生成模型
各個開源的AI 視訊產生模型在 VBench 上的表現如下。
在以上 6 個模型中,可以看到 VideoCrafter-1.0 和 Show-1 在大多數維度都有相對優勢。
新創公司的影片產生模型
#VBench 目前給了Gen-2 和Pika 這兩家創業公司模式的評測結果。
Gen-2 和 Pika 在 VBench 上的表現。在雷達圖中,為了更清晰地視覺化比較,我們加入了 VideoCrafter-1.0 和 Show-1 作為參考,同時將每個維度的評測結果歸一化到了 0.3 與 0.8 之間。
#
Performance of Gen-2 and Pika on VBench. We include the numerical results of VideoCrafter-1.0 and Show-1 as reference.
It can be seen that Gen-2 and Pika have obvious advantages in video quality (Video Quality), such as timing consistency (Temporal Consistency) and single frame quality (Aesthetic Quality and Imaging Quality) related dimensions. In terms of semantic consistency with user input prompts (such as Human Action and Appearance Style), partial-dimensional open source models will be better.
Video generation model VS picture generation model
Video generation model VS Image generation model. Among them, SD1.4, SD2.1 and SDXL are image generation models.
The performance of the video generation model in 8 major scene categories
The following are the performance of different models in 8 different categories evaluation results on.
VBench is now open source and can be installed with one click
At present, VBench is fully open source. And supports one-click installation. Everyone is welcome to play, test the models you are interested in, and work together to promote the development of the video generation community.
#Open source address :https://github.com/Vchitect/VBench
We have also open sourced a series of Prompt Lists : https://github.com/Vchitect/VBench/tree/master/prompts, including Benchmarks for evaluation in different capability dimensions, as well as evaluation Benchmarks on different scenario content.
The word cloud on the left shows the distribution of high-frequency words in our Prompt Suites, and the picture on the right shows the statistics of the number of prompts in different dimensions and categories.
Is VBench accurate?
For each dimension, we calculated the correlation between the VBench evaluation results and the manual evaluation results to verify the consistency of our method with human perception. In the figure below, the horizontal axis represents the manual evaluation results in different dimensions, and the vertical axis shows the results of the automatic evaluation of the VBench method. It can be seen that our method is highly aligned with human perception in all dimensions.
VBench brings thinking to AI video generation
VBench can not only evaluate existing models , More importantly, various problems that may exist in different models can also be discovered, providing valuable insights for the future development of AI video generation.
"Temporal continuity" and "video dynamic level": Don't choose one or the other, but improve both
We found that there is a certain trade-off relationship between temporal coherence (such as Subject Consistency, Background Consistency, Motion Smoothness) and the amplitude of motion in the video (Dynamic Degree). For example, Show-1 and VideoCrafter-1.0 performed very well in terms of background consistency and action smoothness, but scored lower in terms of dynamics; this may be because the generated "not moving" pictures are more likely to appear "in the timing" Very coherent." VideoCrafter-0.9, on the other hand, is weaker on the dimension related to timing consistency, but scores high on Dynamic Degree.
This shows that it is indeed difficult to achieve "temporal coherence" and "higher dynamic level" at the same time; in the future, we should not only focus on improving one aspect, but should also improve "temporal coherence" And "the dynamic level of the video", this is meaningful.
Evaluate by scene content to explore the potential of each model
Some models perform well in different categories There are big differences in performance. For example, in terms of aesthetic quality, CogVideo performs well in the "Food" category, but scores lower in the "LifeStyle" category. If the training data is adjusted, can the aesthetic quality of CogVideo in the "LifeStyle" categories be improved, thereby improving the overall video aesthetic quality of the model?
This also tells us that when evaluating video generation models, we need to consider the performance of the model under different categories or topics, explore the upper limit of the model in a certain capability dimension, and then target Improve the "holding back" scenario category.
Categories with complex motion: poor spatiotemporal performance
Categories with high spatial complexity, Scores in the aesthetic quality dimension are relatively low. For example, the "LifeStyle" category has relatively high requirements for the layout of complex elements in space, and the "Human" category poses challenges due to the generation of hinged structures.
For categories with complex timing, such as the "Human" category which usually involves complex movements and the "Vehicle" category which often moves faster, they score equally in all tested dimensions. relatively low. This shows that the current model still has certain deficiencies in processing temporal modeling. The temporal modeling limitations may lead to spatial blurring and distortion, resulting in unsatisfactory video quality in both time and space.
Difficult to generate categories: little benefit from increasing data volume
We use the commonly used video data set WebVid- 10M conducted statistics and found that about 26% of the data was related to "Human", accounting for the highest proportion among the eight categories we counted. However, in the evaluation results, the “Human” category was one of the worst performing among the eight categories.
This shows that for a complex category like "Human", simply increasing the amount of data may not bring significant improvements to performance. One potential method is to guide the learning of the model by introducing "Human" related prior knowledge or control, such as Skeletons, etc.
Millions of data sets: improving data quality takes precedence over data quantity
Although the "Food" category Occupying only 11% of WebVid-10M, it almost always has the highest aesthetic quality score in the review. So we further analyzed the aesthetic quality performance of different categories of content in the WebVid-10M data set and found that the "Food" category also had the highest aesthetic score in WebVid-10M.
This means that on the basis of millions of data, filtering/improving data quality is more helpful than increasing the amount of data.
Ability to be improved: Accurately generate multiple objects and the relationship between objects
Current video generation The model still cannot catch up with the image generation model (especially SDXL) in terms of "Multiple Objects" and "Spatial Relationship", which highlights the importance of improving combination capabilities. The so-called combination ability refers to whether the model can accurately display multiple objects in video generation, as well as the spatial and interactive relationships between them.
Potential solutions to this problem may include:
- Data labeling: Construct a video dataset to provide A clear description of multiple objects, as well as a description of the spatial positional relationships and interactions between objects.
- Add intermediate modes/modules during the video generation process to assist in controlling the combination and spatial position of objects.
- Using a better text encoder (Text Encoder) will also have a greater impact on the combined generation ability of the model.
- Curve to save the country: hand over the "object combination" problem that T2V cannot do well to T2I, and generate videos through T2I I2V. This approach may also be effective for many other video generation problems.
以上是AI視訊生成框架測試競爭:Pika、Gen-2、ModelScope、SEINE,誰能勝出?的詳細內容。更多資訊請關注PHP中文網其他相關文章!

熱AI工具

Undresser.AI Undress
人工智慧驅動的應用程序,用於創建逼真的裸體照片

AI Clothes Remover
用於從照片中去除衣服的線上人工智慧工具。

Undress AI Tool
免費脫衣圖片

Clothoff.io
AI脫衣器

Video Face Swap
使用我們完全免費的人工智慧換臉工具,輕鬆在任何影片中換臉!

熱門文章

熱工具

記事本++7.3.1
好用且免費的程式碼編輯器

SublimeText3漢化版
中文版,非常好用

禪工作室 13.0.1
強大的PHP整合開發環境

Dreamweaver CS6
視覺化網頁開發工具

SublimeText3 Mac版
神級程式碼編輯軟體(SublimeText3)
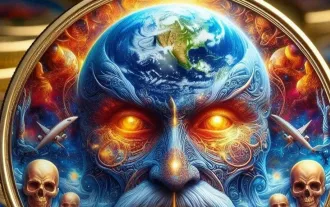
WorldCoin(WLD)凭借其独特的生物识别验证和隐私保护机制,在加密货币市场中脱颖而出,吸引了众多投资者的目光。WLD凭借其创新技术,特别是结合OpenAI人工智能技术,在众多山寨币中表现突出。但未来几年,数字资产的走势如何呢?让我们一起预测WLD的未来价格。2025年WLD价格预测预计2025年WLD将实现显著增长。市场分析显示,WLD平均价格可能达到1.31美元,最高可能触及1.36美元。然而,在熊市情况下,价格可能跌至0.55美元左右。这一增长预期主要源于WorldCoin2.
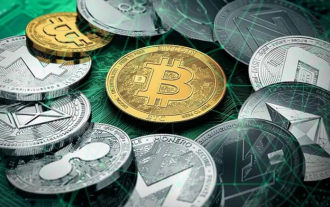
虛擬幣價格上漲因素包括:1.市場需求增加,2.供應量減少,3.利好消息刺激,4.市場情緒樂觀,5.宏觀經濟環境;下降因素包括:1.市場需求減少,2.供應量增加,3.利空消息打擊,4.市場情緒悲觀,5.宏觀經濟環境。
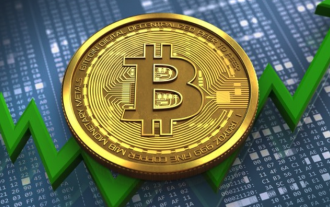
支持跨鏈交易的交易所有:1. Binance,2. Uniswap,3. SushiSwap,4. Curve Finance,5. Thorchain,6. 1inch Exchange,7. DLN Trade,這些平台通過各種技術支持多鏈資產交易。

Aavenomics是修改AAVE協議令牌並引入令牌回購的提議,已為AAVEDAO實現了一個法定人數。 AAVE連鎖計劃(ACI)創始人馬克·澤勒(MarcZeller)在X上宣布了這一點,並指出它標誌著該協議的新時代。 AAVE連鎖倡議(ACI)創始人MarcZeller在X上宣布,Aavenomics提案包括修改AAVE協議令牌和引入令牌回購,已為AAVEDAO實現了法定人數。根據Zeller的說法,這標誌著該協議的新時代。 AaveDao成員以壓倒性的投票支持該提議,即在周三以每週100
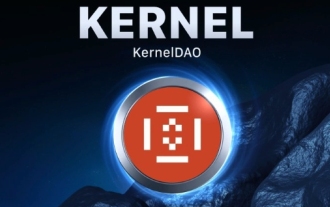
在加密貨幣的繁華世界裡,新機遇總是不斷湧現。當下,KernelDAO (KERNEL) 空投活動正備受矚目,吸引著眾多投資者的目光。那麼,這個項目究竟是什麼來頭? BNB Holder 又能從中獲得怎樣的好處?別急,下面將為你一一揭曉。
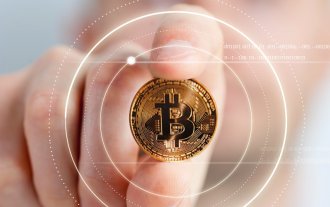
繪製比特幣結構分析圖的步驟包括:1. 確定繪圖目的與受眾,2. 選擇合適的工具,3. 設計框架並填充核心組件,4. 參考現有模板。完整的步驟確保圖表準確且易於理解。
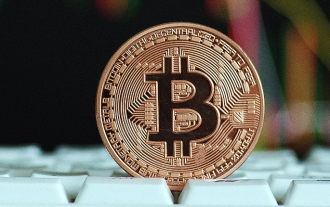
選擇加密貨幣交易所的建議:1. 流動性需求,優先選擇幣安、Gate.io或OKX,因其訂單深度與抗波動能力強。 2. 合規與安全,Coinbase、Kraken、Gemini具備嚴格監管背書。 3. 創新功能,KuCoin的軟質押和Bybit的衍生品設計適合進階用戶。
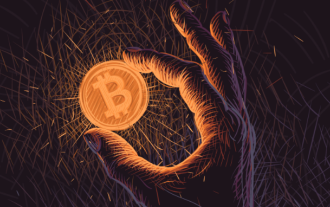
2025年在槓桿交易、安全性和用戶體驗方面表現突出的平台有:1. OKX,適合高頻交易者,提供最高100倍槓桿;2. Binance,適用於全球多幣種交易者,提供125倍高槓桿;3. Gate.io,適合衍生品專業玩家,提供100倍槓桿;4. Bitget,適用於新手及社交化交易者,提供最高100倍槓桿;5. Kraken,適合穩健型投資者,提供5倍槓桿;6. Bybit,適用於山寨幣探索者,提供20倍槓桿;7. KuCoin,適合低成本交易者,提供10倍槓桿;8. Bitfinex,適合資深玩
