What-if I told you complex Data Integration makes good Task Orchestration?
No matter how complex your data problem is, you can always Divide & Conquer it. ???
In this article, we’ll break down how to solve intricate data challenges, data treatment, data management using a fully open-source Python library: Taipy. Whether you're cleaning data, orchestrating tasks, or exploring different outcomes, Taipy's intuitive design empowers you to handle it all.
Star Taipy ⭐️
Taipy's Scenario Management basis
Let’s go through three steps to show how Taipy can help streamline your data workflow:
1. Data Integration: Get the Data You Need
Every AI, ML, and data-driven project starts with data, obviously!
And it’s rarely as simple as a single, clean dataset. In most cases, you'll be pulling data from databases, APIs, flat files, or other external sources, and sometimes, all in the same project. This is where Taipy's data integration comes in—gathering and unifying data from these different sources.
In Taipy, this process is simplified with a key abstraction: the Data Node.
A Data Node represents your data but doesn’t store it directly. Instead, it holds all the necessary metadata to read and write the actual data, whether it’s a CSV file, a database table, or even an API response.
Here’s a quick example of defining a Data Node for a CSV:
from taipy import Config initial_dataset_cfg = Config.configure_data_node(id="initial_dataset", storage_type="csv", path="data/dataset.csv", scope=Scope.GLOBAL)
With this abstraction, Taipy takes care of data management, allowing you to focus on transforming and processing your data.
2. Task Orchestration: What Are You Doing with All That Data?
Now that your data is in place, what do you do with it? In any data workflow, the next step involves defining tasks that process and transform the data. This is what we call the Task Orchestration
A Task in Taipy is like a function, taking Data Node(s) as inputs, performing transformations, and then outputting Data Node(s).
For instance, you may want to filter some data or calculate new metrics. Here's an example of creating a Task to calculate the sum of a column:
clean_data_task_cfg = Config.configure_task(id="clean_data", function=clean_data, input=initial_dataset_cfg, output=cleaned_dataset_cfg, skippable=True)
Once you've defined your tasks, you can arrange them into a pipeline to ensure the steps execute in the correct order. This lets you easily build workflows that transform data, build models, generate reports, and more.
3. What-If Analysis: Explore Different Scenarios
Once your data and workflows are set up, you can begin exploring different scenarios. This is where the What-if analysis comes into play.
A Scenario in Taipy represents a specific instance of a problem you’re trying to solve, allowing you to test different parameters and see how they impact your results. By adjusting input data or assumptions, you can model various scenarios without starting over from scratch.
Here's how you can define a Scenario in Taipy:
scenario_cfg = Config.configure_scenario(id="scenario", task_configs=[clean_data_task_cfg, predict_task_cfg,evaluate_task_cfg], frequency=Frequency.MONTHLY) tp.Core().run() my_first_scenario = create_and_run_scenario(dt.datetime(2021, 1, 25)) predictions = my_first_scenario.predictions.read() print("Predictions\n", predictions)
This makes it easy to perform sensitivity analysis or optimize outcomes, all within the same framework. Want to test different discount rates on your sales model? Just create new scenarios, tweak the parameters, and rerun them.
Taipy vs. Other Pipeline Management Tools
You may be wondering, how does Taipy compare to other popular pipeline orchestration tools like Apache Airflow, Luigi, or Prefect? While those tools are great for managing task scheduling across distributed environments, Taipy stands out by focusing on Python simplicity, especially when it comes to scenario management and what-if analysis.
Airflow/Luigi/Prefect: Generally focus on orchestrating ETL processes, scheduling, and monitoring workflows.
Taipy: It not only provides workflow orchestration but also simplifies what-if analysis with its unique scenario abstraction, allowing you to model various outcomes seamlessly.
For developers looking to handle complex data workflows in Python with minimal setup, Taipy offers a more straightforward, code-first approach.
Conclusion: Divide and Conquer with Taipy
The Divide and Conquer strategy wins every time, no matter the size or complexity of your data problems! With Taipy, you can handle everything from data integration to task orchestration and what-if analysis, all in one place. And you can finalize with data visualization as well.
Ready to give Taipy a try? Check out the GitHub repo and see how it can streamline your data workflows today!
Star Taipy ⭐️
Don’t forget to leave a star ⭐ and share your feedback or scenarios you've worked on in the comments below!
以上是What-if I told you complex Data Integration makes good Task Orchestration?的详细内容。更多信息请关注PHP中文网其他相关文章!

热AI工具

Undresser.AI Undress
人工智能驱动的应用程序,用于创建逼真的裸体照片

AI Clothes Remover
用于从照片中去除衣服的在线人工智能工具。

Undress AI Tool
免费脱衣服图片

Clothoff.io
AI脱衣机

Video Face Swap
使用我们完全免费的人工智能换脸工具轻松在任何视频中换脸!

热门文章

热工具

记事本++7.3.1
好用且免费的代码编辑器

SublimeText3汉化版
中文版,非常好用

禅工作室 13.0.1
功能强大的PHP集成开发环境

Dreamweaver CS6
视觉化网页开发工具

SublimeText3 Mac版
神级代码编辑软件(SublimeText3)
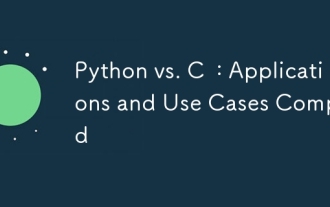
Python适合数据科学、Web开发和自动化任务,而C 适用于系统编程、游戏开发和嵌入式系统。 Python以简洁和强大的生态系统着称,C 则以高性能和底层控制能力闻名。
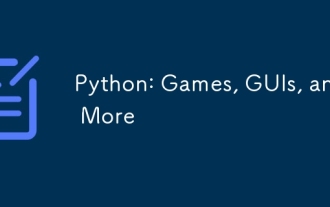
Python在游戏和GUI开发中表现出色。1)游戏开发使用Pygame,提供绘图、音频等功能,适合创建2D游戏。2)GUI开发可选择Tkinter或PyQt,Tkinter简单易用,PyQt功能丰富,适合专业开发。
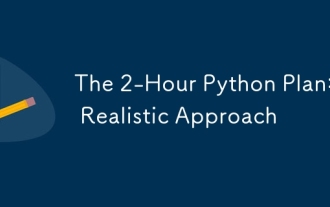
2小时内可以学会Python的基本编程概念和技能。1.学习变量和数据类型,2.掌握控制流(条件语句和循环),3.理解函数的定义和使用,4.通过简单示例和代码片段快速上手Python编程。
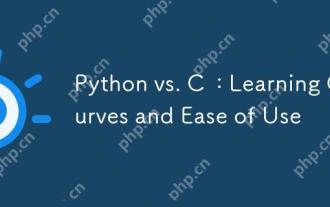
Python更易学且易用,C 则更强大但复杂。1.Python语法简洁,适合初学者,动态类型和自动内存管理使其易用,但可能导致运行时错误。2.C 提供低级控制和高级特性,适合高性能应用,但学习门槛高,需手动管理内存和类型安全。
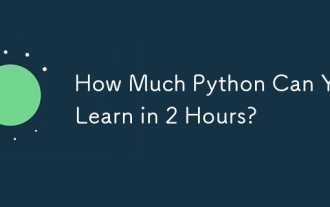
两小时内可以学到Python的基础知识。1.学习变量和数据类型,2.掌握控制结构如if语句和循环,3.了解函数的定义和使用。这些将帮助你开始编写简单的Python程序。
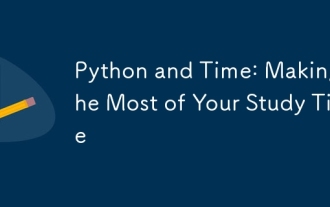
要在有限的时间内最大化学习Python的效率,可以使用Python的datetime、time和schedule模块。1.datetime模块用于记录和规划学习时间。2.time模块帮助设置学习和休息时间。3.schedule模块自动化安排每周学习任务。
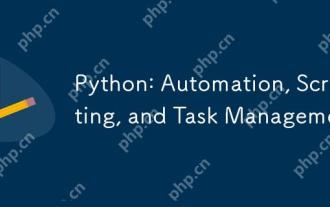
Python在自动化、脚本编写和任务管理中表现出色。1)自动化:通过标准库如os、shutil实现文件备份。2)脚本编写:使用psutil库监控系统资源。3)任务管理:利用schedule库调度任务。Python的易用性和丰富库支持使其在这些领域中成为首选工具。
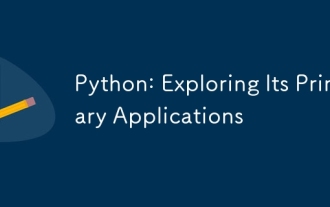
Python在web开发、数据科学、机器学习、自动化和脚本编写等领域有广泛应用。1)在web开发中,Django和Flask框架简化了开发过程。2)数据科学和机器学习领域,NumPy、Pandas、Scikit-learn和TensorFlow库提供了强大支持。3)自动化和脚本编写方面,Python适用于自动化测试和系统管理等任务。
