最新的最佳及时工程技术的年度汇编
对于那些可能是我专栏的人的人,我广泛探讨了AI的最新进展,包括诸如AI的体现,AI推理,AI的高科技突破,AI及时的工程,AI培训,AI,AI,AI,AI,AI,建筑,建筑和新硬件的AI,AI,AI,AI等的新硬件等主题。这是一种折衷的组合。
我通常将迅速的工程在我的AI主题中包括在圆形旋转中,并旨在使最新的突破和示例性的提示方法提升。我的覆盖范围广泛地研究了提示的最佳实践。我更喜欢识别并建议仅迅速得到的工程实践,这些实践得到实践研究的坚实支持,并且我利用自己每天使用生成AI。
及时工程是一个努力领域,它仍然具有至关重要的价值。我预测,迅速的工程具有长腿,并且需要具有足够的技能和技术来熟练有效地进行。正是由于这个原因,我将及时的及时工程前进,并将最佳的新技术引起读者的注意。
有关我对最新AI进步,发现和热门AI创新的总体覆盖,请参阅此处的链接。
此及时的工程汇编简介
在进行汇编之前,有几句话。
简要概述了每种技术,并提供了一个方便的链接,该链接与我的详细覆盖范围提供了说明,该链接说明了如何积极执行该技术并仔细地指出了必要的Ins和Outs。这些深入的文章也显示了示例。
我在2024年5月发布了类似的汇编(请参阅此处的链接),当时包含大约50个重要提示技术。从那以后,我继续定期介绍最新的及时工程。因此,我包括了2024年后期及2025年后期的附加及时工程帖子。总计82个Keystone提示技术。请注意,我的许多其他帖子也提到了临时促进练习,并在这里和那里提供技巧和见解,而我在这里介绍的措施则介绍了整个专栏。
我在演讲和谈话中遇到的最常见问题之一是,某人如何成为一名熟练的及时工程师。
这是我的建议。
仔细研究我在这里布置的每种及时的工程技术。确保使用所提供的在线链接,并充分阅读基于每种技术的详细指示(没有跳过,没有闲置的眼球)。尝试在您喜欢的生成AI应用中广泛使用该技术。测试自己,以仔细检查您真正知道如何使用每种技术。诚实。公平而平方。
完成该任务后,我想说您正在成为一名顶级促进工程师的路。随访是使用这些技术练习,并完全舒适地触手可及,并且很容易包含在您的及时工程心理工具包中。
我推荐的及时工程技术
我已经按字母顺序列出了这些技术。
关于每种技术的命名,没有标准化的行业命名约定,因此,我使用了我认为最常使用的名称或短语。目的是调用广义指示,以便您立即在每种技术所带来的正确的球场上降落。
开始了。
附加提示
您可以使用特殊的附加组件插入生成AI并有助于生成提示或调整提示。有关各种示例以及有关提示附加组件的性质和使用的进一步详细指示,请参阅此处的链接上的覆盖范围。
代理AI提示
代理AI很热。这个想法是,您精心使用生成的AI,端到端执行任务。一个受欢迎的例子是使用生成的AI,不仅建议计划旅行,还可以进行所有飞行和酒店预订。代理AI的出现还提出了需要额外的及时工程技术,以适应AI代理。有关各种示例以及有关代理AI提示性质和使用的进一步详细指示,请参阅此处的链接上的覆盖范围。
AI幻觉避免提示
关于生成AI的最紧迫的问题之一是,AI可以在计算上构成似乎被描绘成真理的虚假性,这是一个被称为AI幻觉的问题(我不喜欢标语,因为它倾向于拟人化AI,但不幸的是,它是一个phrase,我们似乎被认为是phrase,并且似乎陷入困境)。有关AI幻觉性质的各种示例和进一步的详细指示,请参见我在此处的链接,链接以及此处的链接上的广泛报道。
原子(AOT)提示
该技术扩展了著名的经过思考的技术。它是这样的。您告诉AI执行逐步推理,并通过将问题划分为最原子的步骤来做到这一点。美丽是,这不仅倾向于在弄清适当的步骤方面保持AI诚实,而且AI还可以在平行性的AI中并行处理步骤的执行。有关各种示例以及有关提示原子的性质和使用的进一步详细指示,请参见我在此处的链接上的覆盖范围。
击败“反向诅咒”提示
生成的AI因在演绎逻辑的反面方面遇到困难而闻名;因此,请确保熟悉可能降低或克服所谓的“反向诅咒”的方法。有关各种示例以及有关击败反向诅咒提示的性质和使用的进一步详细指示,请参阅此处的链接上的覆盖范围。
“在你的脚趾上”提示
“在您的脚趾上”一词可以用来使生成的AI在产生响应时更彻底,尽管在使用提示技术时需要牢记需要注意的警告和限制。有关各种示例以及有关“脚趾上”提示的进一步详细指示,请参见我在此处的链接上的覆盖范围。
浏览提示
一种常见的提示技术包括写作提示,以寻求锻炼或欺负生成的AI。您需要在使用此类提示时要谨慎。有关各种示例和有关浏览提示的进一步详细指示,请参见我在此处的链接上的覆盖范围。
用于提示的目录或框架
迅速的框架或目录试图对您进行分类并呈现以制作和利用提示的基石方法。有关及时工程框架或目录的性质和使用的各种示例以及进一步的详细指示,请参见此处的链接的覆盖范围。
确定性和不确定性提示
您可以在提示中明确指出,您希望生成AI在提供问题的答案时会发出一定程度的确定性或不确定性。有关各种示例以及有关促使生成AI的确定性和不确定性隐藏作用的性质和使用的进一步详细指示,请参阅此处的链接上的覆盖范围。
连续链(CCOT)提示
假设我们没有处理在这里和那里流动的代币,而是采用了思想链(COT)提示方法,并将其进一步向下移动。它是这样的。一个组件将从其他组件中获得一项经过思考的链条。接收COT的组件将其用作赠品,以执行组件应该做的任何事情。该组件的结果是另一个新设计的思想链,然后沿着线进一步流动。有关各种示例以及有关链接链的提示的性质和使用的进一步详细指示,请参见我在此处的链接上的覆盖范围。
密度链(COD)提示
设计摘要的精明方法涉及一种称为密度链(COD)的巧妙提示策略,该策略旨在增强生成AI,特别是获得出色或至少比平常的摘要。有关各种示例以及有关COD或密度链链提示的性质和使用的进一步详细指示,请参见我在此处的链接上的覆盖范围。
反馈链(COF)提示
经营链(COT)的变体由回馈链(COF)提示技术组成,这似乎降低了生成的AI幻觉程度。有关各种示例以及有关回馈链提示的性质和使用的进一步详细指示,请参见我在此处的链接上的覆盖范围。
经营链(COT)提示
经过深思熟虑(COT)的提示已被认为是最重要的提示技术之一。输入提示时,您可以通过简单地告诉生成AI逐步工作来调用COT。有关各种示例以及有关提示(COT)的性质和使用的进一步详细指示,请参阅此处的链接上的覆盖范围。
经过经过经过经过经过研究的分解促进
您可以通过额外的指示来补充传统的思维链(COT),该指示告诉生物AI在进行经过经过经过经过经过经过经过经验链的一代时会产生一系列问题和答案。您的目标是推动或生成生成的AI,以产生一系列子问题和子序列。有关各种示例以及有关利用分解分解的思想链的性质和使用的进一步详细的迹象,请参阅此处的链接的覆盖范围。
验证链(COV)提示
验证链(称为Cove或Cove,尽管有些人也称COV)是一种先进的及时工程技术,它在一系列的检查和符合量或双重检查中试图提高生成AI响应的有效性。有关各种示例以及有关COV或验证链链的使用的进一步详细指示,请参见此处的链接的覆盖范围。
清单提示
使用清单提示时,您会告诉生成AI为您希望AI解决的任何问题或问题提供清单。这样做有助于AI采取更结构化的方法。次要优点是您通过派生的清单验证了AI验证问题的所有问题或部分的所有问题或至少在求解过程中已解决或至少由AI考虑。有关各种示例以及有关清单提示的性质和使用的进一步详细指示,请参见我在此处的链接上的覆盖范围。
会话提示
提示在避免使用生成AI时似乎会采用的近视的一劳永逸的心态时,请保持流利和互动。有关各种示例以及有关对话提示性质和使用的进一步详细指示,请参见我在此处的链接上的覆盖范围。
会话放大及时工程(CAPE)
提示的高级模式涉及与生成AI进行来回对话,以使您的及时意图完全规定。有时,使用多个提示比一个提示更好。有关各种示例以及有关CAPE性质和使用的进一步详细指示,请参见我在此处的链接上的覆盖范围。
提示的深层效果
毫无疑问,您知道深击,而不同的角度涉及通过生成ai建立一个真实效果,即您自己的假版本是“真实”的,从您真正想设计假的数字双胞胎的意义上。有关各种示例以及有关通过提示从深层效果到Truefakes的性质和使用的进一步详细指示,请参阅此处的链接上的覆盖范围。
定向刺激提示(DSP)和提示
提示中使用微妙或有时高度透明的提示正式称为定向刺激提示(DSP),并且可以大大提高生成性AI响应。有关各种示例以及有关提示性质或方向刺激提示的性质和使用的进一步详细指示,请参阅此处的链接上的覆盖范围。
虚假信息检测和删除提示
社会面临的虚假信息和错误信息的数量不断增长,而令人遗憾的是,似乎不可阻挡。应对的一种值得注意的手段包括使用生成AI作为您的首选过滤器来检测虚假信息和错误信息。有关各种示例以及有关提示检测和减轻错误信息和虚假信息流的性质和使用的进一步详细指示,请参阅此处的链接上的我的覆盖范围。
加倍链条的提示
现在,许多最新的生成AI和LLM都具有内置功能,该功能会自动调用思想链。这很好。但是,对于那些习惯于通过提示直接援引思想链的人来说,存在新的困境。问题在于,如果您要求一项经过思考的链条,并且AI已经自动进行思考,那么加倍就可以加强作品。有关各种示例以及有关加倍链条链链提示的性质和使用的进一步详细指示,请参见此处的链接。
回声提示
ECHO提示就像名字所说:您告诉AI回声回您输入的提示查询。当适当地使用时,这会在AI产生的响应中产生显着和积极的差异。这是一种简单的技术,可以在情况下使用。有关各种示例以及有关回声提示性质和使用的进一步详细指示,请参见此处的链接。
情绪欺骗性的提示
用户已经发现,他们可以在使用正在运行客户服务在线聊天等的生成AI时表达自己的提示,以获取自己的方式。我对情感表达的提示更加深入,以提示我先前介绍了那些试图欺骗或指导AI朝着期望的方向指导AI的最新技巧。有关各种示例以及有关情感欺骗性提示的性质和使用的进一步详细指示,请参见此处的链接。
情绪表达的提示
在与生成AI交谈时,在提示中使用情感表达的措辞会有所不同吗?答案是肯定的。并且有一个合乎逻辑且完全计算的理由,原因是为什么生成AI对您对情感措辞的使用“反应”。有关各种示例以及有关情感措辞提示的性质和使用的进一步详细迹象,请参见我在此处的链接上的覆盖范围。
最终目标提示
强烈推荐的提示策略包括确定您的最终目标是在生成AI中工作,并旨在解决或深入研究特定主题或特定兴趣问题。有关各种示例和有关最终目标计划的进一步详细指示,请参见我在此处的链接上的覆盖范围。
论文压缩提示
有时,您没有得到摘要,而是想压缩一篇文章,这意味着它包含与原始源相同的单词,但抛出了不一定需要的单词。有关各种示例和有关论文压缩提示的进一步详细指示,请参见我在此处的链接上的覆盖范围。
专家角色提示
方便的提示技术涉及告诉生成AI假装成为给定领域的专家。然后,您会有AI答案提示,好像它是如此专家一样。通过让AI假装是众多的专家角色,互相辩论和挑战,可以进一步增强这种情况。有关各种示例和有关专家角色提示的进一步详细指示,请参见此处的链接。
公平思维的提示
您可以使用巧妙的提示,这些提示将使生成性AI倾斜在方向上,而不是已经熟悉的AI熟悉的偏见,旨在在生成的响应中获得更大的公平性。有关各种示例以及有关公平思维提示的性质和使用的进一步详细指示,请参见我在此处的链接上的覆盖范围。
退缩响应提示
如果您使用任何频率的生成AI,您偶尔会知道,AI会告诉您它不能或不会在提示中回答您提出的问题。您将获得AI已调整为提供的言论回应。有明智的方法可以响应后备响应。有关各种示例以及有关后备响应提示的进一步详细指示,请参见我在此处的链接上的覆盖范围。
翻转互动提示
您可以像以前那样翻转脚本,让生成的AI提出问题,而不是让您提出生成AI的问题。有关各种示例以及有关翻转交互的性质和使用的进一步详细指示,请参阅此处的链接上的覆盖范围。
游戏化提示
游戏化是提高促进技能的有用且引人入胜的方法。无论您是新手还是在写作提示上的专家,都可以明显地增强您的及时工程功能。有关各种示例以及有关游戏化提示性质和使用的进一步详细指示,请参见我在此处的链接上的覆盖范围。
自动生成提示
您可以要求生成AI为您创建提示,而不是直接撰写提示。这需要知道哪种提示将为您提供最好的AI生成的提示。有关各种介绍性示例和有关发起提示提示的进一步详细指示,请参阅此处的链接上的我的覆盖范围。
生成提示与手工
提示生成器本质上是使用生成AI为您生成提示。直接的想法是,您要告诉人工智能您想问的方面或指出您想要解决的问题,并会产生提示。提示发电机比自己编写提示更好吗?我比较何时最好的方法。有关各种示例以及有关提示生成器与副构图的进一步详细指示,请参见我在此处的链接上的覆盖范围。
黑客马拉松提示
一种学习新提示和锻炼您的及时工程敏锐度的方便方法,需要参与及时的活动。及时的thon类似于编程黑客马拉松,除非编码,而是使用提示。有关及时及时的各种示例和进一步的详细指示,请参见我在此处的链接上的覆盖范围。
硬提示提示
硬提示是一个提示,它给生成的AI带来了一个特别困难或艰巨的问题。 AI可能根本无法解决问题,但至少会尝试这样做。 AI在尝试这样做时可能会消耗大量时间和费用。更糟糕的是,在试图解决在硬提示中提供的问题或问题的同时,实际上可能会发生所谓的AI幻觉并提供错误的结果。编写硬提示时存在权衡。有关各种示例和有关硬提示提示的进一步详细指示,请参见我在此处的链接上的覆盖范围。
非法或不允许提示
您是否知道大多数Generative AI应用程序的许可协议说您只能以各种严格规定的方式使用生成AI?有关各种示例以及有关被认为是非法提示的性质(即您不应该使用的)性质的进一步详细迹象,请在此处查看我的覆盖范围。
不完美的提示
不完美的提示可能非常有用。有关各种示例以及有关不完美提示的性质和使用的进一步详细指示,请参阅此处的链接上的覆盖范围。
导入文本作为提示技巧
在某些情况下,涉及将文本导入生成AI的情况,这些AI需要仔细的技能,并且需要正确的提示,以使文本合适并适当地注入。有关导入文本提示的各种示例以及进一步的详细指示,请参见此处的链接上的覆盖范围。
交错对话提示
大多数流行的生成AI应用都要求每个对话都与与AI的其他对话不同。最新的趋势需要进行对话的交织,并且需要重新思考您如何撰写提示。有关各种示例以及有关隔行对话提示的进一步详细指示,请参见我在此处的链接上的覆盖范围。
启动提示
提示时,明智的举动是要润滑滑雪或泵启动泵,也称为Kickstart提示,这涉及执行初始提示,该提示将生成的AI纳入您想要解决的任何主题或问题的凹槽中。有关各种示例和有关Kickstart提示性质的进一步详细指示,请参阅此处的链接上的覆盖范围。
知识蒸馏提示
当您想进行数据训练AI时,您可以使用另一个AI,该AI基本上有助于将数据或“知识”从该来源AI传输到目标AI。这被称为知识蒸馏。涉及许多促使复杂性。有关各种示例以及有关知识蒸馏提示性质的进一步详细指示,请参见我在此处的链接上的覆盖范围。
大型概念模型(LCM)提示
一些人认为,生成AI的传统基础将不得不改变,例如拥抱使用“概念”,而不是仅专注于单词和令牌。这些新方法利用了大型概念模型(LCM)。我们仍处于早期LCMS是突破性的打击还是DUD的早期。了解LCM会在提示这种方法时为您提供见解。有关LCMS性质的各种示例和进一步的详细指示,请参阅此处的链接上的覆盖范围。
最小的提示
最小至最少的提示(LTM)是一种涉及指导生成AI首先在最小零件上工作的技术,然后继续进行更艰难的部分(替代方法是至少或MTL提示)。有关LTM和MTL提示性质的各种示例以及进一步的详细指示,请参阅此处的链接上的覆盖范围。
逻辑(批次)提示
逻辑涉及告诉生成AI以高度逻辑的方式解决问题或问题,并尽可能地融入逻辑推理。这样做时,有三个至关重要的要素:(1)逻辑提取,(2)使用命题得出解决方案,以及(3)以简单的语言生成解释。有关各种示例和有关逻辑提示性质的性质的进一步详细指示,请参见我在此处的链接上的覆盖范围。
提示中的宏
类似于在电子表格中使用宏,您可以在生成AI工作时在提示中使用宏。有关各种示例以及有关及时宏的性质和使用的进一步详细指示,请参阅此处的链接上的覆盖范围。
大型梅加角色提示
现在,Mega-Personas的提示已进一步扩展到Mega-Mega角色提示。巨型 - 佩尔森(Mega-Personas)通常是数千个角色,而大型梅加(Mega-Mega)需要援引数百万甚至数十亿个角色。有关各种示例以及有关Mega-Mega角色的性质和使用的进一步详细指示,请参见我在此处的链接上的覆盖范围。
巨型 - 彼得促进
Mega-Personas包括对多人提示的升级。您要求Generative AI伪装成数千个假装。有关各种示例以及有关大型 - 佩纳斯提示的性质和使用的进一步详细指示,请参阅此处的链接上的覆盖范围。
meta-prompts
元提示是一种特殊的提示,可提供有关提示和提示性质的说明或指示。在最简单的用途中,如果您还不熟悉高级提示技术的来龙去脉,则元过程特别方便。 AI可以很容易地为您进行繁重的工作,并添加著名的措辞,从而提高您的原始提示。有关各种示例以及有关元过程性质和使用的进一步详细指示,请参阅此处的链接上的覆盖范围。
多人提示
通过Multi-Persona提示,您可以获得生成的AI来模拟一个或多个角色。有关各种示例以及有关多人提示性质和使用的进一步详细指示,请参见我在此处的链接上的覆盖范围。
近乎内存的记忆提示
有持续的猜测,我们正朝着生成AI和LLM的近距离内存迈进。这个想法是,与AI交谈时,LLM“知道”的现有局限性不是现有的限制,而是可以随时可以访问所需的尽可能多的内存。当前的提示要求您了解盛行的内存限制,而如果我们确实达到了近乎无限的内存,则您的提示应进行相应调整。有关各种示例以及有关近乎内存的性质的性质和使用的进一步详细指示,请参阅此处的链接上的覆盖范围。
克服“愚蠢”提示
知道何时使用简洁或简短的措辞(不公平地表示为“愚蠢”提示),而不是使用更多的冗长或流利的措辞是一项技能,这是一项精通工程及时工程的技能。有关各种示例以及有关避免提示愚蠢的性质和使用的进一步详细指示,请参阅此处的链接上的覆盖范围。
持续的上下文和自定义指令提示
您可以轻松建立一个将持续存在的上下文,并确保生成型AI对您认为重要的东西有所了解,通常是通过自定义说明来设置的。有关各种示例以及有关持久上下文和自定义说明的性质和使用的进一步详细指示,请参见我在此处的链接上的覆盖范围。
窃的促进
您的提示可以通过设计或偶然地进行stoke生成的AI,以产生包含窃内容的响应。要非常小心,因为您可能会因窃而承担任何责任。有关各种示例和进一步的详细指示,可能会引起窃的性质和提示的使用,请参见我在此处的链接和链接的覆盖范围。
礼貌提示
关于生成AI的研究的一个令人惊讶的见解是提示使用请求,并感谢您可以搅动AI以产生更好的结果。确保在提示时使用礼貌,尽管不要过分地在这种措辞中明智。有关各种示例和有关礼貌提示的进一步详细迹象,请参见我在此处的链接上的报道。
先发制检测提示
研究表明,您通常可以成功地告诉生成AI,以避免在产生AI幻觉时纠缠在一起,尤其是AI通常会遵守。因此,要明确警告AI通过在您的提示中明确说出而不要产生AI纵容。这被称为抢先检测提示。有关各种示例和有关先发制检测提示的进一步详细指示,请参见我在此处的链接上的覆盖范围。
隐私保护提示
您是否意识到,当您进入生成AI提示时,通常不能保证您输入的数据或信息将被私有或机密吗?有关各种示例,以及有关性质和使用的进一步详细指示 提示可能会赋予隐私或机密性的提示,请参阅此处的链接上的覆盖范围。
迅速发展生命周期(PDLC)
类似于编程或软件工程如何具有系统开发生命周期(SDLC),也可以说及时工程。通常被称为迅速发展生命周期(PDLC),市场上有许多变化。必须知道PDLC包含的内容以及如何提高您的提示技能是必须的。有关PDLC的性质和使用的各种示例以及进一步的详细指示,请参阅此处的链接上的覆盖范围。
提示盾牌和聚光灯提示
由于试图使生成性AI超越其过滤器和通常的保护措施的各种黑客努力,因此出现了迅速的盾牌和聚光灯提示。这是您需要知道的有用的摘要。有关各种示例以及有关提示盾牌性质和焦点提示性质的进一步详细指示,请参阅此处的链接上的覆盖范围。
及时编码提示
您可以输入提示,这些提示可以告诉生成AI生成常规的编程代码,并为您提供基本上编写程序。有关各种示例以及有关提示制作编程代码的性质和使用的进一步详细指示,请参见我在此处的链接上的覆盖范围。
目的提示
缺乏内部绑定目的的AI系统可能会以人类无目的而徘徊的类似方式徘徊。我们应该确保AI系统始终具有编纂的目的。然后,AI在采取任何行动或执行可以召集的任何能力时可以参考目的。有关各种示例以及有关目的提示性质和使用的进一步详细指示,请参见我在此处的链接上的覆盖范围。
推理模型提示
生成AI和LLM的最新迭代中最大的变化之一是,AI制造商选择将思想链(COT)推理直接包括在AI的内部机制中。这是一个巨大的变化,值得密切关注。提示的性质需要反映出AI将自动执行逐步推理。有关各种示例以及有关推理模型提示的性质和使用的进一步详细指示,请参见我在此处的链接上的覆盖范围。
重新提示提示
如果您不太确定如何用特定的提示措辞,则可以“按原样”输入它,并告诉AI执行复制和回程。这告知AI,而不是直接解释您的提示,它应该首先重新调整输入的提示,这希望可以改善提示,然后继续回复此更好的提示。有关各种示例以及有关复制和回答提示的性质和使用的进一步详细指示,请参阅此处的链接上的覆盖范围。
重新阅读提示
当今大多数流行的生成AI应用程序倾向于在输入的提示下进行一次通行证。第二次通行证可能有助于对问题进行进一步的详细检查。一种清理可能错过或错误计算的问题的清理。您可以使用重新阅读提示技术来调用第二次通过。有关各种示例以及有关重新阅读提示性质和使用的进一步详细指示,请参阅此处的链接上的覆盖范围。
响应时间加速提示
Several clever approaches can be used to speed up the response time to your prompts. The wording of your prompt makes a significant difference in the amount of processing time that will be consumed by the AI when generating a response. These techniques aim to reduce latency or delays in getting generated responses, thus essentially speeding up your response time. For various examples and further detailed indications about the nature and use of response time speed-up prompting, see my coverage at the link here.
Retrieval-Augmented Generation (RAG) Prompting
Retrieval-augmented generation (RAG) is hot and continues to gain steam. You provide external text that gets imported and, via in-context modeling, augments the data training of generative AI. For various examples and further detailed indications about the nature and use of retrieval-augmented generation (RAG), see my coverage at the link here.
Self-Ask Prompting
Self-ask prompting consists of you telling generative AI to solve problems by pursuing an internal question-and-answer divide-and-conquer approach that is to be made visible to you during the solving process. The AI is performing a stepwise self-ask that is an added-value version of chain-of-thought (CoT). For various examples and further detailed indications about the nature and use of self-ask prompting, see my coverage at the link here.
Self-Reflection Prompting
You can enter a prompt into generative AI that tells the AI to essentially be (in a manner of speaking) self-reflective by having the AI double-check whatever generative result it has pending or that it has recently produced. For various examples and further detailed indications about the nature and use of AI self-reflection and AI self-improvement for prompting purposes, see my coverage at the link here.
Sensitivities Prompting
Research shows that there are three keystone sensitivities about generative AI that you need to be aware of when composing your prompts. The sensitivity has to do with the scale or size of the underlying AI model, the aspect of whether topics-based data training of the AI has occurred, and whether you opt to use an example in your prompt (a so-called one-shot). For various examples and further detailed indications about the nature and use of AI sensitivities prompting, see my coverage at the link here.
Show-Me Versus Tell-Me Prompting
Show-me consists of devising a prompt that demonstrates to the generative AI an indication of what you want (show it), while tell-me entails devising a prompt that gives explicit instructions delineating what you want to have done (tell it). For various examples and further detailed indications about the nature and use of the show-me versus tell-me prompting strategy, see my coverage at the link here.
Sinister Prompting
People are using sinister prompts to get generative AI to do foul things, such as scams and the like. I don't want you to do this, but I also think it is valuable for you to know what sinister prompts do and how they work, alerting you to avoid them and not inadvertently fall into the trap of one. For various examples and further detailed indications about the nature and use of sinister prompting, see my coverage at the link here.
Skeleton-of-Thought (SoT) Prompting
Via a prompt akin to Chain-of-Thought (CoT), you tell the generative AI to first produce an outline or skeleton for whatever topic or question you have at center stage, employing a skeleton-of-thought (SoT) method to do so. For various examples and further detailed indications about the nature and use of the skeleton-of-thought approach for prompt engineering, see my coverage at the link here.
Small Language Model (SLM) Prompting
If you've not heard about small language models (SLMs), that's perfectly understandable since they are still not quite up to par. There are a wide variety of experimental SLMs, and some are very handy while others are clunky and less appealing. You need to adjust your prompting approach when using SLMs versus conventional LLMs. For various examples and further detailed indications about the nature and use of prompting for SLMs, see my coverage at the link here.
Star Trek Trekkie Lingo Prompting
An unusual discovery by researchers showcased that using Star Trek Trekkie lingo in your prompts can improve generative AI results. Downsides exist and can undercut your efforts by inadvertent misuse or overuse of this technique. For various examples and further detailed indications about Trekkie prompting, see my coverage at the link here.
Step-Around Prompting Technique
At times, the prompts that you seek to use in generative AI are blocked by the numerous filters that the AI maker has put in place. You can use the step-around prompting technique to get around those blockages. For various examples and further detailed indications about step-around prompting, see my coverage at the link here.
“Take A Deep Breath” Prompting
The prompting phrase of “Take a deep breath” has become lore in prompt engineering, but it turns out that there are limitations and circumstances under which this wording fruitfully works. For various examples and further detailed indications about the nature and use of the take a deep breath prompting, see my coverage at the link here.
Target-Your-Response (TAYOR) Prompting
Target-your-response (TAYOR) is a prompt engineering technique that entails telling generative AI the desired look and feel of to-be-generated responses. For various examples and further detailed indications about the nature and use of TAYOR or target-your-response prompting, see my coverage at the link here.
Temperature Settings Prompting
The temperature setting for generative AI determines how varied the responses by generative AI will be. You can either have the AI produce relatively straightforward and somewhat predictable responses (that's via the use of a low temperature), or you can heat things up and use high temperatures to prod AI toward producing seemingly more creative and less predictable responses. Some AI allows you to modify the temperature settings on your own via prompts. For various examples and further detailed indications about the nature and use of temperature settings, see my coverage at the link here.
Thinking Time Prompting
The latest iterations of generative AI and LLMs now have logical reasoning built directly into the AI architecture, which has led to new options about how much so-called thinking time you want the AI to undertake when responding to a prompt. This processing time will determine the depth and likely aptness of the response. Various prompting avenues arise when specifying the thinking time aspects. For various examples and further detailed indications about the nature and use of thinking time prompting, see my coverage at the link here.
Tree-of-Thoughts (ToT) Prompting
Tree-of-thoughts (ToT) is an advanced prompting technique that involves telling generative AI to pursue multiple avenues or threads of a problem (so-called “thoughts”) and figure out which path will likely lead to the best answer. For various examples and further detailed indications about the nature and use of ToT or tree-of-thoughts prompting, see my coverage at the link here.
Trust Layers For Prompting
Additional components outside of generative AI are being set up to do pre-processing of prompts and post-processing of generated responses, ostensibly doing so to increase a sense of trust about what the AI is doing. For various examples and further detailed indications about the nature and use of trust layers for aiding prompting, see my coverage at the link here.
Vagueness Prompting
The use of purposefully vague prompts can be advantageous for spurring open-ended responses that might land on something new or especially interesting. For various examples and further detailed indications about the nature and use of vagueness while prompting, see my coverage at the link here.
Making A Checklist Of The Prompting Techniques
I mentioned earlier that you might consider trying out the prompt engineering techniques that I've listed, especially ones that you don't already know.
To help you with that fruitful exercise, here's my suggestion. Create a spreadsheet that contains the checklist shown below of the listed prompting techniques. Make a column that you can mark as to whether you are familiar with the specific technique, do so by using a score ranging from 0 to 5, wherein 0 is that you don't know it at all, while the highest score of 5 is that you know it like the back of your hand. Be straightforward and don't give a fake score. Put your real score. This list is solely for your own benefit.
Make another column that has a score showing what you want to become in that technique. For example, suppose that right now, you start as a self-rated 1 on a particular technique and want to end up at a self-rated 4. Finally, include an additional column that will contain a target date of when you hope to attain the heightened score.
You can now use that spreadsheet as your career planning guide for prompt engineering purposes. Keep it updated as you proceed along in your adventure as a prompt engineer who wants to do the best that you can.
Whether you undertake that treasured challenge or not, here's the list with numbers shown as an easy reference (the numbering doesn't represent priority or ranking; they are just a handy reference number), and the list is still in the same alphabetical order as shown above.
Here's the numbered list:
- Add-On Prompting
- Agentic AI Prompting
- AI Hallucination Avoidance Prompting
- Atom-of-Thoughts (AoT) Prompting
- Beat the “Reverse Curse” Prompting
- “Be On Your Toes” Prompting
- Browbeating Prompts
- Catalogs Or Frameworks For Prompting
- Certainty And Uncertainty Prompting
- Chain-of-Continuous-Thought (CCoT) Prompting
- Chain-of-Density (CoD) Prompting
- Chain-of-Feedback (CoF) Prompting
- Chain-of-Thought (CoT) Prompting
- Chain-of-Thought Factored Decomposition Prompting
- Chain-of-Verification (CoV) Prompting
- Checklist Prompting
- Conversational Prompting
- Conversational-Amplified Prompt Engineering (CAPE)
- DeepFakes To TrueFakes Prompting
- Directional Stimulus Prompting (DSP) And Hints
- Disinformation Detection And Removal Prompting
- Doubling-Up Chain-of-Thought Prompting
- Echo Prompting
- Emotionally Deceptive Prompting
- Emotionally Expressed Prompting
- End-Goal Prompting
- Essay-Compression Prompting
- Expert Personas Prompting
- Fair-Thinking Prompting
- Fallback Response Prompting
- Flipped Interaction Prompting
- Gamification Prompting
- Generating Prompts Automatically
- Generating Prompts Versus By-Hand
- Hackathons Prompt-A-Thon
- Hard Prompts Prompting
- Illicit Or Disallowed Prompting
- Imperfect Prompting
- Importing Text As Prompting Skill
- Interlaced Conversations Prompting
- Kickstart Prompting
- Knowledge Distillation Prompting
- Large Concept Model (LCM) Prompting
- Least-to-Most Prompting
- Logic-of-Thought (LoT) Prompting
- Macros In Prompts
- Mega-Mega Personas Prompting
- Mega-Personas Prompting
- Meta-Prompts
- Multi-Persona Prompting
- Near-Infinite Memory Prompting
- Overcoming “Dumbing Down” Prompting
- Persistent Context And Custom Instructions Prompting
- Plagiarism Prompting
- Politeness Prompting
- Preemptive Detection Prompting
- Privacy Protection Prompting
- Prompt Development Life Cycle (PDLC)
- Prompt Shields and Spotlight Prompting
- Prompt-To-Code Prompting
- Purpose Prompting
- Reasoning Model Prompting
- Rephrase-and-Respond prompting
- Re-Read Prompting
- Response Time Speed-up Prompting
- Retrieval-Augmented Generation (RAG) Prompting
- Self-Ask Prompting
- Self-Reflection Prompting
- Sensitivities Prompting
- Show-Me Versus Tell-Me Prompting
- Sinister Prompting
- Skeleton-of-Thought (SoT) Prompting
- Small Language Model (SLM) Prompting
- Star Trek Trekkie Lingo Prompting
- Step-Around Prompting Technique
- “Take A Deep Breath” Prompting
- Target-Your-Response (TAYOR) Prompting
- Temperature Settings Prompting
- Thinking Time Prompting
- Tree-of-Thoughts (ToT) Prompting
- Trust Layers For Prompting
- Vagueness Prompting
I realize this might seem like a daunting list.
I can hear the trolls commenting that this is way too much and that there is no possible way for someone to spend the time and energy needed to learn them all. We each have our daily jobs to deal with and work-life balances that need to be balanced.
Yes, I get that.
My suggestion is that you prioritize the ones that seem to best fit your likely needs as a prompt engineer and focus on those as your mainstay priority. The others you can get around to trying out in your spare time (well, if you ever can squeeze out any spare time).
Prompt Engineering And Ongoing Learning
Lifelong learning.
That's what everyone is talking about these days. We are told time and again that we need to be lifelong learners. I wholeheartedly agree. This comes up here because the latest and greatest in prompt engineering is constantly changing. There are new ideas brewing. New AI research efforts are pending. It is a glorious time to be using generative AI.
I will keep covering prompt engineering and bringing you the newest prompts.
Keep your eyes and ears open, and I'll do my best to make sure you can be a lifelong learner, authentically and profitably.
以上是最新的最佳及时工程技术的年度汇编的详细内容。更多信息请关注PHP中文网其他相关文章!

热AI工具

Undresser.AI Undress
人工智能驱动的应用程序,用于创建逼真的裸体照片

AI Clothes Remover
用于从照片中去除衣服的在线人工智能工具。

Undress AI Tool
免费脱衣服图片

Clothoff.io
AI脱衣机

AI Hentai Generator
免费生成ai无尽的。

热门文章

热工具

记事本++7.3.1
好用且免费的代码编辑器

SublimeText3汉化版
中文版,非常好用

禅工作室 13.0.1
功能强大的PHP集成开发环境

Dreamweaver CS6
视觉化网页开发工具

SublimeText3 Mac版
神级代码编辑软件(SublimeText3)

热门话题
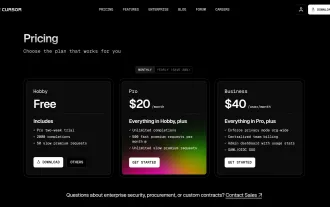
Vibe编码通过让我们使用自然语言而不是无尽的代码行创建应用程序来重塑软件开发的世界。受Andrej Karpathy等有远见的人的启发,这种创新的方法使Dev
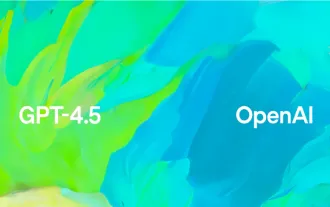
2025年2月,Generative AI又是一个改变游戏规则的月份,为我们带来了一些最令人期待的模型升级和开创性的新功能。从Xai的Grok 3和Anthropic的Claude 3.7十四行诗到Openai的G
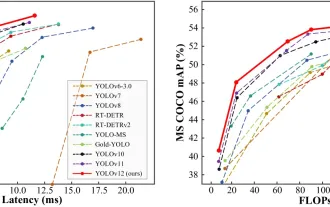
Yolo(您只看一次)一直是领先的实时对象检测框架,每次迭代都在以前的版本上改善。最新版本Yolo V12引入了进步,可显着提高准确性
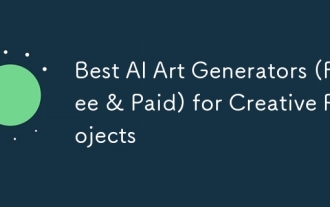
本文回顾了AI最高的艺术生成器,讨论了他们的功能,对创意项目的适用性和价值。它重点介绍了Midjourney是专业人士的最佳价值,并建议使用Dall-E 2进行高质量的可定制艺术。
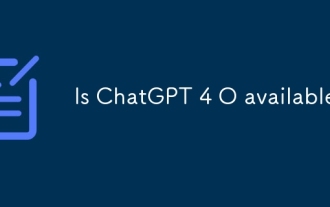
Chatgpt 4当前可用并广泛使用,与诸如ChatGpt 3.5(例如ChatGpt 3.5)相比,在理解上下文和产生连贯的响应方面取得了重大改进。未来的发展可能包括更多个性化的间
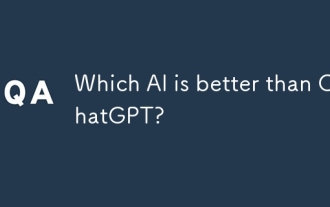
本文讨论了AI模型超过Chatgpt,例如Lamda,Llama和Grok,突出了它们在准确性,理解和行业影响方面的优势。(159个字符)
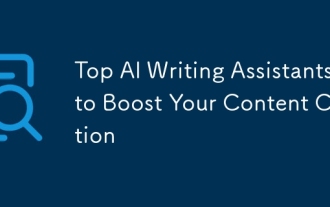
文章讨论了Grammarly,Jasper,Copy.ai,Writesonic和Rytr等AI最高的写作助手,重点介绍了其独特的内容创建功能。它认为Jasper在SEO优化方面表现出色,而AI工具有助于保持音调的组成
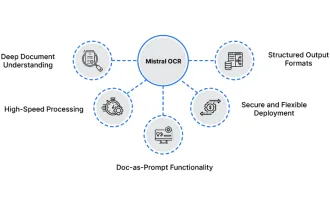
MISTRAL OCR:通过多模式文档理解彻底改变检索效果 检索增强的生成(RAG)系统具有明显高级的AI功能,从而可以访问大量的数据存储,以获得更明智的响应
