如何在Python中对时间序列数据进行重采样
时间序列数据是在固定时间间隔内收集的观测序列。这些数据可以来自于任何领域,如金融、经济、健康和环境科学。我们收集的时间序列数据有时可能具有不同的频率或分辨率,这可能不适合我们的分析和数据建模过程。在这种情况下,我们可以通过上采样或下采样来重新采样时间序列数据,从而改变时间序列的频率或分辨率。本文将介绍不同的方法来上采样或下采样时间序列数据。
Upsampling
Upsampling means increasing the frequency of the time series data. This is usually done when we need a higher resolution or more frequent observations. Python provides several methods for upsampling time series data, including linear interpolation, nearest neighbor interpolation, and polynomial interpolation.
Syntax
DataFrame.resample(rule, *args, **kwargs) DataFrame.asfreq(freq, method=None) DataFrame.interpolate(method='linear', axis=0, limit=None, inplace=False, limit_direction='forward', limit_area=None)
在这里,
The resample function is a method provided by the pandas library to resample time series data. It is applied on a DataFrame and takes the rule parameter, which specifies the desired frequency for resampling. Additional arguments (*args) and keyword arguments (**kwargs) can be provided to customize the resampling behavior, such as specifying the aggregation method or handling missing values.
The asfreq method is used in conjunction with the resample function to convert the frequency of the time series data. It takes the freq parameter, which specifies the desired frequency string for the output. The optional method parameter allows specifying how to handle any missing values introduced during the resampling process, such as forward filling, backward filling, or interpolation.
插值方法用于填充时间序列数据中的缺失值或间隙。它根据指定的方法(例如'linear'、'nearest'、'spline')进行插值,以估计现有观测值之间的值。额外的参数可以控制插值的轴,连续NaN值的填充限制,以及是否在原地修改DataFrame或返回一个新的DataFrame。
线性插值
线性插值用于上采样时间序列数据。它通过在数据点之间绘制直线来填充间隙。可以使用pandas库中的resample函数实现线性插值。
Example
的中文翻译为:示例
In the below example, we have a time series DataFrame with three observations on non−consecutive dates. We convert the 'Date' column to a datetime format and set it as the index. The resample function is used to upsample the data to a daily frequency ('D') using the asfreq method. Finally, the interpolate method with the 'linear' option fills the gaps between the data points using linear interpolation. The DataFrame, df_upsampled, contains the upsampled time series data with interpolated values.
import pandas as pd # Create a sample time series DataFrame data = {'Date': ['2023-06-01', '2023-06-03', '2023-06-06'], 'Value': [10, 20, 30]} df = pd.DataFrame(data) df['Date'] = pd.to_datetime(df['Date']) df.set_index('Date', inplace=True) # Upsample the data using linear interpolation df_upsampled = df.resample('D').asfreq().interpolate(method='linear') # Print the upsampled DataFrame print(df_upsampled)
输出
Value Date 2023-06-01 10.000000 2023-06-02 15.000000 2023-06-03 20.000000 2023-06-04 23.333333 2023-06-05 26.666667 2023-06-06 30.000000
最近邻插值
Nearest neighbor interpolation is a simple method that fills the gaps between data points with the nearest available observation. This method can be useful when the time series exhibits abrupt changes or when the order of observations matters. The interpolate method in pandas can be used with the 'nearest' option to perform nearest neighbor interpolation.
Example
的中文翻译为:示例
In the above example, we use the same original DataFrame as before. After resampling with the 'D' frequency, the interpolate method with the 'nearest' option fills the gaps by copying the nearest available observation. The resulting DataFrame, df_upsampled, now has a daily frequency with the nearest neighbor interpolation.
import pandas as pd # Create a sample time series DataFrame data = {'Date': ['2023-06-01', '2023-06-03', '2023-06-06'], 'Value': [10, 20, 30]} df = pd.DataFrame(data) df['Date'] = pd.to_datetime(df['Date']) df.set_index('Date', inplace=True) # Upsample the data using nearest neighbor interpolation df_upsampled = df.resample('D').asfreq().interpolate(method='nearest') # Print the upsampled DataFrame print(df_upsampled)
输出
Value Date 2023-06-01 10.0 2023-06-02 10.0 2023-06-03 20.0 2023-06-04 20.0 2023-06-05 30.0 2023-06-06 30.0
下采样
降采样用于降低时间序列数据的频率,通常用于获得数据的更广泛视图或简化分析。Python提供了不同的降采样技术,例如在指定的时间间隔内取均值、求和或最大值。
Syntax
DataFrame.mean(axis=None, skipna=None, level=None, numeric_only=None, **kwargs)
在这里,聚合方法,如均值、和或最大值,在重新采样后应用于计算代表每个重新采样间隔内分组观测的单个值。这些方法通常在降采样数据时使用。它们可以直接应用于重新采样的DataFrame,也可以与重新采样函数结合使用,根据特定的频率(如每周或每月),通过指定适当的规则来聚合数据。
Mean Downsampling
的中文翻译为:平均下采样
均值降采样计算每个间隔内数据点的平均值。这种方法在处理高频数据并获得每个间隔的代表性值时非常有用。可以使用resample函数结合mean方法来执行均值降采样。
Example
的中文翻译为:示例
In the below example, we start with a daily time series DataFrame spanning the entire month of June 2023. The resample function with the 'W' frequency downsamples the data to weekly intervals. By applying the mean method, we obtain the average value within each week. The resulting DataFrame, df_downsampled, contains the mean-downsampled time series data.
import pandas as pd # Create a sample time series DataFrame with daily frequency data = {'Date': pd.date_range(start='2023-06-01', end='2023-06-30', freq='D'), 'Value': range(30)} df = pd.DataFrame(data) df.set_index('Date', inplace=True) # Downsampling using mean df_downsampled = df.resample('W').mean() # Print the downsampled DataFrame print(df_downsampled)
输出
Value Date 2023-06-04 1.5 2023-06-11 7.0 2023-06-18 14.0 2023-06-25 21.0 2023-07-02 27.0
Maximum Downsampling
最大降采样计算并设置每个间隔内的最高值。此方法适用于识别时间序列中的峰值或极端事件。在前面的示例中使用max而不是mean或sum允许我们执行最大降采样。
Example
的中文翻译为:示例
In the below example, we start with a daily time series DataFrame spanning the entire month of June 2023. The resample function with the 'W' frequency downsamples the data to weekly intervals. By applying the max method, we obtain the Maximum value within each week. The resulting DataFrame, df_downsampled, contains the maximum-downsampled time series data.
import pandas as pd # Create a sample time series DataFrame with daily frequency data = {'Date': pd.date_range(start='2023-06-01', end='2023-06-30', freq='D'), 'Value': range(30)} df = pd.DataFrame(data) df.set_index('Date', inplace=True) # Downsampling using mean df_downsampled = df.resample('W').max() # Print the downsampled DataFrame print(df_downsampled)
输出
Value Date 2023-06-04 3 2023-06-11 10 2023-06-18 17 2023-06-25 24 2023-07-02 29
结论
在本文中,我们讨论了如何使用Python对时间序列数据进行重新采样。Python提供了各种上采样和下采样技术。我们探讨了线性和最近邻插值用于上采样,以及均值和最大值插值用于下采样。您可以根据手头的问题使用任何一种上采样或下采样技术。
以上是如何在Python中对时间序列数据进行重采样的详细内容。更多信息请关注PHP中文网其他相关文章!

热AI工具

Undresser.AI Undress
人工智能驱动的应用程序,用于创建逼真的裸体照片

AI Clothes Remover
用于从照片中去除衣服的在线人工智能工具。

Undress AI Tool
免费脱衣服图片

Clothoff.io
AI脱衣机

AI Hentai Generator
免费生成ai无尽的。

热门文章

热工具

记事本++7.3.1
好用且免费的代码编辑器

SublimeText3汉化版
中文版,非常好用

禅工作室 13.0.1
功能强大的PHP集成开发环境

Dreamweaver CS6
视觉化网页开发工具

SublimeText3 Mac版
神级代码编辑软件(SublimeText3)
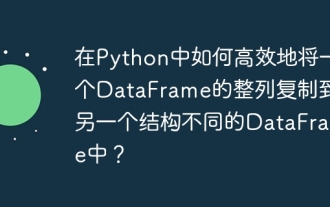
在使用Python的pandas库时,如何在两个结构不同的DataFrame之间进行整列复制是一个常见的问题。假设我们有两个Dat...
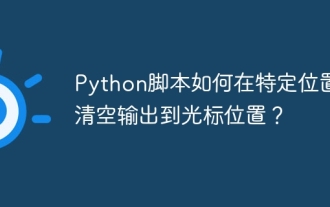
Python脚本如何在特定位置清空输出到光标位置?在编写Python脚本时,如何清空之前的输出到光标位置是个常见的...
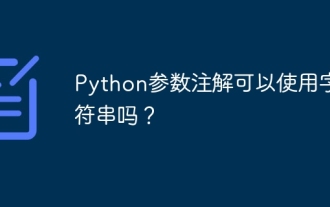
Python参数注解的另类用法在Python编程中,参数注解是一种非常有用的功能,可以帮助开发者更好地理解和使用函...
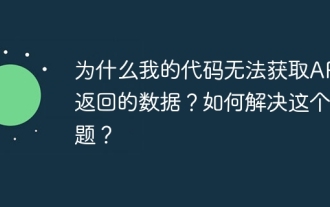
为什么我的代码无法获取API返回的数据?在编程中,我们常常会遇到API调用时返回空值的问题,这不仅让人困惑...
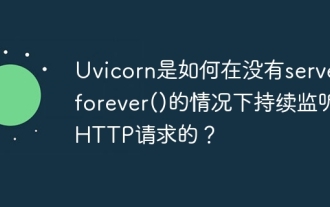
Uvicorn是如何持续监听HTTP请求的?Uvicorn是一个基于ASGI的轻量级Web服务器,其核心功能之一便是监听HTTP请求并进�...
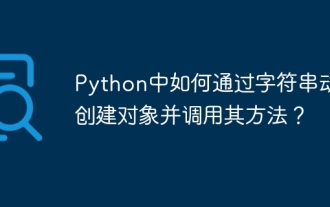
在Python中,如何通过字符串动态创建对象并调用其方法?这是一个常见的编程需求,尤其在需要根据配置或运行...
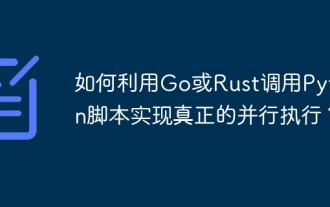
如何利用Go或Rust调用Python脚本实现真正的并行执行?最近在使用Python...
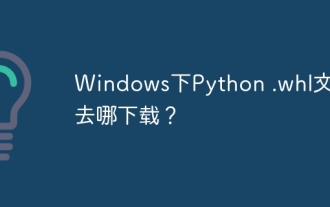
Python二进制库(.whl)下载途径探究许多Python开发者在Windows系统上安装某些库时会遇到难题。一个常用的解决方法�...
