仅用250美元,Hugging Face技术主管手把手教你微调Llama 3
我们熟悉的Meta推出的Llama 3、Mistral AI推出的Mistral和Mixtral模型以及AI21实验室推出的Jamba等开源大语言模型已经成为OpenAI的竞争对手。
在大多数情况下,用户需要根据自己的数据对这些开源模型进行微调,才能充分释放模型的潜力。
在单个GPU上使用Q-Learning对比小的大语言模型(如Mistral)进行微调不是难事,但对像Llama 370b或Mixtral这样的大模型的高效微调直到现在仍然是一个挑战。
因此,Hugging Face技术主管Philipp Schmid介绍了如何利用PyTorch FSDP和Q-Lora,并在Hugging Face的TRL、Transformers、peft和datasets库的帮助下,对Llama 3进行微调。除了FSDP,作者还对PyTorch 2.2更新后的Flash Attention v2进行了适配。
微调主要步骤如下:
- 设置开发环境
- 创建并加载数据集
- 使用 PyTorch FSDP、Q-Lora 和 SDPA 微调大语言模型
- 测试模型并进行推理
请注意:本文进行的实验是在英伟达(NVIDIA)H100和英伟达(NVIDIA)A10G GPU上创建和验证的。配置文件和代码针对4xA10G GPU进行了优化,每个GPU均配备24GB内存。如果使用者有更多的算力,第3步提到的配置文件(yaml文件)需要做相应的修改。
FSDP Q-Lora 背景知识
基于Answer.AI、Q-Lora创始人Tim Dettmers和Hugging Face共同参与的合作项目,作者对Q-Lora和PyTorch FSDP(完全共享数据并行)所能提供的技术支持进行了总结。
FSDP和Q-Lora的结合使用能够让用户在2个消费级GPU(24GB)上就能对Llama 270b或Mixtral 8x7B进行微调,细节可参考下面文章。其中Hugging Face的PEFT库对此有至关重要的作用。
文章地址:https://www.answer.ai/posts/2024-03-06-fsdp-qlora.html
PyTorch FSDP 是一种数据 / 模型并行技术,它可以跨 GPU 分割模型,减少内存需求,并能够更有效地训练更大的模型。Q-LoRA 是一种微调方法,它利用量化和低秩适配器来有效地减少计算需求和内存占用。
设置开发环境
第一步是安装 Hugging Face Libraries 以及 Pyroch,包括 trl、transformers 和 datasets 等库。trl 是建立在 transformers 和 datasets 基础上的一个新库,能让对开源大语言模型进行微调、RLHF 和对齐变得更容易。
# Install Pytorch for FSDP and FA/SDPA%pip install "torch==2.2.2" tensorboard# Install Hugging Face libraries%pip install--upgrade "transformers==4.40.0" "datasets==2.18.0" "accelerate==0.29.3" "evaluate==0.4.1" "bitsandbytes==0.43.1" "huggingface_hub==0.22.2" "trl==0.8.6" "peft==0.10.0"
接下来,登录 Hugging Face 获取 Llama 3 70b 模型。
创建和加载数据集
环境设置完成后,我们就可以开始创建和准备数据集了。微调用的数据集应该包含使用者想要解决的任务的示例样本。阅读《如何在 2024 年使用 Hugging Face 微调 LLM》可以进一步了解如何创建数据集。
文章地址:https://www.philschmid.de/fine-tune-llms-in-2024-with-trl#3-create-and-prepare-the-dataset
作者使用了 HuggingFaceH4/no_robots 数据集,这是一个包含 10,000 条指令和样本的高质量数据集,并且经过了高质量的数据标注。这些数据可用于有监督微调(SFT),使语言模型更好地遵循人类指令。no_robots 数据集以 OpenAI 发表的 InstructGPT 论文中描述的人类指令数据集为原型,并且主要由单句指令组成。
{"messages": [{"role": "system", "content": "You are..."}, {"role": "user", "content": "..."}, {"role": "assistant", "content": "..."}]}{"messages": [{"role": "system", "content": "You are..."}, {"role": "user", "content": "..."}, {"role": "assistant", "content": "..."}]}{"messages": [{"role": "system", "content": "You are..."}, {"role": "user", "content": "..."}, {"role": "assistant", "content": "..."}]}
no_robots 数据集中的 10,000 个样本,被分为 9,500 个训练样本和 500 个测试样本,其中有些样本不包含 system 信息。作者使用 datasets 库加载数据集,添加了缺失的 system 信息,并将它们保存到单独的 json 文件中。示例代码如下所示:
from datasets import load_dataset# Convert dataset to OAI messagessystem_message = """You are Llama, an AI assistant created by Philipp to be helpful and honest. Your knowledge spans a wide range of topics, allowing you to engage in substantive conversations and provide analysis on complex subjects."""def create_conversation(sample):if sample["messages"][0]["role"] == "system":return sampleelse:sample["messages"] = [{"role": "system", "content": system_message}] + sample["messages"]return sample# Load dataset from the hubdataset = load_dataset("HuggingFaceH4/no_robots")# Add system message to each conversationcolumns_to_remove = list(dataset["train"].features)columns_to_remove.remove("messages")dataset = dataset.map(create_conversation, remove_columns=columns_to_remove,batched=False)# Filter out conversations which are corrupted with wrong turns, keep which have even number of turns after adding system messagedataset["train"] = dataset["train"].filter(lambda x: len(x["messages"][1:]) % 2 == 0)dataset["test"] = dataset["test"].filter(lambda x: len(x["messages"][1:]) % 2 == 0)# save datasets to diskdataset["train"].to_json("train_dataset.json", orient="records", force_ascii=False)dataset["test"].to_json("test_dataset.json", orient="records", force_ascii=False)
使用 PyTorch FSDP、Q-Lora 和 SDPA 来微调 LLM
接下来使用 PyTorch FSDP、Q-Lora 和 SDPA 对大语言模型进行微调。作者是在分布式设备中运行模型,因此需要使用 torchrun 和 python 脚本启动训练。
作者编写了 run_fsdp_qlora.py 脚本,其作用是从磁盘加载数据集、初始化模型和分词器并开始模型训练。脚本使用 trl 库中的 SFTTrainer 来对模型进行微调。
SFTTrainer 能够让对开源大语言模型的有监督微调更加容易上手,具体来说有以下几点:
格式化的数据集,包括格式化的多轮会话和指令(已使用)只对完整的内容进行训练,忽略只有 prompts 的情况(未使用)打包数据集,提高训练效率(已使用)支持参数高效微调技术,包括 Q-LoRA(已使用)为会话级任务微调初始化模型和分词器(未使用,见下文)
注意:作者使用的是类似于 Anthropic/Vicuna 的聊天模板,设置了「用户」和「助手」角色。这样做是因为基础 Llama 3 中的特殊分词器(<|begin_of_text|> 及 <|reserved_special_token_XX|>)没有经过训练。
这意味着如果要在模板中使用这些分词器,还需要对它们进行训练,并更新嵌入层和 lm_head,对内存会产生额外的需求。如果使用者有更多的算力,可以修改 run_fsdp_qlora.py 脚本中的 LLAMA_3_CHAT_TEMPLATE 环境变量。
在配置参数方面,作者使用了新的 TrlParser 变量,它允许我们在 yaml 文件中提供超参数,或者通过明确地将参数传递给 CLI 来覆盖配置文件中的参数,例如 —num_epochs 10。以下是在 4x A10G GPU 或 4x24GB GPU 上微调 Llama 3 70B 的配置文件。
%%writefile llama_3_70b_fsdp_qlora.yaml# script parametersmodel_id: "meta-llama/Meta-Llama-3-70b" # Hugging Face model iddataset_path: "."# path to datasetmax_seq_len:3072 # 2048# max sequence length for model and packing of the dataset# training parametersoutput_dir: "./llama-3-70b-hf-no-robot" # Temporary output directory for model checkpointsreport_to: "tensorboard" # report metrics to tensorboardlearning_rate: 0.0002# learning rate 2e-4lr_scheduler_type: "constant"# learning rate schedulernum_train_epochs: 3# number of training epochsper_device_train_batch_size: 1 # batch size per device during trainingper_device_eval_batch_size: 1# batch size for evaluationgradient_accumulation_steps: 2 # number of steps before performing a backward/update passoptim: adamw_torch # use torch adamw optimizerlogging_steps: 10# log every 10 stepssave_strategy: epoch # save checkpoint every epochevaluation_strategy: epoch # evaluate every epochmax_grad_norm: 0.3 # max gradient normwarmup_ratio: 0.03 # warmup ratiobf16: true # use bfloat16 precisiontf32: true # use tf32 precisiongradient_checkpointing: true # use gradient checkpointing to save memory# FSDP parameters: https://huggingface.co/docs/transformers/main/en/fsdpfsdp: "full_shard auto_wrap offload" # remove offload if enough GPU memoryfsdp_config:backward_prefetch: "backward_pre"forward_prefetch: "false"use_orig_params: "false"
注意:训练结束时,GPU 内存使用量会略有增加(约 10%),这是因为模型保存所带来的开销。所以使用时,请确保 GPU 上有足够的内存来保存模型。
在启动模型训练阶段,作者使用 torchrun 来更加灵活地运用样本,并且易于被调整,就像 Amazon SageMaker 及 Google Cloud Vertex AI 一样。
对于 torchrun 和 FSDP,作者需要对环境变量 ACCELERATE_USE_FSDP 和 FSDP_CPU_RAM_EFFICIENT_LOADING 进行设置,来告诉 transformers/accelerate 使用 FSDP 并以节省内存的方式加载模型。
注意:如果想不使用 CPU offloading 功能,需要更改 fsdp 的设置。这种操作只适用于内存大于 40GB 的 GPU。
本文使用以下命令启动训练:
!ACCELERATE_USE_FSDP=1 FSDP_CPU_RAM_EFFICIENT_LOADING=1 torchrun --nproc_per_node=4 ./scripts/run_fsdp_qlora.py --config llama_3_70b_fsdp_qlora.yaml
预期内存使用情况:
- 使用 FSDP 进行全微调需要约 16 块 80GB 内存的 GPU
- FSDP+LoRA 需要约 8 块 80GB 内存的 GPU
- FSDP+Q-Lora 需要约 2 块 40GB 内存的 GPU
- FSDP+Q-Lora+CPU offloading 技术需要 4 块 24GB 内存的 GPU,以及一块具备 22 GB 内存的 GPU 和 127 GB 的 CPU RAM,序列长度为 3072、batch 大小为 1。
在 g5.12xlarge 服务器上,基于包含 1 万个样本的数据集,作者使用 Flash Attention 对 Llama 3 70B 进行 3 个 epoch 的训练,总共需要 45 小时。每小时成本为 5.67 美元,总成本为 255.15 美元。这听起来很贵,但可以让你在较小的 GPU 资源上对 Llama 3 70B 进行微调。
如果我们将训练扩展到 4x H100 GPU,训练时间将缩短至大约 125 小时。如果假设 1 台 H100 的成本为 5-10 美元 / 小时,那么总成本将在 25-50 美元之间。
我们需要在易用性和性能之间做出权衡。如果能获得更多更好的计算资源,就能减少训练时间和成本,但即使只有少量资源,也能对 Llama 3 70B 进行微调。对于 4x A10G GPU 而言,需要将模型加载到 CPU 上,这就降低了总体 flops,因此成本和性能会有所不同。
注意:在作者进行的评估和测试过程中,他注意到大约 40 个最大步长(将 80 个样本堆叠为长度为三千的序列)就足以获得初步结果。40 个步长的训练时间约为 1 小时,成本约合 5 美元。
可选步骤:将 LoRA 的适配器融入原始模型
使用 QLoRA 时,作者只训练适配器而不对整个模型做出修改。这意味着在训练过程中保存模型时,只保存适配器权重,而不保存完整模型。
如果使用者想保存完整的模型,使其更容易与文本生成推理器一起使用,则可以使用 merge_and_unload 方法将适配器权重合并到模型权重中,然后使用 save_pretrained 方法保存模型。这将保存一个默认模型,可用于推理。
注意:CPU 内存需要大于 192GB。
#### COMMENT IN TO MERGE PEFT AND BASE MODEL ##### from peft import AutoPeftModelForCausalLM# # Load PEFT model on CPU# model = AutoPeftModelForCausalLM.from_pretrained(# args.output_dir,# torch_dtype=torch.float16,# low_cpu_mem_usage=True,# )# # Merge LoRA and base model and save# merged_model = model.merge_and_unload()# merged_model.save_pretrained(args.output_dir,safe_serialization=True, max_shard_size="2GB")
模型测试和推理
训练完成后,我们要对模型进行评估和测试。作者从原始数据集中加载不同的样本,并手动评估模型。评估生成式人工智能模型并非易事,因为一个输入可能有多个正确的输出。阅读《评估 LLMs 和 RAG,一个使用 Langchain 和 Hugging Face 的实用案例》可以了解到关于评估生成模型的相关内容。
文章地址:https://www.philschmid.de/evaluate-llm
import torchfrom peft import AutoPeftModelForCausalLMfrom transformers import AutoTokenizerpeft_model_id = "./llama-3-70b-hf-no-robot"# Load Model with PEFT adaptermodel = AutoPeftModelForCausalLM.from_pretrained(peft_model_id,torch_dtype=torch.float16,quantization_config= {"load_in_4bit": True},device_map="auto")tokenizer = AutoTokenizer.from_pretrained(peft_model_id)
接下来加载测试数据集,尝试生成指令。
from datasets import load_datasetfrom random import randint# Load our test dataseteval_dataset = load_dataset("json", data_files="test_dataset.json", split="train")rand_idx = randint(0, len(eval_dataset))messages = eval_dataset[rand_idx]["messages"][:2]# Test on sampleinput_ids = tokenizer.apply_chat_template(messages,add_generation_prompt=True,return_tensors="pt").to(model.device)outputs = model.generate(input_ids,max_new_tokens=512,eos_token_id= tokenizer.eos_token_id,do_sample=True,temperature=0.6,top_p=0.9,)response = outputs[0][input_ids.shape[-1]:]print(f"**Query:**\n{eval_dataset[rand_idx]['messages'][1]['content']}\n")print(f"**Original Answer:**\n{eval_dataset[rand_idx]['messages'][2]['content']}\n")print(f"**Generated Answer:**\n{tokenizer.decode(response,skip_special_tokens=True)}")# **Query:**# How long was the Revolutionary War?# **Original Answer:**# The American Revolutionary War lasted just over seven years. The war started on April 19, 1775, and ended on September 3, 1783.# **Generated Answer:**# The Revolutionary War, also known as the American Revolution, was an 18th-century war fought between the Kingdom of Great Britain and the Thirteen Colonies. The war lasted from 1775 to 1783.
至此,主要流程就介绍完了,心动不如行动,赶紧从第一步开始操作吧。
以上是仅用250美元,Hugging Face技术主管手把手教你微调Llama 3的详细内容。更多信息请关注PHP中文网其他相关文章!

热AI工具

Undresser.AI Undress
人工智能驱动的应用程序,用于创建逼真的裸体照片

AI Clothes Remover
用于从照片中去除衣服的在线人工智能工具。

Undress AI Tool
免费脱衣服图片

Clothoff.io
AI脱衣机

Video Face Swap
使用我们完全免费的人工智能换脸工具轻松在任何视频中换脸!

热门文章

热工具

记事本++7.3.1
好用且免费的代码编辑器

SublimeText3汉化版
中文版,非常好用

禅工作室 13.0.1
功能强大的PHP集成开发环境

Dreamweaver CS6
视觉化网页开发工具

SublimeText3 Mac版
神级代码编辑软件(SublimeText3)

热门话题
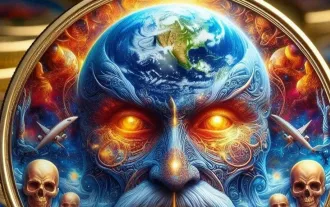
WorldCoin(WLD)凭借其独特的生物识别验证和隐私保护机制,在加密货币市场中脱颖而出,吸引了众多投资者的目光。 WLD凭借其创新技术,特别是结合OpenAI人工智能技术,在众多山寨币中表现突出。但未来几年,数字资产的走势如何呢?让我们一起预测WLD的未来价格。 2025年WLD价格预测预计2025年WLD将实现显着增长。市场分析显示,WLD平均价格可能达到1.31美元,最高可能触及1.36美元。然而,在熊市情况下,价格可能跌至0.55美元左右。这一增长预期主要源于WorldCoin2.
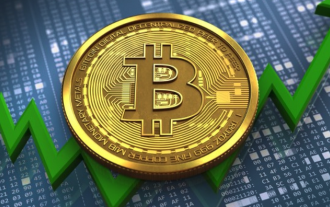
币安是全球数字资产交易生态的霸主,其特点包括:1. 日均交易量突破$1500亿,支持500 交易对,覆盖98%主流币种;2. 创新矩阵涵盖衍生品市场、Web3布局和教育体系;3. 技术优势为毫秒级撮合引擎,峰值处理量达140万笔/秒;4. 合规进展持有15国牌照,并在欧美设立合规实体。
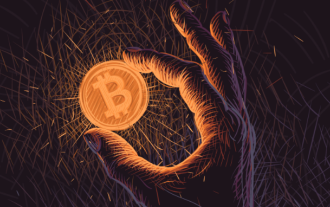
2025年在杠杆交易、安全性和用户体验方面表现突出的平台有:1. OKX,适合高频交易者,提供最高100倍杠杆;2. Binance,适用于全球多币种交易者,提供125倍高杠杆;3. Gate.io,适合衍生品专业玩家,提供100倍杠杆;4. Bitget,适用于新手及社交化交易者,提供最高100倍杠杆;5. Kraken,适合稳健型投资者,提供5倍杠杆;6. Bybit,适用于山寨币探索者,提供20倍杠杆;7. KuCoin,适合低成本交易者,提供10倍杠杆;8. Bitfinex,适合资深玩
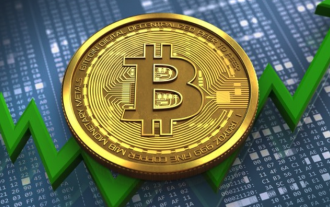
支持跨链交易的交易所有:1. Binance,2. Uniswap,3. SushiSwap,4. Curve Finance,5. Thorchain,6. 1inch Exchange,7. DLN Trade,这些平台通过各种技术支持多链资产交易。
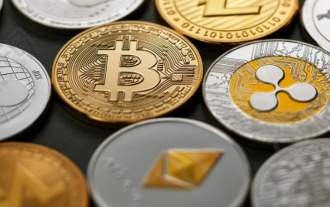
在当今的加密货币市场中,交易所扮演着至关重要的角色,它们不仅是投资者进行买卖交易的平台,更是市场流动性和价格发现的重要来源。全球最大的虚拟货币交易所排行前十,这些交易所不仅在交易量上遥遥领先,而且在用户体验、安全性和创新服务方面也各有千秋。排行榜首的交易所通常拥有庞大的用户基础和广泛的市场影响力,它们的交易量和资产种类往往是其他交易所难以企及的。
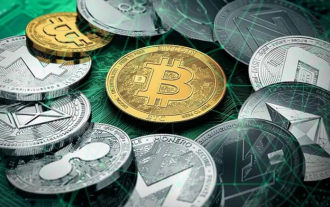
虚拟币价格上涨因素包括:1.市场需求增加,2.供应量减少,3.利好消息刺激,4.市场情绪乐观,5.宏观经济环境;下降因素包括:1.市场需求减少,2.供应量增加,3.利空消息打击,4.市场情绪悲观,5.宏观经济环境。
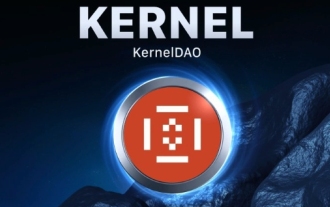
在加密货币的繁华世界里,新机遇总是不断涌现。当下,KernelDAO (KERNEL) 空投活动正备受瞩目,吸引着众多投资者的目光。那么,这个项目究竟是什么来头?BNB Holder 又能从中获得怎样的好处?别急,下面将为你一一揭晓。

Aavenomics是修改AAVE协议令牌并引入令牌回购的提议,已为AAVEDAO实现了一个法定人数。AAVE连锁计划(ACI)创始人马克·泽勒(MarcZeller)在X上宣布了这一点,并指出它标志着该协议的新时代。AAVE连锁倡议(ACI)创始人MarcZeller在X上宣布,Aavenomics提案包括修改AAVE协议令牌和引入令牌回购,已为AAVEDAO实现了法定人数。根据Zeller的说法,这标志着该协议的新时代。AaveDao成员以压倒性的投票支持该提议,即在周三以每周100
